Sobel
Roberts算子:
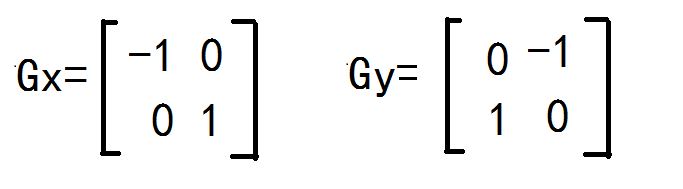
Prewitt算子:
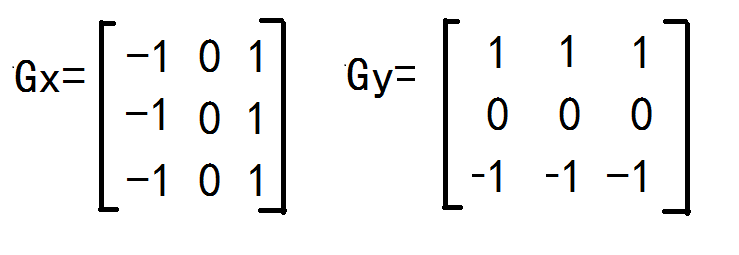
Sobel算子:
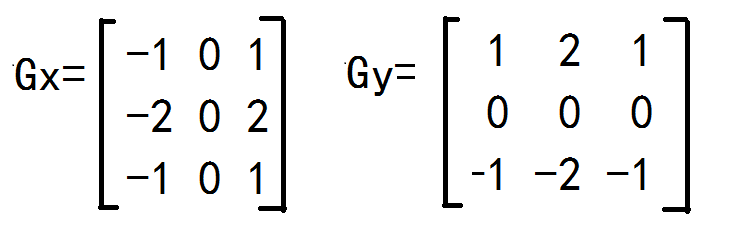
Laplacian算子:
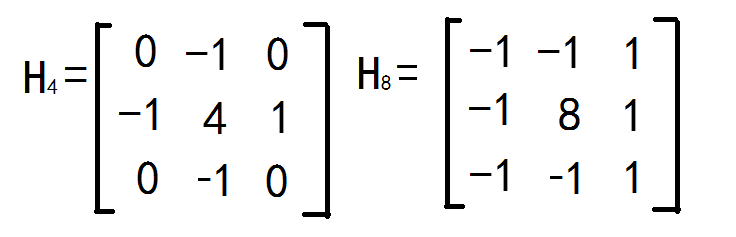
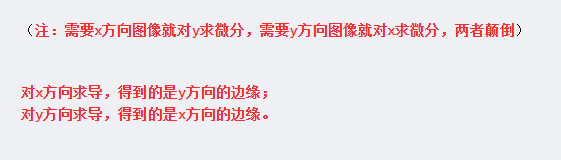
步骤:
灰度图与卷积核相卷
对结果求绝对值
方法2=方法1
#方法1:
sx = cv2.Sobel(img,ddepth=cv2.CV_64F,dx=1,dy=0,ksize=3)
sx = cv2.convertScaleAbs(sx)
#方法2:
kernelx = np.array([[-1,0,1],[-2,0,2],[-1,0,1]],dtype=int)
ix = cv2.filter2D(img,cv2.CV_64F,kernelx)
ix = cv2.convertScaleAbs(ix)
iy = cv2.filter2D(img,cv2.CV_64F,kernely)
iy = cv2.convertScaleAbs(iy)
ixy = cv2.addWeighted(ix, 0.5, iy, 0.5, 0)
Canny
步骤:
高斯滤波
sobel计算梯度幅值和方向
NMS非极大值抑制:8个方向,4条直线,前后直线上最大值则保留,否为0
双阈值检测:>th2保留,<th1去掉,中间看四周有大的就保留
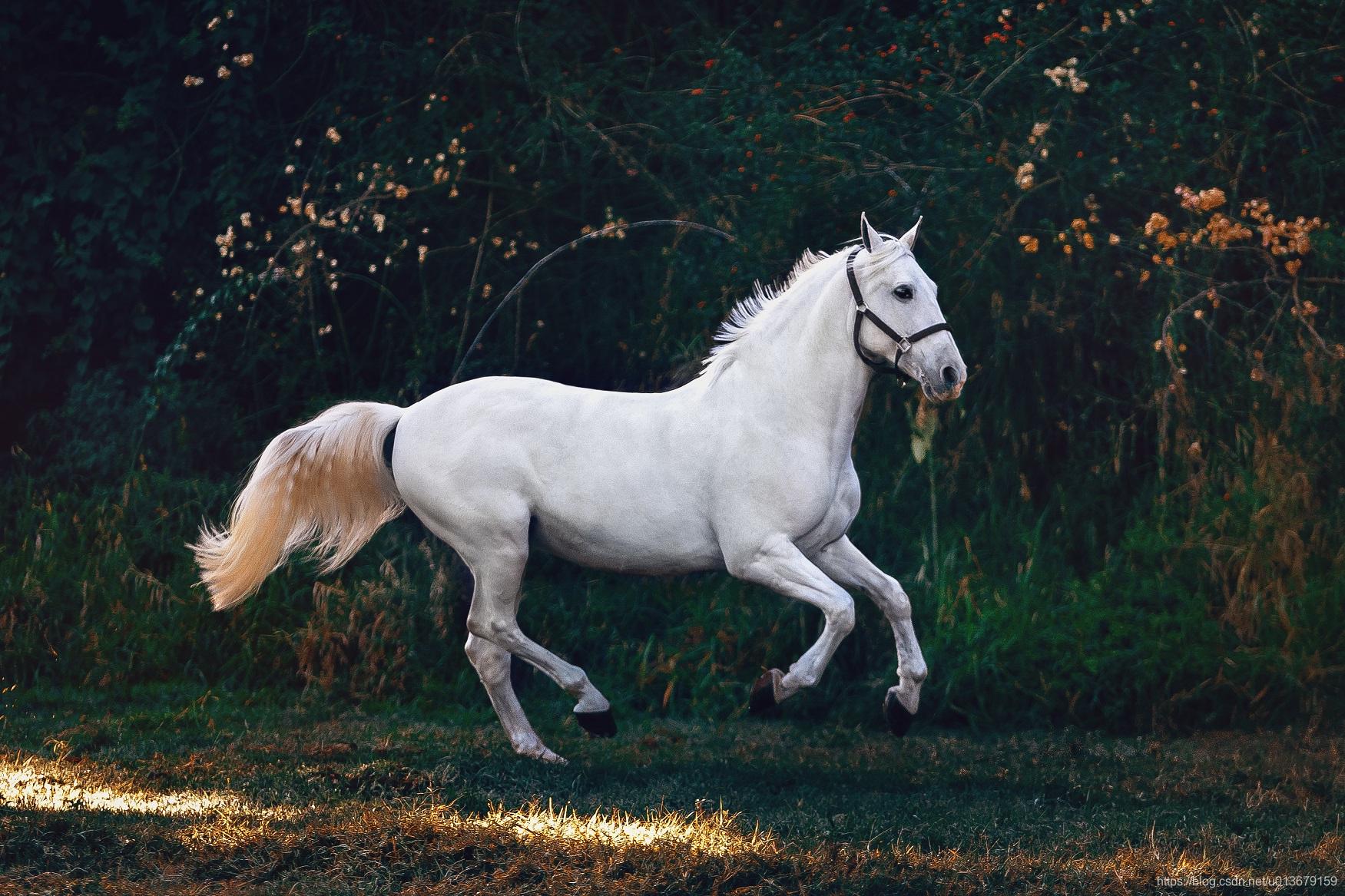
代码就不一一贴了,都放在后面了。
第一步,高斯滤波就是卷积高斯核

第二步,梯度方向和幅值
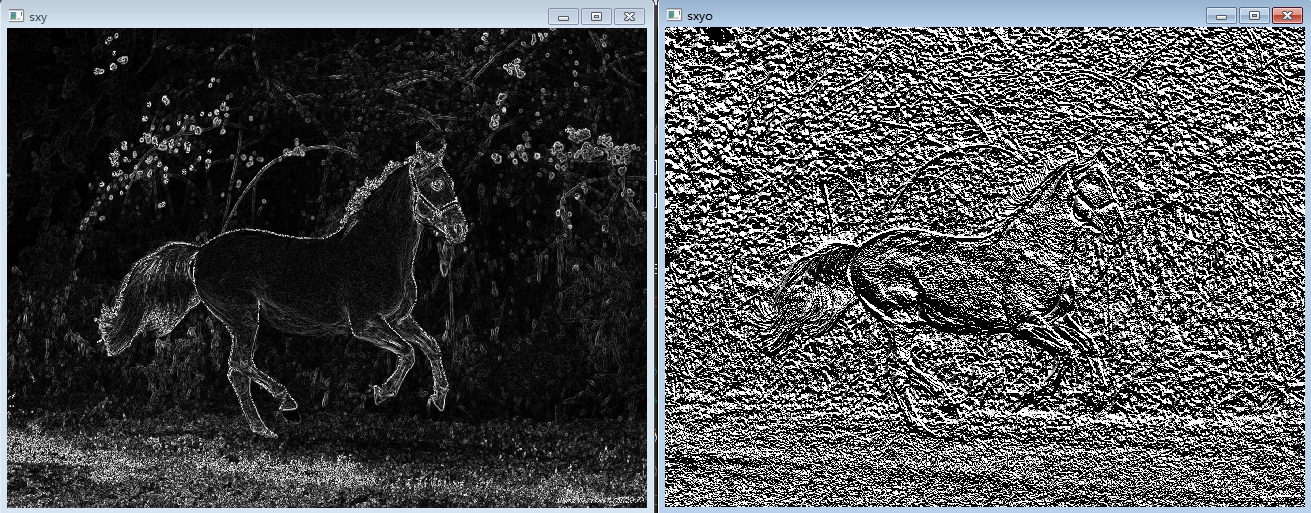
第三步,NMS,暴力计算,很慢,被去掉了很多
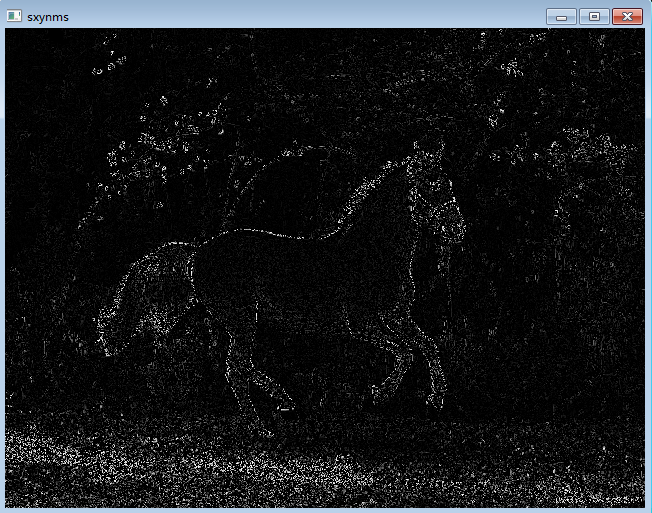
第四步,双阈值检测,很慢
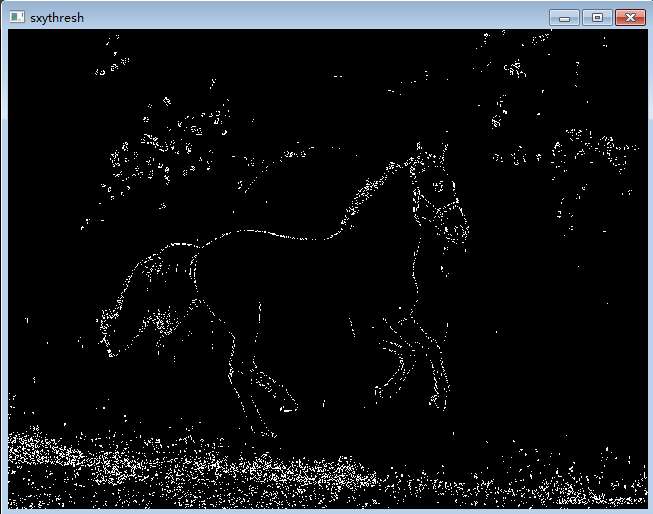
cv2接口运行结果,还是存在一些差别
imgcanny = cv2.Canny(img,th1,th2,apertureSize=3,L2gradient=True)
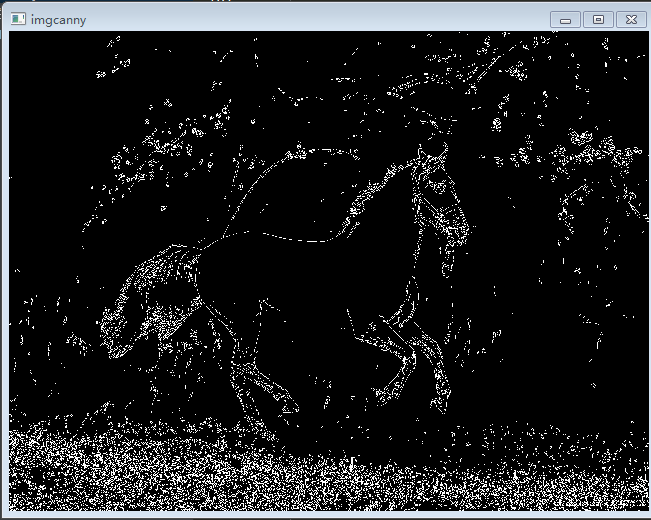
代码。
def CannyApi(th1,th2):
#第一步,高斯模糊
kernel = cv2.getGaussianKernel(5,1)* cv2.getGaussianKernel(5,1).T
imgblur = cv2.filter2D(img, cv2.CV_64F, kernel)
imgblur = cv2.convertScaleAbs(imgblur)
#第二步,计算梯度幅值和强度,同sobel
sx = cv2.Sobel(imgblur, ddepth=cv2.CV_64F, dx=1, dy=0, ksize=3)
sy = cv2.Sobel(imgblur, ddepth=cv2.CV_64F, dx=0, dy=1, ksize=3)
sxy =np.sqrt(sx*sx+sy*sy)
sxy = np.array(sxy,dtype=np.uint8)#显示很重要
sxy[sxy>255] = 255
cv2.namedWindow("sxy",0)
cv2.resizeWindow("sxy",640,480)
cv2.imshow("sxy",sxy)
# #显示结果不一样dtype=np.uint8,数据对比一下
# ix = cv2.convertScaleAbs(sx)
# c = ix-np.sqrt(sx*sx)
# print(np.max(c),np.min(c))
#cv2.imshow("ix", ix)
#方向
sxyo = np.arctan2(sy,sx)
cv2.namedWindow("sxyo",0)
cv2.resizeWindow("sxyo",640,480)
cv2.imshow("sxyo", sxyo)
# cv2.waitKey(0)
##第三步,NMS非极大值抑制,遍历在它的方向上是局部最大值,四个方向
sxynms = sxy
for i in range(1,sxy.shape[0]-1):#rows1167
for j in range(1,sxy.shape[1]-1):#cols1751
dir = sxyo[i][j]*180/np.pi#[-90,90]
dir = dir+180 if dir<0 else dir#[0,180]
c = sxy[i][j]
p=255
n=255
if dir< 22.5 or dir>157.5:
p = sxy[i][j+1]
n = sxy[i][j-1]
elif dir>=22.5 and dir<67.5:
p = sxy[i-1][j+1]
n = sxy[i+1][j-1]
elif dir >= 67.5 and dir < 112.5:
p = sxy[i-1][j]
n = sxy[i+1][j]
elif dir>=112.5 and dir<=157.5:
p = sxy[i+1][j+1]
n = sxy[i-1][j-1]
if c<p or c<n:
sxynms[i][j] = 0
c = sxy - sxynms
print(np.max(c),np.min(c))
cv2.namedWindow("sxynms",0)
cv2.resizeWindow("sxynms",640,480)
cv2.imshow("sxynms", sxynms)
#cv2.waitKey(0)
#第四步,双阈值,> Y,<N,看周围有没有
sxythresh = sxynms
for i in range(0, sxynms.shape[0]): # rows1167
for j in range(0, sxynms.shape[1]): # cols1751
c = sxynms[i][j]
si = 0 if i-1<0 else i-1
ei = sxynms.shape[0]-1 if i+2>sxynms.shape[0]-1 else i+2
sj = 0 if j-1<0 else j-1
ej = sxynms.shape[1]-1 if j+2>sxynms.shape[1]-1 else j+2
s = sxynms[si:ei,sj:ej]
if np.max(s)>=th2 and c>=th1:
sxythresh[i][j] = 255
else:
sxythresh[i][j] = 0
cv2.namedWindow("sxythresh",0)
cv2.resizeWindow("sxythresh",640,480)
cv2.imshow("sxythresh", sxythresh)
#API调用
imgcanny = cv2.Canny(img,th1,th2,apertureSize=3,L2gradient=True)
c = imgcanny - sxythresh
print(np.max(c),np.min(c))
cv2.namedWindow("imgcanny",0)
cv2.resizeWindow("imgcanny",640,480)
cv2.imshow("imgcanny", imgcanny)
cv2.waitKey(0)