Distributed TensorFlow allows us to share parts of a TensorFlow graph between multiple processes, possibly each on a different machine.
Why might we want to do this? The classic use case is to harness the power of multiple machines for training, with shared parameters between all machines. Even if we’re just running on a single machine, though, I’ve come across two examples from reinforcement learning where sharing between processes has been necessary:
- In A3C, multiple agents run in parallel in multiple processes, exploring different copies of the environment at the same time. Each agent generates parameter updates, which must also be sent to the other agents in different processes.
- In Deep Reinforcement Learning from Human Preferences, one process runs an agent exploring the environment, with rewards calculated by a network trained from human preferences about agent behaviour. This network is trained asynchronously from the agent, in a separate process, so that the agent doesn’t have to wait for each training cycle to complete before it can continue exploring.
Unfortunately, the official documentation on Distributed TensorFlow rather jumps in at the deep end. For a slightly more gentle introduction - let’s run through some really basic examples with Jupyter.
If you’d like to follow along with this notebook interactively, sources can be found at GitHub.
(Note: some of the explanations here are my own interpretation of empirical results/TensorFlow documentation. If you see anything that’s wrong, give me a shout!)
Introduction
import tensorflow as tf
Let’s say we want multiple processes to have shared access to some common parameters. For simplicity, suppose this is just a single variable:
var = tf.Variable(initial_value=0.0)
As a first step, we can imagine that each process would need its own session. (Pretend session 1 is created in one process, and session 2 in another.)
sess1 = tf.Session()
sess2 = tf.Session()
sess1.run(tf.global_variables_initializer())
sess2.run(tf.global_variables_initializer())
Each call to tf.Session() creates a separate execution engine, then connects the session handle to the execution engine. The execution engine is what actually stores variable values and runs operations.
Normally, execution engines in different processes are unlinked. Changing var in one session (on one execution engine) won’t affect var in the other session.
print("Initial value of var in session 1:", sess1.run(var))
print("Initial value of var in session 2:", sess2.run(var))
sess1.run(var.assign_add(1.0))
print("Incremented var in session 1")
print("Value of var in session 1:", sess1.run(var))
print("Value of var in session 2:", sess2.run(var))
输出:
Initial value of var in session 1: 0.0
Initial value of var in session 2: 0.0
Incremented var in session 1
Value of var in session 1: 1.0
Value of var in session 2: 0.0
Distributed TensorFlow
In order to share variables between processes, we need to link the different execution engines together. Enter Distributed TensorFlow.
With Distributed TensorFlow, each process runs a special execution engine: a TensorFlow server. Servers are linked together as part of a cluster. (Each server in the cluster is also known as a task.)
The first step is to define what the cluster looks like. We start off with the simplest possible cluster: two servers (two tasks), both on the same machine; one that will listen on port 2222, one on port 2223.
tasks = ["localhost:2222", "localhost:2223"]
Each task is associated with a job, which is a collection of related tasks. We associate both tasks with a job called “local”.
jobs = {"local": tasks}
This completes the definition of the cluster.
cluster = tf.train.ClusterSpec(jobs)
We can now launch the servers, specifying which server in the cluster definition each server corresponds to. Each server starts immediately, listening on the port specified in the cluster definition.
# "This server corresponds to the the first task (task_index=0)
# of the tasks associated with the 'local' job."
server1 = tf.train.Server(cluster, job_name="local", task_index=0)
server2 = tf.train.Server(cluster, job_name="local", task_index=1)
With the servers linked together in the same cluster, we can now experience the main magic of Distributed TensorFlow: any variable with the same name will be shared between all servers.
The simplest example is to run the same graph on all servers, each graph with just one variable, as before:
tf.reset_default_graph()
var = tf.Variable(initial_value=0.0, name='var')
sess1 = tf.Session(server1.target)
sess2 = tf.Session(server2.target)
Modifications made to the variable on one server will now be mirrored on the second server.
sess1.run(tf.global_variables_initializer())
sess2.run(tf.global_variables_initializer())
print("Initial value of var in session 1:", sess1.run(var))
print("Initial value of var in session 2:", sess2.run(var))
sess1.run(var.assign_add(1.0))
print("Incremented var in session 1")
print("Value of var in session 1:", sess1.run(var))
print("Value of var in session 2:", sess2.run(var))
输出:
Initial value of var in session 1: 0.0
Initial value of var in session 2: 0.0
Incremented var in session 1
Value of var in session 1: 1.0
Value of var in session 2: 1.0
(Note that because we only have one variable, and that variable is shared between both sessions, the second run of global_variables_initializer here is redundant.)
Placement
A question that might be in our minds at this point is: which server does the variable actually get stored on? And for operations, which server actually runs them?
Empirically, it seems that by default, variables and operations get placed on the first task in the cluster.
def run_with_location_trace(sess, op):
# From https://stackoverflow.com/a/41525764/7832197
run_options = tf.RunOptions(trace_level=tf.RunOptions.FULL_TRACE)
run_metadata = tf.RunMetadata()
sess.run(op, options=run_options, run_metadata=run_metadata)
for device in run_metadata.step_stats.dev_stats:
print(device.device)
for node in device.node_stats:
print(" ", node.node_name)
For example, if we do something to var using the session connected to the first task, everything happens on that task:
run_with_location_trace(sess1, var)
输出:
/job:local/replica:0/task:0/device:CPU:0
_SOURCE
var
run_with_location_trace(sess1, var.assign_add(1.0))
输出:
/job:local/replica:0/task:0/device:CPU:0
_SOURCE
AssignAdd_1/value
var
AssignAdd_1
But if we try and try do something to var using the session connected to the second task, the graph nodes still get run on the first task.
run_with_location_trace(sess2, var)
输出:
/job:local/replica:0/task:1/device:CPU:0
_SOURCE
/job:local/replica:0/task:0/device:CPU:0
_SOURCE
var
To fix a variable or an operation to a specific task, we can use tf.device:
with tf.device("/job:local/task:0"):
var1 = tf.Variable(0.0, name='var1')
with tf.device("/job:local/task:1"):
var2 = tf.Variable(0.0, name='var2')
# (This will initialize both variables)
sess1.run(tf.global_variables_initializer())
Now var1 runs on the first task, as before.
run_with_location_trace(sess1, var1)
输出:
/job:local/replica:0/task:0/device:CPU:0
_SOURCE
var1
But var2 runs on the second task. Even if we try to evaluate it using the session connected to the first task, it still runs on the second task.
run_with_location_trace(sess1, var2)
输出:
/job:local/replica:0/task:0/device:CPU:0
_SOURCE
/job:local/replica:0/task:1/device:CPU:0
_SOURCE
var2
And vice-versa with var2.
run_with_location_trace(sess2, var2)
输出:
/job:local/replica:0/task:1/device:CPU:0
_SOURCE
var2
run_with_location_trace(sess2, var1)
输出:
/job:local/replica:0/task:1/device:CPU:0
_SOURCE
/job:local/replica:0/task:0/device:CPU:0
_SOURCE
var1
Graphs
There are a couple of things to note about how graphs work with Distributed TensorFlow.
Who builds the graph?
First, although variable values are shared throughout the cluster, the graph is not automatically shared.
Let’s create a fresh cluster with two servers, and set up the first server with an explicitly-created graph.
cluster = tf.train.ClusterSpec({"local": ["localhost:2224", "localhost:2225"]})
server1 = tf.train.Server(cluster, job_name="local", task_index=0)
server2 = tf.train.Server(cluster, job_name="local", task_index=1)
graph1 = tf.Graph()
with graph1.as_default():
var1 = tf.Variable(0.0, name='var')
sess1 = tf.Session(target=server1.target, graph=graph1)
print(graph1.get_operations())
输出:
[<tf.Operation 'var/initial_value' type=Const>, <tf.Operation 'var' type=VariableV2>, <tf.Operation 'var/Assign' type=Assign>, <tf.Operation 'var/read' type=Identity>]
If we then create a session connected to the second server, note that the graph does not automatically get mirrored.
graph2 = tf.Graph()
sess2 = tf.Session(target=server2.target, graph=graph2)
print(graph2.get_operations())
输出:
[]
To access the shared variable, we must manually add a variable with the same name to the second graph.
with graph2.as_default():
var2 = tf.Variable(0.0, name='var')
Only then can we access it.
sess1.run(var1.assign(1.0))
sess2.run(var2)
输出:
1.0
The takeaway is: each server is responsible for building its own graph.
Does the graph have to be the same on all servers?
So far, all our examples have run the same graph structure on both servers. This is known as in-graph replication.
For example, let’s say we have a cluster containing three servers. Server 1 holds shared parameters, while server 2 and server 3 are worker nodes, each with local variables. With in-graph replication, each server’s graphs would look like:
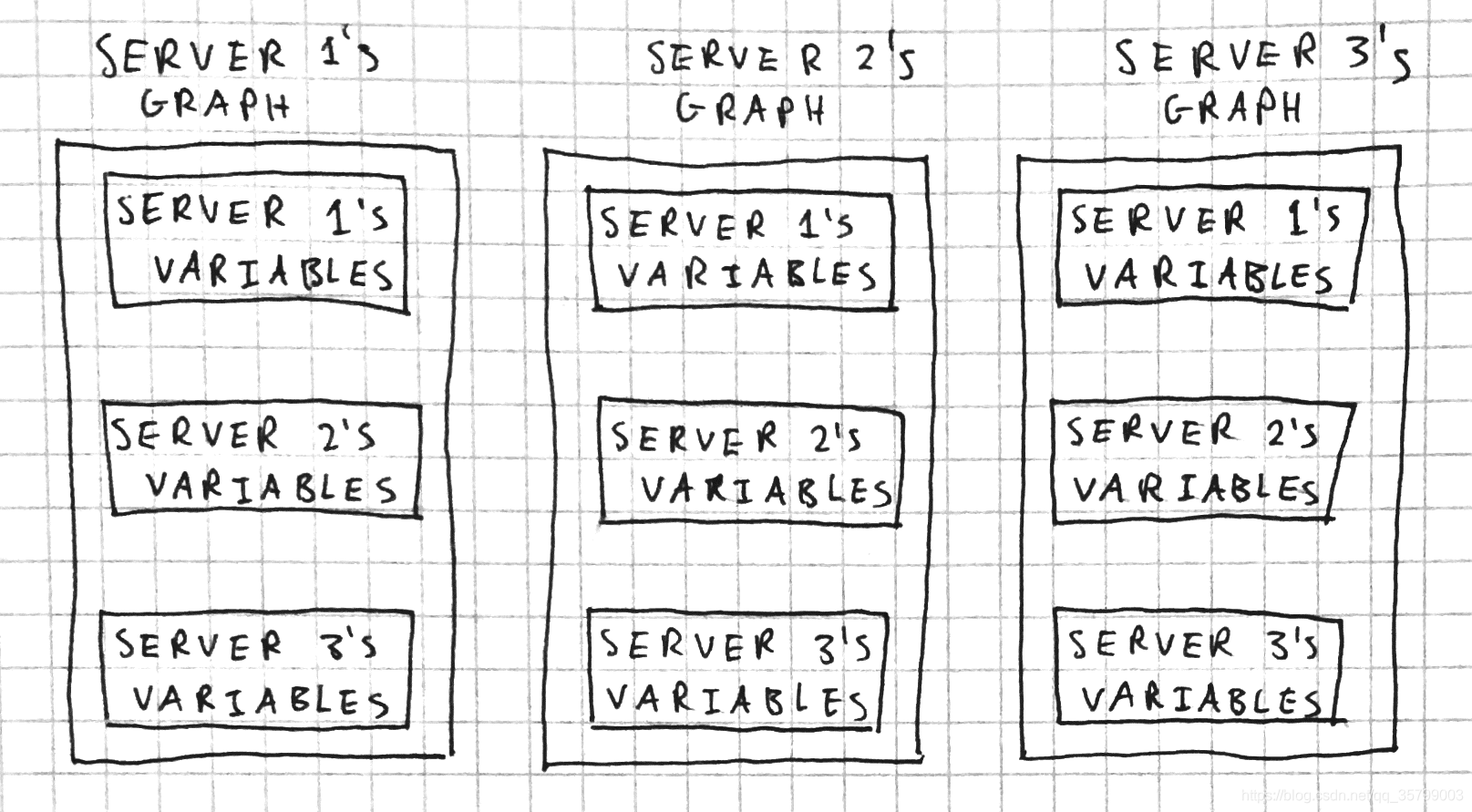
The issue with in-graph replication is that every server has to have a copy of the entire graph, including the parts of the graph that might only be relevant for other servers. This can lead to graphs growing very large.
The alternative is between-graph replication. Here, each server runs a graph containing only the shared parameters, and whatever variables and operations are relevant to that individual server.
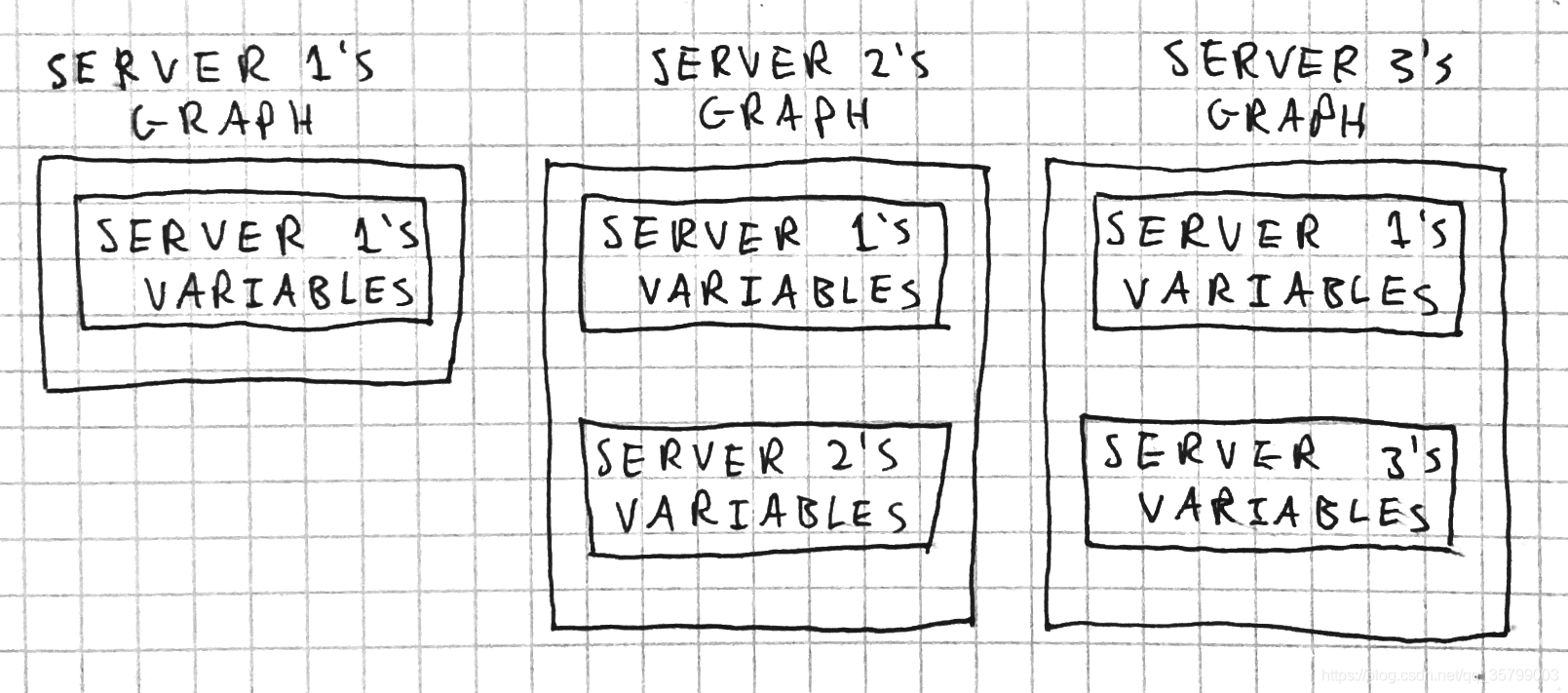
Because it keeps graph sizes smaller, between-graph replication is the recommended approach.
原文:http://amid.fish/assets/Distributed TensorFlow - A Gentle Introduction.html