for _ in range(n_layers):
layers.append(block())
return nn.Sequential(\*layers)
class ResidualDenseBlock_5C(nn.Module):
def __init__(self, nf=64, gc=32, bias=True):
super(ResidualDenseBlock_5C, self).init()
# gc: growth channel, i.e. intermediate channels
self.conv1 = nn.Conv2d(nf, gc, 3, 1, 1, bias=bias)
self.conv2 = nn.Conv2d(nf + gc, gc, 3, 1, 1, bias=bias)
self.conv3 = nn.Conv2d(nf + 2 * gc, gc, 3, 1, 1, bias=bias)
self.conv4 = nn.Conv2d(nf + 3 * gc, gc, 3, 1, 1, bias=bias)
self.conv5 = nn.Conv2d(nf + 4 * gc, nf, 3, 1, 1, bias=bias)
self.lrelu = nn.LeakyReLU(negative_slope=0.2, inplace=True)
# initialization
# mutil.initialize\_weights([self.conv1, self.conv2, self.conv3, self.conv4, self.conv5], 0.1)
def forward(self, x):
x1 = self.lrelu(self.conv1(x))
x2 = self.lrelu(self.conv2(torch.cat((x, x1), 1)))
x3 = self.lrelu(self.conv3(torch.cat((x, x1, x2), 1)))
x4 = self.lrelu(self.conv4(torch.cat((x, x1, x2, x3), 1)))
x5 = self.conv5(torch.cat((x, x1, x2, x3, x4), 1))
return x5 \* 0.2 + x
class RRDB(nn.Module):
‘’‘Residual in Residual Dense Block’‘’
def \_\_init\_\_(self, nf, gc=32):
super(RRDB, self).__init__()
self.RDB1 = ResidualDenseBlock_5C(nf, gc)
self.RDB2 = ResidualDenseBlock_5C(nf, gc)
self.RDB3 = ResidualDenseBlock_5C(nf, gc)
def forward(self, x):
out = self.RDB1(x)
out = self.RDB2(out)
out = self.RDB3(out)
return out \* 0.2 + x
class RRDBNet(nn.Module):
def __init__(self, in_nc, out_nc, nf, nb, gc=32):
super(RRDBNet, self).init()
RRDB_block_f = functools.partial(RRDB, nf=nf, gc=gc)
self.conv_first = nn.Conv2d(in_nc, nf, 3, 1, 1, bias=True)
self.RRDB_trunk = make_layer(RRDB_block_f, nb)
self.trunk_conv = nn.Conv2d(nf, nf, 3, 1, 1, bias=True)
#### upsampling
self.upconv1 = nn.Conv2d(nf, nf, 3, 1, 1, bias=True)
self.upconv2 = nn.Conv2d(nf, nf, 3, 1, 1, bias=True)
self.HRconv = nn.Conv2d(nf, nf, 3, 1, 1, bias=True)
self.conv_last = nn.Conv2d(nf, out_nc, 3, 1, 1, bias=True)
self.lrelu = nn.LeakyReLU(negative_slope=0.2, inplace=True)
def forward(self, x):
fea = self.conv_first(x)
trunk = self.trunk_conv(self.RRDB_trunk(fea))
fea = fea + trunk
fea = self.lrelu(self.upconv1(F.interpolate(fea, scale_factor=2, mode='nearest')))
fea = self.lrelu(self.upconv2(F.interpolate(fea, scale_factor=2, mode='nearest')))
out = self.conv_last(self.lrelu(self.HRconv(fea)))
return out
#### RDN
**论文**:<https://arxiv.org/abs/1802.08797>
**代码**:
TensorFlow <https://github.com/hengchuan/RDN-TensorFlow>
Pytorch <https://github.com/lizhengwei1992/ResidualDenseNetwork-Pytorch>
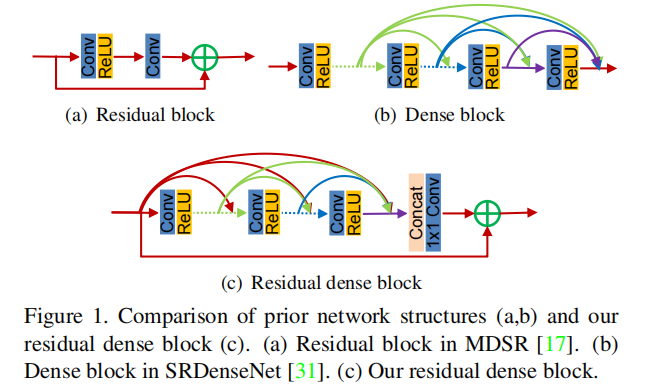
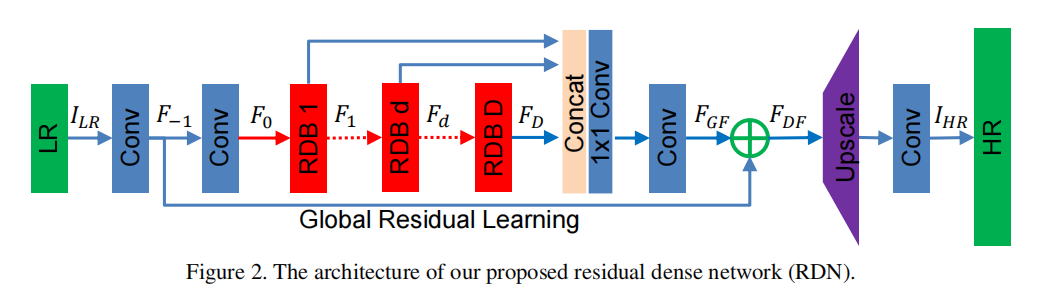
1. **创新点**:提出Residual Dense Block (RDB) 结构;
2. **好处**:残差学习和密集连接有效缓解网络深度增加引发的梯度消失的现象,其中密集连接加强特征传播, 鼓励特征复用。
3. **核心代码**:
import torch
