多模态预训练论文
ViLBERT: Pretraining Task-Agnostic Visiolinguistic Representations for Vision-and-Language Tasks
vision-and-language tasks:
visual question answering,visual commonsense reasoning, referring expressions, and caption-based image
retrieval and a special experiment setting
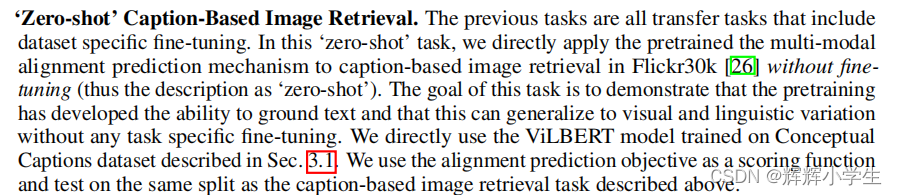
key technical innovation:
introducing separate streams for vision and language processing that communicate through co-attentional transformer layers.
why two-stream?
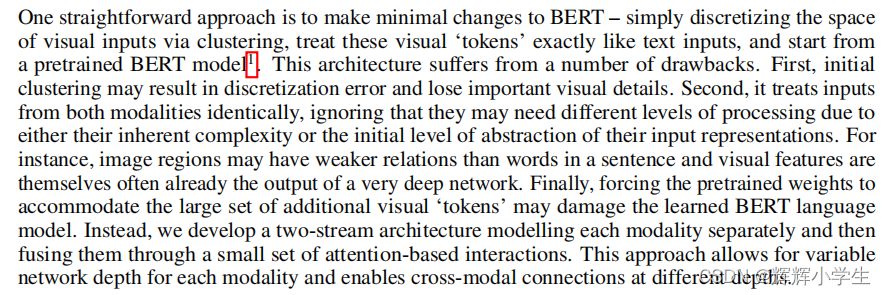
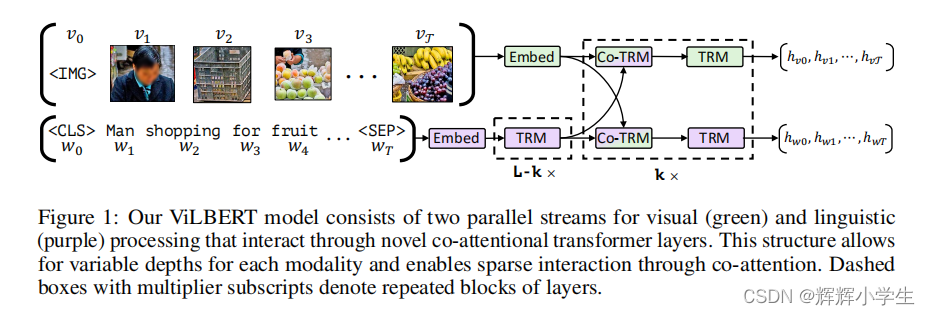
notes:
Given an image
I
represented as a set of region features v
1
, . . . , v
T
and a text input
w
0
, . . . , w
T
, our model outputs fifinal representations
h
v
0
, . . . , h
v
T
and
h
w
0
, . . . , h
wT
. Notice that
exchange between the two streams is restricted to be between specifific
layers and that the text stream has signifificantly more processing before interacting with visual features
– matching our intuitions that our chosen visual features are already fairly high-level and require
limited context-aggregation compared to words in a sentence.
