0 前言
来自官方教程,对于萌新学习用Pytorch做NLP任务有很大的帮助,就翻译过来,顺便自己Mark一下,因为打开官网有时候太慢了,还是看自己写的Blog比较快。另外,之前在做⭐ 李宏毅2020机器学习作业4-RNN:句子情感分类的时候,代码看起来有些难度。之前的几个作业都还能看懂,但是作业4实在跳跃度太大了,就先拿这几个练个手。
这是官方教程中同一个系列的文章,总共有3篇:
- 第一篇,教你搭建一个字母级别(character-level)的RNN,对名字进行分类,是一个分类的任务。
- 第二篇,教你搭建一个字母级别(character-level)的RNN,生成名字,是一个自然语言生成的任务。
- 第三篇,教你搭建一个Seq2Seq的RNN,进行机器翻译,也是一个自然语言生成的任务。Seq2Seq是序列到序列的模型,类似于单词级别(word-level)的RNN。
博主更新完的本系列文章:
- 第一篇:【Pytorch官方教程】从零开始自己搭建RNN1 - 字母级RNN的分类任务
- 第二篇:【Pytorch官方教程】从零开始自己搭建RNN2 - 字母级RNN的生成任务
- 第三篇:【Pytorch官方教程】从零开始自己搭建RNN3 - 含注意力机制的Seq2Seq机器翻译模型
1 数据与说明
数据下载
数据下载链接:点击下载
数据是一个data.zip压缩包,解压后的目录树如下所示:
D:.
│ eng-fra.txt
│
└─names
Arabic.txt
Chinese.txt
Czech.txt
Dutch.txt
English.txt
French.txt
German.txt
Greek.txt
Irish.txt
Italian.txt
Japanese.txt
Korean.txt
Polish.txt
Portuguese.txt
Russian.txt
Scottish.txt
Spanish.txt
Vietnamese.txt
eng-fra.txt
是第三篇翻译任务中要用到的,这次我们只用到 /name
这个文件夹下的18个文件,每个文件以语言命名,格式为:[Language].txt
。打开后,里面是该语言中常用的姓/名。
比如:打开我们最熟悉的 Chinese.txt
,可以看到每一行是一个姓或者名(有一些姓/名确实有点点奇怪,但整体来说问题不大)。
Ang
Au-Yong
Bai
Ban
Bao
Bei
Bian
Bui
Cai
Cao
Cen
……
任务说明
这次任务的目标是,输入一个姓名,根据它的拼写,用循环神经网络对它分类,判断它属于哪个语言里的姓名。
比如:
$ python predict.py Hinton
(-0.47) Scottish
(-1.52) English
(-3.57) Irish
$ python predict.py Schmidhuber
(-0.19) German
(-2.48) Czech
(-2.68) Dutch
- Hinton 这个姓名很有可能是Scottish,其次可能是English,再其次可能是Irish。
- Schmidhuber 这个姓名很有可能是German,其次可能是Czech,再其次可能是Dutch。
2 基础原理
RNN
在讲解代码前,我们简单回顾/了解一下循环神经网络。如果你已经了解,直接可以跳过。
一般的神经网络都是单向的,一层连着下一层。而循环神经网络(Recurrent Neural Network)和它的名字一样,里面引入了循环体结构,就像我们写代码的 for
或者 while
循环一样,某一步的循环体就像下面这样:
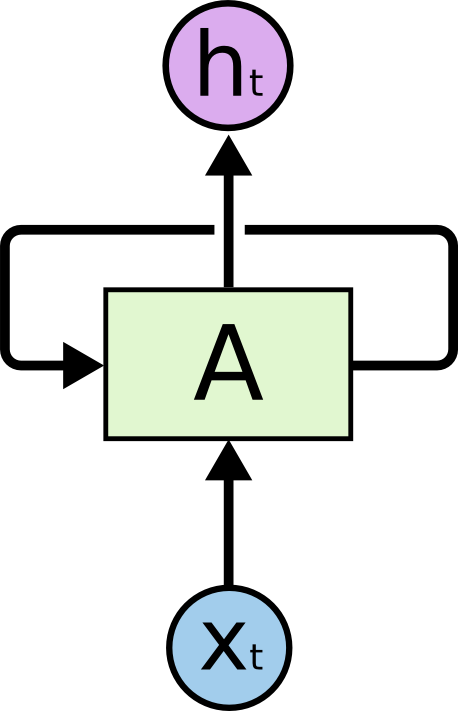
x t x_t xt是第 t 步循环时的输入, h t h_t ht是第 t 步循环的输出,它们都是向量,不是标量(一个数值)。这样一个循环体就可以把信息从上一步传递到下一步。不过,这样的循环体看起来不太好懂,让我们把它按时序展平(降维攻击!!!),变成一般的神经网络那样的单向传播结构。展开后就是一个链状结构:
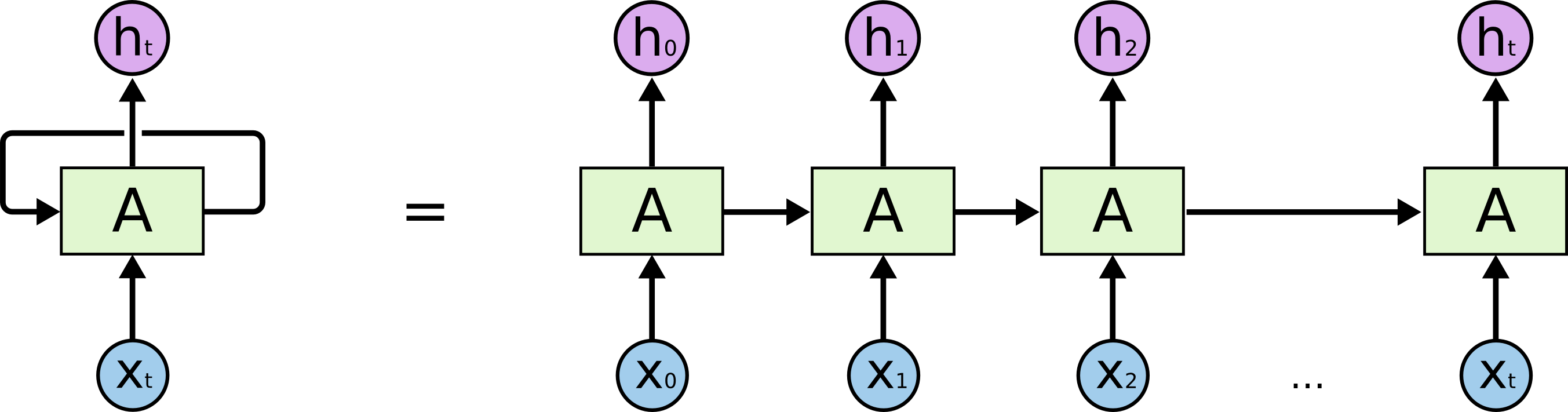
这样我们就可以看到,从第0步到第t步之间都发生了一些什么。每一个A
块里的东西都是一样的,你可以理解成 for (i=0; i<t; i++)
或者 for i in range(t)
块中的代码。所以,我们只需要写某一步的变量更新方式,然后让它循环就可以了。
现在的问题是:变量到底应该怎么更新?输入的
x
t
x_t
xt应该如何处理,才能变成输出的
h
t
h_t
ht?图里的 A
内部具体的更新结构如下:
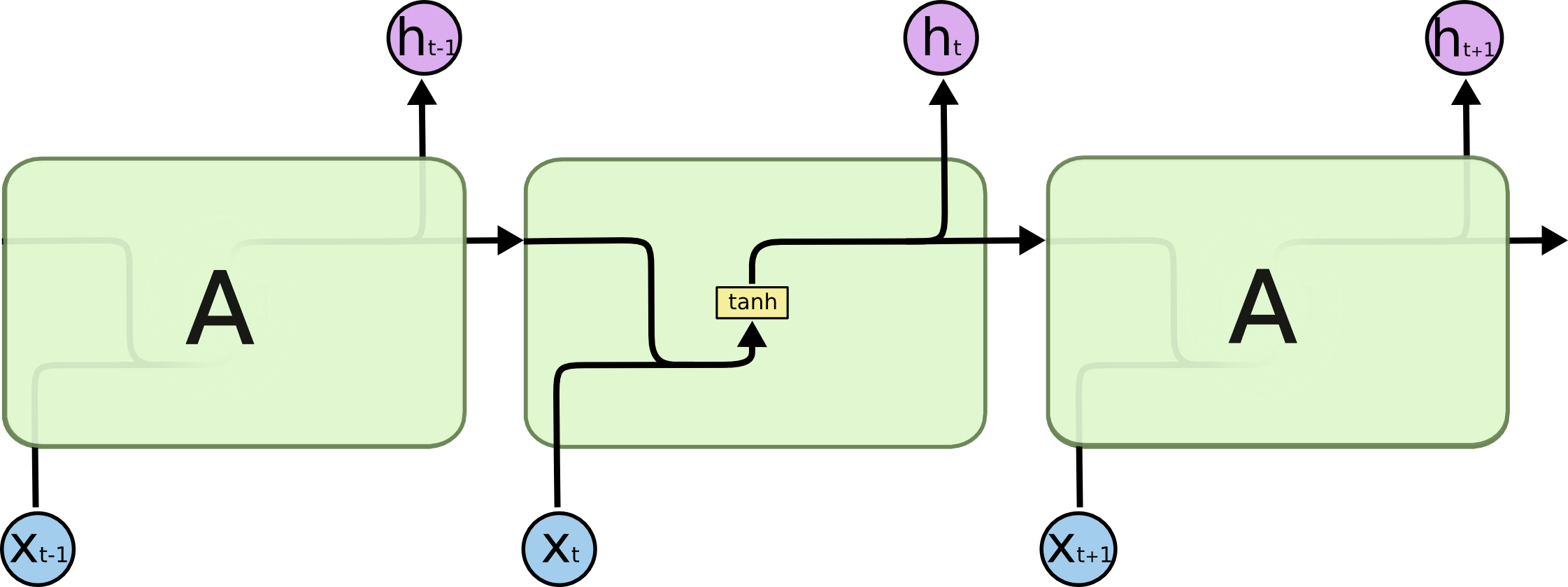
流程如下:
- 把上一步输出的
h
t
−
1
h_{t-1}
ht−1乘上一个权重矩阵
W
h
W_h
Wh,变成
W
h
h
t
−
1
W_hh_{t-1}
Whht−1。
h
是隐藏层(hidden layer)的简写 - 这一步输入的
x
t
x_t
xt也乘上一个权重矩阵
W
i
W_i
Wi,变成
W
i
x
t
W_ix_{t}
Wixt。
i
是输入(input)的简写 - 把它们相加,变成 W h h t − 1 + W i x t W_hh_{t-1}+W_ix_{t} Whht−1+Wixt
- 经过一个 tanh ( ⋅ ) \tanh(·) tanh(⋅) 函数的处理,就得到了这一步的输出: h t = tanh ( W h h t − 1 + W i x t ) h_t = \tanh(W_hh_{t-1}+W_ix_{t}) ht=tanh(Whht−1+Wixt)
把流程1到流程4反复循环,就是一个最简单的RNN。
如果你看到了这里,恭喜你!你已经学会RNN了!
如果你还不会,我们再演示下一遍循环:
- 把上一步输出的 h t h_{t} ht乘上一个权重矩阵 W h W_h Wh,变成 W h h t W_hh_{t} Whht
- 这一步输入的 x t + 1 x_{t+1} xt+1也乘上一个权重矩阵 W i W_i Wi,变成 W i x t + 1 W_ix_{t+1} Wixt+1
- 把它们相加,变成 W h h t + W i x t + 1 W_hh_{t}+W_ix_{t+1} Whht+Wixt+1
- 经过一个 tanh ( ⋅ ) \tanh(·) tanh(⋅) 函数的处理,就得到了这一步的输出: h t + 1 = tanh ( W h h t + W i x t + 1 ) h_{t+1} = \tanh(W_hh_{t}+W_ix_{t+1}) ht+1=tanh(Whht+Wixt+1)
你会发现,变化的只有 h t h_{t} ht 和 x t + 1 x_{t+1} xt+1 ,它们的下标从 t − 1 t-1 t−1 变成了 t t t,从 t t t 变成了 t + 1 t+1 t+1,而 W h W_h Wh 和 W i W_i Wi 并没有与时间步 t t t 相关的下标,始终只有这两个矩阵,当然,你在训练过程中,这两个矩阵中的数字会发生变化,因为它们是模型要学习的“参数”。
另外,你可能会看到一种带有偏置向量 b b b 的更新方式:
h t = tanh ( ( W h h t − 1 + b h ) + ( W i x t + b i ) ) h_t = \tanh((W_hh_{t-1}+b_h)+(W_ix_{t}+b_i)) ht=tanh((Whht−1+bh)+(Wixt+bi))
我们这里进行了简化,即令所有的向量 b b b 都为0。另外,我们在初始化向量 h 0 h_0 h0的时候,也会把它初始化成全为0的向量。
RNN这样一个结构用来处理有前后关联的序列非常有效,因此在自然语言处理里也取得了不错的成绩。因为一句话可以看成是许多词组成的序列,这些词之前有前后文/上下文关系。
LSTM
不过,普通的RNN有一个长短句依赖的问题(不细讲了,反正就是不太好使),所以有人提出了LSTM来改进RNN。LSTM是长短期记忆网络(LSTM,Long Short-Term Memory),通过三个门(遗忘门、输入门、输出门)的控制,存储短期记忆或长期记忆。它的整体流程还是这样:
