前言
最近在重刷李航老师的《统计机器学习方法》尝试将其与NLP结合,通过具体的NLP应用场景,强化对书中公式的理解,最终形成「统计机器学习方法 for NLP」的系列。这篇将介绍隐含狄利克雷分布,即LDA,并基于LDA完成对论文主题提取的任务。
隐含狄利克雷分布是什么?
隐含狄利克雷分布(Latent Dirichlet Allocation, LDA) 由戴维·布雷(David Blei)、吴恩达(对,就是那个吴恩达)和迈克尔·乔丹(Michael Jordan)于2003年提出,用来推测文档的主题分布,是一个无监督的生成模型。
首先我们认为每个文档是不同主题的集合,同时每个主题是不同单词的集合。
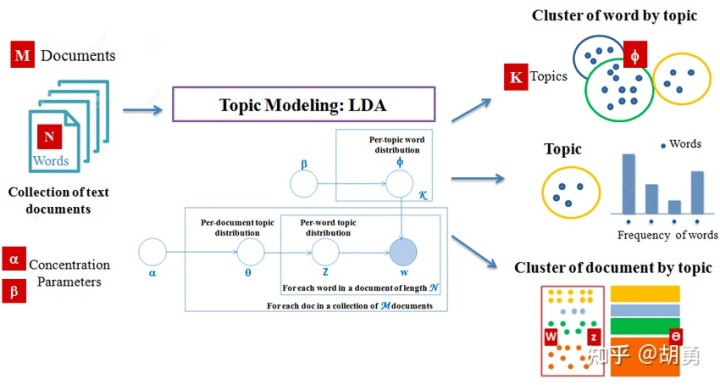
假定现在整个语料
- 从狄利克雷分布(Dirichlet distribution)
中,生成文档
的主题分布
- 从主题分布
中生成文档
中第
个单词的主题
- 从狄利克雷分布(Dirichlet distribution)
中,生成主题
的单词分布
- 从单词分布
中生成最终的单词
所以对于LDA模型,输入
对于LDA算法的求解过程为Gibbs采样法,本文先略过,具体可以参考:文本主题模型之LDA(二) LDA求解之Gibbs采样算法 。
基于LDA的主题模型
下面我们将基于LDA实现一个对发表在NeurIPS上的2000篇论文,做主题模型的分析。
首先进行数据的加载和数据清洗,只保留论文的正问部分,并且移除文本中的标点符号,停用词,并统一转换成小写,转换完成的数据如下所示:
# data_words
[
['actor', 'critic', 'algorithms', 'risk', 'sensitive', 'mdps', 'prashanth', 'la', 'inria', 'lille', ...],
['mixed', 'vine', 'copulas', 'joint', 'models', 'spike', 'counts', 'local', 'field', 'potentials', ...],
['estimating', 'bayes', 'risk', 'sample', 'data', 'robert', 'snapp', 'tong', 'xu', 'computer', ...],
...