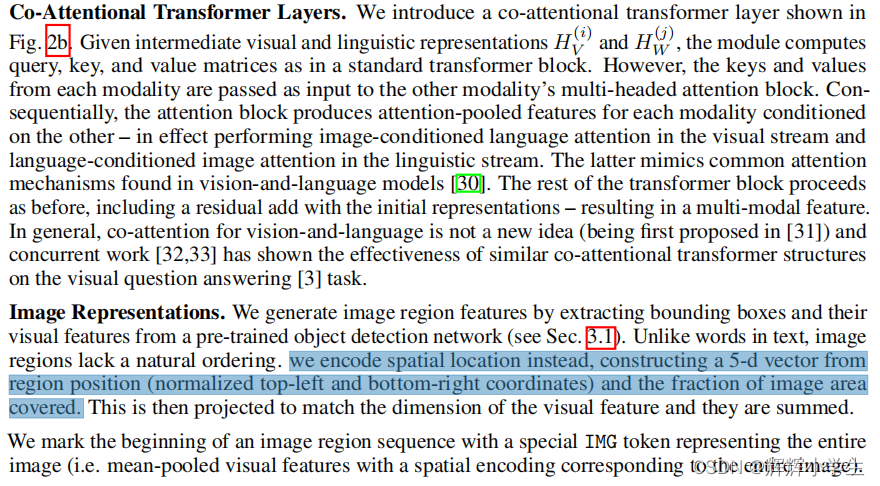
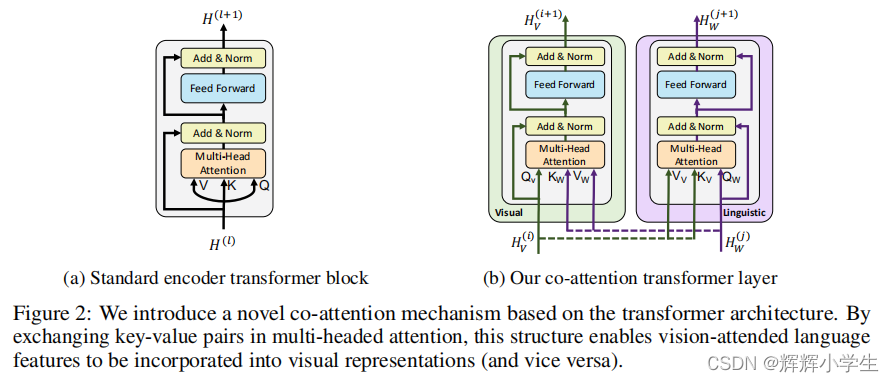
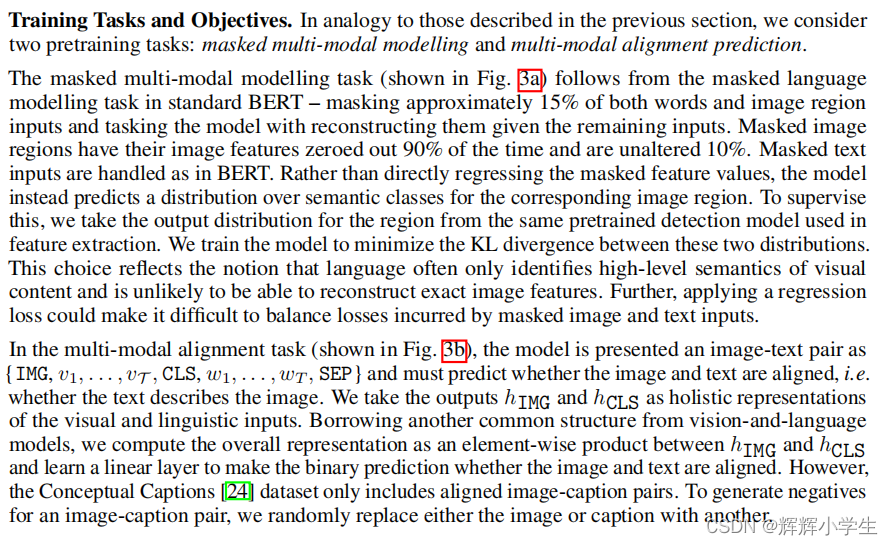
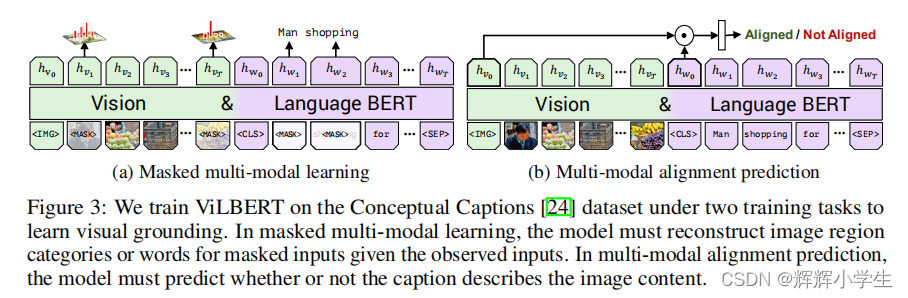

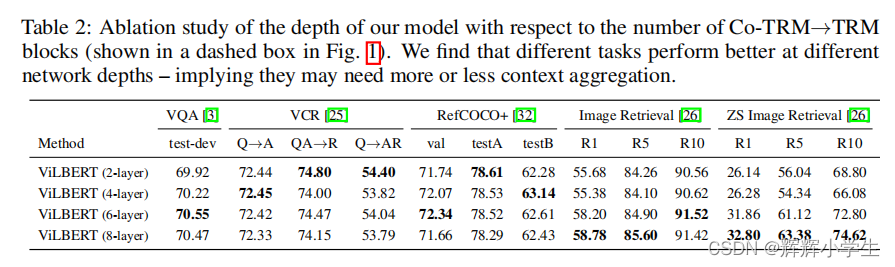
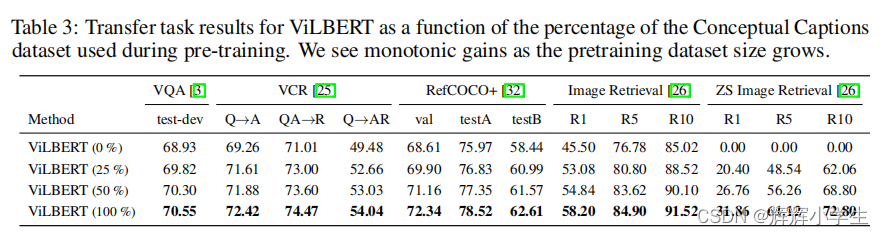
The first work is over.
V
ISUAL
BERT: A Simple And Performant Baseline For Vision And Language
two
visually-grounded
language model objectives for pre-training:
(1) part of the text is
masked and the model learns to predict the masked words based on the remaining text and visual
context;
(2) the model is trained to determine whether the provided text matches the image. We
show that such pre-training on image caption data is important for VisualBERT to learn transferable
text and visual representations.
conduct comprehensive experiments on four vision-and-language tasks:VQA VCR NLVR
regionto-phrase grounding
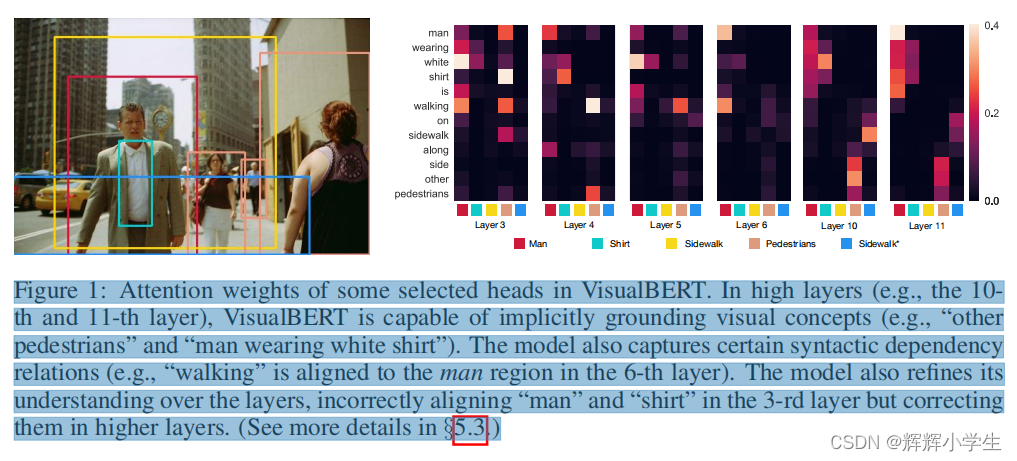
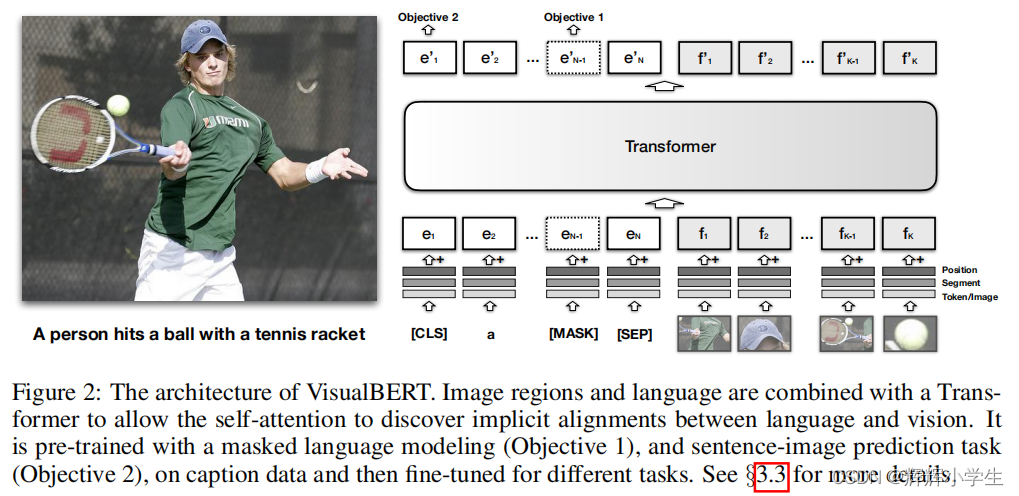
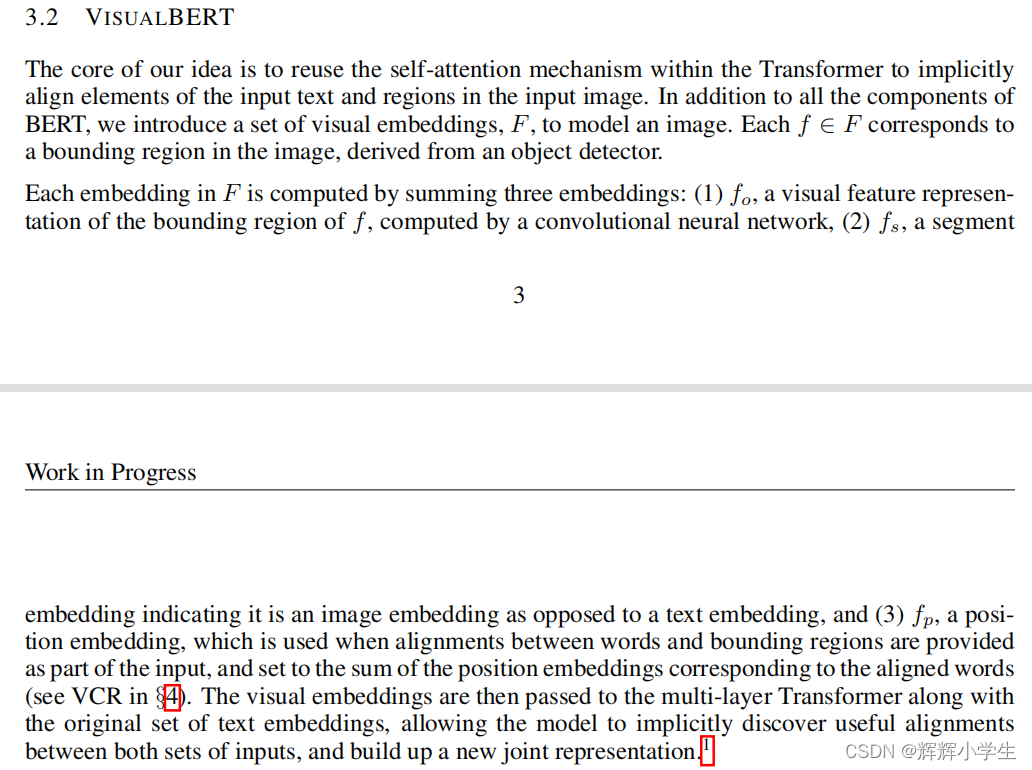
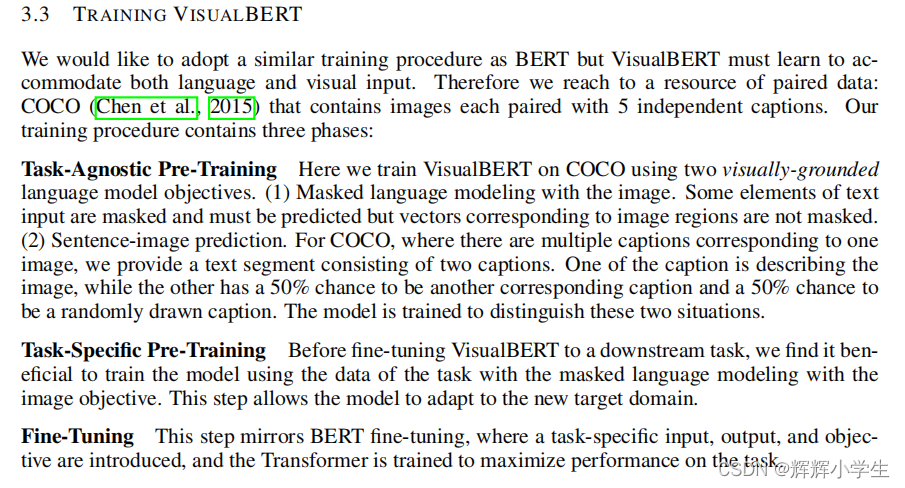
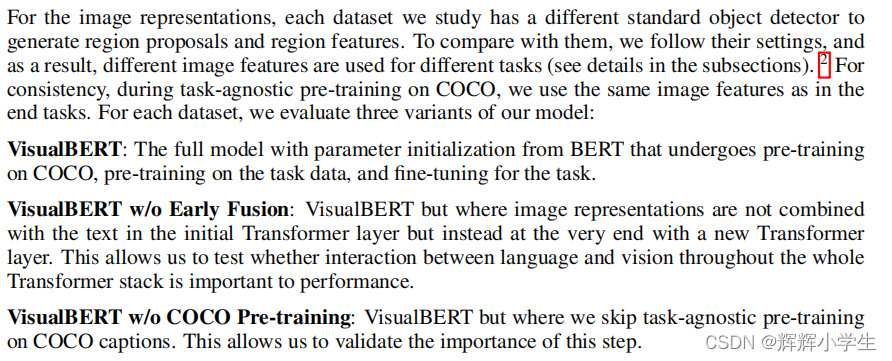
The second work is over.
Unicoder-VL: A Universal Encoder for Vision and Language by Cross-Modal Pre-Training