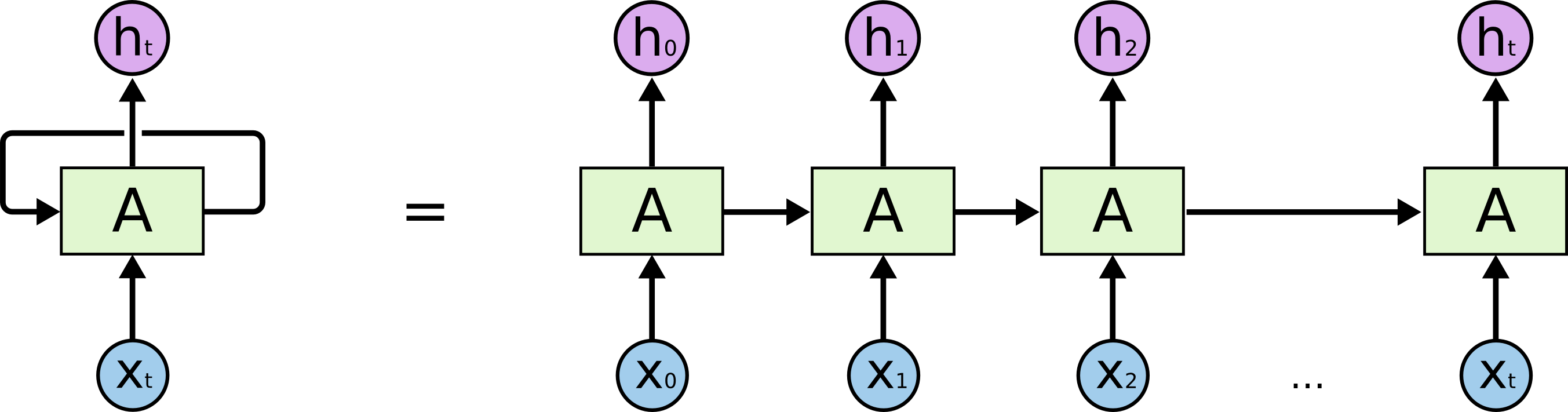
但是,LSTM里的一个 A
内部的结构变成了这样子:
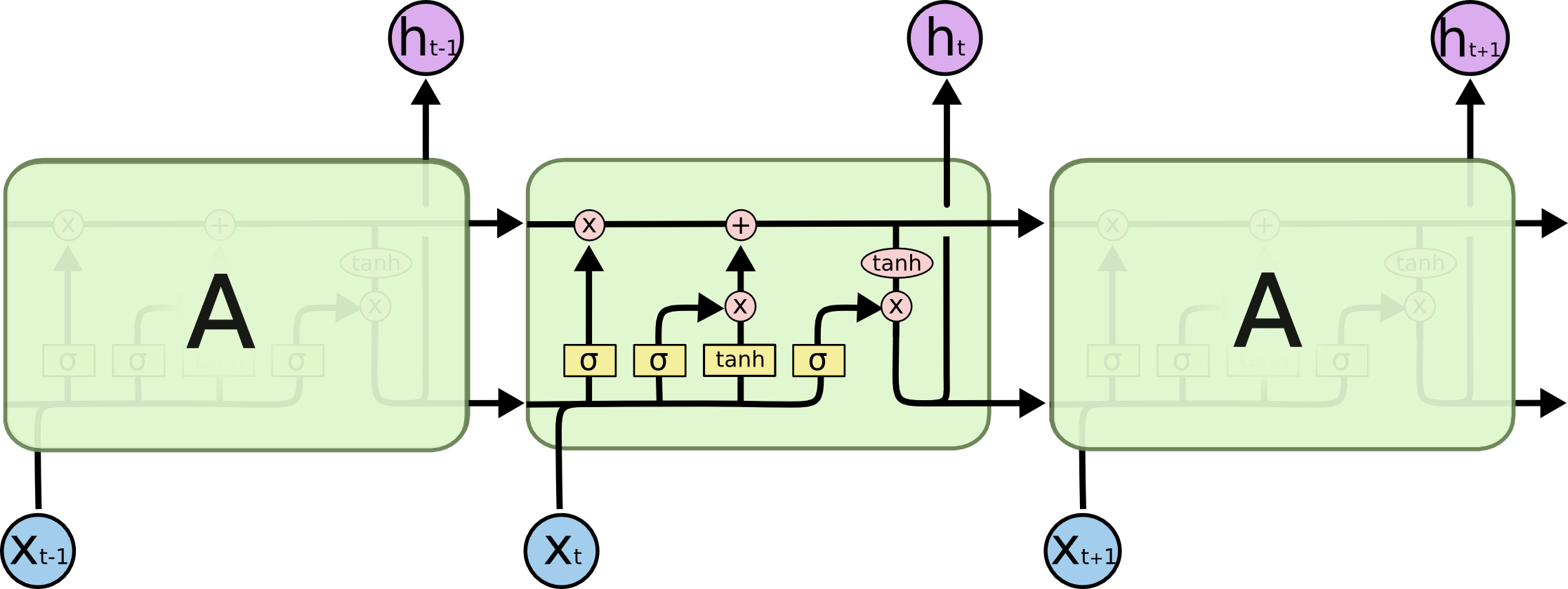
自从RNN整容成LSTM后,你再也不认识它了……
说实话,这张图美则美矣,我觉得还是李宏毅老师的简化版容易入门,一起贴上来吧!图里省略了几个
tanh
(
⋅
)
\tanh(·)
tanh(⋅) 函数,更方便理解:
图中是第 t 步的更新情况, 就是
σ
(
⋅
)
\sigma(·)
σ(⋅)的S型曲线,即sigmoid函数:
σ ( x ) = 1 1 + e − x \sigma(x)=\frac{1}{1+e^{-x}} σ(x)=1+e−x1
画出来就长这样了:
我们先看李宏毅老师的高级简化版:
对同一个输入 x t x_t xt,乘上不同的权重 W f W_f Wf、 W i W_i Wi、 W W W、 W o W_o Wo就变成了四个不同的值:
- z f = W f x t z_f=W_fx_t zf=Wfxt,是遗忘门(Forget Gate)的输入
- z i = W i x t z_i=W_ix_t zi=Wixt,是输入门(Input Gate)的输入
- z = W x t z=Wx_t z=Wxt,是真正的输入(和输入门的输入是不一样的)
- z o = W o x t z_o=W_ox_t zo=Woxt,是输出门(Output Gate)的输入
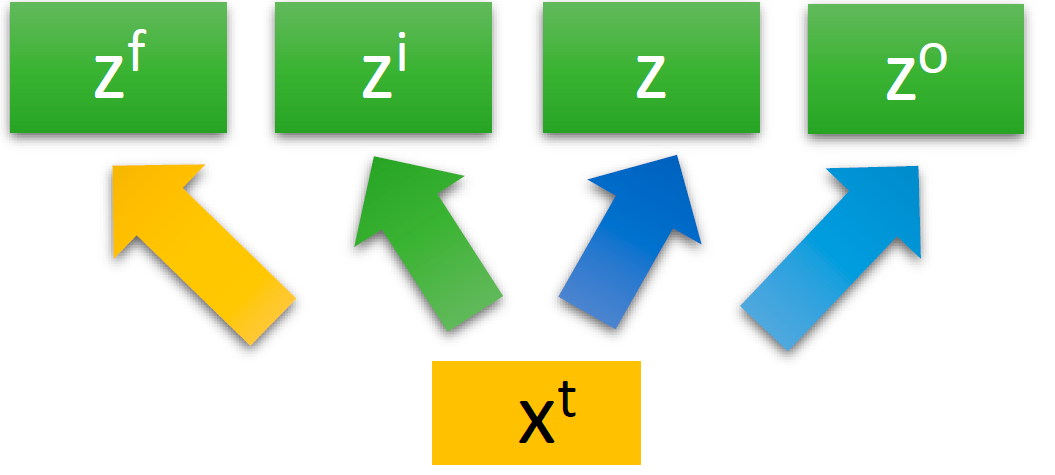
右边的图从下往上看,我们先来看 红配绿 红色方框圈出来的部分,输入和输入门的更新,它负责判断是否要接受新的输入:
- z i z_i zi乘以权重 W i W_i Wi,加上偏置向量 b i b_i bi,经过输入门,变成了 i t = σ ( W i z i + b i ) i_t = \sigma(W_iz_i+b_i) it=σ(Wizi+bi)
- z z z乘以权重 W c W_c Wc,加上偏置向量 b c b_c bc,经过 tanh ( ⋅ ) \tanh(·) tanh(⋅),变成了 c ~ t = σ ( W c z + b c ) \tilde{c}_t=\sigma(W_cz+b_c) c~t=σ(Wcz+bc)。这里的 c c c是上面的cell的简写,它是一个存储单元
- 把 i t i_t it 和 c ~ t \tilde{c}_t c~t 按元素相乘(Hadamard乘积,运算符为 ⊙ \odot ⊙或 ∗ * ∗),得到 i t ∗ c ~ t i_t*\tilde{c}_t it∗c~t,然后输入cell
以上就这样完了。
Hadamard乘积:
假设输入矩阵 A = { a i j } A=\{a_{ij}\} A={aij}, B = { b i j } B=\{b_{ij}\} B={bij},都是大小为 m × n m\times n m×n的矩阵,那么
A ∗ B = [ a 11 b 11 a 12 b 12 ⋯ a 1 n b 1 n a 21 b 21 a 22 b 22 ⋯ a 2 n b 2 n ⋮ ⋮ ⋮ a m 1 b m 1 a m 2 b m 2 ⋯ a m n b m n ] A* B=\left[\begin{array}{cccc} a_{11} b_{11} & a_{12} b_{12} & \cdots & a_{1 n} b_{1 n} \\ a_{21} b_{21} & a_{22} b_{22} & \cdots & a_{2 n} b_{2 n} \\ \vdots & \vdots & & \vdots \\ a_{m 1} b_{m 1} & a_{m 2} b_{m 2} & \cdots & a_{m n} b_{m n} \end{array}\right] A∗B=⎣⎢⎢⎢⎡a11b11a21b21⋮am1bm1a12b12a22b22⋮am2bm2⋯⋯⋯a1nb1na2nb2n⋮amnbmn⎦⎥⎥⎥⎤
然后,我们来看看蓝色圈出来的部分,是遗忘门的更新,它负责判断是否要更新cell中的值,如果更新了,就要忘记之前的值,写入新的值:
- cell中存放了上一步的存储向量 c t − 1 c_{t-1} ct−1
- z f z_f zf乘以权重 W f W_f Wf,加上偏置向量 b f b_f bf,经过遗忘门,变成了 f t = σ ( W f z f + b f ) f_t=\sigma(W_fz_f+b_f) ft=σ(Wfzf+bf)
- 在cell中,把 c t − 1 c_{t-1} ct−1 和 f t f_t ft同样按元素相乘,然后和刚才提到的 i t ∗ c ~ t i_t*\tilde{c}_t it∗c~t相加,就变成了新的存储向量 c t = c t − 1 ∗ f t + i t ∗ c ~ t c_t=c_{t-1}* f_t+i_t*\tilde{c}_t ct=ct−1∗ft+it∗c~t
最后,我们来看一下橙色圈出来的部分,输出门的更新,它负责判断是否要输出最后的值:
- 新的 c t c_t ct 先通过一个 tanh ( ⋅ ) \tanh(·) tanh(⋅) 函数,变成 tanh ( c t ) \tanh(c_t) tanh(ct)
- z o z_o zo乘以权重 W o W_o Wo,加上偏置向量 b o b_o bo,经过输出门,变成了 o t = σ ( W o z o + b o ) o_t=\sigma(W_oz_o+b_o) ot=σ(Wozo+bo)
- 把 tanh ( c t ) \tanh(c_t) tanh(ct) 和 o t o_t ot 按元素相乘,得到新的隐藏层状态 h t = o t ∗ tanh ( c t ) h_t=o_t*\tanh(c_t) ht=ot∗tanh(ct)
- 隐藏层状态 h t h_t ht 通过一个softmax函数,得到最后的输出 y t = s o f t m a x ( h t ) y_t=softmax(h_t) yt=softmax(ht)
以上,就是LSTM中某一步的状态更新情况。我们再回头看这张图:
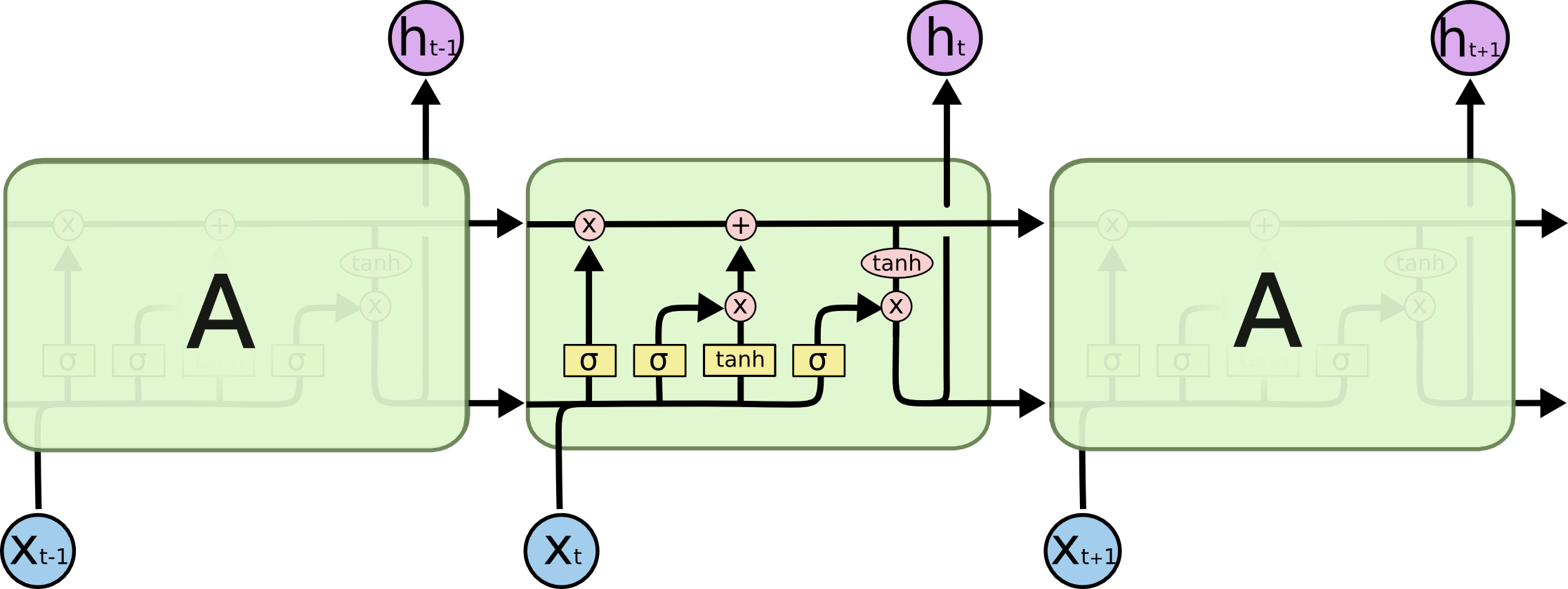
之前, z f z_f zf、 z i z_i zi、 z z z、 z o z_o zo都是用 x t x_t xt 乘以不同的权重得到的。但是,光凭 x t x_t xt ,不足以传递足够多的信息,我们把 x t x_t xt 和上一步输出的隐藏层状态 h t − 1 h_{t-1} ht−1 拼在一起,变成一个新的输入向量 [ x t , h t − 1 ] [x_t, h_{t-1}] [xt,ht−1]。上面的更新公式变成了下面这样:
说到这里,所谓的“门”,实际上就是一个
σ
(
⋅
)
\sigma(·)
σ(⋅) 或者
tanh
(
⋅
)
\tanh(·)
tanh(⋅) 函数。不过,一个好的命名更便于装X 形象化地理解。
因为 LSTM 实在比 RNN 优秀太多,所以我们一般称循环神经网络的时候,其实都是在说LSTM。
GRU
因为LSTM太复杂,而且容易过拟合,有一个简化版的LSTM,叫做GRU,它把遗忘门和输入门合并成了一个更新门,从三个门减少到了两个门,更新公式如下:
这里省略了偏置向量
b
b
b。
one-hot 编码
刚才说到,输入是 x t x_t xt。在自然语言处理中,我们不可能把一个字母作为输入,进行向量、矩阵的乘法,因此,我们需要把它变成一个特征向量。 x t x_t xt可以是字母的特征向量,或者单词的特征向量,或者句子的特征向量。
本文中, x t x_t xt 是一个字母的特征向量。
我们知道,计算机存储字母一般是用ASCII编码,比如a是97,b是98,c是99,d是100……或者我们也可以说,a是1,b是2,c是3……但是,用这样的连续值表示字母,有一个问题,这意味着 a 和 b 的关系比较近,a 和 z 的关系比较远。虽然 a 和 z 在字母表里确实隔得比较远,但是实际上,它们并没有这种内在的关系。我们用一种编码表示它们的时候,它们应该是相互独立的,即对它们进行离散化。
