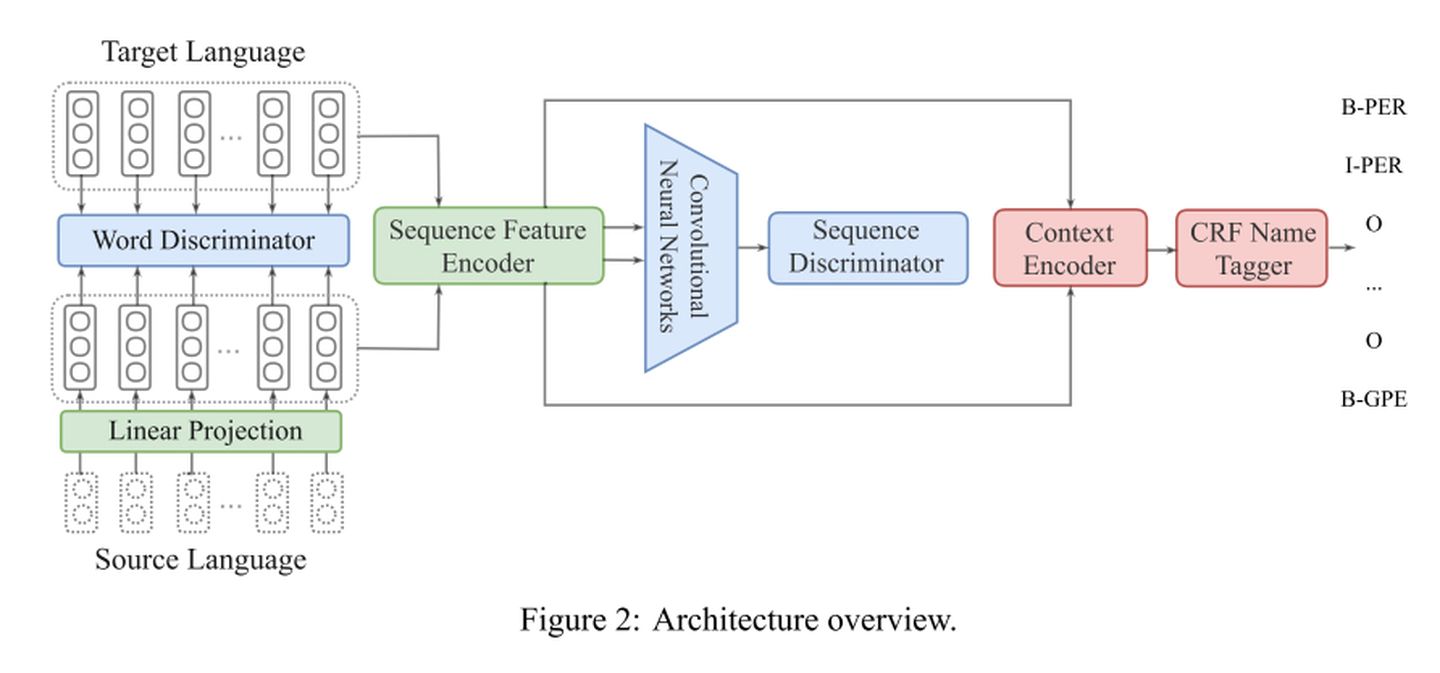
Cross-lingual Multi-Level Adversarial Transfer to Enhance Low-Resource Name Tagging 论文笔记
原文 | Github
原文是 NAACL 2019 的长文,最近因工作需要略读了此文,并将文章中部分内容整理成笔记,有需要的朋友可以通过本文,快速了解原文的。
原文的任务是解决多语言中低资源语言的 Name Tagging 任务,文章认为现有的两大方法都会在传递知识时引入噪声。故此提出了一套多层次对抗训练的学习框架。一方面,在单词级别将源语言词汇和目标语言词汇映射到相同的语义空间中;另一方面,在句子级别训练语言无关的序列特征编码器。通过 Multi-Level 的对抗训练实现更加有效的知识迁移。
具体的实现流程本文不多作赘述,重点是其结合两种层次的对抗训练,设计合理并且有效提升了模型的效果,对相关工作大有裨益。
Abstract
We focus on improving name tagging for low-resource languages using annotations from related languages. Previous studies either directly project annotations from a source language to a target language using cross-lingual representations or use a shared encoder in a multitask network to transfer knowledge. These approaches inevitably introduce noise to the target language annotation due to mismatched source-target sentence structures. To effectively transfer the resources, we develop a new neural architecture that leverages multi-level adversarial transfer: (1) word-level adversarial training, which projects source language words into the same semantic space as those of the target language without using any parallel corpora or bilingual gazett