本文结合Deep learning的一个应用,Convolution Neural Network 进行一些基本应用,参考Lecun的Document 0.1进行部分拓展,与结果展示(in Python)。
分为以下几部分:
1. Convolution(卷积)
2. Pooling(降采样过程)
3. CNN结构
4. 跑实验
下面分别介绍。
PS:本篇blog为ese机器学习短期班参考资料(20140516课程),本文只是简要讲最naive最simple的思想,重在实践部分,原理课上详述。
1. Convolution(卷积)
类似于高斯卷积,对imagebatch中的所有image进行卷积。对于一张图,其所有feature map用一个filter卷成一张feature map。 如下面的代码,对一个imagebatch(含两张图)进行操作,每个图初始有3张feature map(R,G,B), 用两个9*9的filter进行卷积,结果是,每张图得到两个feature map。
卷积操作由theano的conv.conv2d实现,这里我们用随机参数W,b。结果有点像edge detector是不是?
Code: (详见注释)
-
-
-
-
-
-
-
-
-
-
-
- from theano.tensor.nnet import conv
- import theano.tensor as T
- import numpy, theano
-
-
- rng = numpy.random.RandomState(23455)
-
-
- input = T.tensor4(name = 'input')
-
-
- w_shape = (2,3,9,9)
- w_bound = numpy.sqrt(3*9*9)
- W = theano.shared(numpy.asarray(rng.uniform(low = -1.0/w_bound, high = 1.0/w_bound,size = w_shape),
- dtype = input.dtype),name = 'W')
-
- b_shape = (2,)
- b = theano.shared(numpy.asarray(rng.uniform(low = -.5, high = .5, size = b_shape),
- dtype = input.dtype),name = 'b')
-
- conv_out = conv.conv2d(input,W)
-
-
-
-
-
-
- output = T.nnet.sigmoid(conv_out + b.dimshuffle('x',0,'x','x'))
- f = theano.function([input],output)
-
-
-
-
-
-
- import pylab
- from PIL import Image
-
-
-
- img1 = Image.open(open('//home//rachel//Documents//ZJU_Projects//DL//Dataset//rachel.jpg'))
- width1,height1 = img1.size
- img1 = numpy.asarray(img1, dtype = 'float32')/256.
-
-
- img1_rgb = img1.swapaxes(0,2).swapaxes(1,2).reshape(1,3,height1,width1)
-
-
-
- img2 = Image.open(open('//home//rachel//Documents//ZJU_Projects//DL//Dataset//rachel1.jpg'))
- width2,height2 = img2.size
- img2 = numpy.asarray(img2,dtype = 'float32')/256.
- img2_rgb = img2.swapaxes(0,2).swapaxes(1,2).reshape(1,3,height2,width2)
-
-
-
-
- minibatch_img = numpy.concatenate((img1_rgb,img2_rgb),axis = 0)
- filtered_img = f(minibatch_img)
-
-
-
- pylab.subplot(2,3,1);pylab.axis('off');
- pylab.imshow(img1)
-
- pylab.subplot(2,3,4);pylab.axis('off');
- pylab.imshow(img2)
-
- pylab.gray()
- pylab.subplot(2,3,2); pylab.axis("off")
