1 资源
- paper : https://arxiv.org/abs/2001.11180
- code : **
- MOT 评价指标:https://blog.csdn.net/ycc2011/article/details/86633768
2 名词释义
- Multiple Object Tracking (MOT):多目标跟踪;
- Re- Identification (Re-ID):重识别/再识别;
- Deep Neural Networks (DNNs):深度神经网络;
- Flow-Fuse-Tracker (FFT):流动融合跟踪器;
- Multiple Object Tracking Accuracy(MOTA):跟踪准确度;
3 摘要
Abstract: Most of Multiple Object Tracking (MOT) approaches compute individual target features for two subtasks: estimating target-wise motions and conducting pair-wise Re-Identification (Re-ID). Because of the indefinite number of targets among video frames, both subtasks are very difficult to scale up efficiently in end-to-end Deep Neural Networks (DNNs). In this paper, we design an end-to-end DNN tracking approach, Flow-Fuse-Tracker (FFT), that addresses the above issues with two efficient techniques: target flowing and target fusing. Specifically, in target flowing, a FlowTracker DNN module learns the indefinite number of target-wise motions jointly from pixel-level optical flows. In target fusing, a FuseTracker DNN module refines and fuses targets proposed by FlowTracker and frame-wise object detection, instead of trusting either of the two inaccurate sources of target proposal. Because FlowTracker can explore complex target-wise motion patterns and Fuse-Tracker can refine and fuse targets from FlowTracker and detectors, our approach can achieve the state-of-the-art results on several MOT benchmarks. As an online MOT approach, FFT produced the top MOTA of 46.3 on the 2DMOT15, 56.5 on the MOT16, and 56.5 on the MOT17 tracking benchmarks, surpassing all the online and offline methods in existing publications.
摘要: 大多数的多目标跟踪(MOT)方法都是为两个子任务计算单独的目标特征:目标运动方向的估计和成对目标的再识别(Re-ID)。由于视频帧之间的目标数目不确定,这两个子任务在端到端深度神经网络(DNNs)中都很难有效地扩展。针对上述问题,本文设计了一种端到端DNN跟踪方法——流动融合跟踪器(Flow-Fuse-Tracker,FFT)。具体来说,在目标流动中,流动跟踪DNN模块从像素级的光流中联合学习目标运动的不确定数目。在目标融合中,一个流动跟踪DNN模块是通过流动跟踪器和按帧的目标检测提取细化和融合的目标,而不是取信于两种不准确的目标融合源的任何一种。因此流动跟踪可以探索复杂的目标运动模式和来自跟踪器与检测器的目标细化和融合。我们的方法可以在多个MOT基准测试上获得最佳的效果。作为一种在线MOT方法,FFT获得MOTA分别在2DMOT15、MOT16、MOT17为46.3、56.5、56.5的效果,超过了现有出版物中所有的在线和离线方法。
4 Overview
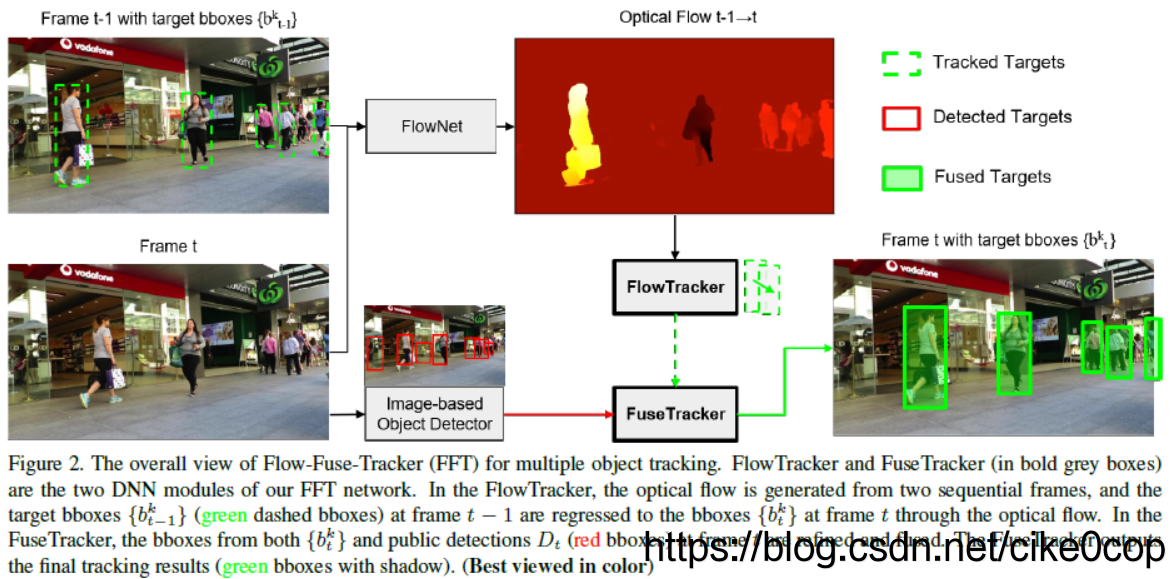
5 理解概述
本文介绍关于多目标追踪的技术