笔者环境win1064位
1.anaconda python 3.5 64为安装包
2.安装tensflow1.0
### 第一部分(图片物体识别)
- 1.下载tensflow的源码包,并解压
- 2.下载 [Protoc](https://github.com/google/protobuf/releases/download/v3.4.0/protoc-3.4.0-win32.zip) protoc-3.4.0-win32.zip
解压protoc-3.4.0-win32.zip,并将bin文件夹内的protoc.exe拷贝到c:\windows\system32目录下(也可将其添加到环境变量中)
- 3.cd "F:\test\models-master\object_detection"在终端执行
```
protoc object_detection/protos/*.proto --python_out=.
```
- 4.在终端执行ipython notebook
- 5.在浏览器打开object_detection_tutorial.ipynb运行
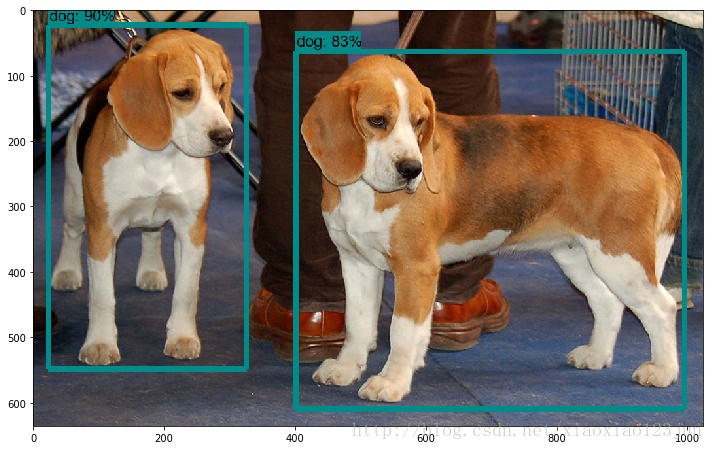
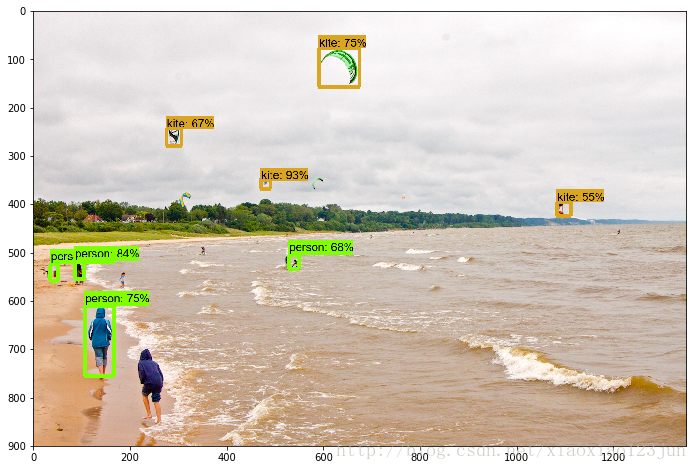
说明:
1.PATH_TO_TEST_IMAGES_DIR = 'test_images' 未测试文件夹
2.MODEL_NAME = 'ssd_mobilenet_v1_coco_11_06_2017'
mode有四种:
```
MODEL_NAME = 'ssd_inception_v2_coco_11_06_2017'
MODEL_NAME = 'rfcn_resnet101_coco_11_06_2017'
MODEL_NAME = 'faster_rcnn_resnet101_coco_11_06_2017'
MODEL_NAME = 'faster_rcnn_inception_resnet_v2_atrous_coco_11_06_2017'
```
其中faster_rcnn_inception_resnet_v2_atrous_coco_11_06_2017'的准确路最高,但时间也最长
### 第二部分(视频中物体识别)
[参考自github上面的完整代码](https://github.com/priya-dwivedi/Deep-Learning/blob/master/Object_Detection_Tensorflow_API.ipynb)下面我们在google的object_detection_tutorial.ipynb基础上改造。
1.安装环境准备
- a: 安装a.opencv的cv2包 :
conda install --channel https://conda.anaconda.org/menpo opencv3
可以在python 命令行中import cv2 测试一下
- b: 安装imageio: conda install -c conda-forge imageio
- c: 安装moviepy:pip install moviepy
2. 导入opencv包
```
import cv2
```
3.下载视频剪辑程序,此处消耗时间有点长
```
# Import everything needed to edit/save/watch video clips
import imageio
imageio.plugins.ffmpeg.download()
```
下载过程如下:
```
Imageio: 'ffmpeg-win32-v3.2.4.exe' was not found on your computer; downloading it now.
