目的:了解Keras上的IMDB数据集是怎么一回事。
前文主要是我的理解,官方解释在后文,觉得官方解析得不够到位。
数据源可以在[这里]找到。这数据集包含了50000条偏向明显的评论,其中25000条作为训练集,25000作为测试集。label为pos(positive)和neg(negative)。
比较难理解的是这些影评被处理后得到的词序列或者词向量。
另外在kaggle也有相似的数据,这里下载。
kaggle的IMDB数据集提供了一个CSV文件,而keras自带的那个数据源没有。看kaggle的CSV文件:
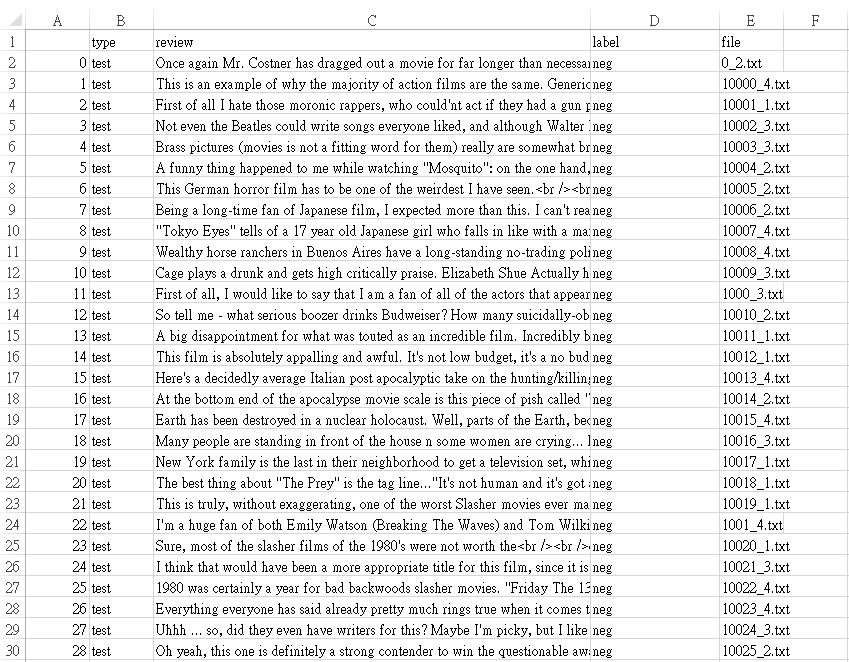
" Once again Mr. Costner has dragged out a movie for far longer than necessary. Aside from the terrific sea rescue sequences, of which there are very few I just did not care about any of the characters. Most of us have ghosts in the closet, and Costner’s character are realized early on, and then forgotten until much later, by which time I did not care. The character we should really care about is a very cocky, overconfident Ashton Kutcher. The problem is he comes off as kid who thinks he’s better than anyone else around him and shows no signs of a cluttered closet. His only obstacle appears to be winning over Costner. Finally when we are well past the half way point of this stinker, Costner tells us all about Kutcher’s ghosts. We are told why Kutcher is driven to be the best with no prior inkling or foreshadowing. No magic here, it was all I could do to keep from turning it off an hour in."
