该系列仅在原课程基础上部分知识点添加个人学习笔记,或相关推导补充等。如有错误,还请批评指教。在学习了 Andrew Ng 课程的基础上,为了更方便的查阅复习,将其整理成文字。因本人一直在学习英语,所以该系列以英文为主,同时也建议读者以英文为主,中文辅助,以便后期进阶时,为学习相关领域的学术论文做铺垫。- ZJ
转载请注明作者和出处:ZJ 微信公众号-「SelfImprovementLab」
知乎:https://zhuanlan.zhihu.com/c_147249273
CSDN:http://blog.csdn.net/junjun_zhao/article/details/79184743
2.9 What is end- to-end deep learning (什么是端到端的深度学习)
(字幕来源:网易云课堂)
One of the most exciting recent developments in deep learning, has been the rise of end-to-end deep learning.So what is the end-to-end learning?Briefly, there have been some data processing systems, or learning systems that require multiple stages of processing.And what end-to-end deep learning does, is it can take all those multiple stages, and replace it usually with just a single neural network.Let’s look at some examples.Take speech recognition as an example, where your goal is to take an input x such an audio clip, and map it to an output y, which is a transcript of the audio clip.So traditionally, speech recognition required many stages of processing.First, you will extract some features, some hand-designed features of the audio.So if you’ve heard of MFCC, that’s an algorithm for extracting a certain set of hand designed features for audio.And then having extracted some low level features, you might apply a machine learning algorithm, to find the phonemes in the audio clip.So phonemes are the basic units of sound.So for example, the word cat is made out of three sounds.The Cu- Ah- and Tu- so they extract those.And then you string together phonemes to form individual words.And then you string those together to form the transcripts of the audio clip.
深度学习中最令人振奋的最新动态之一,就是端到端深度学习的兴起,那么端到端学习到底是什么呢?简而言之 以前有一些数据处理系统,或者学习系统 它们需要多个阶段的处理,那么端到端深度学习,就是忽略所有这些不同的阶段,用单个神经网络代替它,我们来看一些例子,以语音识别为例,你的目标是输入 x 比如说一段音频,然后把它映射到一个输出 y,就是这段音频的听写文本,所以传统上 语音识别需要很多阶段的处理,首先你会提取一些特征,一些手工设计的音频特征,也许你听过 MFCC,这种算法是用来从音频中提取一组特定的人工设计的特征,在提取出一些低层次特征之后,你可以应用机器学习算法,在音频片段中找到音位,所以音位是声音的基本单位,比如说 Cat 这个词是三个音节构成的,Cu-Ah-和Tu- 算法就把这三个音位提取出来,然后你将音位串在一起构成独立的词,然后你将词串起来构成音频片段的听写文本。
So, in contrast to this pipeline with a lot of stages, what end-to-end deep learning does, is you can train a huge neural network to just input the audio clip, and have it directly output the transcript.One interesting sociological effect in AI is that as end-to-end deep learning started to work better, there were some researchers that had for example spent many years of their career designing individual steps of the pipeline.So there were some researchers in different disciplines not just in speech recognition.Maybe in computer vision, and other areas as well, that had spent a lot of time you know, written multiple papers, maybe even built a large part of their career, engineering features or engineering other pieces of the pipeline.And when end-to-end deep learning just took the last training set and learned the function mapping from x and y directly, really bypassing a lot of these intermediate steps, it was challenging for some disciplines to come around to accepting this alternative way of building AI systems.Because it really obsoleted in some cases, many years of research in some of the intermediate components.