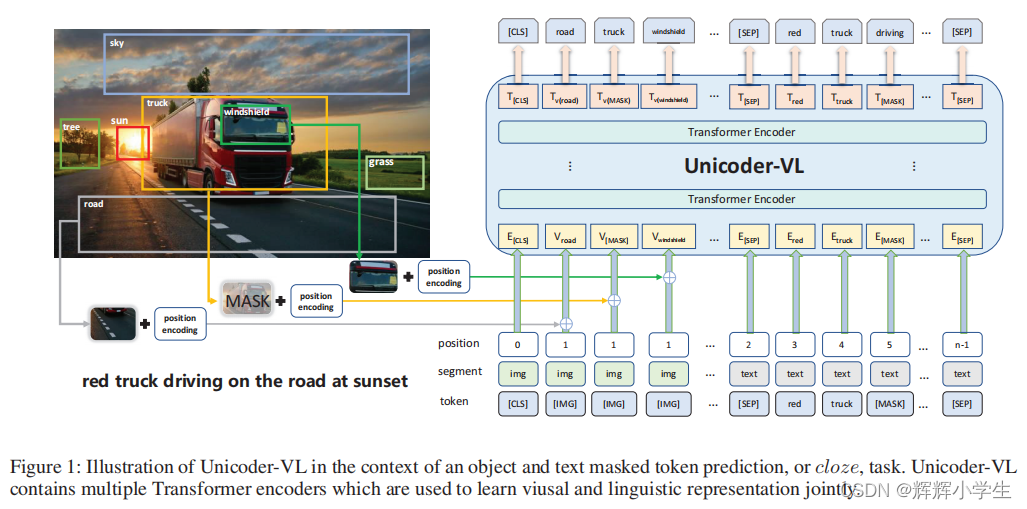
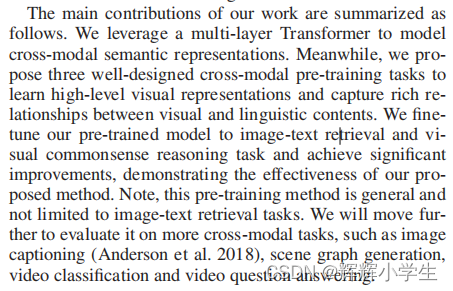
approach
Pre-training Tasks:MLM MOC VLM
Fine-tune on Downstream Tasks:Image-Text Retrieval.Visual Commonsense Reasoning.and
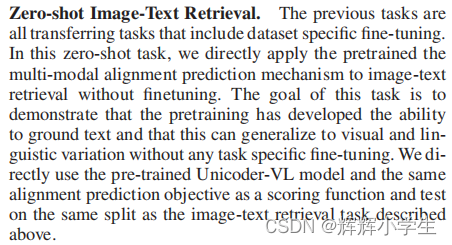
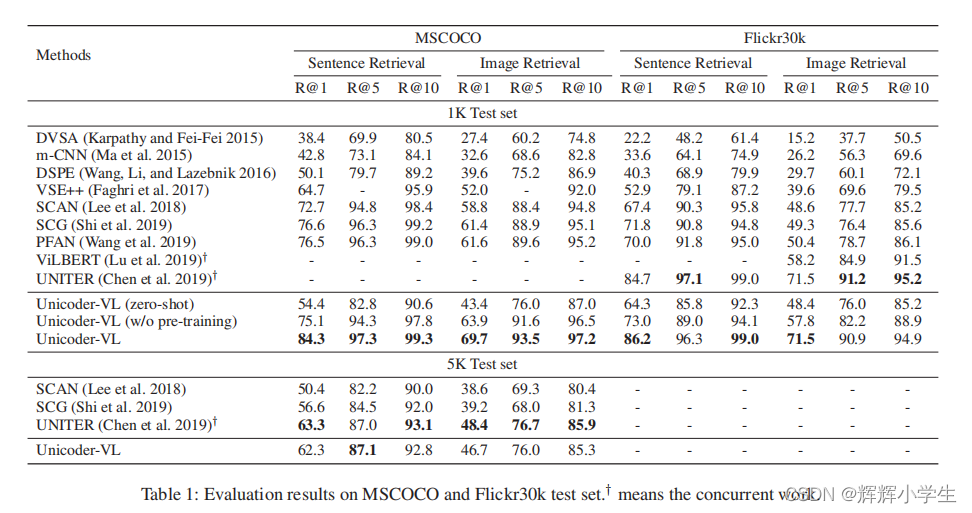
The third word is over.
LXMERT: Learning Cross-Modality Encoder Representations from Transformers
It consists of three Transformer
: encoders: an object
relationship encoder, a language encoder, and across-modality encoder.
pre-train our model with fifive diverse representative tasks:
(1) masked cross
modality language modeling
(2) masked object
prediction via RoI-feature regression
(3) masked
object prediction via detected-label classifification,
(4) cross-modality matching
(5) image question answering.