Try 1. Download from https://github.com/imageio/imageio-binaries/raw/master/ffmpeg/ffmpeg-win32-v3.2.4.exe (34.1 MB)
Downloading: 8192/35749888 bytes (0.0%)16384/35749888 bytes (0.0%)32768/35749888 bytes (0.1%)65536/35749888 bytes (0.2%)98304/35749888 bytes (0.3%)131072/35749888 bytes (0.4%)147456/35749888 bytes (0.4%)163840/35749888 bytes (0.5%)180224/35749888 bytes (0.5%)19660**********************
(99.7%)35651584/35749888 bytes (99.7%)35667968/35749888 bytes (99.8%)35684352/35749888 bytes (99.8%)35700736/35749888 bytes (99.9%)35717120/35749888 bytes (99.9%)35733504/35749888 bytes (100.0%)35749888/35749888 bytes (100.0%)35749888/35749888 bytes (100.0%)
Done
File saved as C:\Users\Administrator\AppData\Local\imageio\ffmpeg\ffmpeg-win32-v3.2.4.exe.
```
4.
```
from moviepy.editor import VideoFileClip
from IPython.display import HTML
```
5.
```
def detect_objects(image_np, sess, detection_graph):
# Expand dimensions since the model expects images to have shape: [1, None, None, 3]
image_np_expanded = np.expand_dims(image_np, axis=0)
image_tensor = detection_graph.get_tensor_by_name('image_tensor:0')
# Each box represents a part of the image where a particular object was detected.
boxes = detection_graph.get_tensor_by_name('detection_boxes:0')
# Each score represent how level of confidence for each of the objects.
# Score is shown on the result image, together with the class label.
scores = detection_graph.get_tensor_by_name('detection_scores:0')
classes = detection_graph.get_tensor_by_name('detection_classes:0')
num_detections = detection_graph.get_tensor_by_name('num_detections:0')
# Actual detection.
(boxes, scores, classes, num_detections) = sess.run(
[boxes, scores, classes, num_detections],
feed_dict={image_tensor: image_np_expanded})
# Visualization of the results of a detection.
vis_util.visualize_boxes_and_labels_on_image_array(
image_np,
np.squeeze(boxes),
np.squeeze(classes).astype(np.int32),
np.squeeze(scores),
category_index,
use_normalized_coordinates=True,
line_thickness=8)
return image_np
def process_image(image):
# NOTE: The output you return should be a color image (3 channel) for processing video below
# you should return the final output (image with lines are drawn on lanes)
with detection_graph.as_default():
with tf.Session(graph=detection_graph) as sess:
image_process = detect_objects(image, sess, detection_graph)
return image_process
```
6.开启视频标记,将视频video1.mp4放入到“"F:\test\models-master\object_detection” subclip(25,30)代表识别视频中25-30s这一时间段。
```
white_output = 'video1_out.mp4'
clip1 = VideoFileClip("video1.mp4").subclip(25,30)
white_clip = clip1.fl_image(process_image) #NOTE: this function expects color images!!s
%time white_clip.write_videofile(white_output, audio=False)
```
7.在浏览器中展示
```
HTML("""
<video width="960" height="540" controls>
<source src="{0}">
</video>
""".format(white_output))
```
8.保存为gif格式
```
from moviepy.editor import *
clip1 = VideoFileClip("video1_out.mp4")
clip1.write_gif("final.gif")
```
本文参考:
http://m.blog.csdn.net/xiaoxiao123jun/article/details/76605928