目录
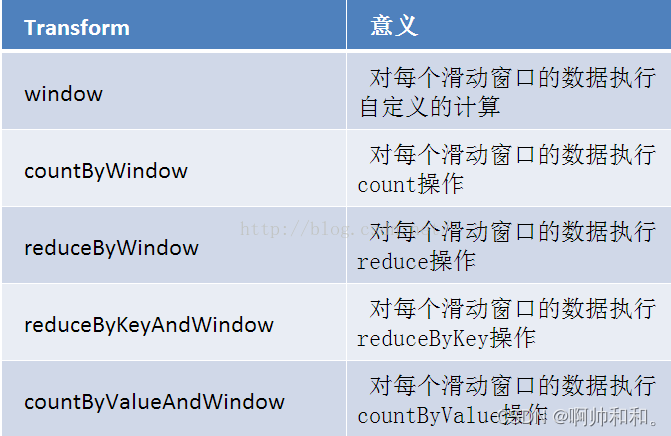
提前封装好的重复代码
按照我的习惯,先把重复的代码做一个简单的封装,后面直接继承就可,这里的窗口长度为3,滑动频率为1
package com.shujia.test
import org.apache.spark.SparkContext
import org.apache.spark.sql.SparkSession
import org.apache.spark.streaming.{Durations, StreamingContext}
import org.apache.spark.streaming.dstream.{DStream, ReceiverInputDStream}
abstract class WindowTestTool {
var spark: SparkSession = _
var sc: SparkContext = _
var ssc:StreamingContext = _
def main(args: Array[String]): Unit = {
spark = SparkSession
.builder()
.master("local[*]")
.appName("WindowTest")
.getOrCreate()
sc = spark.sparkContext
val ssc= new StreamingContext(sc,Durations.seconds(1))
ssc.checkpoint("/data/checkpoint")
val lines: ReceiverInputDStream[String] = ssc.socketTextStream("master",8888)
val words: DStream[String] = lines.flatMap(_.split(" "))
this.run(words)
ssc.start()
ssc.awaitTermination()
ssc.stop()
}
def run(words:DStream[String])
}
这里提前开启一个端口,但要是端口没有正常关闭被占用了,可以先清除
这样就可以了
将日志信息调整为ERROR
在没经过修改之前,日志的等级为INFO,也就是平时所有的信息都会出现,这里修改为ERROR,只让idea在程序报错的时候才出现信息
window
// 1.对每个滑动窗口的数据执行自定义的计算
// window(windowLength, slideInterval)
// 该操作由一个DStream对象调用,传入一个窗口长度参数,
// 一个窗口移动速率参数,然后将当前时刻当前长度窗口中的元素取出形成一个新的DStream
object WindowTest extends WindowTestTool {
override def run(words: DStream[String]): Unit = {
// 1.对每个滑动窗口的数据执行自定义的计算
// window(windowLength, slideInterval)
// 该操作由一个DStream对象调用,传入一个窗口长度参数,
// 一个窗口移动速率参数,然后将当前时刻当前长度窗口中的元素取出形成一个新的DStream
val windowWords: DStream[String] = words.window(Durations.seconds(3),Durations.seconds(1))
windowWords.print()
}
}
countByWindow
//2.对每个滑动窗口的数据执行count操作
//countByWindow(windowLength,slideInterval)
//返回指定长度窗口中的元素个数
object WindowTest extends WindowTestTool {
override def run(words: DStream[String]): Unit = {
//2.对每个滑动窗口的数据执行count操作
//countByWindow(windowLength,slideInterval)
//返回指定长度窗口中的元素个数
val windowWords: DStream[Long] = words.countByWindow(Durations.seconds(3),Durations.seconds(1))
windowWords.print()
}
}
reduceByWindow
//3.对每个滑动窗口的数据执行reduce操作
//这里不再是对整个调用DStream进行reduce操作,
//而是在调用DStream上首先取窗口函数的元素形成新的DStream,
// 然后在窗口元素形成的DStream上进行reduce
object WindowTest extends WindowTestTool {
override def run(words: DStream[String]): Unit = {
//3.对每个滑动窗口的数据执行reduce操作
//这里不再是对整个调用DStream进行reduce操作,
//而是在调用DStream上首先取窗口函数的元素形成新的DStream,
// 然后在窗口元素形成的DStream上进行reduce
val windowWords: DStream[String] = words.reduceByWindow(_+"-"+_,Durations.seconds(3),Durations.seconds(1))
}
}
reduceByKeyAndWindow
不保留数值
//4.对每个滑动窗口的数据执行reduceByKey操作
//reduceByKeyAndWindow(func,windowLength, slideInterval, [numTasks])
//调用该操作的DStream中的元素格式为(k, v),整个操作类似于前面的reduceByKey,
//只不过对应的数据源不同,reduceByKeyAndWindow的数据源是基于该DStream的窗口长度中的所有数据。该操作也有一个可选的并发数参数
object WindowTest extends WindowTestTool {
override def run(words: DStream[String]): Unit = {
//4.对每个滑动窗口的数据执行reduceByKey操作
//reduceByKeyAndWindow(func,windowLength, slideInterval, [numTasks])
//调用该操作的DStream中的元素格式为(k, v),整个操作类似于前面的reduceByKey,
//只不过对应的数据源不同,reduceByKeyAndWindow的数据源是基于该DStream的窗口长度中的所有数据。该操作也有一个可选的并发数参数
val pairs: DStream[(String, Int)] = words.map(
word => (word, 1)
)
val windowWords: DStream[(String, Int)] = pairs.reduceByKeyAndWindow(
(a: Int, b: Int) => (a + b),
Durations.seconds(3),
Durations.seconds(1)
)
windowWords.print()
}
}
保留数值
object WindowTest extends WindowTestTool {
override def run(words: DStream[String]): Unit = {
//两个参数:会对原来的数据进行一个保存
val pairs: DStream[(String, Int)] = words.map(
word => (word, 1)
)
val windowWords: DStream[(String, Int)] = pairs.reduceByKeyAndWindow(
(a: Int, b: Int) => (a + b),
(a: Int, b: Int) => (a - b),
Durations.seconds(3),
Durations.seconds(1)
)
windowWords.print()
}
}
countByValueAndWindow
//5.对每个滑动窗口的数据执行countByValue操作
//countByValueAndWindow(windowLength,slideInterval, [numTasks])
//类似于前面的countByValue操作,调用该操作的DStream数据格式为(K, v),
// 返回的DStream格式为(K, Long)。统计当前时间窗口中元素值相同的元素的个数
object WindowTest extends WindowTestTool {
override def run(words: DStream[String]): Unit = {
val windowWords: DStream[(String, Long)] = words.countByValueAndWindow(Durations.seconds(3),Durations.seconds(1))
windowWords.print()
}
}
感谢阅读,我是啊帅和和,一位大数据专业大四学生,祝你快乐。