才发现TensorBoard这个工具也太好用了吧。可以很清楚的看到你构建的网络框架,比如输入变量输出变量,选取的激活函数等。
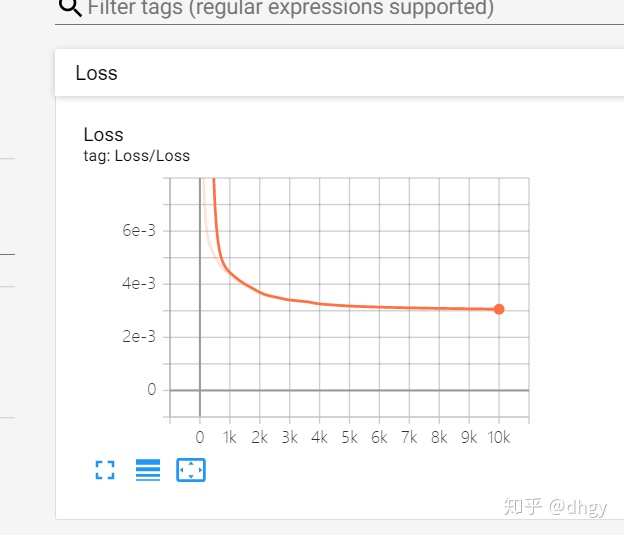
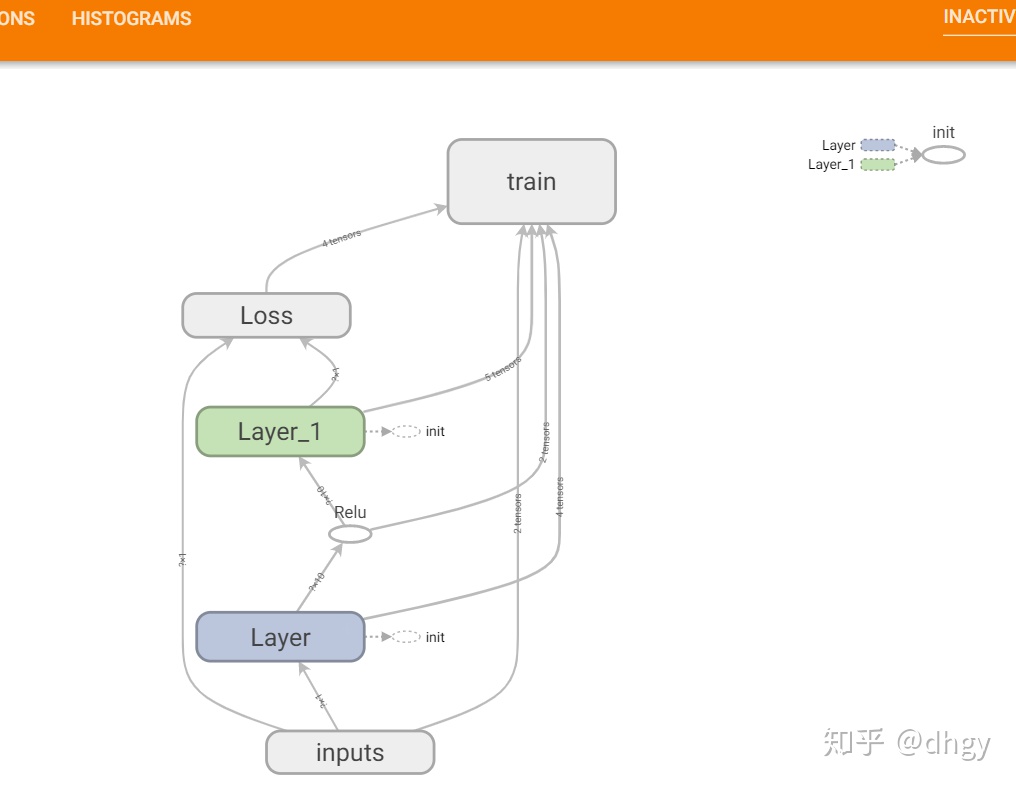
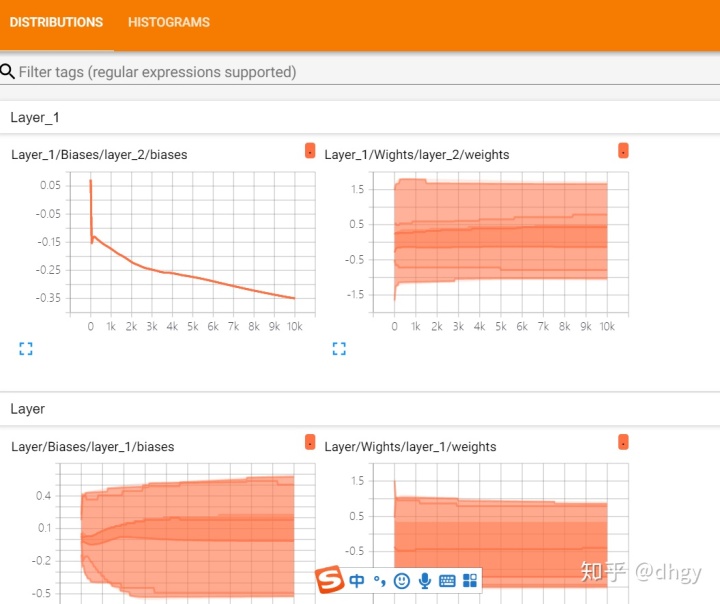
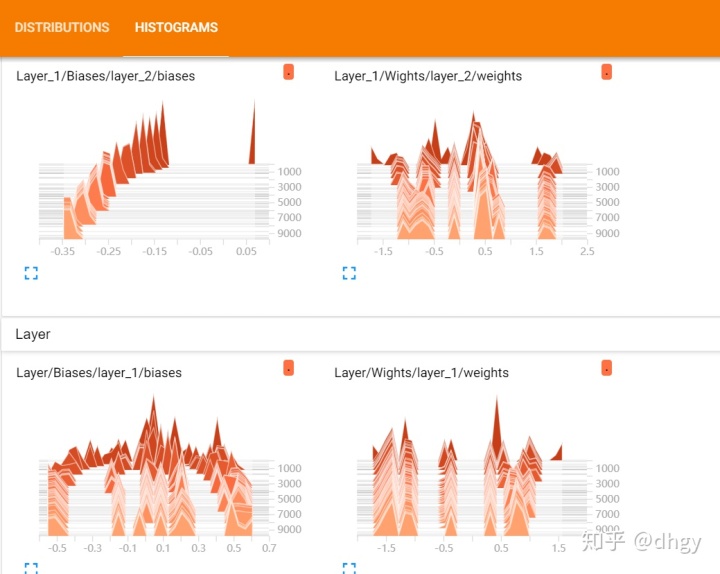
首先使用cmd打开命令行窗口,激活tensorflow 使用activate tensorflow