- pylab.imshow(filtered_img[0,0,:,:])
-
- pylab.subplot(2,3,3); pylab.axis("off")
- pylab.imshow(filtered_img[0,1,:,:])
-
- pylab.subplot(2,3,5); pylab.axis("off")
- pylab.imshow(filtered_img[1,0,:,:])
-
- pylab.subplot(2,3,6); pylab.axis("off")
- pylab.imshow(filtered_img[1,1,:,:])
- pylab.show()
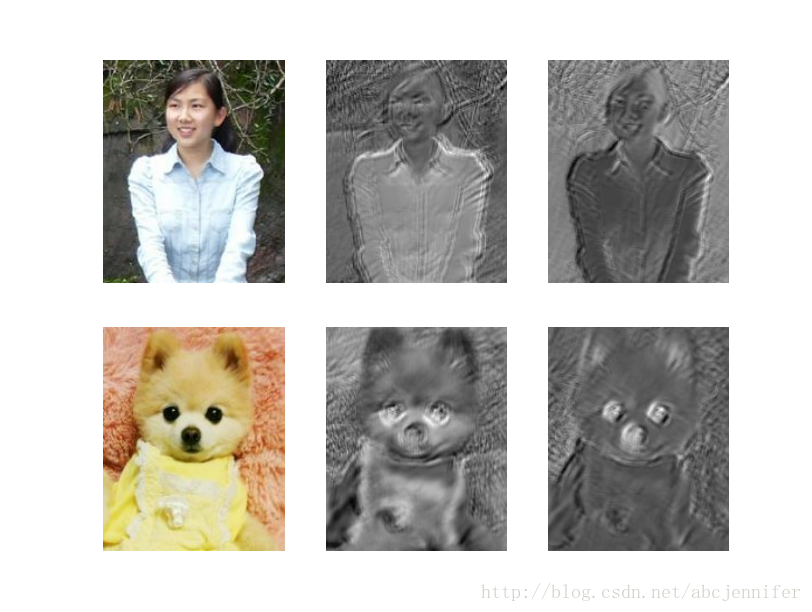
2. Pooling(降采样过程)
最常用的Maxpooling. 解决了两个问题:
1. 减少计算量
2. 旋转不变性 (原因自己悟)
PS:对于旋转不变性,回忆下SIFT,LBP:采用主方向;HOG:选择不同方向的模版
Maxpooling的降采样过程会将feature map的长宽各减半。(下面结果图中没有体现出来,python自动给拉到一样大了,但实际上像素数是减半的)
Code: (详见注释)
-
-
-
-
-
-
-
-
-
-
-
- from theano.tensor.nnet import conv
- import theano.tensor as T
- import numpy, theano
-
-
- rng = numpy.random.RandomState(23455)
-
-
- input = T.tensor4(name = 'input')
-
-
- w_shape = (2,3,9,9)
- w_bound = numpy.sqrt(3*9*9)
- W = theano.shared(numpy.asarray(rng.uniform(low = -1.0/w_bound, high = 1.0/w_bound,size = w_shape),
- dtype = input.dtype),name = 'W')
-
- b_shape = (2,)
- b = theano.shared(numpy.asarray(rng.uniform(low = -.5, high = .5, size = b_shape),
- dtype = input.dtype),name = 'b')
-
- conv_out = conv.conv2d(input,W)
-
-
-
-
-
-
- output = T.nnet.sigmoid(conv_out + b.dimshuffle('x',0,'x','x'))
- f = theano.function([input],output)
-
-
-
-
-
-
- import pylab
- from PIL import Image
- from matplotlib.pyplot import *
-
-
- img = Image.open(open('//home//rachel//Documents//ZJU_Projects//DL//Dataset//rachel.jpg'))
- width,height = img.size
- img = numpy.asarray(img, dtype = 'float32')/256.
-
-
-
- img_rgb = img.swapaxes(0,2).swapaxes(1,2)
- minibatch_img = img_rgb.reshape(1,3,height,width)
- filtered_img = f(minibatch_img)
-
-
-
- pylab.figure(1)
- pylab.subplot(1,3,1);pylab.axis('off');
- pylab.imshow(img)
- title('origin image')
-
- pylab.gray()
- pylab.subplot(2,3,2); pylab.axis("off")
- pylab.imshow(filtered_img[0,0,:,:])
- title('convolution 1')
-
- pylab.subplot(2,3,3); pylab.axis("off")
- pylab.imshow(filtered_img[0,1,:,:])
- title('convolution 2')
-
-
-
-
-
-
-
- from theano.tensor.signal import downsample
-
- input = T.tensor4('input')
