问题描述
- 求一个序列的最大子段和即最大连续子序列之和。例如序列[4, -3, 5, -2, -1, 2, 6, -2]的最大子段和为11=[4+(-3)+5+(-2)+(-1)+(2)+(6)]。
1. 蛮力算法
- 思想:从序列首元素开始穷举所有可能的子序列。
- 代码示例(C++):
#include<iostream>
using namespace std;
int MaxSubsequenceSum(const int array[], int n)
{
int tempSum, maxSum;
maxSum = 0;
for (int i = 0;i < n;i++) // 子序列起始位置
{
for (int j = i;j < n;j++) // 子序列终止位置
{
tempSum = 0;
for (int k = i;k < j;k++) // 子序列遍历求和
tempSum += array[k];
if (tempSum > maxSum) // 更新最大和值
maxSum = tempSum;
}
}
return maxSum;
}
int main()
{
const int a[] = { 4, -3, 5, -2, -1, 2, 6, -2 };
int maxSubSum = MaxSubsequenceSum(a, 8);
cout << "The max subsequence sum of a is: " << maxSubSum << endl;
system("pause");
return 0;
}
- 1
- 2
- 3
- 4
- 5
- 6
- 7
- 8
- 9
- 10
- 11
- 12
- 13
- 14
- 15
- 16
- 17
- 18
- 19
- 20
- 21
- 22
- 23
- 24
- 25
- 26
- 27
- 28
- 算法复杂度为O(n3)O(n3)
2. 改进的蛮力算法
- 思想:直接在划定子序列时累加元素值,减少一层循环。
- 代码示例(C++):
#include<iostream>
using namespace std;
int MaxSubsequenceSum(const int array[],int n)
{
int tempSum, maxSum;
maxSum = 0;
for (int i = 0;i < n;i++)
{
tempSum = 0;
for (int j = i;j < n;j++)
{
tempSum += array[j];
if (tempSum > maxSum)
maxSum = tempSum;
}
}
return maxSum;
}
int main()
{
const int a[] = { 4, -3, 5, -2, -1, 2, 6, -2 };
int maxSubSum = MaxSubsequenceSum(a, 8);
cout << "The max subsequence sum of a is: " << maxSubSum << endl;
system("pause");
return 0;
}
- 1
- 2
- 3
- 4
- 5
- 6
- 7
- 8
- 9
- 10
- 11
- 12
- 13
- 14
- 15
- 16
- 17
- 18
- 19
- 20
- 21
- 22
- 23
- 24
- 25
- 26
- 27
- 算法复杂度为O(n2)O(n2)
3. 分治递归的算法
- 思想:将序列划分为左右两部分,则最大子段和可能在三处出现:左半部、右半部以及跨越左右边界的部分。递归的终止条件是:left == right。
- 代码示例:
#include<iostream>
using namespace std;
int max3(int a, int b, int c) // 求三个数的最大值
{
int max = a;
if (b > max)
max = b;
if (c > max)
max = c;
return max;
}
int MaxSubsequenceSum(const int array[], int left, int right)
{
if (left == right) // 设置基准,即递归终止条件
return array[left];
int middle = (left + right) / 2;
int leftMaxSubsequenceSum = MaxSubsequenceSum(array, left, middle); // 求左半部分最大子序列和
int rightMaxSubsquenceSum = MaxSubsequenceSum(array, middle + 1, right); // 求右半部分最大子序列和
// 处理左右边界问题:最大子序列跨越中间,包含左半部分最右一个数,同时包含右半部分最左一个数
int maxLeftBorderSum = 0;
int maxRightBorderSum = 0;
int tempSum = 0; // 临时求和变量