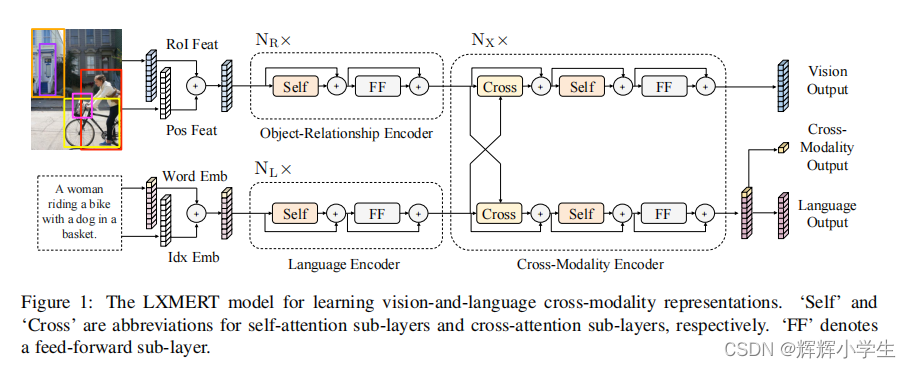
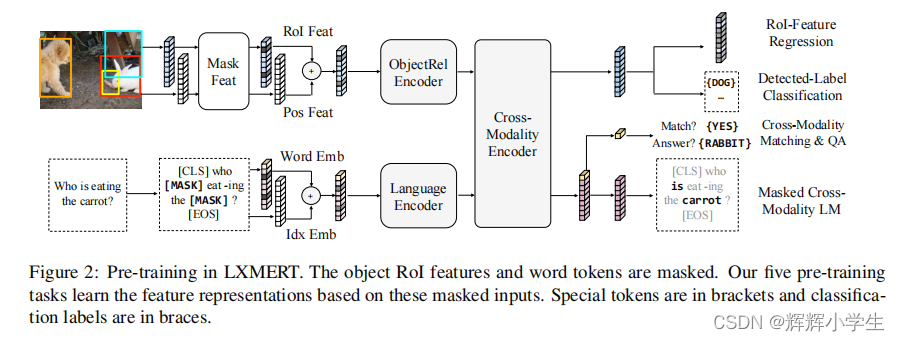
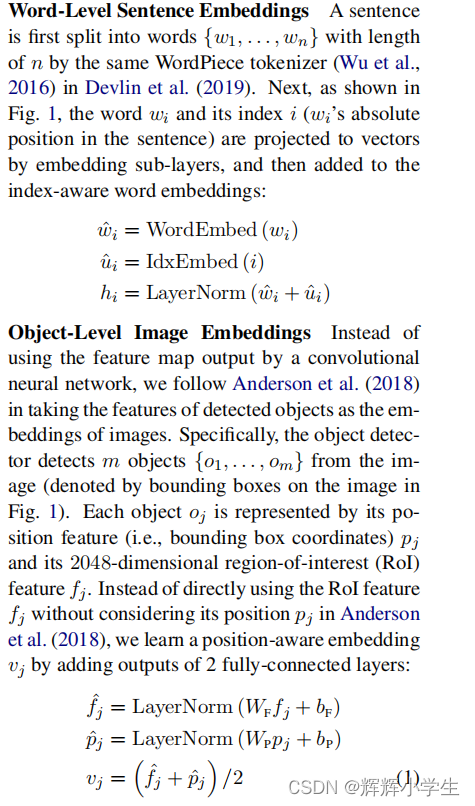
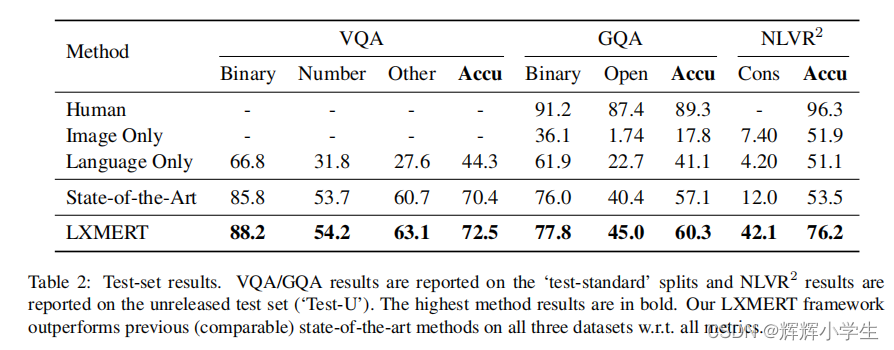
over