import torch.nn as nn
import torch.nn.functional as F
class sub_pixel(nn.Module):
def __init__(self, scale, act=False):
super(sub_pixel, self).init()
modules = []
modules.append(nn.PixelShuffle(scale))
self.body = nn.Sequential(*modules)
def forward(self, x):
x = self.body(x)
return x
class make_dense(nn.Module):
def __init__(self, nChannels, growthRate, kernel_size=3):
super(make_dense, self).init()
self.conv = nn.Conv2d(nChannels, growthRate, kernel_size=kernel_size, padding=(kernel_size-1)//2, bias=False)
def forward(self, x):
out = F.relu(self.conv(x))
out = torch.cat((x, out), 1)
return out
Residual dense block (RDB) architecture
class RDB(nn.Module):
def __init__(self, nChannels, nDenselayer, growthRate):
super(RDB, self).init()
nChannels_ = nChannels
modules = []
for i in range(nDenselayer):
modules.append(make_dense(nChannels_, growthRate))
nChannels_ += growthRate
self.dense_layers = nn.Sequential(*modules)
self.conv_1x1 = nn.Conv2d(nChannels_, nChannels, kernel_size=1, padding=0, bias=False)
def forward(self, x):
out = self.dense_layers(x)
out = self.conv_1x1(out)
out = out + x
return out
Residual Dense Network
class RDN(nn.Module):
def __init__(self, args):
super(RDN, self).init()
nChannel = args.nChannel
nDenselayer = args.nDenselayer
nFeat = args.nFeat
scale = args.scale
growthRate = args.growthRate
self.args = args
# F-1
self.conv1 = nn.Conv2d(nChannel, nFeat, kernel_size=3, padding=1, bias=True)
# F0
self.conv2 = nn.Conv2d(nFeat, nFeat, kernel_size=3, padding=1, bias=True)
# RDBs 3
self.RDB1 = RDB(nFeat, nDenselayer, growthRate)
self.RDB2 = RDB(nFeat, nDenselayer, growthRate)
self.RDB3 = RDB(nFeat, nDenselayer, growthRate)
# global feature fusion (GFF)
self.GFF_1x1 = nn.Conv2d(nFeat\*3, nFeat, kernel_size=1, padding=0, bias=True)
self.GFF_3x3 = nn.Conv2d(nFeat, nFeat, kernel_size=3, padding=1, bias=True)
# Upsampler
self.conv_up = nn.Conv2d(nFeat, nFeat\*scale\*scale, kernel_size=3, padding=1, bias=True)
self.upsample = sub_pixel(scale)
# conv
self.conv3 = nn.Conv2d(nFeat, nChannel, kernel_size=3, padding=1, bias=True)
def forward(self, x):
F_ = self.conv1(x)
F_0 = self.conv2(F_)
F_1 = self.RDB1(F_0)
F_2 = self.RDB2(F_1)
F_3 = self.RDB3(F_2)
FF = torch.cat((F_1, F_2, F_3), 1)
FdLF = self.GFF_1x1(FF)
FGF = self.GFF_3x3(FdLF)
FDF = FGF + F_
us = self.conv_up(FDF)
us = self.upsample(us)
output = self.conv3(us)
return output
#### WDSR
**论文**:<https://arxiv.org/abs/1808.08718>
**代码**:
TensorFlow <https://github.com/ychfan/tf_estimator_barebone>
Pytorch <https://github.com/JiahuiYu/wdsr_ntire2018>
Keras <https://github.com/krasserm/super-resolution>
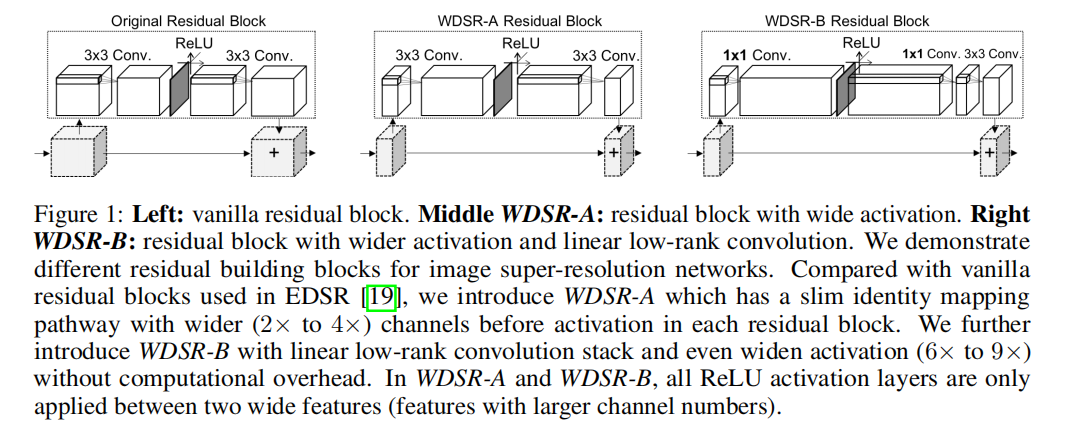
![在这里插入图片描述](https://img-blog.csdnimg.cn/e3454b6d9a4046b88d35b8ec