- maxpool_shape = (2,2)
- pooled_img = downsample.max_pool_2d(input,maxpool_shape,ignore_border = False)
-
- maxpool = theano.function(inputs = [input],
- outputs = [pooled_img])
-
- pooled_res = numpy.squeeze(maxpool(filtered_img))
-
- pylab.subplot(235);pylab.axis('off');
- pylab.imshow(pooled_res[0,:,:])
- title('down sampled 1')
-
- pylab.subplot(236);pylab.axis('off');
- pylab.imshow(pooled_res[1,:,:])
- title('down sampled 2')
-
- pylab.show()
3. CNN结构
想必大家随便google下CNN的图都滥大街了,这里拖出来那时候学CNN的时候一张图,自认为陪上讲解的话画得还易懂(<!--囧-->)
废话不多说了,直接上Lenet结构图:(从下往上顺着箭头看,最下面为底层original input)
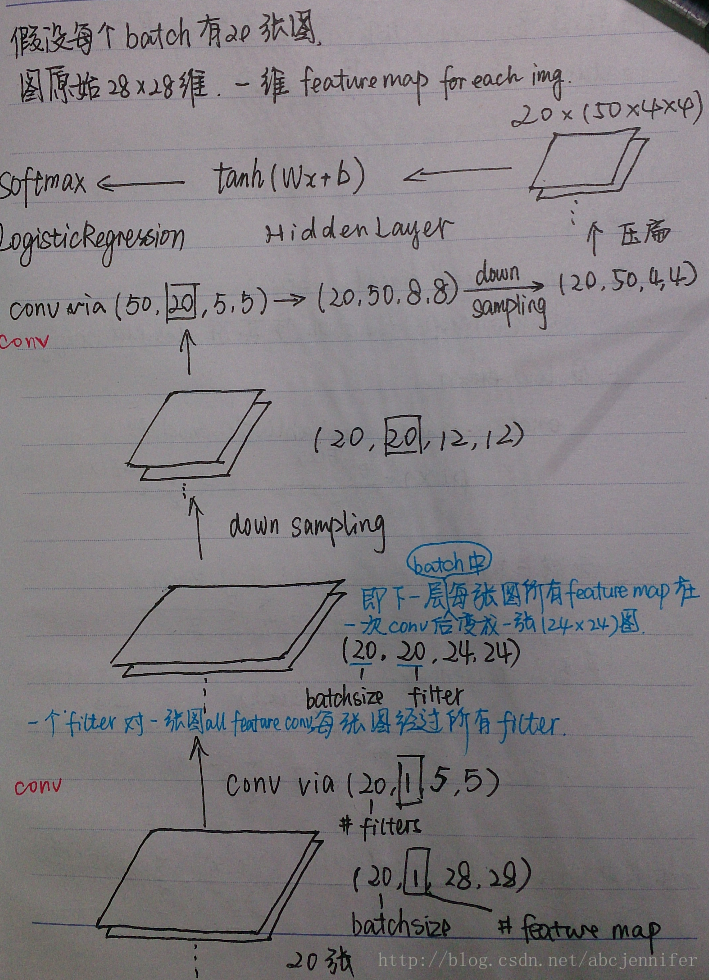
4. CNN代码
去资源里下载吧,我放上去了喔~(in python)
这里贴少部分代码,仅表示建模的NN:
- rng = numpy.random.RandomState(23455)
-
-
-
- N_filters_0 = 20
- D_features_0= 1
- layer0_input = x.reshape((batch_size,D_features_0,28,28))
- layer0 = LeNetConvPoolLayer(rng, input = layer0_input, filter_shape = (N_filters_0,D_features_0,5,5),
- image_shape = (batch_size,1,28,28))
-
-
- N_filters_1 = 50
- D_features_1 = N_filters_0
- layer1 = LeNetConvPoolLayer(rng,input = layer0.output, filter_shape = (N_filters_1,D_features_1,5,5),
- image_shape = (batch_size,N_filters_0,12,12))
-
-
- layer2_input = layer1.output.flatten(2)
- layer2 = HiddenLayer(rng,layer2_input,n_in = 50*4*4,n_out = 500, activation = T.tanh)
-
- layer3 = LogisticRegression(input = layer2.output, n_in = 500, n_out = 10)
layer0, layer1 :分别是卷积+降采样
layer2+layer3:组成一个MLP(ANN)
训练模型:
- cost = layer3.negative_log_likelihood(y)
- params = layer3.params + layer2.params + layer1.params + layer0.params
- gparams = T.grad(cost,params)
-
- updates = []
- for par,gpar in zip(params,gparams):
- updates.append((par, par - learning_rate * gpar))
-
- train_model = theano.function(inputs = [minibatch_index],
- outputs = [cost],
- updates = updates,
- givens = {x: train_set_x[minibatch_index * batch_size : (minibatch_index+1) * batch_size],
- y: train_set_y[minibatch_index * batch_size : (minibatch_index+1) * batch_size]})
根据cost(最上层MLP的输出NLL),对所有层的parameters进行训练
剩下的具体见代码和注释。
PS:数据为MNIST所有数据