所以和这种有很多阶段的流水线相比,端到端深度学习做的是,你训练一个巨大的神经网络 输入就是一段音频,输出直接是听写文本,AI 的其中一个有趣的社会学效应是,随着端到端深度学习系统表现开始更好,有一些花了大量时间 或者整个事业生涯,设计出流水线各个步骤的研究员,还有其他领域的研究员 不只是语言识别领域的,也许是计算机视觉 还有其他领域,他们花了大量的时间,写了很多论文 有些甚至整个职业生涯的一大部分都投入到,开发这个流水线的功能或者其他构件上去了,而端到端深度学习就只需要把训练集拿过来,直接学到了 x 和 y 之间的函数映射,直接绕过了其中很多步骤,对一些学科里的人来说 这点相当难以接受,他们无法接受这样构建AI系统,因为有些情况 端到端方法完全取代了旧系统,某些投入了多年研究的中间件也许已经过时了。
It turns out that one of the challenges of end-to-end deep learning is that you might need a lot of data before it works well.So for example, if you’re training on3,000 hours of data to build a speech recognition system, then the traditional pipeline, the full traditional pipeline works really well.It’s only when you have a very large data set, you know one to say 10,000 hours of data, anything going up to maybe 100,000 hours of data that the end-to end-approach then suddenly starts to work really well.So when you have a smaller data set, the more traditional pipeline approach actually works just as well.Often works even better.And you need a large data set before the end-to-end approach really shines.And if you have a medium amount of data, then there are also intermediate approaches where maybe you input audio and bypass the features and just learn to output the phonemes of the neural network, and then at some other stages as well.So this will be a step toward end-to-end learning, but not all the way there.
事实证明 端到端深度学习的挑战之一是,你可能需要大量数据才能让系统表现良好,比如 你只有 3000 小时数据,去训练你的语音识别系统,那么传统的流水线,传统的流水线效果真的很好,但当你拥有非常大的数据集时,比如 10000 小时数据,或者 100,000 小时数据,这样端到端方法突然开始很厉害了,所以当你的数据集较小的时候,传统流水线方法其实效果也不错,通常做得更好,你需要大数据集 才能让端到端方法真正发出耀眼光芒,如果你的数据量适中,那么也可以用中间件方法 你可能输入还是音频,然后绕过特征提取 直接尝试从神经网络输出音位,然后也可以在其他阶段用,所以这是往端到端学习迈出的一小步,但还没有到那里。
So this is a picture of a face recognition turnstile built by a researcher, yuanqing Lin at Baidu, where this is a camera and it looks at the person approaching the gate, and if it recognizes the person then, you know the turnstile automatically lets them through.So rather than needing to swipe an RFID badge to enter this facility, in increasingly many offices in China and hopefully more and more in other countries as well, you can just approach the turnstile and if it recognizes your face it just lets you through without needing you to carry an RFID badge.So, how do you build a system like this?Well, one thing you could do is just look at the image that the camera is capturing.Right? So, I guess this is my bad drawing, but maybe this is a camera image.And you know, you have someone approaching the turnstile.So this might be the image x that you that your camera is capturing.And one thing you could do is try to learn a function mapping directly from the image x to the identity of the person y.It turns out this is not the best approach.And one of the problems is that you know, the person approaching the turnstile can approach from lots of different directions.So they could be green positions, they could be in blue position.you know, sometimes they’re closer to the camera, so they appear bigger in the image.And sometimes they’re already closer to the camera, so that face appears much bigger.So what it has actually done to build these turnstiles, is not to just take the raw image and feed it to a neural net to try to figure out a person’s identity.
