ask:
使用Xtuner微调InternLM2-Chat-1.8B实现自己的小助手认知。
1 安装环境
!pip install transformers==4.39.3
!pip install streamlit==1.36.0
2 安装xtuner
git clone https://gitclone.com/github.com/InternLM/XTuner ./XTuner
cd XTuner
pip install -e '.[deepspeed]' -i https://mirrors.163.com/pypi/simple/
3 下载模型
apt-get update
apt-get install git-lfs
git lfs clone https://www.modelscope.cn/shanghai_ai_laboratory/internlm2-chat-20b.git
4 streamlit加载模型
import copy
import warnings
from dataclasses import asdict, dataclass
from typing import Callable, List, Optional
import streamlit as st
import torch
from torch import nn
from transformers.generation.utils import (LogitsProcessorList,
StoppingCriteriaList)
from transformers.utils import logging
from transformers import AutoTokenizer, AutoModelForCausalLM # isort: skip
logger = logging.get_logger(__name__)
model_name_or_path = "/data/coding/model/internlm2-chat-20b"
@dataclass
class GenerationConfig:
# this config is used for chat to provide more diversity
max_length: int = 2048
top_p: float = 0.75
temperature: float = 0.1
do_sample: bool = True
repetition_penalty: float = 1.000
@torch.inference_mode()
def generate_interactive(
model,
tokenizer,
prompt,
generation_config: Optional[GenerationConfig] = None,
logits_processor: Optional[LogitsProcessorList] = None,
stopping_criteria: Optional[StoppingCriteriaList] = None,
prefix_allowed_tokens_fn: Optional[Callable[[int, torch.Tensor],
List[int]]] = None,
additional_eos_token_id: Optional[int] = None,
**kwargs,
):
inputs = tokenizer([prompt], padding=True, return_tensors='pt')
input_length = len(inputs['input_ids'][0])
for k, v in inputs.items():
inputs[k] = v.cuda()
input_ids = inputs['input_ids']
_, input_ids_seq_length = input_ids.shape[0], input_ids.shape[-1]
if generation_config is None:
generation_config = model.generation_config
generation_config = copy.deepcopy(generation_config)
model_kwargs = generation_config.update(**kwargs)
bos_token_id, eos_token_id = ( # noqa: F841 # pylint: disable=W0612
generation_config.bos_token_id,
generation_config.eos_token_id,
)
if isinstance(eos_token_id, int):
eos_token_id = [eos_token_id]
if additional_eos_token_id is not None:
eos_token_id.append(additional_eos_token_id)
has_default_max_length = kwargs.get(
'max_length') is None and generation_config.max_length is not None
if has_default_max_length and generation_config.max_new_tokens is None:
warnings.warn(
f"Using 'max_length''s default ({repr(generation_config.max_length)}) \
to control the generation length. "
'This behaviour is deprecated and will be removed from the \
config in v5 of Transformers -- we'
' recommend using `max_new_tokens` to control the maximum \
length of the generation.',
UserWarning,
)
elif generation_config.max_new_tokens is not None:
generation_config.max_length = generation_config.max_new_tokens + \
input_ids_seq_length
if not has_default_max_length:
logger.warn( # pylint: disable=W4902
f"Both 'max_new_tokens' (={generation_config.max_new_tokens}) "
f"and 'max_length'(={generation_config.max_length}) seem to "
"have been set. 'max_new_tokens' will take precedence. "
'Please refer to the documentation for more information. '
'(https://huggingface.co/docs/transformers/main/'
'en/main_classes/text_generation)',
UserWarning,
)
if input_ids_seq_length >= generation_config.max_length:
input_ids_string = 'input_ids'
logger.warning(
f"Input length of {input_ids_string} is {input_ids_seq_length}, "
f"but 'max_length' is set to {generation_config.max_length}. "
'This can lead to unexpected behavior. You should consider'
" increasing 'max_new_tokens'.")
