java 亚马逊 mws
https://www.deepnetts.com/blog/deep-netts-community-editionInterest in machine learning has grown steadily over recent years. Specifically, enterprises now use machine learning for image recognition in a wide variety of use cases. There are applications in the automotive industry, healthcare, security, retail, automated product tracking in warehouses, farming and agriculture, food recognition and even real-time translation by pointing your phone’s camera. Thanks to machine learning and visual recognition, machines can detect cancer and COVID-19 in MRIs and CT scans.
https://www.deepnetts.com/blog/deep-netts-community-edition对机器学习的兴趣近年来一直在稳步增长。 具体来说,企业现在在多种使用案例中使用机器学习进行图像识别。 指着手机的摄像头 ,可用于汽车工业 , 医疗保健 , 安全 , 零售 , 仓库 , 农业和农业中的 自动产品跟踪 , 食品识别甚至实时翻译等应用 。 由于机器学习和视觉识别,机器可以在MRI和CT扫描中检测出癌症和COVID-19 。
Today, many of these solutions are primarily developed in Python using open source and proprietary ML toolkits, each with their own APIs. Despite Java's popularity in enterprises, there aren’t any standards to develop machine learning applications in Java. JSR-381 was developed to address this gap by offering Java application developers a set of standard, flexible and Java-friendly APIs for Visual Recognition (VisRec) applications such as image classification and object detection. JSR-381 has several implementations that rely on machine learning platforms such as TensorFlow, MXNet and DeepNetts. One of these implementations is based on Deep Java Library (DJL), an open source library developed by Amazon to build machine learning in Java. DJL offers hooks to popular machine learning frameworks such as TensorFlow, MXNet, and PyTorch by bundling requisite image processing routines, making it a flexible and simple choice for JSR-381 users.
如今,这些解决方案中的许多解决方案主要是使用开放源代码和专有的ML工具包在Python中开发的,每个工具包都有自己的API。 尽管 Java在企业中的流行,没有任何标准可以用Java开发机器学习应用程序。 通过为Java应用程序开发人员提供一组用于视觉识别(VisRec)应用程序的标准,灵活和Java友好的API(例如图像分类和对象检测)来开发JSR-381 ,以解决这一问题。 JSR-381的一些实现依赖于机器学习平台,例如TensorFlow,MXNet和DeepNetts。 其中之一 实现是基于 深度Java库 (DJL),这是由Amazon开发的开放源代码库,用于在Java中构建机器学习。 DJL通过捆绑必要的图像处理例程,提供了与流行的机器学习框架(如TensorFlow , MXNet和PyTorch)的挂钩 ,从而使其成为JSR-381用户的灵活简便的选择。
In this article, we demonstrate how Java developers can use the JSR-381 VisRec API to implement image classification or object detection with DJL’s pre-trained models in less than 10 lines of code. We also demonstrate how users can use pre-trained machine learning models in less than 10 minutes with two examples. Let’s get started!
在本文中,我们演示了Java开发人员如何使用JSR-381 VisRec API通过DJL的预训练模型在不到10行代码中实现图像分类或对象检测。 我们还将通过两个示例演示用户如何在不到10分钟的时间内使用经过预先训练的机器学习模型。 让我们开始吧!
使用预先训练的模型识别手写数字 (Recognizing handwritten digits using a pre-trained model)
A useful application and ‘hello world’ example of visual recognition is recognizing handwritten digits. Recognizing handwritten digits is seemingly easy for a human. Thanks to the processing capability and cooperation of the visual and pattern matching subsystems in our brains, we can usually correctly discern the correct digit from a sloppily handwritten document. However this seemingly straightforward task is incredibly complex for a machine due to many possible variations. This is a good use case for machine learning, specifically visual recognition. The JSR 381 repo has a great example that uses the JSR-381 VisRec API to correctly recognize handwritten digits. This example compares handwritten digits, against the MNIST handwritten digit dataset, a publicly available database of over 60K images. Predicting what an image represents is called image classification. Our example looks at a new image and attempts to determine the probabilities of what specific digit it is.
