Word2Vec简介
Word2Vec在2013年由Google提出,是用来生成词向量(word vector或word embedding)的工具,这样词与词之间就可以定量的去度量他们之间的关系,挖掘词之间的联系。
Word2Vec工具主要包含两个模型:跳字模型(Skip-gram)和连续词袋模型(Continuous Bag-of-Word,简称CBoW)。
CBow:周围词预测中心词。
Skip-gram:中心词预测周围词。

LSA和word2vec作为两大类方法的代表,一个是利用了全局特征的矩阵分解方法(Matrix Factorization Methods),一个是利用局部上下文的方法(Shallow Window-Based Methods、基于浅窗口的方法)。
Wikipedia2Vec简介
Wikipedia2Vec是一个从维基百科学习单词和实体嵌入的工具。学习的嵌入映射相似的单词和实体在一个连续的向量空间中彼此接近。
这个工具通过迭代整个维基百科页面来学习单词和实体的嵌入,并联合优化以下三个子模型:Wikipedia link graph model、Word-based skip-gram model、Anchor context model,这3个子模型基于skip-gram model。
提取特征
WiKipedia2Vec支持12种语言,在“Pretrained Embeddings”选项卡内下载参数文件,如图我们下载3个维度(100d、300d、500d)的在English Dump of Wikipedia上预训练的模型参数文件。
单词
注意转换前将 单词转为小写,否则可能word2vec失败(即wikipedia2vec中是小写单词与feature vector之间的对应关系)
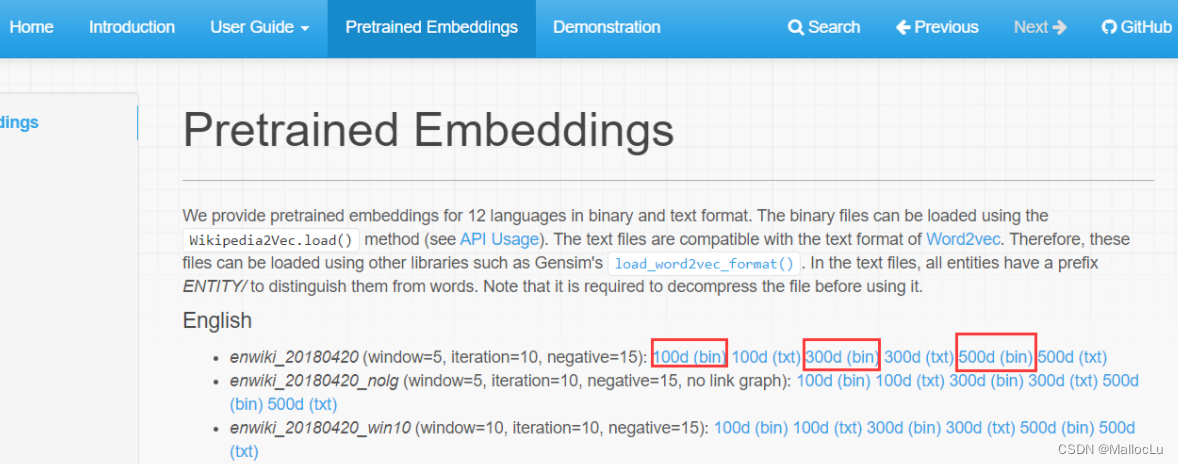
将上面的3个.pkl文件与main.py文件放入同一目录下即可
"""
main.py
**注意转换前将 单词转为小写,否则可能word2vec失败**
官网:https://wikipedia2vec.github.io/wikipedia2vec/
环境:
pip install wikipedia2vec
pip install nltk
功能:
wordpunctAndStopwords 获取单个句子中的单词列表 转小写(可选) 去标点(可选) 去停用词(可选)
(使用English Dump of Wikipedia训练的skip-gram model模型提取单词特征)
wikipediaWord2Vec 将 单个单词 转换为 词向量(注意转换前将 单词转为小写,否则可能word2vec失败)
wikipediaWords2Vec 将 列表单词 转换为 词向量列表(注意转换前将 单词转为小写,否则可能word2vec失败)
wikipediaSent2Vec 将 句子中的单词 转换为 词向量列表(注意参数to_lower=True将会转换句子为小写)
"""
from wikipedia2vec import Wikipedia2Vec
import numpy as np
from nltk.corpus import stopwords
from nltk import wordpunct_tokenize
import string
wiki2vec500 = Wikipedia2Vec.load('enwiki_20180420_500d.pkl')
wiki2vec300 = Wikipedia2Vec.load('enwiki_20180420_300d.pkl')
wiki2vec100 = Wikipedia2Vec.load('enwiki_20180420_100d.pkl')
def wikipediaWord2Vec(word, vecLen=500):
"""
将 单个单词 转换为 词向量
:param word: 单个单词
:param vecLen: 词向量长度 100/300/500
:return: numpy array float32 shape[0](转换失败)或shape[vecLen](转换成功)
"""
if vecLen != 100 and vecLen != 300 and vecLen != 500:
print('vecLen must in [100, 300, 500]')
return np.array([])
res = []
try:
if vecLen == 500:
res = wiki2vec500.get_word_vector(word)
elif vecLen == 300:
res = wiki2vec300.get_word_vector(word)
else:
res = wiki2vec100.get_word_vector(word)
except:
print(f'不存在单词{word}')
return np.array(res)
def wikipediaWords2Vec(words, vecLen=500):
"""
将 列表单词 转换为 词向量列表
:param words: 单词列表
:param vecLen: 词向量长度 100/300/500
:return: numpy array float32 shape[0]或shape[n, vecLen](n为单词数)
"""
if vecLen != 100 and vecLen != 300 and vecLen != 500:
print('vecLen must in [100, 300, 500]')
return np.array([])
result = []
for item in words:
t = wikipediaWord2Vec(item, vecLen)
if t.__len__() > 0:
result.append(t)
return np.array(result)
def wordpunctAndStopwords(sent, without_stopwords=True, without_punctuation=True, to_lower=True):
"""
获取单个句子中的单词列表 转小写(可选) 去标点(可选) 去停用词(可选)
:param sent: 单个句子
:param without_stopwords: 去停用词
:param without_punctuation: 去标点
:param to_lower: 转小写
:return: 单词列表
"""
# 转小写
if to_lower:
sent = sent.lower()