]
接着我们引入一个词表,并通过基础的词袋模型将文章进行表示
import gensim.corpora as corpora
# Create Dictionary
id2word = corpora.Dictionary(data_words)
# Create Corpus
texts = data_words
# Term Document Frequency
corpus = [id2word.doc2bow(text) for text in texts]
# take a look
print(corpus[0][:30])
"""
[(token_id, token_count),]
[(0, 1), (1, 1), (2, 8), (3, 5), (4, 1), (5, 12), (6, 4), (7, 31), (8, 1), (9, 1), (10, 1), (11, 1), (12, 1), (13, 1), (14, 2), (15, 1), (16, 4), (17, 2), (18, 1), (19, 1), (20, 2), (21, 3), (22, 2), (23, 17), (24, 54), (25, 1), (26, 1), (27, 2), (28, 3), (29, 1)]
"""
下面我们就可以进行LDA模型的训练了,设定有10个类别,这里直接使用gensim.models.LdaMulticore
import gensim
# number of topics
num_topics = 10
# Build LDA model
lda_model = gensim.models.LdaMulticore(corpus=corpus,
id2word=id2word,
num_topics=num_topics)
将10个类别打印出来看一下
pprint(lda_model.print_topics())
[(0,
'0.006*"data" + 0.006*"model" + 0.005*"learning" + 0.005*"using" + '
'0.004*"set" + 0.004*"algorithm" + 0.004*"one" + 0.004*"figure" + '
'0.003*"two" + 0.003*"training"'),
(1,
'0.006*"data" + 0.006*"learning" + 0.005*"set" + 0.005*"algorithm" + '
'0.005*"function" + 0.004*"model" + 0.004*"time" + 0.004*"problem" + '
'0.003*"using" + 0.003*"matrix"'),
(2,
'0.007*"learning" + 0.006*"model" + 0.005*"data" + 0.005*"algorithm" + '
'0.004*"time" + 0.004*"function" + 0.004*"using" + 0.004*"two" + 0.004*"one" '
'+ 0.003*"number"'),
(3,
'0.007*"learning" + 0.007*"model" + 0.006*"algorithm" + 0.004*"one" + '
'0.004*"data" + 0.004*"set" + 0.004*"using" + 0.004*"two" + 0.003*"function" '
'+ 0.003*"results"'),
(4,
'0.007*"model" + 0.006*"set" + 0.005*"learning" + 0.005*"data" + '
'0.004*"using" + 0.004*"algorithm" + 0.004*"function" + 0.004*"models" + '
'0.003*"time" + 0.003*"training"'),
(5,
'0.006*"learning" + 0.006*"algorithm" + 0.005*"data" + 0.005*"one" + '
'0.004*"model" + 0.004*"set" + 0.004*"function" + 0.004*"using" + '
'0.004*"number" + 0.003*"time"'),
(6,
'0.006*"model" + 0.006*"function" + 0.006*"data" + 0.006*"algorithm" + '
'0.005*"learning" + 0.005*"set" + 0.004*"using" + 0.004*"two" + 0.003*"one" '
'+ 0.003*"number"'),
(7,
'0.007*"model" + 0.006*"data" + 0.006*"learning" + 0.006*"algorithm" + '
'0.004*"models" + 0.004*"set" + 0.004*"function" + 0.004*"one" + '
'0.004*"using" + 0.003*"time"'),
(8,
'0.007*"learning" + 0.006*"model" + 0.005*"data" + 0.004*"algorithm" + '
'0.004*"using" + 0.004*"set" + 0.004*"used" + 0.003*"time" + 0.003*"one" + '
'0.003*"neural"'),
(9,
'0.006*"model" + 0.005*"data" + 0.005*"set" + 0.005*"algorithm" + '
'0.005*"learning" + 0.004*"function" + 0.004*"using" + 0.004*"problem" + '
'0.004*"time" + 0.003*"figure"')]
更进一步我们可以采用pyldavis进行进一步交互式的分析,更好看出topic自身的特点以及topic之间的关系
import pyLDAvis.gensim_models
import pyLDAvis
# Visualize the topics
pyLDAvis.enable_notebook()
LDAvis_prepared = pyLDAvis.gensim_models.prepare(lda_model, corpus, id2word)
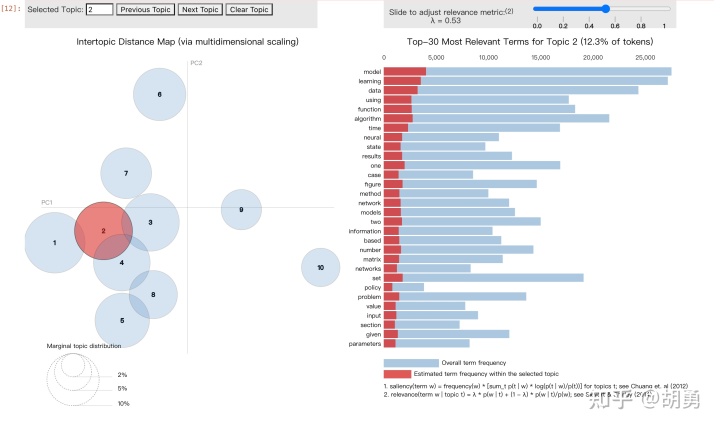