#include <iostream>
#include <vector>
#include <string>
#include <iomanip>
int main() {
int n, m;
// 输入矩阵的数字个数n和行数m
std::cin >> n >> m;
// 计算矩阵的列数cols,使用 std::vector 初始化矩阵
int cols = n / m + 1;
std::vector<std::vector<std::string>> matrix(m, std::vector<std::string>(cols, " "));
int num = 1, row = 0, col = 0;
while (num <= n) {
// 向右填充
while (0 <= col && col < cols && matrix[row][col] == " ") {
matrix[row][col] = (num <= n) ? std::to_string(num) : "*";
num++, col++;
}
// std::cout << "col = " << col << std::endl;
row++, col--;
// 向下填充
while (0 <= row && row < m && matrix[row][col] == " ") {
matrix[row][col] = (num <= n) ? std::to_string(num) : "*";
num++, row++;
}
row--, col--;
// 向左填充
if (0 <= row && row < m) {
while (0 <= col && col < cols && matrix[row][col] == " ") {
matrix[row][col] = (num <= n) ? std::to_string(num) : "*";
num++, col--;
}
col++, row--;
}
// 向上填充
if (0 <= col && col < cols) {
while (0 <= row && row < m && matrix[row][col] == " ") {
matrix[row][col] = (num <= n) ? std::to_string(num) : "*";
num++, row--;
}
row++, col++;
}
}
// 输出矩阵
for (const auto &rows: matrix) {
for (const auto &elem: rows) {
std::cout << std::setw(3) << std::right << elem << " ";
}
std::cout << std::endl;
}
return 0;
}
OD_01
最新推荐文章于 2024-10-06 14:42:09 发布
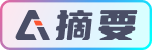