Medical Image Analysis -- An Important Application in the Field of Image Analysis
Author: Zhikang Zhang
目录
Image registration and information fusion
Functional analysis of sequential images
3.Functional development and application
1.Background
In the freshman seminar of last semester, professor Zeng Bing explained and popularized the principle and application of image analysis and video processing in detail, which aroused my keen interest in medical image analysis in image processing.
Medical image analysis attempts to transform medical simulation image into digital image, and carries out preliminary research on computer aided diagnosis (CAD), which is intended to assist doctors in medical image interpretation to a certain extent, and to eliminate human subjective factors, so as to improve diagnostic accuracy and efficiency. The interdisciplinary fields of medical image analysis, comprehensive medical image, mathematical modeling, digital image processing and analysis, artificial intelligence and numerical algorithm, etc.
2.Theory
Image registration and information fusion
The purpose of early medical image registration is to display medical images showing different information of human body (structural information and functional information) in a unified coordinate system. With the development of morphological analysis of brain structure, image data registration of patients with different periods and the same disease and standard atlas to specific image data sets have emerged. In order to eliminate the influence of object motion in the imaging process, image registration is also the first step of sequential image analysis. It is the basic task of medical image registration to find the relationship between the corresponding pixels in different images. By looking for appropriate spatial transformation, the image data can be located and registered in spatial position, and then the image fusion can be carried out. Image registration methods can be divided into two categories: external feature based and internal feature based. Registration is achieved by manually setting markers with external features, such as three-dimensional frame positioning, skin labeling, etc. Based on the internal feature method, registration is achieved by finding the feature points on the internal anatomical structure of the image or the corresponding relations between the external contour and the surface. Mutual information is a measure of statistical correlation of random variables and has been widely used in medical image registration. Since the maximum mutual information method does not need to assume the correlation of image grayscale under different imaging modes, nor does it need to segment and preprocess images, it is very suitable for 3d multi-mode medical image registration and has the characteristics of high precision and strong robustness. At present, medical image registration technology and segmentation technology, there are many mature methods and software, is one of the basic technology of medical image analysis.
Visualization
Visualization plays an important role in medical image analysis. Medical image visualization technology is the use of obtained from the experiment, scanner measured, calculating model of synthetic data of medical images, three-dimensional image reconstruction model, qualitative and quantitative analysis, to provide users with realistic 3 d medical image, make people more clear understanding of the complex structure of implication in vivo data, observation and analysis, the doctor multi-angle multi-level to doctors and able to participate effectively in the process of data processing and analysis. Medical image visualization technology, according to the different methods of data description in the rendering process, is usually divided into two categories: one-side rendering and volume rendering. Surface rendering technology refers to the reconstruction of the volume surface, that is, extracting the equivalent surface from the three-dimensional data field provided by slicing data, and then using traditional graphics technology to achieve surface rendering. Surface rendering can effectively draw the surface of an object, but it lacks the expression of the internal information of the object. Volume rendering takes voxel as the basic unit to generate 3d object images directly from slicing data to represent the internal information of the object. However, it requires a lot of calculation. In addition, the reconstruction algorithms that combine the characteristics of these two technologies are classified into the third category -- mixed rendering technology.
Volume rendering technology does not need to construct intermediate objects, but directly reconstruct objects from 3d data itself. Each data in 3d data is regarded as a basic unit of entity representation -- voxel. Each voxel has corresponding properties such as color, opacity, gradient and so on. The basic principle of volume rendering is to simulate the cumulative change of energy when light passes through the translucent material, that is, the theory of light transmission. Specifically, each voxel is first assigned an opacity and color value (R, G, B); Then the illumination intensity of voxel was calculated according to the gradient of each voxel and the illumination model. Then, according to the light model, the opacity and color value of each voxel projected to the same pixel point in the image plane are combined to generate the final image. Typical algorithms of volume rendering technology include ray projection method, footprint method, cross-cutting deformation method, hardware-based 3d texture mapping, frequency-domain volume rendering method, wavelet-based volume rendering method, etc.
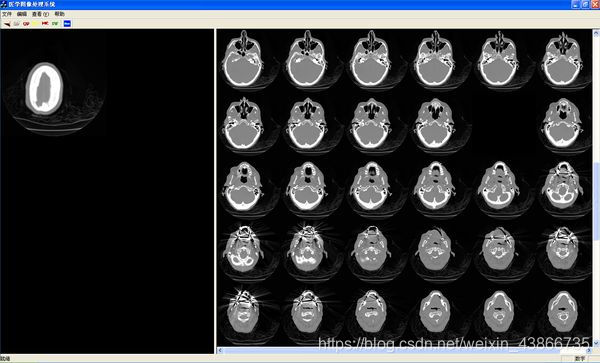
Functional analysis of sequential images
The purpose of medical image analysis is to obtain quantitative information of physiological process and provide more sufficient basis for clinical diagnosis and treatment. In the past, medical imaging mainly focused on anatomical structure imaging and morphological analysis. In recent years, rapid advances in imaging speed of medical devices have made it possible to observe the dynamics of physiological processes. For example, Functional MRI has been widely used in the field of brain Functional imaging. PET(positron emission tomography) and SPECT(single photon emission computer tomography) have been extensively applied in dynamic imaging of brain and heart functions. In terms of MR heart function imaging, Tagging MR technology and phase contrast MR imaging technology provide a basis for quantitatively analyzing the heart function of the image in physics. In addition, the advent of three-dimensional ultrasound provides a means to observe ventricular and valvular motion in real time. All the above devices can generate two-dimensional or three-dimensional medical images of time series, reflecting the dynamic functional information of physiological processes. The corresponding medical image analysis also develops from image segmentation and registration technology in the category of image processing to the accurate and quantitative analysis of organ functions and physiological processes with medical images as the media.
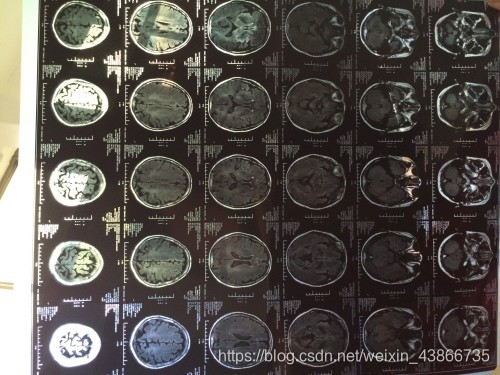
3.Functional development and application
Content-based image retrieval has made some progress in natural image retrieval and video retrieval. By extracting the features of the input image, a fast retrieval method for the retrieval of adjacent similar images in the feature space in the image database has been proposed. With the increasing trend of medical data quantization, the research and application of content-based medical image retrieval have become a hot topic in recent years. Image retrieval system can help doctors quickly find medical images with similar pathological features and have been diagnosed in massive databases, and improve the accuracy of disease diagnosis. Medical image retrieval has the following difficulties: first, the content and characteristics of medical images obtained by different imaging devices vary greatly, so it is difficult to use unified algorithm to automatically analyze and extract features; Secondly, the classification of medical images involves professional knowledge. For example, to distinguish different types of brain tumor images, specialized imaging knowledge must be combined in some form. Thirdly, modern medical images are mostly three-dimensional or higher dimensional, which also poses new challenges to feature extraction and retrieval algorithms. The difficulties mentioned above make the current image retrieval research focus on medical images targeted at specific imaging devices and specific anatomical locations, or disease types. At present, most studies are limited to theoretical studies, and only a few image retrieval systems have reported the clinical evaluation performance and practical application.