我们通常用到的是 one-hot 编码。它是一个长度为 n n n 的向量,只有 1 1 1 个数字是1,其它的 n − 1 n-1 n−1 个数字都是0。one-hot 编码使得每个字母在它们各自的维度上,与其它字母是独立的。
比如一个单词 “apple”,就分别对a、p、p、l、e 编码,作为输入,LSTM需要循环5次。
- x 0 = [ 1 , 0 , 0 , 0 , 0 … , 0 , 0 ] x_0 = [1,0,0,0,0\ldots,0,0] x0=[1,0,0,0,0…,0,0]: a
- x 1 = [ 0 , 1 , 0 , 0 , 0 … , 0 , 0 ] x_1 = [0,1,0,0,0\ldots,0,0] x1=[0,1,0,0,0…,0,0]: p
- x 2 = [ 0 , 1 , 0 , 0 , 0 … , 0 , 0 ] x_2 = [0,1,0,0,0\ldots,0,0] x2=[0,1,0,0,0…,0,0]: p
- x 3 = [ 0 , 0 , 1 , 0 , 0 … , 0 , 0 ] x_3 = [0,0,1,0,0\ldots,0,0] x3=[0,0,1,0,0…,0,0]: l
- x 4 = [ 0 , 0 , 0 , 1 , 0 … , 0 , 0 ] x_4 = [0,0,0,1,0\ldots,0,0] x4=[0,0,0,1,0…,0,0]: e
上面就是本文提到的字母级(character-level)RNN,而单词级(word-level)RNN就是把整个单词 “apple” 编码成一个向量。通常,对单词的编码用于Seq2Seq模型,即处理的是一个序列 “An apple a day keeps the doctor away”:
- x 0 = [ 1 , 0 , 0 , 0 , 0 , 0 , 0 , 0 , … , 0 , 0 ] x_0 = [1,0,0,0,0,0,0,0,\ldots,0,0] x0=[1,0,0,0,0,0,0,0,…,0,0]: an
- x 1 = [ 0 , 1 , 0 , 0 , 0 , 0 , 0 , 0 , … , 0 , 0 ] x_1 = [0,1,0,0,0,0,0,0,\ldots,0,0] x1=[0,1,0,0,0,0,0,0,…,0,0]: apple
- x 2 = [ 0 , 0 , 1 , 0 , 0 , 0 , 0 , 0 , … , 0 , 0 ] x_2 = [0,0,1,0,0,0,0,0,\ldots,0,0] x2=[0,0,1,0,0,0,0,0,…,0,0]: a
- x 3 = [ 0 , 0 , 0 , 1 , 0 , 0 , 0 , 0 , … , 0 , 0 ] x_3 = [0,0,0,1,0,0,0,0,\ldots,0,0] x3=[0,0,0,1,0,0,0,0,…,0,0]: day
- x 4 = [ 0 , 0 , 0 , 0 , 1 , 0 , 0 , 0 , … , 0 , 0 ] x_4 = [0,0,0,0,1,0,0,0,\ldots,0,0] x4=[0,0,0,0,1,0,0,0,…,0,0]: keeps
- x 5 = [ 0 , 0 , 0 , 0 , 0 , 1 , 0 , 0 , … , 0 , 0 ] x_5 = [0,0,0,0,0,1,0,0,\ldots,0,0] x5=[0,0,0,0,0,1,0,0,…,0,0]: the
- x 6 = [ 0 , 0 , 0 , 0 , 0 , 0 , 1 , 0 … , 0 , 0 ] x_6 = [0,0,0,0,0,0,1,0\ldots,0,0] x6=[0,0,0,0,0,0,1,0…,0,0]: doctor
- x 7 = [ 0 , 0 , 0 , 0 , 0 , 0 , 0 , 1 … , 0 , 0 ] x_7 = [0,0,0,0,0,0,0,1\ldots,0,0] x7=[0,0,0,0,0,0,0,1…,0,0]: away
不过,本文中, x t x_t xt 是一个字母的特征向量,而不是一个单词的特征向量。
3 代码
数据预处理
首先,我们把所有的 /name/[Language].txt
文件读进来。
n_letters
表示所有字母的数量。因为某些语言的字母和常见的英文字母不太一样,所以我们需要把它转化成普普通通的英文字母,用到了 unicodeToAscii()
函数。
from __future__ import unicode_literals,print_function,division
from io import open
import glob
import os
def findFiles(path): return glob.glob(path)
print(findFiles('data/names/*.txt'))
import unicodedata
import string
all_letters = string.ascii_letters + " .,;'"
n_letters = len(all_letters)
def unicodeToAscii(s):
return ''.join(
c for c in unicodedata.normalize('NFD',s)
if unicodedata.category(c)!='Mn'
and c in all_letters
)
print(unicodeToAscii('Ślusàrski'))
Out:
['data/names/Greek.txt', 'data/names/Dutch.txt', 'data/names/Irish.txt', 'data/names/Arabic.txt', 'data/names/Korean.txt', 'data/names/French.txt', 'data/names/Spanish.txt', 'data/names/German.txt', 'data/names/Portuguese.txt', 'data/names/Italian.txt', 'data/names/Vietnamese.txt', 'data/names/Russian.txt', 'data/names/Scottish.txt', 'data/names/Chinese.txt', 'data/names/English.txt', 'data/names/Japanese.txt', 'data/names/Czech.txt', 'data/names/Polish.txt']
Slusarski
文件 [Language].txt
的命名中,Language
就是类别 category
。把每个文件打开,读入每一行,放入一个数组 lines = [names ...]
。建立一个词典 category_lines = {language: lines}
category_lines = {}
all_categories = []
def readLines(filename):
lines = open(filename,encoding='utf-8').read().strip().split('\n')
return [unicodeToAscii(line) for line in lines]
for filename in findFiles('data/names/*.txt'):
category = os.path.splitext(os.path.basename(filename))[0]
all_categories.append(category)
lines = readLines(filename)
category_lines[category] = lines
n_categories = len(all_categories)
print(all_categories)
print(category_lines['Italian'])
Out:
['Greek', 'Dutch', 'Irish', 'Arabic', 'Korean', 'French', 'Spanish', 'German', 'Portuguese', 'Italian', 'Vietnamese', 'Russian', 'Scottish', 'Chinese', 'English', 'Japanese', 'Czech', 'Polish']
['Abandonato', 'Abatangelo', 'Abatantuono', 'Abate', 'Abategiovanni', 'Abatescianni', 'Abba', 'Abbadelli', 'Abbascia', 'Abbatangelo', 'Abbatantuono', 'Abbate', 'Abbatelli', 'Abbaticchio', 'Abbiati', 'Abbracciabene', 'Abbracciabeni', 'Abelli', 'Abello', 'Abrami', 'Abramo', 'Acardi', 'Accardi', 'Accardo', 'Acciai', 'Acciaio', 'Acciaioli', 'Acconci', 'Acconcio', 'Accorsi', 'Accorso', 'Accosi', 'Accursio', 'Acerbi', 'Acone', 'Aconi', 'Acqua', 'Acquafredda', 'Acquarone', 'Acquati', 'Adalardi', 'Adami', 'Adamo', 'Adamoli', 'Addario', 'Adelardi', 'Adessi', 'Adimari', 'Adriatico', 'Affini', 'Africani', 'Africano', 'Agani', 'Aggi', 'Aggio', 'Agli', 'Agnelli', 'Agnellutti', 'Agnusdei', 'Agosti', 'Agostini', 'Agresta', 'Agrioli', 'Aiello', 'Aiolfi', 'Airaldi', 'Airo', 'Aita', 'Ajello', 'Alagona', 'Alamanni', 'Albanesi', 'Albani', 'Albano', 'Alberghi', 'Alberghini', 'Alberici', 'Alberighi', 'Albero', 'Albini', 'Albricci', 'Albrici', 'Alcheri', 'Aldebrandi', 'Alderisi', 'Alduino', 'Alemagna', 'Aleppo', 'Alesci', 'Alescio', 'Alesi', 'Alesini', 'Alesio', 'Alessandri', 'Alessi', 'Alfero', 'Aliberti', 'Alinari', 'Aliprandi', 'Allegri', 'Allegro', 'Alo', 'Aloia', 'Aloisi', 'Altamura', 'Altimari', 'Altoviti', 'Alunni', 'Amadei', 'Amadori', 'Amalberti', 'Amantea', 'Amato', 'Amatore', 'Ambrogi', 'Ambrosi', 'Amello', 'Amerighi', 'Amoretto', 'Angioli', 'Ansaldi', 'Anselmetti', 'Anselmi', 'Antonelli', 'Antonini', 'Antonino', 'Aquila', 'Aquino', 'Arbore', 'Ardiccioni', 'Ardizzone', 'Ardovini', 'Arena', 'Aringheri', 'Arlotti', 'Armani', 'Armati', 'Armonni', 'Arnolfi', 'Arnoni', 'Arrighetti', 'Arrighi', 'Arrigucci', 'Aucciello', 'Azzara', 'Baggi', 'Baggio', 'Baglio', 'Bagni', 'Bagnoli', 'Balboni', 'Baldi', 'Baldini', 'Baldinotti', 'Baldovini', 'Bandini', 'Bandoni', 'Barbieri', 'Barone', 'Barsetti', 'Bartalotti', 'Bartolomei', 'Bartolomeo', 'Barzetti', 'Basile', 'Bassanelli', 'Bassani', 'Bassi', 'Basso', 'Basurto', 'Battaglia', 'Bazzoli', 'Bellandi', 'Bellandini', 'Bellincioni', 'Bellini', 'Bello', 'Bellomi', 'Belloni', 'Belluomi', 'Belmonte', 'Bencivenni', 'Benedetti', 'Benenati', 'Benetton', 'Benini', 'Benivieni', 'Benvenuti', 'Berardi', 'Bergamaschi', 'Berti', 'Bertolini', 'Biancardi', 'Bianchi', 'Bicchieri', 'Biondi', 'Biondo', 'Boerio', 'Bologna', 'Bondesan', 'Bonomo', 'Borghi', 'Borgnino', 'Borgogni', 'Bosco', 'Bove', 'Bover', 'Boveri', 'Brambani', 'Brambilla', 'Breda', 'Brioschi', 'Brivio', 'Brunetti', 'Bruno', 'Buffone', 'Bulgarelli', 'Bulgari', 'Buonarroti', 'Busto', 'Caiazzo', 'Caito', 'Caivano', 'Calabrese', 'Calligaris', 'Campana', 'Campo', 'Cantu', 'Capello', 'Capello', 'Capello', 'Capitani', 'Carbone', 'Carboni', 'Carideo', 'Carlevaro', 'Caro', 'Carracci', 'Carrara', 'Caruso', 'Cassano', 'Castro', 'Catalano', 'Cattaneo', 'Cavalcante', 'Cavallo', 'Cingolani', 'Cino', 'Cipriani', 'Cisternino', 'Coiro', 'Cola', 'Colombera', 'Colombo', 'Columbo', 'Como', 'Como', 'Confortola', 'Conti', 'Corna', 'Corti', 'Corvi', 'Costa', 'Costantini', 'Costanzo', 'Cracchiolo', 'Cremaschi', 'Cremona', 'Cremonesi', 'Crespo', 'Croce', 'Crocetti', 'Cucinotta', 'Cuocco', 'Cuoco', "D'ambrosio", 'Damiani', "D'amore", "D'angelo", "D'antonio", 'De angelis', 'De campo', 'De felice', 'De filippis', 'De fiore', 'De laurentis', 'De luca', 'De palma', 'De rege', 'De santis', 'De vitis', 'Di antonio', 'Di caprio', 'Di mercurio', 'Dinapoli', 'Dioli', 'Di pasqua', 'Di pietro', 'Di stefano', 'Donati', "D'onofrio", 'Drago', 'Durante', 'Elena', 'Episcopo', 'Ermacora', 'Esposito', 'Evangelista', 'Fabbri', 'Fabbro', 'Falco', 'Faraldo', 'Farina', 'Farro', 'Fattore', 'Fausti', 'Fava', 'Favero', 'Fermi', 'Ferrara', 'Ferrari', 'Ferraro', 'Ferrero', 'Ferro', 'Fierro', 'Filippi', 'Fini', 'Fiore', 'Fiscella', 'Fiscella', 'Fonda', 'Fontana', 'Fortunato', 'Franco', 'Franzese', 'Furlan', 'Gabrielli', 'Gagliardi', 'Gallo', 'Ganza', 'Garfagnini', 'Garofalo', 'Gaspari', 'Gatti', 'Genovese', 'Gentile', 'Germano', 'Giannino', 'Gimondi', 'Giordano', 'Gismondi', 'Giugovaz', 'Giunta', 'Goretti', 'Gori', 'Greco', 'Grillo', 'Grimaldi', 'Gronchi', 'Guarneri', 'Guerra', 'Guerriero', 'Guidi', 'Guttuso', 'Idoni', 'Innocenti', 'Labriola', 'Laconi', 'Lagana', 'Lagomarsino', 'Lagorio', 'Laguardia', 'Lama', 'Lamberti', 'Lamon', 'Landi', 'Lando', 'Landolfi', 'Laterza', 'Laurito', 'Lazzari', 'Lecce', 'Leccese', 'Leggieri', 'Lemmi', 'Leone', 'Leoni', 'Lippi', 'Locatelli', 'Lombardi', 'Longo', 'Lupo', 'Luzzatto', 'Maestri', 'Magro', 'Mancini', 'Manco', 'Mancuso', 'Manfredi', 'Manfredonia', 'Mantovani', 'Marchegiano', 'Marchesi', 'Marchetti', 'Marchioni', 'Marconi', 'Mari', 'Maria', 'Mariani', 'Marino', 'Marmo', 'Martelli', 'Martinelli', 'Masi', 'Masin', 'Mazza', 'Merlo', 'Messana', 'Micheli', 'Milani', 'Milano', 'Modugno', 'Mondadori', 'Mondo', 'Montagna', 'Montana', 'Montanari', 'Monte', 'Monti', 'Morandi', 'Morello', 'Moretti', 'Morra', 'Moschella', 'Mosconi', 'Motta', 'Muggia', 'Muraro', 'Murgia', 'Murtas', 'Nacar', 'Naggi', 'Naggia', 'Naldi', 'Nana', 'Nani', 'Nanni', 'Nannini', 'Napoleoni', 'Napoletani', 'Napoliello', 'Nardi', 'Nardo', 'Nardovino', 'Nasato', 'Nascimbene', 'Nascimbeni', 'Natale', 'Nave', 'Nazario', 'Necchi', 'Negri', 'Negrini', 'Nelli', 'Nenci', 'Nepi', 'Neri', 'Neroni', 'Nervetti', 'Nervi', 'Nespola', 'Nicastro', 'Nicchi', 'Nicodemo', 'Nicolai', 'Nicolosi', 'Nicosia', 'Nicotera', 'Nieddu', 'Nieri', 'Nigro', 'Nisi', 'Nizzola', 'Noschese', 'Notaro', 'Notoriano', 'Oberti', 'Oberto', 'Ongaro', 'Orlando', 'Orsini', 'Pace', 'Padovan', 'Padovano', 'Pagani', 'Pagano', 'Palladino', 'Palmisano', 'Palumbo', 'Panzavecchia', 'Parisi', 'Parma', 'Parodi', 'Parri', 'Parrino', 'Passerini', 'Pastore', 'Paternoster', 'Pavesi', 'Pavone', 'Pavoni', 'Pecora', 'Pedrotti', 'Pellegrino', 'Perugia', 'Pesaresi', 'Pesaro', 'Pesce', 'Petri', 'Pherigo', 'Piazza', 'Piccirillo', 'Piccoli', 'Pierno', 'Pietri', 'Pini', 'Piovene', 'Piraino', 'Pisani', 'Pittaluga', 'Poggi', 'Poggio', 'Poletti', 'Pontecorvo', 'Portelli', 'Porto', 'Portoghese', 'Potenza', 'Pozzi', 'Profeta', 'Prosdocimi', 'Provenza', 'Provenzano', 'Pugliese', 'Quaranta', 'Quattrocchi', 'Ragno', 'Raimondi', 'Rais', 'Rana', 'Raneri', 'Rao', 'Rapallino', 'Ratti', 'Ravenna', 'Re', 'Ricchetti', 'Ricci', 'Riggi', 'Righi', 'Rinaldi', 'Riva', 'Rizzo', 'Robustelli', 'Rocca', 'Rocchi', 'Rocco', 'Roma', 'Roma', 'Romagna', 'Romagnoli', 'Romano', 'Romano', 'Romero', 'Roncalli', 'Ronchi', 'Rosa', 'Rossi', 'Rossini', 'Rotolo', 'Rovigatti', 'Ruggeri', 'Russo', 'Rustici', 'Ruzzier', 'Sabbadin', 'Sacco', 'Sala', 'Salomon', 'Salucci', 'Salvaggi', 'Salvai', 'Salvail', 'Salvatici', 'Salvay', 'Sanna', 'Sansone', 'Santini', 'Santoro', 'Sapienti', 'Sarno', 'Sarti', 'Sartini', 'Sarto', 'Savona', 'Scarpa', 'Scarsi', 'Scavo', 'Sciacca', 'Sciacchitano', 'Sciarra', 'Scordato', 'Scotti', 'Scutese', 'Sebastiani', 'Sebastino', 'Segreti', 'Selmone', 'Selvaggio', 'Serafin', 'Serafini', 'Serpico', 'Sessa', 'Sgro', 'Siena', 'Silvestri', 'Sinagra', 