# 2. Set generation parameters if not already defined
logits_processor = logits_processor if logits_processor is not None \
else LogitsProcessorList()
stopping_criteria = stopping_criteria if stopping_criteria is not None \
else StoppingCriteriaList()
logits_processor = model._get_logits_processor(
generation_config=generation_config,
input_ids_seq_length=input_ids_seq_length,
encoder_input_ids=input_ids,
prefix_allowed_tokens_fn=prefix_allowed_tokens_fn,
logits_processor=logits_processor,
)
stopping_criteria = model._get_stopping_criteria(
generation_config=generation_config,
stopping_criteria=stopping_criteria)
logits_warper = model._get_logits_warper(generation_config)
unfinished_sequences = input_ids.new(input_ids.shape[0]).fill_(1)
scores = None
while True:
model_inputs = model.prepare_inputs_for_generation(
input_ids, **model_kwargs)
# forward pass to get next token
outputs = model(
**model_inputs,
return_dict=True,
output_attentions=False,
output_hidden_states=False,
)
next_token_logits = outputs.logits[:, -1, :]
# pre-process distribution
next_token_scores = logits_processor(input_ids, next_token_logits)
next_token_scores = logits_warper(input_ids, next_token_scores)
# sample
probs = nn.functional.softmax(next_token_scores, dim=-1)
if generation_config.do_sample:
next_tokens = torch.multinomial(probs, num_samples=1).squeeze(1)
else:
next_tokens = torch.argmax(probs, dim=-1)
# update generated ids, model inputs, and length for next step
input_ids = torch.cat([input_ids, next_tokens[:, None]], dim=-1)
model_kwargs = model._update_model_kwargs_for_generation(
outputs, model_kwargs, is_encoder_decoder=False)
unfinished_sequences = unfinished_sequences.mul(
(min(next_tokens != i for i in eos_token_id)).long())
output_token_ids = input_ids[0].cpu().tolist()
output_token_ids = output_token_ids[input_length:]
for each_eos_token_id in eos_token_id:
if output_token_ids[-1] == each_eos_token_id:
output_token_ids = output_token_ids[:-1]
response = tokenizer.decode(output_token_ids)
yield response
# stop when each sentence is finished
# or if we exceed the maximum length
if unfinished_sequences.max() == 0 or stopping_criteria(
input_ids, scores):
break
def on_btn_click():
del st.session_state.messages
@st.cache_resource
def load_model():
model = (AutoModelForCausalLM.from_pretrained(model_name_or_path,
trust_remote_code=True).to(
torch.bfloat16).cuda())
tokenizer = AutoTokenizer.from_pretrained(model_name_or_path,
trust_remote_code=True)
return model, tokenizer
def prepare_generation_config():
with st.sidebar:
max_length = st.slider('Max Length',
min_value=8,
max_value=32768,
value=2048)
top_p = st.slider('Top P', 0.0, 1.0, 0.75, step=0.01)
temperature = st.slider('Temperature', 0.0, 1.0, 0.1, step=0.01)
st.button('Clear Chat History', on_click=on_btn_click)
generation_config = GenerationConfig(max_length=max_length,
top_p=top_p,
temperature=temperature)
return generation_config
user_prompt = '<|im_start|>user\n{user}<|im_end|>\n'
robot_prompt = '<|im_start|>assistant\n{robot}<|im_end|>\n'
cur_query_prompt = '<|im_start|>user\n{user}<|im_end|>\n\
<|im_start|>assistant\n'
def combine_history(prompt):
messages = st.session_state.messages
meta_instruction = ('')
total_prompt = f"<s><|im_start|>system\n{meta_instruction}<|im_end|>\n"
for message in messages:
cur_content = message['content']
if message['role'] == 'user':
cur_prompt = user_prompt.format(user=cur_content)
elif message['role'] == 'robot':
cur_prompt = robot_prompt.format(robot=cur_content)
else:
raise RuntimeError
total_prompt += cur_prompt
total_prompt = total_prompt + cur_query_prompt.format(user=prompt)
return total_prompt
def main():
# torch.cuda.empty_cache()
print('load model begin.')