# 去除标点符号
if without_punctuation:
sent = sent.translate(str.maketrans(string.punctuation, " " * len(string.punctuation)))
# 分词
result = wordpunct_tokenize(sent)
# 去停用词
if without_stopwords:
stop_words = stopwords.words('english')
result = [w for w in result if not w in stop_words]
return result
def wikipediaSent2Vec(sent, vecLen=500, without_stopwords=True, without_punctuation=True, to_lower=True):
"""
将 句子中的单词 转换为 词向量列表
:param sent: 单个句子
:param vecLen: 词向量长度 100/300/500
:param without_stopwords: 去停用词
:param without_punctuation: 去标点
:param to_lower: 转小写
:return: numpy array float32 shape[0]或shape[n, vecLen](n为可用单词数)
"""
if vecLen != 100 and vecLen != 300 and vecLen != 500:
print('vecLen must in [100, 300, 500]')
return np.array([])
return wikipediaWords2Vec(wordpunctAndStopwords(sent, without_stopwords, without_punctuation, to_lower), vecLen)
if __name__ == '__main__':
# 测试
sent = 'One of the largest segments of the service industry, although largely unrecognised as an entity ' \
'in some of these countries, is travel and tourism.'
vecs1 = wikipediaSent2Vec(sent, vecLen=100)
print(vecs1.shape)
# (12, 100)
vecs2 = wikipediaSent2Vec(sent, vecLen=300)
print(vecs2.shape)
# (12, 300)
vecs3 = wikipediaSent2Vec(sent, vecLen=500)
print(vecs3.shape)
# (12, 500)
vecs4 = wikipediaSent2Vec(sent, vecLen=500, without_stopwords=False)
print(vecs4.shape)
# (24, 500)
vecs5 = wikipediaSent2Vec(sent, vecLen=500, without_punctuation=False)
print(vecs5.shape)
# 不存在单词,
# 不存在单词,
# 不存在单词.
# (12, 500)
vecs6 = wikipediaSent2Vec(sent, vecLen=500, to_lower=False)
print(vecs6.shape)
# 不存在单词One
# (11, 500)
# 单词字典键
the in of a and is to was by for
on as at from with an it that also which
first this has he one his are after who were
two its new be or but had their been born
not other all have during time when may they into
...
实体
注意实体均为开头字母大写的单词或单词组
from wikipedia2vec import Wikipedia2Vec
import numpy as np
from nltk.corpus import stopwords
from nltk import wordpunct_tokenize
import string
wiki2vec500 = Wikipedia2Vec.load('enwiki_20180420_500d.pkl')
wiki2vec300 = Wikipedia2Vec.load('enwiki_20180420_300d.pkl')
wiki2vec100 = Wikipedia2Vec.load('enwiki_20180420_100d.pkl')
print(wiki2vec100.get_entity_vector('Joel Asaph Allen'))
# 实体字典键
United_States Race_and_ethnicity_in_the_United_States_Census Association_football World_War_II France Germany India New_York_City United_Kingdom Canada
Iran Australia Moth England World_War_I Italy National_Register_of_Historic_Places London Japan China
Russia Village Catholic_Church California Romanization Brazil Spain Species Los_Angeles Genus
Census Netherlands The_New_York_Times Paris Family_(biology) Departments_of_France Soviet_Union Mexico Sweden American_football
Population_density Washington,_D.C. Communes_of_France Poland Europe United_States_Census_Bureau Scotland South_Africa Unincorporated_area U.S._state
...