import gensim
import math
import jieba
import jieba.posseg as posseg
from jieba import analyse
from gensim import corpora, models
import functools
import numpy as np
# 停用词表加载方法
# 停用词表存储路径,每一行为一个词,按行读取进行加载
# 进行编码转换确保匹配准确率
def get_stopword_list():
stopword_path = '.\\stopword.txt'
stopword_list = [sw.replace('\n', '') for sw in open(stopword_path, encoding='utf8').readlines()]
return stopword_list
# 分词方法,调用结巴接口
def seg_to_list(sentence, pos=False):
if not pos:
# 不进行词性标注分词
seg_list = jieba.cut(sentence)
else:
# 进行词性标注分词
seg_list = posseg.cut(sentence)
return seg_list
# 去除干扰词
def word_filter(seg_list, pos=False):
stopword_list = get_stopword_list()
filter_list = []
# 根据POS参数选择是否词性过滤
# 不进行词性过滤,则将词性都标记为n,表示全部保留
for seg in seg_list:
if not pos:
word = seg
flag = 'n'
else:
word = seg.word
flag = seg.flag
if not flag.startswith('n'):
continue
# 过滤停用词表中的词,以及长度为<2的词
if word not in stopword_list and len(word) > 1:
filter_list.append(word)
return filter_list
# 数据加载,pos为是否词性标注的参数,corpus_path为数据集路径
def load_data(pos=False, corpus_path='.\\corpus.txt'):
# 调用上面方式对数据集进行处理,处理后的每条数据仅保留非干扰词
doc_list = []
for line in open(corpus_path, 'r', encoding='utf8'):
content = line.strip()
seg_list = seg_to_list(content, pos)
filter_list = word_filter(seg_list, pos)
doc_list.append(filter_list)
return doc_list
# idf值统计方法
def train_idf(doc_list):
idf_dic = {}
# 总文档数
tt_count = len(doc_list)
# 每个词出现的文档数 集合是去重的
for doc in doc_list:
for word in set(doc): # 这是一个集合 这个很关键 集合是去重的
idf_dic[word] = idf_dic.get(word, 0.0)+1.0
# 按公式转换为idf值,分母加1进行平滑处理
for k, v in idf_dic.items():
idf_dic[k] = math.log(tt_count/(v+1.0))
# 对于没有在字典中的词,默认其仅在一个文档出现,得到默认idf值
default_idf = math.log(tt_count/1.0)
return idf_dic, default_idf
# 排序函数,用于topK关键词的按值排序
def cmp(e1,
gensim进行LSI LSA LDA主题模型,TFIDF关键词提取,jieba TextRank关键词提取代码实现示例
最新推荐文章于 2024-06-04 16:21:44 发布
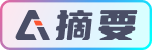