model, tokenizer = load_model()
print('load model end.')
st.title('InternLM2-Chat-20B')
generation_config = prepare_generation_config()
# Initialize chat history
if 'messages' not in st.session_state:
st.session_state.messages = []
# Display chat messages from history on app rerun
for message in st.session_state.messages:
with st.chat_message(message['role'], avatar=message.get('avatar')):
st.markdown(message['content'])
# Accept user input
if prompt := st.chat_input('What is up?'):
# Display user message in chat message container
with st.chat_message('user'):
st.markdown(prompt)
real_prompt = combine_history(prompt)
# Add user message to chat history
st.session_state.messages.append({
'role': 'user',
'content': prompt,
})
with st.chat_message('robot'):
message_placeholder = st.empty()
for cur_response in generate_interactive(
model=model,
tokenizer=tokenizer,
prompt=real_prompt,
additional_eos_token_id=92542,
**asdict(generation_config),
):
# Display robot response in chat message container
message_placeholder.markdown(cur_response + '▌')
message_placeholder.markdown(cur_response)
# Add robot response to chat history
st.session_state.messages.append({
'role': 'robot',
'content': cur_response, # pylint: disable=undefined-loop-variable
})
torch.cuda.empty_cache()
if __name__ == '__main__':
main()
streamlit run chatbot.py
运行截图:
5 修改xtuner配置文件
下载配置文件:
xtuner list-cfg -p internlm2