这张图上是一个研究员做的人脸识别门禁,是百度的林元庆研究员做的,这是一个相机 它会拍下接近门禁的人,如果它认出了那个人,门禁系统就自动打开 让他通过,所以你不需要刷一个RFID工卡就能进入这个设施,系统部署在越来越多的中国办公室,希望在其他国家也可以部署更多,你可以接近门禁 如果它认出你的脸,它就直接让你通过 你不需要带 RFID工卡,那么 怎么搭建这样的系统呢? 你可以做的第一件事是 看看相机拍到的照片,对吧? 我想我画的不太好,但也许这是相机照片,你知道 有人接近门禁了,所以这可能是相机拍到的图像 x,有件事你可以做 就是尝试直接学习,图像 x 到人物 y 身份的函数映射,事实证明这不是最好的方法,其中一个问题是,人可以从很多不同的角度接近门禁,他们可能在绿色位置,可能在蓝色位置,有时他们更靠近相机,所以他们看起来更大,有时候他们非常接近相机,那照片中脸就很大了,在实际研制这些门禁系统时,他不是直接将原始照片,喂到一个神经网络 试图找出一个人的身份。
Instead, the best approach to date, seems to be a multi-step approach, where first, you run one piece of software to detect the person’s face.So this first detector to figure out where’s the person’s face.Having detected the person’s face, you then zoom in to that part of the image and crop that image so that the person’s face is centered.Then, it is this picture that I guess I drew here in red, this is then fed to the neural network, to then try to learn, or estimate the person’s identity.And what researchers have found, is that instead of trying to learn everything on one step, by breaking this problem down into two simpler steps, first is figure out where is the face.And second, is look at the face and figure out who this actually is.This second approach allows the learning algorithm or really two learning algorithms to solve two much simpler tasks and results in overall better performance.By the way, if you want to know how the second step actually works I’ve simplified the discussion.
相反 迄今为止最好的方法,似乎是一个多步方法 首先,你运行一个软件来检测人脸,所以第一个检测器找的是人脸位置,检测到人脸,然后放大图像的那部分,并裁剪图像 使人脸居中显示,然后就是这里红线框起来的照片,再喂到神经网络里,让网络去学习,或估计那人的身份,研究人员发现,比起一步到位 一步学习,把这个问题分解成两个更简单的步骤,首先是弄清楚脸在哪里,第二步是看着脸 弄清楚这是谁,这第二种方法让学习算法 或者说两个学习算法,分别解决两个更简单的任务 并在整体上得到更好的表现,顺便说一句 如果你想知道,第二步实际是怎么工作的 我这里其实省略了很多。
By the way, if you want to know how step two here actually works,I’ve actually simplified the description a bit.The way the second step is actually trained, as you train in your network, that takes as input two images, and what then your network does is it takes this input two images and it tells you if these two are the same person or not.So if you then have say 10,000 employees IDs on file, you can then take this image in red, and quickly compare it against maybe all10,000 employee IDs on file to try to figure out if this picture in red is indeed one of your 10000 employees that you should allow into this facility or that should allow into your office building.This is a turnstile that is giving employees access to a workplace.So why is it that the two step approach works better?There are actually two reasons for that.One is that each of the two problems you’re solving is actually much simpler.But second, is that you have a lot of data for each of the two sub-tasks.In particular, there is a lot of data you can obtain for face detection, for task one over here, where the task is to look at an image and figure out where is the person’s face and the image.So there is a lot of data.There is a lot of label data x, comma y where x is a picture and y shows the position of the person’s face.So you could build a neural network to do task one quite well.And then separately, there’s a lot of data for task two as well.Today, leading companies have let’s say, hundreds of millions of pictures of people’s faces.So given a closely cropped image, like this red image or this one down here, today leading face recognition teams have at least hundreds of millions of images that they could use to look at two images and try to figure out the identity or to figure out if it’s the same person or not.So there’s also a lot of data for task two.But in contrast, if you were to try to learn everything at the same time, there is much less data of the form x comma y.Where x is image like this taken from the turnstile, and y is the identity of the person.So because you don’t have enough data to solve this end-to-end learning problem, but you do have enough data to solve sub-problems one and two, in practice, breaking this down to two sub-problems results in better performance than a pure end-to-end deep learning approach.Although if you had enough data for the end-to-end approach, maybe the end-to-end approach would work better, but that’s not actually what works best in practice today.