'Sinagra', 'Soldati', 'Somma', 'Sordi', 'Soriano', 'Sorrentino', 'Spada', 'Spano', 'Sparacello', 'Speziale', 'Spini', 'Stabile', 'Stablum', 'Stilo', 'Sultana', 'Tafani', 'Tamaro', 'Tamboia', 'Tanzi', 'Tarantino', 'Taverna', 'Tedesco', 'Terranova', 'Terzi', 'Tessaro', 'Testa', 'Tiraboschi', 'Tivoli', 'Todaro', 'Toloni', 'Tornincasa', 'Toselli', 'Tosetti', 'Tosi', 'Tosto', 'Trapani', 'Traversa', 'Traversi', 'Traversini', 'Traverso', 'Trucco', 'Trudu', 'Tumicelli', 'Turati', 'Turchi', 'Uberti', 'Uccello', 'Uggeri', 'Ughi', 'Ungaretti', 'Ungaro', 'Vacca', 'Vaccaro', 'Valenti', 'Valentini', 'Valerio', 'Varano', 'Ventimiglia', 'Ventura', 'Verona', 'Veronesi', 'Vescovi', 'Vespa', 'Vestri', 'Vicario', 'Vico', 'Vigo', 'Villa', 'Vinci', 'Vinci', 'Viola', 'Vitali', 'Viteri', 'Voltolini', 'Zambrano', 'Zanetti', 'Zangari', 'Zappa', 'Zeni', 'Zini', 'Zino', 'Zunino']
接下来,就是要对字母进行one-hot编码,转成 tensor。
假设字母表中的字母数量为 n_letters
, 一个字母的向量就是 < 1 × n_letters >
维,只有 1
个维度是1,其他 n_letters-1
维是0。
一个长度为 line_length
的单词,它的向量维度是 < line_length × n_letters >
维。
在机器学习中,通常我们会按照 batch 来训练,所以这里设定一个单词的 batch 是1,单词的向量维度变成了 < line_length × 1 × n_letters >
import torch
device = torch.device("cuda:0" if torch.cuda.is_available() else "cpu")
# 返回字母 letter 的索引 index
def letterToIndex(letter):
return all_letters.find(letter)
# 把一个字母编码成tensor
def letterToTensor(letter):
tensor = torch.zeros(1,n_letters)
# 把字母 letter 的索引设定为1,其它都是0
tensor[0][letterToIndex(letter)] = 1
return tensor.to(device)
# 把一个单词编码成tensor
def lineToTensor(line):
tensor = torch.zeros(len(line),1,n_letters)
# 遍历单词中的所有字母,对每个字母 letter 它的索引设定为1,其它都是0
for li, letter in enumerate(line):
tensor[li][0][letterToIndex(letter)] = 1
return tensor.to(device)
print(letterToTensor('J'))
print(lineToTensor('Jones').size())
Out:
tensor([[0., 0., 0., 0., 0., 0., 0., 0., 0., 0., 0., 0., 0., 0., 0., 0., 0., 0.,
0., 0., 0., 0., 0., 0., 0., 0., 0., 0., 0., 0., 0., 0., 0., 0., 0., 1.,
0., 0., 0., 0., 0., 0., 0., 0., 0., 0., 0., 0., 0., 0., 0., 0., 0., 0.,
0., 0., 0.]], device='cuda:0')
torch.Size([5, 1, 57])
模型搭建
然后就是我们的模型部分,一个最普通的RNN。
它是一个两层的结构,i2h
是输入
x
t
x_t
xt 到隐藏层
h
t
h_t
ht ,i2o
是输入
x
t
x_t
xt 到输出
o
t
o_t
ot,softmax
是把输出
o
t
o_t
ot 变成 预测值
y
t
y_t
yt。 实际上,它在这里是一个 LogSoftmax
函数,对应的损失函数是NLLLoss()
,而如果它是一般的 Softmax
函数,对应的损失函数就是交叉熵损失 CrossEntropy() = Log (NLLLoss())
。
这里设定隐藏层的向量维度为128维,为了简单,可以说是隐藏层的大小是128维。
模型真正的运行步骤在 forward()
函数中,它的输入 input
即为
x
t
x_t
xt,隐藏层hidden
即为
h
t
h_t
ht:
combined = torch.cat((input,hidden),1)
:把 x t x_t xt 和上一步的 h t − 1 h_{t-1} ht−1 拼接在一起,变成 [ x t , h t − 1 ] [ x_t, h_{t-1}] [xt,ht−1]hidden = self.i2h(combined)
:我们之前说,把输入 [ x t , h t − 1 ] [ x_t, h_{t-1}] [xt,ht−1] 乘上权重 W h W_h Wh 变成新的隐藏层 h t = W h [ x t , h t − 1 ] h_t = W_h[ x_t, h_{t-1}] ht=Wh[xt,ht−1],这里实际上就是通过一个线性的全连接层i2h
,它的输入大小是input_size + hidden_size
, 输出大小是hidden_size
output = self.i2o(combined)
:把输入 [ x t , h t − 1 ] [ x_t, h_{t-1}] [xt,ht−1] 乘上权重 W o W_o Wo ,得到输出 o t = W o [ x t , h t − 1 ] o_t = W_o[ x_t, h_{t-1}] ot=Wo[xt,ht−1],同样是通过一个线性的全连接层i2o
,它的输入大小是input_size + hidden_size
, 输出大小是output_size
output = self.softmax(output)
:通过一个softmax函数,把输出 o t o_t ot 变成预测值 y t = log s o f t m a x ( o t ) y_t = \log softmax(o_t) yt=logsoftmax(ot)
import torch.nn as nn
class RNN(nn.Module):
# 初始化定义每一层的输入大小,输出大小
def __init__(self, input_size, hidden_size, output_size):
super(RNN,self).__init__()
self.hidden_size = hidden_size
self.i2h = nn.Linear(input_size + hidden_size, hidden_size)
self.i2o = nn.Linear(input_size + hidden_size, output_size)
self.softmax = nn.LogSoftmax(dim=1)