接着到你保存的路径文件夹下

接着就会出现地址类似这样的

复制这个地址在浏览器打开,然后就可以看到可视化结果了。
可是我的这个地址打不开,然后加了host就可以了

访问的网址:http://localhost:6006/ 或者http://127.0.0.1:6006/ 都可以了
生成流程图的代码如下:
import numpy as np
import tensorflow as tf
def add_layer(inputs, in_size, out_size, activation_function=None):
# 每使用该函数创建一层,则生成一个名为Layer_n的外层框
with tf.name_scope('Layer'):
# 内层权重框
with tf.name_scope('Wights'):
Weights = tf.Variable(tf.random_normal([in_size, out_size]))
# 内层Bias框
with tf.name_scope('Biases'):
biases = tf.Variable(tf.zeros([1, out_size]) + 0.1)
# 内层z(x,w,b)框
with tf.name_scope('Wx_plus_b'):
Wx_plus_b = tf.matmul(inputs, Weights) + biases
if activation_function is None:
outputs = Wx_plus_b
else:
outputs = activation_function(Wx_plus_b)
return outputs
# 准备数据
x_data = np.linspace(-1, 1, 300)[:, np.newaxis]
noise = np.random.normal(0, 0.05, x_data.shape)
y_data = np.square(x_data) - 0.5 + noise
# 使用tensorboard画inputs层
with tf.name_scope('inputs'): # 一个名为inputs的外层框
# x_input和y_input
xs = tf.placeholder(tf.float32, [None, 1], name='x_input')
ys = tf.placeholder(tf.float32, [None, 1], name='y_input')
l1 = add_layer(xs, 1, 10, activation_function=tf.nn.relu)
prediction = add_layer(l1, 10, 1, activation_function=None)
# Loss框,其中包含计算Loss的各个步骤,例如sub,square,sum,mean等
with tf.name_scope("Loss"):
loss = tf.reduce_mean(tf.reduce_sum(tf.square(ys - prediction),
reduction_indices=[1]))
# train框,其中包含梯度下降步骤和权重更新步骤
with tf.name_scope('train'):
train_step = tf.train.GradientDescentOptimizer(0.1).minimize(loss)
init = tf.compat.v1.global_variables_initializer()
with tf.Session() as sess:
# 将图写入文件夹logs
writer = tf.compat.v1.summary.FileWriter('logs/')
# 写入文件,名为events.out.tfevents.1561191707.06P2GHW85CAH236
writer.add_graph(sess.graph)
sess.run(init)