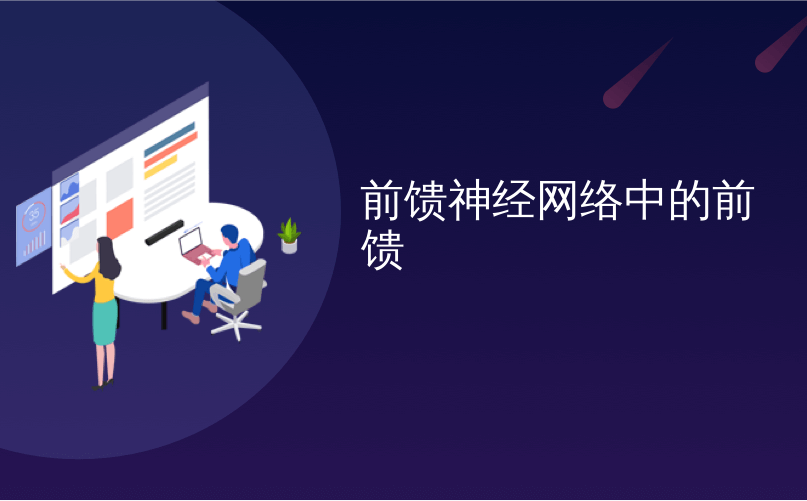
前馈神经网络中的前馈
PyBrain-使用前馈网络 (PyBrain - Working with Feed-Forward Networks)
A feed-forward network is a neural network, where the information between nodes moves in the forward direction and will never travel backward. Feed Forward network is the first and the simplest one among the networks available in the artificial neural network. The information is passed from the input nodes, next to the hidden nodes and later to the output node.
前馈网络是一个神经网络,其中节点之间的信息沿向前方向移动,并且永远不会向后传播。 前馈网络是人工神经网络中可用的网络中的第一个和最简单的网络。 信息从输入节点传递到隐藏节点之后,再传递到输出节点。
In this chapter we are going to discuss how to −
在本章中,我们将讨论如何-
- Create Feed-Forward Networks 创建前馈网络
- Add Connection and Modules to FFN 将连接和模块添加到FFN
创建前馈网络 (Creating a Feed Forward Network)
You can use the python IDE of your choice, i.e., PyCharm. In this, we are using Visual Studio Code to write the code and will execute the same in terminal.
您可以使用自己选择的python IDE,即PyCharm。 在此,我们使用Visual Studio Code编写代码,并将在终端中执行相同的代码。
To create a feedforward network, we need to import it from pybrain.structure as shown below −
要创建前馈网络,我们需要从pybrain.structure导入它,如下所示-
ffn.py (ffn.py)
from pybrain.structure import FeedForwardNetwork
network = FeedForwardNetwork()
print(network)
Execute ffn.py as shown below −
执行如下所示的ffn.py-
C:\pybrain\pybrain\src>python ffn.py
FeedForwardNetwork-0
Modules:
[]
Connections:
[]
We have not added any modules and connections to the feedforward network. Hence the network shows empty arrays for Modules and Connections.
我们尚未向前馈网络添加任何模块和连接。 因此,网络显示模块和连接的空数组。
添加模块和连接 (Adding Modules and Connections)
First we will create input, hidden, output layers and add the same to the modules as shown below −
首先,我们将创建输入,隐藏,输出层,并将其添加到模块中,如下所示:
ffy.py (ffy.py)
from pybrain.structure import FeedForwardNetwork
from pybrain.structure import LinearLayer, SigmoidLayer
network = FeedForwardNetwork()
#creating layer for input => 2 , hidden=> 3 and output=>1
inputLayer = LinearLayer(2)
hiddenLayer = SigmoidLayer(3)
outputLayer = LinearLayer(1)
#adding the layer to feedforward network
network.addInputModule(inputLayer)
network.addModule(hiddenLayer)
network.addOutputModule(outputLayer)
print(network)
输出量 (Output)
C:\pybrain\pybrain\src>python ffn.py
FeedForwardNetwork-3
Modules:
[]
Connections:
[]
We are still getting the modules and connections as empty. We need to provide a connection to the modules created as shown below −
我们仍然使模块和连接为空。 我们需要提供与创建的模块的连接,如下所示:
Here is the code where we have created a connection between input, hidden and output layers and add the connection to the network.
这是我们在输入,隐藏和输出层之间创建连接并将代码添加到网络的代码。
ffy.py (ffy.py)
from pybrain.structure import FeedForwardNetwork
from pybrain.structure import LinearLayer, SigmoidLayer
from pybrain.structure import FullConnection
network = FeedForwardNetwork()
#creating layer for input => 2 , hidden=> 3 and output=>1
inputLayer = LinearLayer(2)
hiddenLayer = SigmoidLayer(3)
outputLayer = LinearLayer(1)
#adding the layer to feedforward network
network.addInputModule(inputLayer)
network.addModule(hiddenLayer)
network.addOutputModule(outputLayer)
#Create connection between input ,hidden and output
input_to_hidden = FullConnection(inputLayer, hiddenLayer)
hidden_to_output = FullConnection(hiddenLayer, outputLayer)
#add connection to the network
network.addConnection(input_to_hidden)
network.addConnection(hidden_to_output)
print(network)
输出量 (Output)
C:\pybrain\pybrain\src>python ffn.py
FeedForwardNetwork-3
Modules:
[]
Connections:
[]
We are still not able to get the modules and connections. Let us now add the final step, i.e., we need to add the sortModules() method as shown below −
我们仍然无法获得模块和连接。 现在让我们添加最后一步,即,我们需要添加sortModules()方法,如下所示:
ffy.py (ffy.py)
from pybrain.structure import FeedForwardNetwork
from pybrain.structure import LinearLayer, SigmoidLayer
from pybrain.structure import FullConnection
network = FeedForwardNetwork()
#creating layer for input => 2 , hidden=> 3 and output=>1
inputLayer = LinearLayer(2)
hiddenLayer = SigmoidLayer(3)
outputLayer = LinearLayer(1)
#adding the layer to feedforward network
network.addInputModule(inputLayer)
network.addModule(hiddenLayer)
network.addOutputModule(outputLayer)
#Create connection between input ,hidden and output
input_to_hidden = FullConnection(inputLayer, hiddenLayer)
hidden_to_output = FullConnection(hiddenLayer, outputLayer)
#add connection to the network
network.addConnection(input_to_hidden)
network.addConnection(hidden_to_output)
network.sortModules()
print(network)
输出量 (Output)
C:\pybrain\pybrain\src>python ffn.py
FeedForwardNetwork-6
Modules:
[<LinearLayer 'LinearLayer-3'gt;, <SigmoidLayer 'SigmoidLayer-7'>,
<LinearLayer 'LinearLayer-8'>]
Connections:
[<FullConnection 'FullConnection-4': 'SigmoidLayer-7' -> 'LinearLayer-8'>,
<FullConnection 'FullConnection-5': 'LinearLayer-3' -> 'SigmoidLayer-7'>]
We are now able to see the modules and the connections details for feedforwardnetwork.
现在,我们可以看到feedforwardnetwork的模块和连接详细信息。
翻译自: https://www.tutorialspoint.com/pybrain/pybrain_working_with_feed_forward_networks.htm
前馈神经网络中的前馈