顺便说一下 如果你想知道第二步实际怎么工作的,我其实省略了很多细节,训练第二步的方式,训练网络的方式,就是输入两张图片,然后你的网络做的就是,将输入的两张图比较一下 判断是否是同一个人,比如你记录了10000个员工ID,你可以把红色框起来的图像,快速比较..也许是,全部10,000个员工记录在案的ID 看看,这张红线内的照片 是不是那10000个员工之一,应该允许进入这个设施 或者进入这个办公楼,这是一个门禁系统 允许员工进入工作场所的门禁,为什么两步法更好呢?实际上有两个原因,一是你解决的两个问题 每个问题实际上要简单得多,但第二 两个子任务的训练数据都很多,具体来说 有很多数据可以用于人脸识别训练,对于这里的任务1来说,任务就是观察一张图 找出人脸所在的位置,把人脸图像框出来,所以有很多数据,有很多标签数据 x,y,其中 x 是图片 y 是表示人脸的位置,你可以建立一个神经网络 可以很好地处理任务 1,然后任务 2 也有很多数据可用,今天 业界领先的公司拥有 比如说,数百万张人脸照片,所以输入一张裁剪得很紧凑的照片,比如这张红色照片 下面这个,今天业界领先的人脸识别团队有,至少数亿的图像,他们可以用来观察两张图片,并试图判断照片里人的身份,确定是否同一个人,所以任务2还有很多数据,相比之下 如果你想一步到位,这样x,y的数据对就少得多,其中x是门禁系统拍摄的图像,y是那人的身份,因为你没有足够多的数据去解决这个端到端学习问题,但你却有足够多的数据来解决子问题 1 和子问题 2 实际上,把这个分成两个子问题,比纯粹的端到端深度学习方法 那达到更好的表现,不过如果你有足够多的数据来做端到端学习,也许端到端方法效果更好,但在今天的实践中 并不是最好的方法。
Let’s look at a few more examples.Take machine translation.Traditionally, machine translation systems also had a long complicated pipeline, where you first take say English, text and then do text analysis.Basically, extract a bunch of features off the text, and so on.And after many many steps you’d end up with say, a translation of the English text into French.Because, for machine translation, you do have a lot of pairs of English comma French sentences.End-to-end deep learning works quite well for machine translation.And that’s because today, it is possible to gather large data sets of x-y pairs where that’s the English sentence and that’s the corresponding French translation.So in this example, end-to-end deep learning works well.One last example, let’s say that you want to look at an x-ray picture of a hand of a child, and estimate the age of a child.you know, when I first heard about this problem,I thought this is a very cool crime scene investigation task where you find maybe tragically the skeleton of a child, and you want to figure out how the child was.It turns out that typical application of this problem, estimating age of a child from an x-ray is less dramatic than this crime scene investigation I was picturing.It turns out that pediatricians use this to estimate whether or not a child is growing or developing normally.But a non end-to-end approach to this, would be you locate an image and then you segment out or recognize the bones.So, just try to figure out where is that bone segment?Where is that bone segment?Where is that bone segment? And so on. And then.Knowing the lengths of the different bones, you can sort of go to a look up table showing the average bone lengths in a child’s hand and then use that to estimate the child’s age.And so this approach actually works pretty well.In contrast, if you were to go straight from the image to the child’s age, then you would need a lot of data to do that directly and as far as I know, this approach does not work as well today just because there isn’t enough data to train this task in an end-to-end fashion.Whereas in contrast, you can imagine that by breaking down this problem into two steps.Step one is a relatively simple problem.Maybe you don’t need that much data.Maybe you don’t need that many x-ray images to segment out the bones.And task two, by collecting statistics of a number of children’s hands, you can also get decent estimates of that without too much data.So this multi-step approach seems promising.Maybe more promising than the end-to-end approach, at least until you can get more data for the end-to-end learning approach.