# 前向传播过程
def forward(self, input, hidden):
combined = torch.cat((input,hidden),1)
hidden = self.i2h(combined)
output = self.i2o(combined)
output = self.softmax(output)
return output, hidden
# 初始化隐藏层状态 h0
def initHidden(self):
return torch.zeros(1,self.hidden_size).to(device)
n_hidden = 128
rnn = RNN(n_letters, n_hidden, n_categories)
rnn = rnn.to(device)
输入一个字母 A 测试一下:
input = letterToTensor('A')
hidden =torch.zeros(1, n_hidden).to(device)
output, next_hidden = rnn(input, hidden)
print(output)
Out:
tensor([[-2.8972, -2.8574, -2.9266, -2.9602, -2.9735, -3.0286, -2.9190, -2.7779,
-2.8833, -2.8667, -2.9404, -2.8011, -2.8216, -2.8422, -2.8119, -2.8525,
-2.9453, -2.9611]], device='cuda:0', grad_fn=<LogSoftmaxBackward>)
再输入名字 Albert 的第一个字母 A 测试一下:
input = lineToTensor('Albert')
hidden = torch.zeros(1, n_hidden).to(device)
output, next_hidden = rnn(input[0], hidden)
print(output)
Out:
tensor([[-2.8972, -2.8574, -2.9266, -2.9602, -2.9735, -3.0286, -2.9190, -2.7779,
-2.8833, -2.8667, -2.9404, -2.8011, -2.8216, -2.8422, -2.8119, -2.8525,
-2.9453, -2.9611]], device='cuda:0', grad_fn=<LogSoftmaxBackward>)
定义一个函数 categoryFromOutput()
可以把
y
t
y_t
yt 变成对应的类别。用 Tensor.topk
选出18个概率中,概率最大的那个的下标 category_i
,就是
y
t
y_t
yt 的类别。
def categoryFromOutput(output):
top_n, top_i = output.topk(1)
category_i = top_i[0].item()
return all_categories[category_i], category_i
print(categoryFromOutput(output))
Out:
('German', 7)
训练
因为目前模型还没有被训练,所以上面的概率可以认为是随机产生的。接下来,我们要训练模型。这个教程里不是把所有的数据都拿来训练,而是随机采样一部分数据来训练。
用 randomChoice()
从所有数据中随机采样,先采样得到类别category
,再从类别category
中随机采样,得到姓名line
。
randomTrainingExample()
将采样得到的 category-line
对变成tensor。
import random
def randomChoice(l):
return l[random.randint(0,len(l)-1)]
def randomTrainingExample():
category = randomChoice(all_categories)
line = randomChoice(category_lines[category])
category_tensor = torch.tensor([all_categories.index(category)], dtype=torch.long).to(device)
line_tensor = lineToTensor(line)
return category, line, category_tensor, line_tensor
for i in range(10):
category, line, category_tensor, line_tensor = randomTrainingExample()
print('category = ', category, '/ line = ', line)
看看随机采样10个样本的情况:
Out:
category = Scottish / line = Mckenzie
category = Irish / line = Cormac
category = German / line = Farber
category = French / line = David
category = Russian / line = Yanaslov
category = Korean / line = Gwang
category = Chinese / line = Hiu
category = Russian / line = Turchak
category = Portuguese / line = Madeira
category = Spanish / line = Castillo
定义损失函数为 NLLLoss()
, 学习率0.005。
在训练的每个循环会执行以下过程:
- 创建输入tensor和目标tensor
- 初始化隐藏层状态 h 0 h_0 h0
- 输入每个字母 x t x_t xt 并且
- 保存下一个字母需要的隐藏层状态 h t h_t ht
- 将模型预测的输出 y t y_t yt 和目标 y t ∗ y^*_t yt∗ 之间进行对比
- 梯度反向传播
- 返回输出和损失函数
criterion = nn.NLLLoss()
learning_rate = 0.005
def train(category_tensor, line_tensor):
hidden = rnn.initHidden()
rnn.zero_grad()
# RNN的循环
for i in range(line_tensor.size()[0]):
output, hidden = rnn(line_tensor[i],hidden)
loss = criterion(output, category_tensor)
loss.backward()
# 更新参数
for p in rnn.parameters():
p.data.add_(p.grad.data, alpha=-learning_rate)
return output, loss.item()
下面正式开始训练模型。
timeSince()
可以计算出训练时间。总共训练n_iters
次,每次用1个样本作为训练。每 print_every
次打印当前的训练损失,每 plot_every
次把损失保存到 all_losses
数组中,便于之后画图。
import time
import math
n_iters = 100000
print_every = 5000
plot_every = 1000
current_loss = 0
all_losses = []
def timeSince(since):
now = time.time()
s = now-since
return '%dm %ds'%(s//60,s%60)
start = time.time()
for iter in range(1, n_iters + 1):
category, line, category_tensor, line_tensor = randomTrainingExample()