视觉识别的一个有用的应用程序和“ hello world”示例是识别手写数字。 识别手写数字对于人类来说似乎很容易。 由于我们大脑中视觉和模式匹配子系统的处理能力和协作能力,我们通常可以正确地识别出草率手写文档中的正确数字。 但是,由于许多可能的变化,对于机器而言,这项看似简单的任务非常复杂。 这是机器学习(尤其是视觉识别)的良好用例。 JSR 381存储库就是一个很好的示例 ,该示例使用JSR-381 VisRec API正确识别手写数字。 本示例将手写数字与 MNIST手写数字数据集 ,一个超过60K图像的公共可用数据库。 预测图像代表什么称为图像分类。 我们的示例查看一个新图像,并尝试确定它是特定数字的概率。
For this task, the VisRec API provides an ImageClassifier interface which can be specialized for specific Java classes for input images using generic parameters. It also provides a classify() method which performs image classification and returns a Map of class probabilities for all possible image classes. By convention in the VisRec API, each model provides a static builder() method that returns a corresponding builder object, and allows the developer to configure all relevant settings, e.g. imageHeight, imageWidth.
对于此任务,VisRec API提供了一个ImageClassifier接口,该接口可以专用于使用通用参数的特定Java类,用于输入图像。 它还提供了classify()方法,该方法执行图像分类并返回所有可能图像类别的类别概率图。 根据VisRec API中的约定,每个模型都提供一个静态builder()方法,该方法返回相应的构建器对象,并允许开发人员配置所有相关设置,例如imageHeight,imageWidth。
To define an image classifier for our handwritten digit example, you configure the input handling using inputClass(BufferedImage.class). With that you specify the class which is used to represent the image.
You use imageHeight(28)
and imageWidth(28)
to resize the input image into a 28x28 shape, since that was the original size that was used for training the model.
要为我们的手写数字示例定义图像分类器,请使用inputClass(BufferedImage.class). With that you specify the class which is used to represent the image.
配置输入处理inputClass(BufferedImage.class). With that you specify the class which is used to represent the image.
inputClass(BufferedImage.class). With that you specify the class which is used to represent the image.
使用imageHeight(28)
和i mageWidth(28)
将输入图像的大小调整为28x28形状,因为那是用于训练模型的原始大小。
Once you build the classifier object, feed the input image to the classifier to recognize the image.
构建分类器对象后,将输入图像输入分类器以识别图像。
Running this code yields the following output.
运行此代码将产生以下输出。
The model identifies five possible options for the digit embedded in the image with the associated probabilities for each option. The classifier correctly predicts that the underlying digit is 0 with an overwhelming probability of 99.98%
该模型为图像中嵌入的数字标识了五个可能的选项,以及每个选项的相关概率。 分类器正确地预测基础数字为0,压倒性概率为99.98%
One obvious generalization of this case is the question of what to do when you need to detect different objects in the same image?
这种情况的一个明显概括是,当您需要检测同一图像中的不同对象时该怎么办?
使用预先训练的单发检测器(SSD)模型识别物体 (Recognizing objects using a pre-trained Single Shot Detector (SSD) model)
Single Shot Detector (SSD) is a mechanism that detects objects in images using a single deep neural network. In this example, you recognize objects in an image using a pre-trained SSD model. Object detection is a more challenging visual recognition task. In addition to classifying objects in images, object detection also identifies the location of objects in an image. It can also draw a bounding box around each object of interest along with a class (text) label.
单发检测器 (SSD)是一种使用单个深度神经网络检测图像中对象的机制。 在这 例如 ,您使用预先训练的SSD模型识别图像中的对象。 目标检测是一项更具挑战性的视觉识别任务。 除了对图像中的对象进行分类之外,对象检测还可以识别图像中对象的位置。 它还可以围绕每个感兴趣的对象以及一个类(文本)标签绘制一个边界框。
The SSD mechanism is a recent development in machine learning that detects objects surprisingly quickly, while also maintaining accuracy compared to more computationally intensive models. You can learn more about the SSD model through the Understanding SSD MultiBox — Real-Time Object Detection In Deep Learning blog post and this exercise in the Dive into Deep Learning book.
SSD机制是机器学习中的最新发展,与令人费解的计算模型相比,它出奇地快速检测对象,同时还保持了准确性。 您可以通过以下方式了解有关SSD型号的更多信息 了解SSD MultiBox —深度学习博客文章中的实时对象检测 演习在 深入学习深度学习书 。
With DJL’s implementation of JSR-381, users have access to a pre-trained implementation of the SSD model that’s ready for immediate use. DJL uses ModelZoo to simplify deploying models. In the following code block, you load a pre-trained model with the ModelZoo.loadModel(), instantiate an Object detector class and apply this model on a sample image.
通过DJL的JSR-381实施,用户可以访问可立即使用的,经过预先培训的SSD模型实施。 DJL使用ModelZoo简化了模型的部署。 在下面的代码块中,您将使用ModelZoo.loadModel()加载预训练的模型,实例化对象检测器类,然后将此模型应用于样本图像。
Here is a new image that we can use.