for (int i = middle;i >= left;i--)
{
tempSum += array[i];
if (tempSum > maxLeftBorderSum)
maxLeftBorderSum = tempSum; // 左边包含边界最大序列和
}
tempSum = 0;
for (int i = middle + 1;i < right;i++)
{
tempSum += array[i];
if (tempSum > maxRightBorderSum)
maxRightBorderSum = tempSum; // 右边包含边界最大序列和
}
int maxBorderSum = maxRightBorderSum + maxLeftBorderSum; // 最大边界子序列和等于两部分边界之和
return max3(leftMaxSubsquenceSum, maxBorderSum, rightMaxSubsquenceSum); // 返回三个部分的最大子序列和
}
int main()
{
const int a[] = { 4, -3, 5, -2, -1, 2, 6, -2 };
int maxSubSum = MaxSubsequenceSum(a, 0, 7);
cout << "The max subsequence sum of a is: " << maxSubSum << endl;
system("pause");
return 0;
}
- 1
- 2
- 3
- 4
- 5
- 6
- 7
- 8
- 9
- 10
- 11
- 12
- 13
- 14
- 15
- 16
- 17
- 18
- 19
- 20
- 21
- 22
- 23
- 24
- 25
- 26
- 27
- 28
- 29
- 30
- 31
- 32
- 33
- 34
- 35
- 36
- 37
- 38
- 39
- 40
- 41
- 42
- 43
- 44
- 45
- 46
- 47
- 48
- 49
- 50
- 51
- 52
算法复杂度分析:假设求解NN
递归算法的基本准则:
- (1) 基准情形:存在最小子问题的解,也称为递归终止的条件。
- (2) 不断推进:每一次递归调用都要使得求解状况不断地朝基准情形方向推进。
- (3) 设计法则:假设所有递归调用都能运行。
- (4) 合成效益法则:在求解一个问题的同一实例式,要避免在不同的递归调用中做重复的工作。如:递归求斐波那契数就是一个不好的例子。
4. 动态规划的算法
- 原问题:考虑最大子段和原问题:给定nn
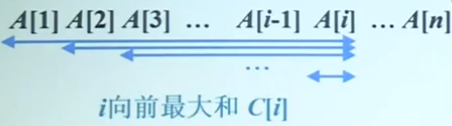
- 递推方程满足:
C[i]=max{C[i−1]+A[i], A[i]}i=2,...,nC[1]={A[1] ifA[1]>00 ifA[1]<0 C[i]=max{C[i−1]+A[i], A[i]}i=2,...,nC[1]={A[1] ifA[1]>00 ifA[1]<0
- 代码示例:
#include<iostream>
using namespace std;
int MaxSubsequenceSum(const int A[], int n)
{
int tempSum = 0;
int maxSum = 0;
for (int j = 0;j < n;j++) // 子问题后边界
{
tempSum = (tempSum + A[j]) > A[j] ? (tempSum + A[j]) : A[j];
if (tempSum > maxSum) // 更新最大和
maxSum = tempSum;
}
return maxSum;
}
int main()
{
const int a[] = { 4, -3, 5, -2, -1, 2, 6, -2 };
int maxSubSum = MaxSubsequenceSum(a, 8);
cout << "The max subsequence sum of a is: " << maxSubSum << endl;
system("pause");
return 0;
}
- 1
- 2
- 3
- 4
- 5
- 6
- 7
- 8
- 9
- 10
- 11
- 12
- 13
- 14
- 15
- 16
- 17
- 18
- 19
- 20
- 21
- 22
- 23
- 24
- 25
- 算法复杂度为O(n)O(n)。
- 动态规划算法设计要点:
- (1) (划分)多阶段决策过程,每步处理一个子问题,界定子问题的边界(初值问题)。
- (2) 列出优化函数的递推方程及初值(无比关键)。
- (3) 问题要满足优化原则或者最优子结构性质。即:一个最优决策序列的任何子序列本身一定是相对于子序列的初始和结束状态的最优决策序列。
- 更多关于动态优化的理解参考知乎回答:什么是动态规划?动态规划的意义是什么?
参考资料
Mark Allen Weiss: 数据结构与算法分析
屈婉玲教授 :【北大公开课】 算法设计与分析