转自http://aa13382036813.blog.163.com/blog/static/12907901420091030101817372/
Introduction
There is many real-life problems which requires fast analyses and fast search in multidimensional data. The most popular way used for this problem is the so called k-d tree. It has the advantage that is easy to built and has a simple algorithm for closest points and ranged search. In this article I had studied the performance of the k-d tree for nearest-neighbour search. The analyses shows that k-d works quite well for small dimensions. However the number of the searched nodes rises exponentially with the space dimension and for N>10 k-d may become too slow. Another drawback is that the bassic k-d tree do not allows balancing, you should reorder the entire tree periodically to improve it balancing, which is not convenient for changing data and large datasets. There is some extensions of k-d which makes possible run-time balancing, but the problem with the big dimensions remains as well for all k-d variations. This leaves a space for new ideas and algorithms, with this article I hope to start a discussion about the k-d enhancements and more effective solutions of the multidimensional nearest neighbour problem.
The sources contain C++ template of k-d tree and the project which I used to test the tree. If you are not familiar with the binary trees you can read my introduction article about binary trees here.
Background
First I will define the problem which the KD solves. Lets have a N-dimensional point P with coordinates Px,Py.....Pn. The point coordinates are in real orthogonal and normalized cartesian space (I suppose that the KD tree can be built for arbitrary space as long as a distance() function is provided). We search in a dataset for all points which are inside a sphere with radius R and center in the given point - ranged search, or for just one or more nearest neighbours. Note that in a N-dimensional cartesian space the distance between two points is determined by:
where x1 and x2 are the both points coordinates and the superscript is the dimension index. It is possible to find the closest to P points as first just find all points closest to P in the first dimension, i.e. minimal (x1-x2)2distance, then to find among them all with minimal distance in the second dimension, and so on but it will be inefficient and very slow for large datasets. Even if we built N binary trees or sorted indexes in each dimension, there is no guarantee that if the both points are too closes in one dimension they are not going to be far in other and in such way the distance between them will be large. For a ranged search with radius R this means that we should check all points inside a cube with sides NR, besides that we will had to make N binary searches for each dimension. This is almost as much inefficient as a simple sequential search through all data.
From the said above it follows that there is no simple and trivial solution of the multi-dimensional nearest neighbours problem, it can not re reduced to N one-dimensional problems and there is no N-dimensional sorting and binary search.
In 3D the problem described above is contained in the following SQL query:


SELECT * FROM Table1 WHERE SQUARE(X - X0) + SQUARE(Y - Y0) + SQUARE(Z - Y0) <= SQUARE(R0) ;
for all points inside a sphere with center (x0,y0,z0) and radius R0, and:


SELECT * FROM Table1 WHERE ABS(X - X0) <= a/2 AND ABS(Y - Y0) <= a/2 AND ABS(Z - Y0) <= a/2 ;
for a cube with side a (Table1 contains 3 real-values columns - X,Y,z).
A typical relational database solves similar queries very inefficiently, for example in the second case it will first find all points that fulfils the first condition - de>ABS(X - X0) <= a/2de>, among them it will find all which fulfills the second condition - de>ABS(Y - Y0) <= a/2de> and then the third - de>ABS(Z - Z0) <= a/2de>. It is almost the same to check all records, which it will do when resolves complex equations like in the first query.
To find a more efficient way to search through N-dimensional data a generalization of the Binary Tree had to be built. It is accepted this tree to be called k-d Tree. The k-d tree nodes are just as the Binary Tree, each node has de>Left de>and de>Right de>neighbour and de>Parent de>link. However,instead of one value, each node contains a multidimensional point with N coordinates and also has a de>axis de>member which defines the split plane dimension. The split dimensions are rotated from the first to the N-th, it means that the nodes from the first level of the tree are splited to left and right with respect to the first dimension (x), the second level with respect to to the second dimension (y) and so on. When the N-th level is reached the split plane index is set again to zero. The scheme below shows the k-d tree structure:
The nodes from the first level are divided to left and right with respect to the X-axis, for a 2D space with (x,y) points this means that if x_node < x_parent, the node is placed to the left, otherwise is placed to the right. For the second level the only difference is that the split plane is y, if node_y < parent_y the node is placed to left otherwise is placed in the right. In this way the tree height rises linearly with space dimension. The problem with this depth rotation is that the tree can not be easily balanced, if we want to move a node up in the tree we had to displace nodes with different split planes, which destroys the tree dimension ordering.
Code
The declaration of k-d tree node for fixed space dimension SD is given below:


//KDTNode template class declarationKDTEMPLATE class KDNode{//members public: int axis ; //split dimension Xtype x[SD]; //N-dimensional point unsigned int id ; bool checked ; //flag needed for recursive parent check//member functionspublic: KDNode(Xtype* x0, int split_axis); //contructor KDNODE* Insert(Xtype* x); KDNODE* FindParent(Xtype* x0); KDNODE* Parent ; KDNODE* Left ; KDNODE* Right ;};
The axis members is integer with values between 0 and SD-1, it contain the dimension index of the split plane. The de>FindParent()de> member function finds the parent of a target point:


KDTEMPLATEKDNODE* KDNODE::FindParent(Xtype* x0){ KDNODE* parent ; KDNODE* next = this ; int split ; while(next) { split = next->axis ; parent = next ; if(x0[split] > next->x[split]) next = next->Right ; else next = next->Left ; } return parent ;}
Insert function links the new node and rotates the split plane index:


KDTEMPLATEKDNODE* KDNODE::Insert(Xtype* p){ KDNODE* parent = FindParent(p); if(equal(p, parent->x, SD)) return NULL ; KDNODE* newNode = new KDNODE(p, parent->axis +1 < SD? parent->axis+1:0); newNode->Parent = parent ; if(p[parent->axis] > parent->x[parent->axis]) { parent->Right = newNode ; newNode->orientation = 1 ; } else { parent->Left = newNode ; newNode->orientation = 0 ; } return newNode ;}
Nearest Neighbours Search
The nearest neighbour to a target point not contained in the tree can be found in several ways. The most easy way is the up-down search, it first find the target parent with de>FindParent()de>. Then it determines the distance to the parent - de>d_minde>. Each node of the tree splits the space in to halfs according to it split axis, all half-spaces which overlaps with a sphere with radius < de>d_minde> and centered in the target should be checked. The up-down function starts again from the tree root and eliminate all halfspaces too far from the target:


/* find the nearest neighbour, it start from the root finds the target parent, then start again from the root and and eliminate all halfspaces too far from the target*/KDTEMPLATEKDNODE* KDTREE::find_nearest(Xtype* x){ if(!Root) return NULL ; checked_nodes = 0; KDNODE* parent = Root->FindParent(x); nearest_neighbour = parent ; d_min = distance2(x, parent->x, SD); ; if(equal(x, parent->x, SD)) return nearest_neighbour ; check_subtree(Root, x); return nearest_neighbour ;}KDTEMPLATEvoid KDTREE::check_subtree(KDNODE* node, Xtype* x){ if(!node) return ; checked_nodes++ ; Xtype d = distance2(x, node->x, SD); ; if(d<d_min) { d_min = d; nearest_neighbour = node ; } int dim = node->axis ; d= node->x[dim] - x[dim]; if (d*d > d_min) { if (node->x[dim] > x[dim]) check_subtree(node->Left, x); else check_subtree(node->Right, x); } // If the distance to the target is // less than the nearest distance, we still need to look // in both directions. else { check_subtree(node->Left, x); check_subtree(node->Right, x); }}
The de>check_subtree()de> inspect all subtrees hyper-rectangles which are closer to the target then the current minimal distance. This eleminates all hyperrectangles which intersect the sphere and can contain child nodes closer to d_min.
However the up-down function will chech too much halfspaces when the tree root and the target point are too far away. Thus I developed a search function which start from the target parent and move up in the tree - down-up search. It uses the de>Parent de>link and goes up until the target is closed from all sides with large enough hyperrectangle, or if the tree root is reached:


KDTEMPLATEKDNODE* KDTREE::find_nearest(Xtype* x){ if(!Root) return NULL ; checked_nodes = 0; KDNODE* parent = Root->FindParent(x); nearest_neighbour = parent ; d_min = distance2(x, parent->x, SD); ; if(equal(x, parent->x, SD)) return nearest_neighbour ; search_parent(parent, x); uncheck(); return nearest_neighbour ;}KDTEMPLATEKDNODE* KDTREE::search_parent(KDNODE* parent, Xtype* x){ for(int k=0; k<SD; k++) { x_min[k] = x_max[k] = 0; max_boundary[k] = min_boundary[k] = 0; } n_boundary = 0; Xtype dx; KDNODE* search_root = parent ; while(parent && n_boundary != 2*SD) { check_subtree(parent, x); search_root = parent ; parent = parent->Parent ; } return search_root ;}
de>search_parent()de> goes up in the tree until the number of the founded boundaries becomes bigger then 2SD or the tree parent is reached. Each parent is checked recursively from de>check_subtree()de> which changes the minimal distance and increase n_boundary when finds a node further then d_min in it split dimension. I estimated that this is sometimes more then twice faster then the up-down search. However, as I mentioned in introduction, for both methods the number of the checked nodes rises as power of 2 factor with the space dimension. Below I had plotted the number of the checked nodes for the down-up search, the tests are made for 10000 points for SD between 2 and 10:
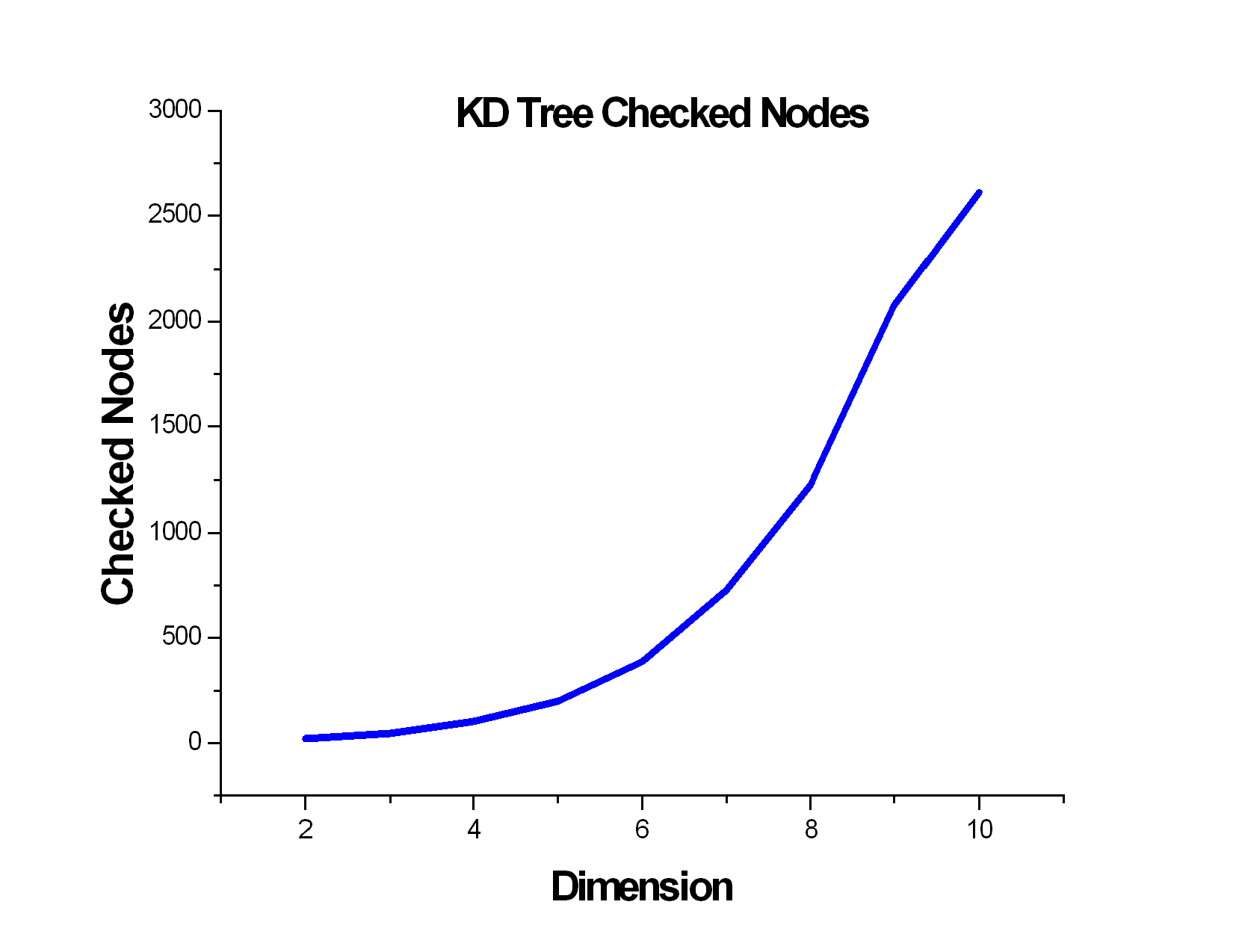
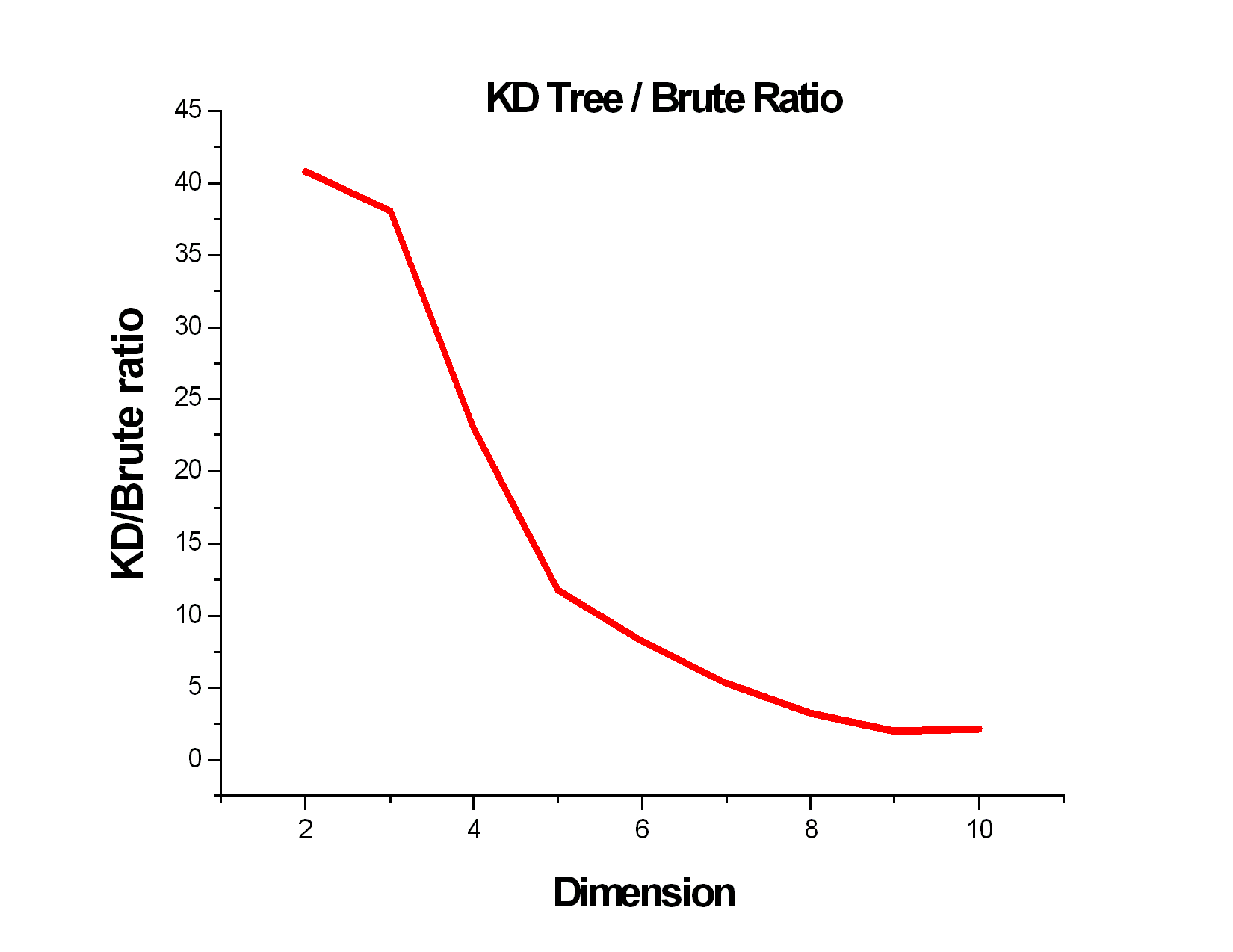