我们再来看几个例子,比如机器翻译,传统上 机器翻译系统也有一个很复杂的流水线,比如英语机翻,得到文本 然后做文本分析,基本上 要从文本中提取一些特征 之类的,经过很多步骤 你最后会..,将英文文本翻译成法文,因为 对于机器翻译来说,的确有很多(英文,法文)的数据对,端到端深度学习在机器翻译领域非常好用,那是因为在今天,可以收集 x-y 对的大数据集,就是英文句子和对应的法语翻译,所以在这个例子中,端到端深度学习效果很好,最后一个例子 比如说你希望,观察一个孩子手部的x光照片,并估计一个孩子的年龄,你知道 当我第一次听到这个问题的时候,我以为这是一个非常酷的犯罪现场调查任务,你可能悲剧的发现了一个孩子的骨架,你想弄清楚孩子在生时是怎么样的,事实证明 这个问题的典型应用,从x射线图估计孩子的年龄,是我想太多了 没有我想象的犯罪现场调查脑洞那么大,结果这是儿科医生用来,判断一个孩子的发育是否正常,处理这个例子的一个非端到端方法,就是照一张图 然后分割出每一块骨头,所以就是分辨出那段骨头应该在哪里,那段骨头在哪里,那段骨头在哪里? 等等 然后,知道不同骨骼的长度,你可以去查表,查到儿童手中骨头的平均长度,然后用它来估计孩子的年龄,所以这种方法实际上很好,相比之下 如果你直接从图像去判断孩子的年龄,那么你需要大量的数据去直接训练 据我所知,这种做法今天还是不行的,因为没有足够的数据来用端到端的方式来训练这个任务,相比之下 你可以想象一下如何将这个问题分解成两个步骤,第一步是一个比较简单的问题,也许你不需要那么多数据,也许你不需要许多x射线图像来切分骨骼,而任务二 收集儿童手部的骨头长度的统计数据,你不需要太多数据也能做出相当准确的估计,所以这个多步方法看起来很有希望,也许比端对端方法更有希望,至少直到你能获得更多端到端学习的数据之前。
So an end-to-end deep learning works.It can work really well and it can really simplify the system and not require you to build so many hand-designed individual components.But it’s also not panacea, it doesn’t always work.In the next video,I want to share with you a more systematic description of when you should, and maybe when you shouldn’t use end-to-end deep learning and how to piece together these complex machine learning systems.
所以端到端深度学习系统是可行的,它表现可以很好 也可以简化系统架构,让你不需要搭建那么多手工设计的单独组件,但它也不是灵丹妙药,并不是每次都能成功,在下一个视频中,我想与你分享一个更系统的描述 什么时候你应该使用,或者不应该使用端到端的深度学习,以及如何组装这些复杂的机器学习系统。
重点总结:
端到端深度学习
定义:
相对于传统的一些数据处理系统或者学习系统,它们包含了多个阶段的处理过程,而端到端的深度学习则忽略了这些阶段,用单个神经网络来替代。
语音识别例子:
在少数据集的情况下传统的特征提取方式可能会取得好的效果;如果在有足够的大量数据集情况下,端到端的深度学习会发挥巨大的价值。
优缺点:
优点:
- 端到端学习可以直接让数据“说话”;
- 所需手工设计的组件更少。
缺点:
- 需要大量的数据;
- 排除了可能有用的手工设计组件。
应用端到端学习的 Key question:是否有足够的数据能够直接学习到从 x 映射到 y 的足够复杂的函数。
参考文献:
[1]. 大树先生.吴恩达Coursera深度学习课程 DeepLearning.ai 提炼笔记(3-2)– 机器学习策略(2)
PS: 欢迎扫码关注公众号:「SelfImprovementLab」!专注「深度学习」,「机器学习」,「人工智能」。以及 「早起」,「阅读」,「运动」,「英语 」「其他」不定期建群 打卡互助活动。
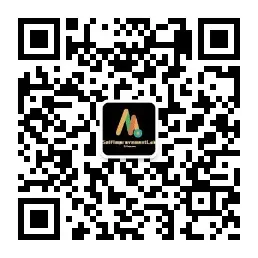