ISLR(6)-压缩估计方法与正则化
Ridge & Lasso 在信用卡数据集的应用
笔记要点:
0.Shrinkage Method
1.Ridge Regression
-- scale control
2.Lasso
3.参考
0. Shrinkage Method
除了使用最小二乘法对包含预测变量子集的线性模型进行拟合, 还可以使用对系数进行约束或加罚的技巧对包含
- 引入这种约束会增加一点点误差, 但「显著减少了估计量方差」
1. Ridge Regression
在简单线性回归中, 通过最小化残差平方和RSS进行估计
岭回归的系数估计值通过最小化RSS+penalty的权衡得到
-
: 压缩惩罚(shrinkage penalty)
- it has the effect of shrinking the estimates of
towards zero
- it has the effect of shrinking the estimates of
-
: 调节参数 (tuning parameter)
-
controls the impact on the regression coefficient estimates
-
: the penalty grows, and all
will be minimized to 0
-
-
-
- 可以通过交叉验证选择合适的
- 可以通过交叉验证选择合适的
岭回归处理信用卡数据集
当
income
,
limit
,
rating
,
student
的岭回归系数估计值
「趋近0」
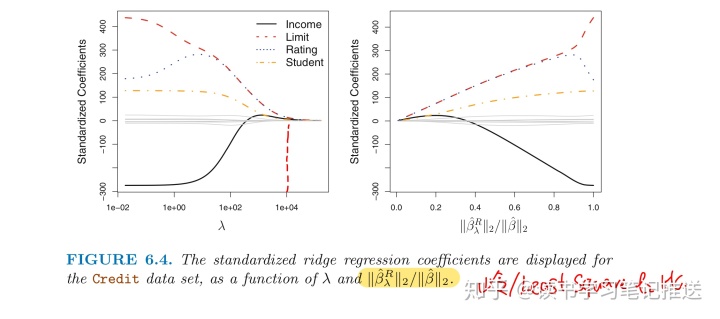
-
: the vector of
-
: the
norm」
from zero
- As
increases, the
norm of
will
0」
- and 「
will decrease」
- 「
norm ratio」
- a small value = close to zero
- and 「
「范围控制」
最小二乘估计是尺度不变的(scale invariant)
- Multiplying
by a constant
simply leads to a
」
- Regardless of how the
predictor is scaled,
will remain the same
岭回归的估计系数会随着预测变量的改变而显著改变:
- 因为岭回归公式中的
, 变量的扩大缩小倍数不能简单的改变系数估计值的变化
-
的最终预测结果不只是取决于
, 也取决于
的尺度
通过标准化将岭回归的所有变量缩到同一尺度:
- The denominator is the estimated standard deviation of the jth predictor among n samples
- 标准化后的变量标准差为1, 最后的拟合不再受变量尺度的影响
为什么岭回归提升最小二乘效果?
与最小二乘相比, 岭回归的优势在于它权衡了误差和方差
- as
increases, the flexibility of the ridge regression fit decreases,
- leading to slight increased bias
- but significant decreased variance
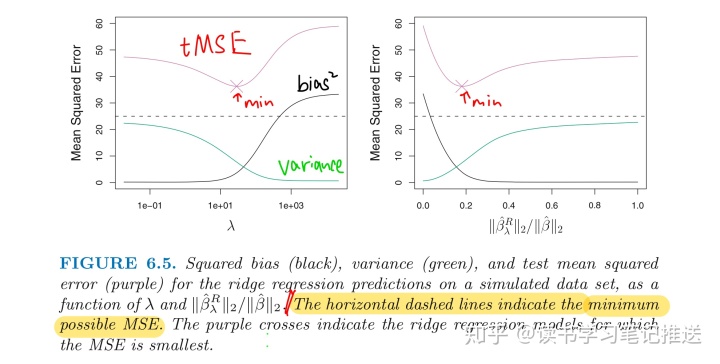
当响应变量和预测变量的关系接近线性时, 最小二乘估计会有较低的方差和「较大的方差」
- 训练集数据一个「微小的改变」可能导致最小二乘「系数的较大改变」
-
方差很大
-
最小二乘估计没有唯一解
- 岭回归通过偏差小幅上升来换取方差「大幅度下降」
-
「Ridge的优点」
Ridge regression has substantial computational advantages over best subset selection
- which requires searching through
models
For any fixed value of
- the model-fitting procedure can be performed as quickly as least squares
2. Lasso
「Ridge的劣势」
最优子集, 逐步方法会选择出变量的一个子集进行建模, 岭回归的最终模型包含全部p个变量
- 「
惩罚项」
「Lasso」可以克服岭回归的缺点:
「
- Lasso和最优子集法类似, 完成了变量选择
- Lasso建立的模型与岭回归相比, 更易于解释
- Sparse Model 稀疏模型: the model that involve only a subset of the variables
- 同样可以通过交叉验证的方法选择好的
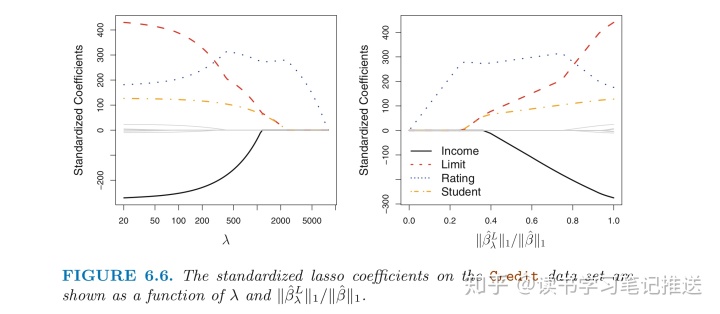
右图与岭回归的表现有很大的差异:
-
,
从1到0 (右=>左)
- Lasso首先和最小二乘一样, 然后
student
和limit
归零- 最后得到一个只包含
rating
的模型,
- 最后得到一个只包含
根据不同的
- 岭回归得到的模型自始至终包含所有变量, 虽然系数估计值的大小会随着
变化
4. 参考:
- 《Introduction to Statistical Learning》
- 《老董聊卡》