这是我们可以使用的新图像。
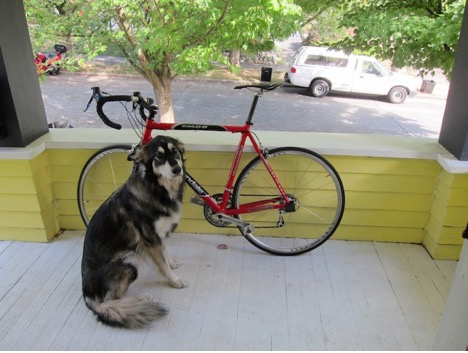
Running our code on this image yields the following result:
在此图像上运行我们的代码会产生以下结果:
If you want to add bounding boxes around each detected object onto the image, you can with only a few additional lines of code. For more information, see the complete GitHub example.The model classifies the three objects of interest (bicycle, car and dog), draws a bounding box around each, and provides a confidence level reflected by the probabilities.
如果要在图像上的每个检测到的对象周围添加边框,则只需添加几行代码即可。 有关更多信息,请参见完整的 GitHub示例 。该模型将三个感兴趣的对象(自行车,汽车和狗)分类,在每个对象周围绘制一个边界框,并提供由概率反映的置信度。
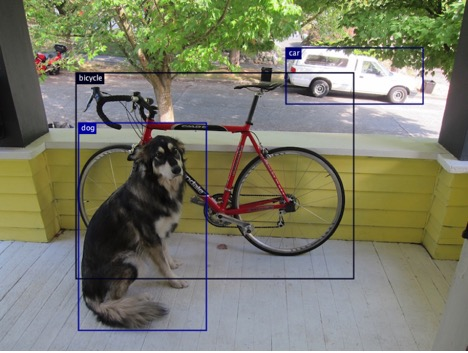
It's worth noting that detection accuracy with pre-trained models depends on the images used to train the model. The model accuracy can be improving by retraining or developing a custom model with a set of images more representative of the end application. This approach however is time consuming, and requires access to a large amount of training data. With many ML applications, it's often worth establishing a baseline with a pre-trained model. This can save a significant amount of time associated with gathering, preparing data, and training the model from scratch.
值得注意的是,预训练模型的检测精度取决于训练模型所使用的图像。 通过重新训练或开发具有一组代表最终应用程序的图像的自定义模型,可以提高模型的准确性。 但是,这种方法很耗时,并且需要访问大量的训练数据。 对于许多ML应用程序,通常值得使用预先训练的模型来建立基线。 这样可以节省大量时间,从头开始收集,准备数据和训练模型。
下一步是什么? (What’s next?)
In this post, we just scratched the surface of what you can do with the DJL implementation of the JSR-381 API. You can explore and implement many more models with the repository of pre-trained models in ModelZoo, or bring in your own model.
在本文中,我们只是简单地介绍了如何使用JSR-381 API的DJL实现。 您可以使用ModelZoo中的预训练模型库来探索和实现更多模型,也可以引入自己的模型。
We also invite you to check out DJL, an open source library built by Java developers at Amazon for the Java community. We’ve attempted to simplify developing and deploying machine learning in Java. Please join us in our mission.
我们还邀请您签出 DJL,这是由Amazon的Java开发人员为Java社区构建的开源库。 我们试图简化用Java开发和部署机器学习的过程。 请加入我们的使命。
There are many use cases for DJL, you can develop a Question Answering application for customer service, implement pose estimation on your yoga poses or train your own model to detect intruders in your backyard. Our Spring Boot starter kit also makes it straightforward to integrate ML with your Spring Boot applications. You can learn more about DJL through our introductory blog, website and repository of examples. Head over to our Github repository and collaborate with us on our Slack channel.
DJL的用例很多,您可以开发用于客户服务的Question Answering应用程序 , 对瑜伽姿势进行姿势估计 ,或者训练自己的模型以检测后院的入侵者。 我们的Spring Boot入门工具包还使将ML与Spring Boot应用程序集成起来变得非常简单。 您可以通过我们的介绍性博客 , 网站和示例存储库了解有关DJL的更多信息。 前往我们的 Github存储库,并在我们的Slack上与我们合作 渠道 。
参考资料 (References)
- Getting Started Guide - Visual Recognition (VisRec) JSR #381
- VisRec API JSR381 implementation with DJL
- Official JSR381 page
java 亚马逊 mws