output, loss = train(category_tensor, line_tensor)
current_loss += loss
if iter % print_every == 0:
guess, guess_i = categoryFromOutput(output)
correct = '√' if guess==category else '×(%s)'%category
print('%d %d%% (%s) %.4f %s / %s %s' %
(iter, iter/n_iters*100,timeSince(start),loss,line,guess,correct))
if iter % plot_every == 0:
all_losses.append(current_loss/plot_every)
current_loss = 0
Out:
5000 5% (0m 37s) 2.6348 Awdyukoff / English ×(Russian)
10000 10% (1m 14s) 1.2936 Dong / Vietnamese ×(Chinese)
15000 15% (1m 50s) 2.5938 Peij / Korean ×(Dutch)
20000 20% (2m 27s) 0.9246 Kwang / Korean √
25000 25% (3m 3s) 0.7240 Echeverria / Spanish √
30000 30% (3m 39s) 2.3746 Benn / Chinese ×(German)
35000 35% (4m 16s) 3.1926 Wright / English ×(Scottish)
40000 40% (4m 53s) 1.5385 Vodden / Dutch ×(English)
45000 45% (5m 30s) 2.7799 Pander / German ×(Dutch)
50000 50% (6m 8s) 1.6615 Beckert / Dutch ×(German)
55000 55% (6m 46s) 0.4871 Mcdonald / Scottish √
60000 60% (7m 24s) 2.0091 Glenn / Scottish ×(English)
65000 65% (8m 2s) 1.5405 Flater / German √
70000 70% (8m 38s) 3.4448 Navara / Spanish ×(Czech)
75000 75% (9m 13s) 2.4132 Poulin / Russian ×(French)
80000 80% (9m 48s) 1.0501 Cao / Chinese ×(Vietnamese)
85000 85% (10m 22s) 1.1398 Sung / Chinese ×(Korean)
90000 90% (10m 57s) 2.2497 Frankland / Polish ×(English)
95000 95% (11m 32s) 1.3177 Williamson / Scottish √
100000 100% (12m 7s) 0.5656 Said / Arabic √
画图
画出损失函数随着训练的变化情况:
import matplotlib.pyplot as plt
import matplotlib.ticker as ticker
plt.figure()
plt.plot(all_losses)
为了看看模型在各个分类上的预测情况,我们要画出18国语言的混淆矩阵。每一行是真实的语言,每一列是预测的语言。用函数 evaluate()
来计算混淆矩阵。evaluate()
和 train()
非常相似,但是不需要梯度反向传播。
confusion = torch.zeros(n_categories, n_categories)
n_confusion = 10000
def evaluate(line_tensor):
hidden = rnn.initHidden()
for i in range(line_tensor.size()[0]):
output, hidden = rnn(line_tensor[i],hidden)
return output
for i in range(n_confusion):
category, line, category_tensor,line_tensor = randomTrainingExample()
output = evaluate(line_tensor)
guess, guess_i = categoryFromOutput(output)
category_i = all_categories.index(category)
confusion[category_i][guess_i] += 1
for i in range(n_categories):
confusion[i] = confusion[i] / confusion[i].sum()
fig = plt.figure()
ax = fig.add_subplot(111)
cax = ax.matshow(confusion.numpy())
fig.colorbar(cax)
ax.set_xticklabels(['']+all_categories,rotation=90)
ax.set_yticklabels(['']+all_categories)
ax.xaxis.set_major_locator(ticker.MultipleLocator(1))
ax.yaxis.set_major_locator(ticker.MultipleLocator(1))
plt.show()
两种语言连线处的正方形颜色越偏向暖色,表示两种语言的姓名越相似。从图中可以看到,有一些比较容易混淆语言,比如Chinese和Korean,还有Chinese和Vietnamese,English和Scottish。
预测
对于每个名字 input_line
,每次预测 n_predictions=3
个最有可能的类别,并且输出它们对应的概率:
def predict(input_line, n_predictions=3):
print('\n> %s'%input_line)
with torch.no_grad():
output = evaluate(lineToTensor(input_line))
topv, topi = output.topk(n_predictions,1,True)
predictions = []
for i in range(n_predictions):
value = topv[0][i].item()
category_index = topi[0][i].item()
print('(%.2f) %s' % (value, all_categories[category_index]))
predictions.append([value, all_categories[category_index]])
predict('Dovesky')
predict('Jackson')
predict('Satoshi')
Out:
> Dovesky
(-0.41) Russian
(-1.33) Czech
(-3.52) Polish
> Jackson
(-0.58) Scottish
(-1.61) English
(-2.41) Russian
> Satoshi
(-1.29) Italian
(-1.40) Japanese
(-1.62) Arabic
到这里,我们就结束了本次的教程。你还可以在该教程基础上做更多的尝试:
- 尝试不同的训练集,来进行 line -> category 的映射,比如:
任何单词 -> 语言
姓名 -> 性别
角色名 -> 作者 - 尝试改变模型,获得更好的结果,比如:
增加更多的全连接层
试图使用 nn.LSTM 或者 nn.GRU
把多个RNN合并起来,作为一个更高层次的网络