如何将这个内容转为一个词向量?
首先要有一个字典,字典有固定的长度,字典囊括了数据集中出现的词,词在字典中的位置按照词在数据集中出现的次数从大到小排列。比如这个字典中,‘the’在评论中出现次数最大,the放在字典的第一个位置上;‘and’出现的次数第二多,所以排在第二 …
我们可以在下载的数据中找到一个imdb.vocab文件,那就是字典,这个字典大小为89527。
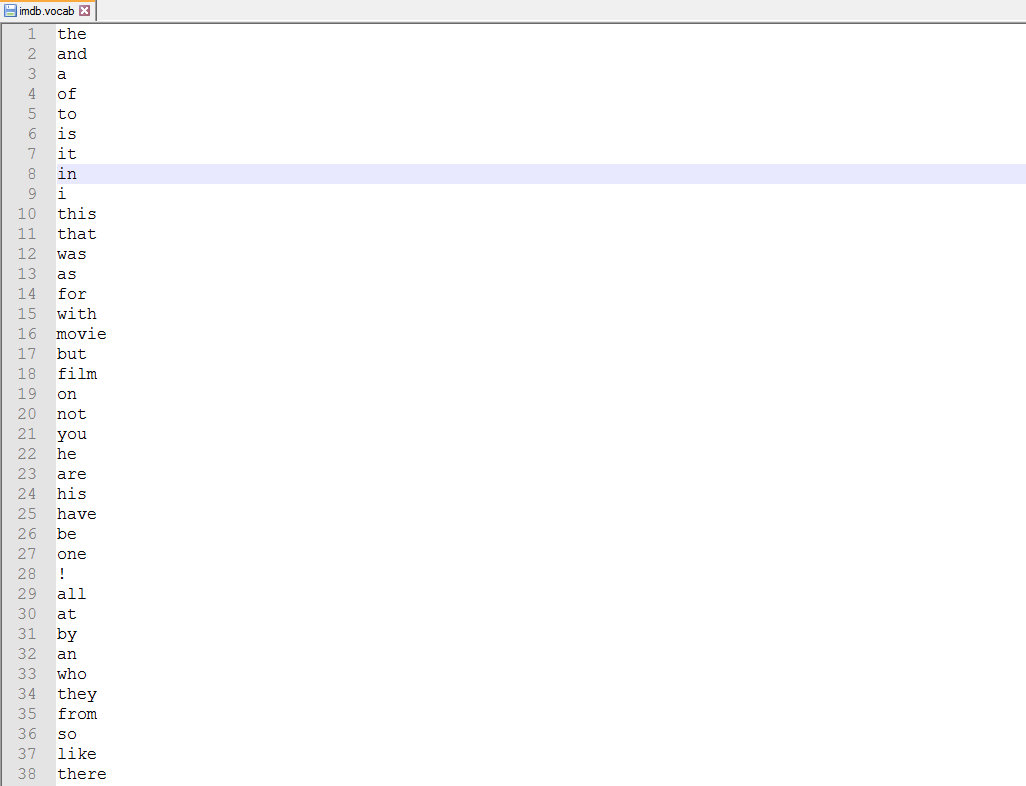
举个栗子:
评论为“I like this movie!”
‘I’在字典中的index为9;
‘like’在字典中的index为37;
‘this’‘在字典中的index为10;
‘movie’在字典中的index为16;
‘!’在字典中的index为28;
这个评论对应的词向量为[9 37 10 16 28]
比如:
# Embedding
max_features = 20000
maxlen = 100
embedding_size = 128
(x_train, y_train), (x_test, y_test) = imdb.load_data(num_words=max_features)
print(len(x_train), 'train sequences')
print(len(x_test), 'test sequences')
print(len(x_train[0]))
print(x_train[0])
# 设定向量的最大长度,小于这个长度的补0,大于这个长度的直接截端
x_train = sequence.pad_sequences(x_train, maxlen=maxlen)
x_test = sequence.pad_sequences(x_test, maxlen=maxlen)
print('x_train shape:', x_train.shape)
print('x_test shape:', x_test.shape)
25000 train sequences
25000 test sequences
218