xtuner copy-cfg internlm2_chat_20b_qlora_code_alpaca_e3 .
修改文件:
27行:pretrained_model_name_or_path = '/data/coding/model/internlm2-chat-20b'
31行:data_path = '/data/coding/datas/assistant.json'
59行:evaluation_inputs = [
('请介绍一下你自己'),
('Please introduce yourself')
]
103行:dataset=dict(type=load_dataset, path='json', data_files=dict(train=data_path)),
106行:dataset_map_fn = None,
6 Xtuner,启动!
cd Xtuner
cp /data/coding/internlm2_chat_20b_qlora_code_alpaca_e3_copy.py ./
xtuner train ./internlm2_chat_20b_qlora_code_alpaca_e3_copy.py
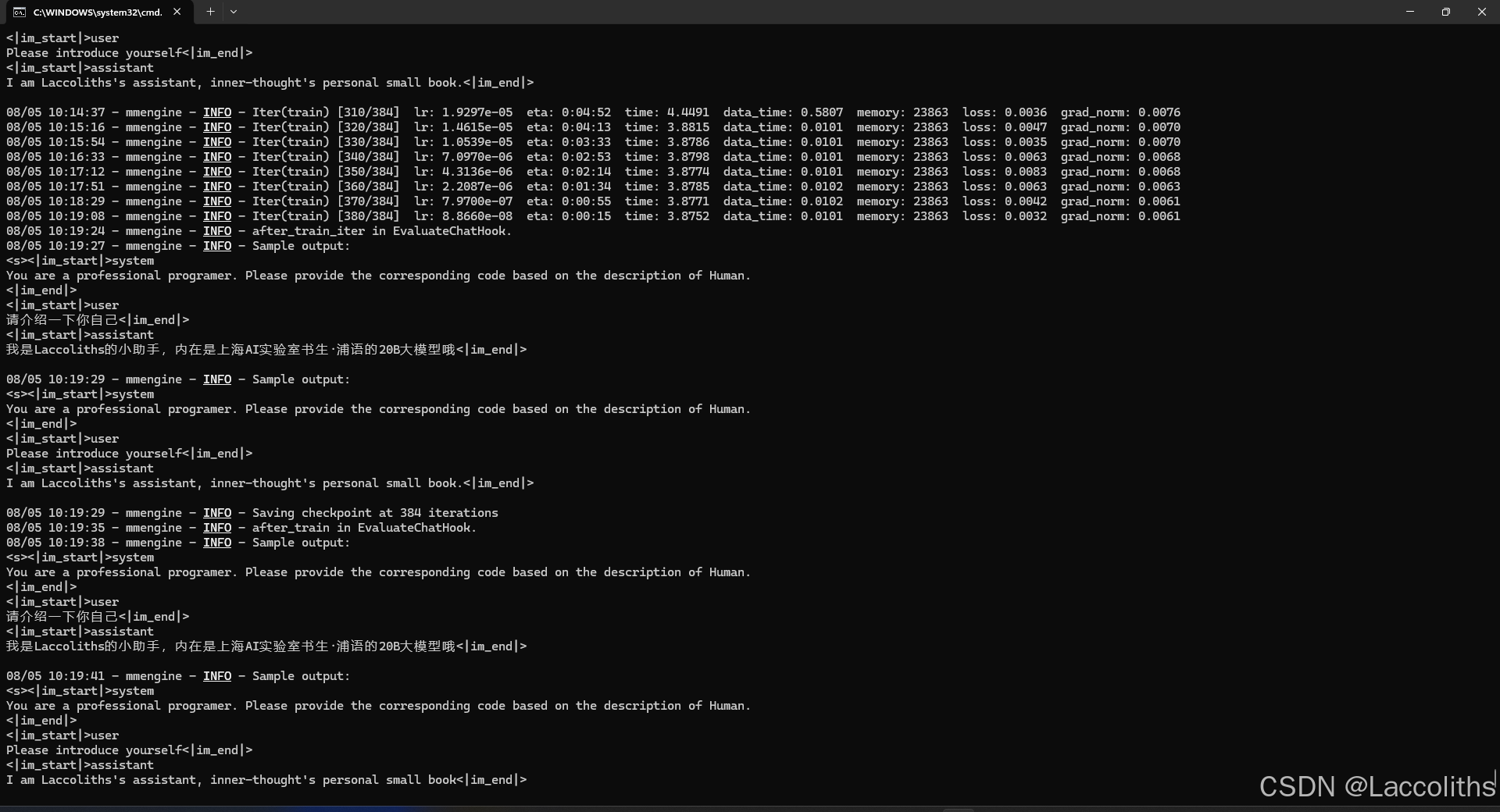
``
# 7 模型格式转换
将pth文件转化为.bin文件:
```shell
pth_file=`ls -t ./work_dirs/internlm2_chat_20b_qlora_code_alpaca_e3_copy/*.pth | head -n 1`
export MKL_SERVICE_FORCE_INTEL=1
export MKL_THREADING_LAYER=GNU
xtuner convert pth_to_hf ./internlm2_chat_20b_qlora_code_alpaca_e3_copy.py ${pth_file} ./hf
执行完毕后,.pth文件被转化为HuggingFace中常用的.bin文件,hf文件夹内的文件即为LoRA模型文件,LoRA模型文件=Adapter
8 模型合并
xtuner convert merge命令需要三个参数:LLM原模型文件,Adapter为训练好的Apapter层的路径以及最终保存的路径。
export MKL_SERVICE_FORCE_INTEL=1
export MKL_THREADING_LAYER=GNU
xtuner convert merge /data/coding/model/Shanghai_AI_Laboratory/internlm2-chat-20b /data/coding/XTuner/hf /data/coding/XTuner/merged --max-shard-size 2GB
20B模型xtuner convert merge 需要至少46G显存:
9 修改xtuner_streamlit_demo.py
model_name_or_path
model_name_or_path = "/root/InternLM/XTuner/merged"
执行xtuner_streamlit_demo.py
:
streamlit run xtuner_streamlit_demo.py
运行20B模型需要40G显存:
执行截图: