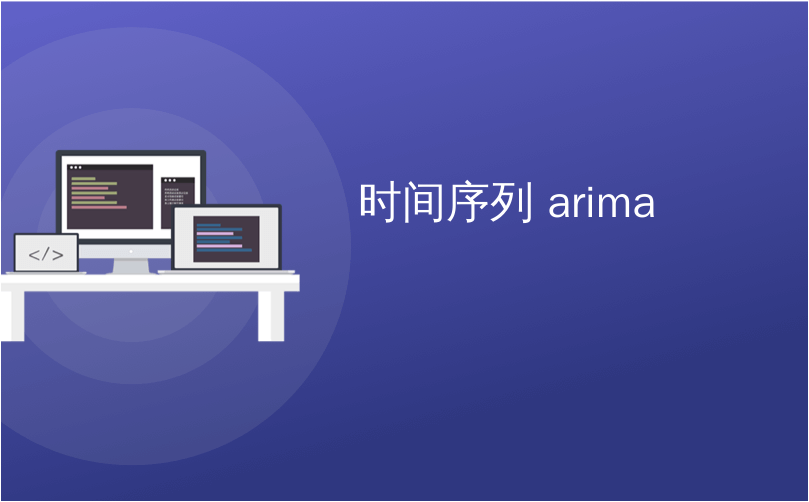
时间序列 arima
时间序列-ARIMA的变化 (Time Series - Variations of ARIMA)
In the previous chapter, we have now seen how ARIMA model works, and its limitations that it cannot handle seasonal data or multivariate time series and hence, new models were introduced to include these features.
在上一章中,我们现在看到了ARIMA模型的工作原理,以及它不能处理季节性数据或多元时间序列的局限性,因此引入了包含这些功能的新模型。
A glimpse of these new models is given here −
这些新模型的概览在这里给出-
向量自回归(VAR) (Vector Auto-Regression (VAR))
It is a generalized version of auto regression model for multivariate stationary time series. It is characterized by ‘p’ parameter.
它是用于多元平稳时间序列的自动回归模型的通用版本。 它以“ p”参数为特征。
向量移动平均线(VMA) (Vector Moving Average (VMA))
It is a generalized version of moving average model for multivariate stationary time series. It is characterized by ‘q’ parameter.
它是用于多元平稳时间序列的移动平均模型的广义版本。 它以“ q”参数为特征。
向量自回归移动平均值(VARMA) (Vector Auto Regression Moving Average (VARMA))
It is the combination of VAR and VMA and a generalized version of ARMA model for multivariate stationary time series. It is characterized by ‘p’ and ‘q’ parameters. Much like, ARMA is capable of acting like an AR model by setting ‘q’ parameter as 0 and as a MA model by setting ‘p’ parameter as 0, VARMA is also capable of acting like an VAR model by setting ‘q’ parameter as 0 and as a VMA model by setting ‘p’ parameter as 0.
它是VAR和VMA的组合,以及用于多元平稳时间序列的ARMA模型的广义版本。 它的特征是“ p”和“ q”参数。 很像,ARMA可以通过将“ q”参数设置为0来充当AR模型,而通过将“ p”参数设置为0来作为MA模型,VARMA也可以通过设置“ q”参数来充当VAR模型。通过将“ p”参数设置为0,将其设置为0并作为VMA模型。
I