[1, 14, 22, 16, 43, 530, 973, 1622, 1385, 65, 458, 4468, 66, 3941, 4, 173, 36, 256, 5, 25, 100, 43, 838, 112, 50, 670, 2, 9, 35, 480, 284, 5, 150, 4, 172, 112, 167, 2, 336, 385, 39, 4, 172, 4536, 1111, 17, 546, 38, 13, 447, 4, 192, 50, 16, 6, 147, 2025, 19, 14, 22, 4, 1920, 4613, 469, 4, 22, 71, 87, 12, 16, 43, 530, 38, 76, 15, 13, 1247, 4, 22, 17, 515, 17, 12, 16, 626, 18, 19193, 5, 62, 386, 12, 8, 316, 8, 106, 5, 4, 2223, 5244, 16, 480, 66, 3785, 33, 4, 130, 12, 16, 38, 619, 5, 25, 124, 51, 36, 135, 48, 25, 1415, 33, 6, 22, 12, 215, 28, 77, 52, 5, 14, 407, 16, 82, 10311, 8, 4, 107, 117, 5952, 15, 256, 4, 2, 7, 3766, 5, 723, 36, 71, 43, 530, 476, 26, 400, 317, 46, 7, 4, 12118, 1029, 13, 104, 88, 4, 381, 15, 297, 98, 32, 2071, 56, 26, 141, 6, 194, 7486, 18, 4, 226, 22, 21, 134, 476, 26, 480, 5, 144, 30, 5535, 18, 51, 36, 28, 224, 92, 25, 104, 4, 226, 65, 16, 38, 1334, 88, 12, 16, 283, 5, 16, 4472, 113, 103, 32, 15, 16, 5345, 19, 178, 32]
x_train shape: (25000, 100)
x_test shape: (25000, 100)
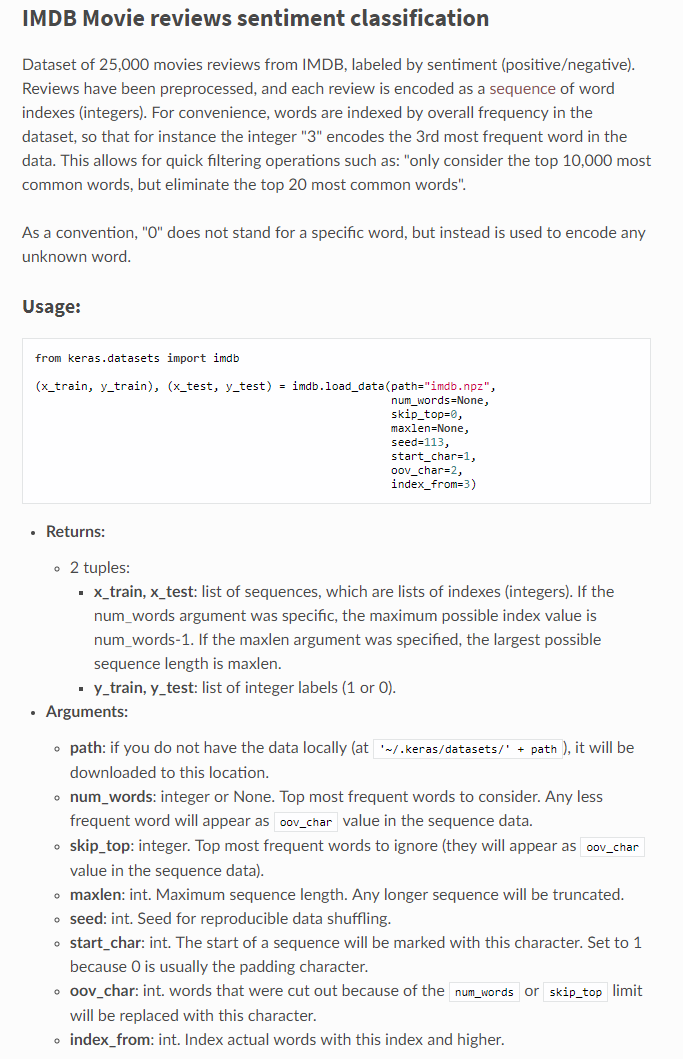
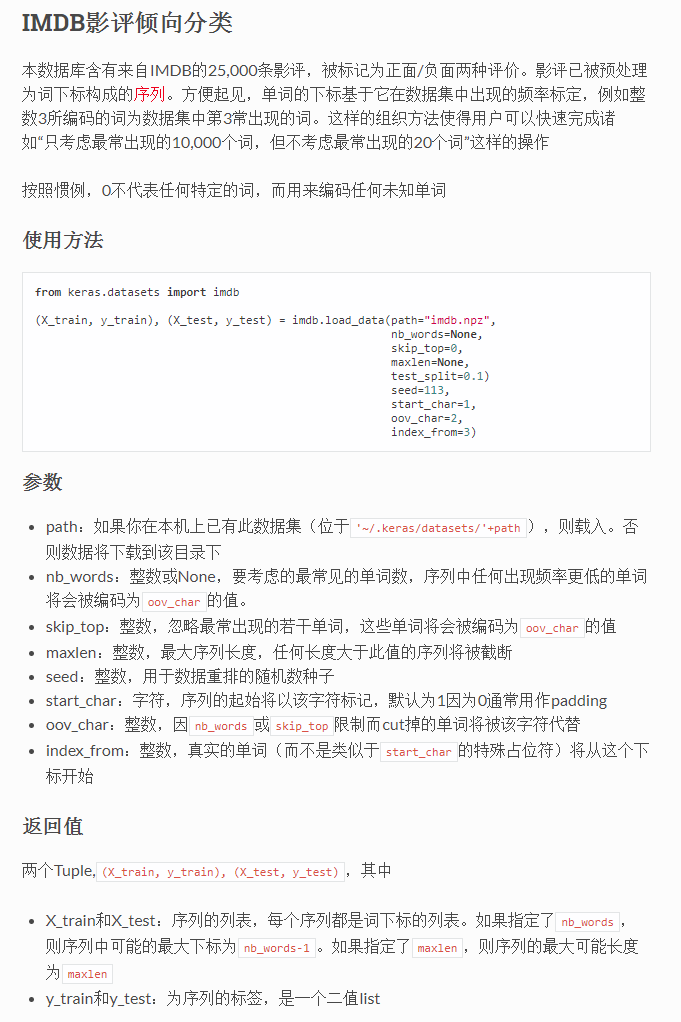