For small dimensions the KD-tree can be much faster then sequential search, however for 10-dimensional space the checked nodes for rises to about 25% for. It is possible to reduce this number 2-3 times if the tree is balanced but the tendency of exponential rise of the checked nodes makes the KD unpracticle above 15 dimensions. The second curve shows how KD / Brute ratio changes with space dimension, Brute stands for sequential search, it checks all nodes. Practiclly, for 10000 points, the KD tree becomes as fast as the sequential search for dimensions bigger then 10.
With the analyses made in this article I showed that the standard KD-tree is not good for dimensions beyond 10. In my next article about KD trees and the nearest neighbours search in N-dimensions I will introduce an extention of the quad tree for N-dimensional data - QD-tree, it is faster then KD and allows run-time balancing. However for big dimensions it again becomes slow. This problem is well known in the computational geometry, this would be so for any data structure based only on tree. From another hand it is not possible to make a multidimensional linked-list and to include it in the tree. The problem is quite complicated and requires new kinds data structures.
The Test Project
To test the tree just compile the console with Visual C++ 6.0 or later MS Visual Studio and run it in release mode. The program will generate random points and will report the tree creation times, the averaged search results for KD and sequential search and the search errors if there is such:
To modify the search for other dimensions and test point number use the following constants defined inKDtree.h:
- de>SD de>- the space dimension
- de>POINT_NUMde> - the random points inserted in the tree
- de>TEST_NUMde> - the random test points used for the tests
- de>KD_MAX_POINTSde> - change it if you want to test the tree for more then 2,000,000 points