gradient,Sobel、Canny、Seam Carving
提示:写完文章后,目录可以自动生成,如何生成可参考右边的帮助文档
提示:代码能直接跑的,放图片文章过长了,想看哪个图 直接imshow吧
一、图片求梯度
- 需要理解导数、微分、偏导数、梯度、差分,间有何区别。
- 连续函数的偏导数:
对于离散数据,用差分近似偏导数。 近似表示时,又有向前、向后、中心差分等
二、Sobel
https://homepages.inf.ed.ac.uk/rbf/HIPR2/sobel.htm
- sobel的核
Sobel算子结合了高斯平滑和微分操作,能够减少噪声的影响,在一定程度上保持了边缘的连续性和定位精度。
x方向的核:
y方向的核:
三、Canny
1. 算法流程
- smoothing
- gradient
- Non-maximum suppression:线性插值,将软边缘变成硬边缘
- Linking and thresholding:设置max, min 高于上阈值的当作真边缘,低于min当作假边缘,处于中间的,看周围是否有和真边缘连接,有则保留。
2. 代码实现
- smooth
def smooth(im, gauss_kernel):
W, H = im.shape
k_size = gauss_kernel.shape[0]
new_gray = np.zeros([W - k_size + 1, H - k_size + 1])
for i in range(W - k_size):
for j in range(H - k_size):
new_gray[i, j] = np.sum(im[i: i+k_size, j: j+k_size] * gauss_kernel)
return new_gray
- gradients
def gradients(im, x_kernel, y_kernel):
W, H = im.shape
if(x_kernel.shape[0] != y_kernel.shape[0]):
print("x_kernel.shape[0] != y_kernel.shape[0]")
exit(1)
k_size = x_kernel.shape[0]
dx = np.zeros([W - k_size + 1, H - k_size + 1])
dy = np.zeros([W - k_size + 1, H - k_size + 1])
M = np.zeros([W - k_size + 1, H - k_size + 1])
for i in range(W - k_size):
for j in range(H - k_size):
dx[i, j] = np.sum(im[i: i+k_size, j: j+k_size] * x_kernel)
dy[i, j] = np.sum(im[i: i+k_size, j: j+k_size] * y_kernel)
M[i, j] = np.sqrt(np.square(dx[i, j]) + np.square(dy[i, j]))
return dx, dy, M
- Non-maximum suppression
def NMS(M, dx, dy):
d = np.copy(M)
W, H = M.shape
NMS = np.copy(d)
NMS[0, :] = NMS[W - 1, :] = NMS[:, 0] = NMS[:, H - 1] = 0
for i in range(1, W - 1):
for j in range(1, H - 1):
if M[i, j] == 0:
NMS[i, j] = 0
else:
gradX = dx[i, j]
gradY = dy[i, j]
gradTemp = d[i, j]
if np.abs(gradY) > np.abs(gradX):
weight = np.abs(gradX) / np.abs(gradY)
grad2 = d[i - 1, j]
grad4 = d[i + 1, j]
if gradX * gradY > 0:
grad1 = d[i - 1, j - 1]
grad3 = d[i + 1, j + 1]
else:
grad1 = d[i - 1, j + 1]
grad3 = d[i + 1, j - 1]
else:
weight = np.abs(gradY) / np.abs(gradX)
grad2 = d[i, j - 1]
grad4 = d[i, j + 1]
if gradX * gradY > 0:
grad1 = d[i + 1, j - 1]
grad3 = d[i - 1, j + 1]
else:
grad1 = d[i - 1, j - 1]
grad3 = d[i + 1, j + 1]
gradTemp1 = weight * grad1 + (1 - weight) * grad2
gradTemp2 = weight * grad3 + (1 - weight) * grad4
if gradTemp >= gradTemp1 and gradTemp >= gradTemp2:
NMS[i, j] = gradTemp
else:
NMS[i, j] = 0
return NMS
- thresholding
def double_threshold(NMS, minval, maxval):
W, H = NMS.shape
DT = np.zeros([W, H])
TL = minval * np.max(NMS)
TH = maxval * np.max(NMS)
for i in range(1, W - 1):
for j in range(1, H - 1):
if (NMS[i, j] < TL):
DT[i, j] = 0
elif (NMS[i, j] > TH):
DT[i, j] = 1
elif (NMS[i - 1, j - 1:j + 1] < TH).any() or (
NMS[i + 1, j - 1:j + 1].any() or (NMS[i, [j - 1, j + 1]] < TH).any()):
DT[i, j] = 1
return DT
main
import cv2
import numpy as np
import os
# 生成高斯核
def gaussian_kernel_2d(size, sigma_x, sigma_y=None):
if sigma_y is None:
sigma_y = sigma_x
x, y = np.meshgrid(np.linspace(-(size//2), size//2, size),
np.linspace(-(size//2), size//2, size))
kernel_2d = (1 / (2 * np.pi * sigma_x * sigma_y)) * np.exp(-(x ** 2 / (2 * sigma_x ** 2) + y ** 2 / (2 * sigma_y ** 2)))
# 归一化核
kernel_2d /= kernel_2d.sum()
return kernel_2d
if __name__ == '__main__':
list_path=""
list_data=os.listdir(list_path)
gauss_kernel = gaussian_kernel_2d(3, 2)
x_kel = np.array([[-1, 0, 1], [-2, 0, 2], [-1, 0, 1]])
y_kel = np.array([[1, 2, 1], [0, 0, 0], [-1, -2, -1]])
for i in list_data:
im_path=list_path+"/"+i
im=cv2.imread(im_path,cv2.IMREAD_GRAYSCALE)
new_im=smooth(im, gauss_kernel)
cv2.imwrite("./{}_smooth.jpg".format(i),new_im)
x,y,M=gradients(new_im,x_kel,y_kel)
cv2.imwrite("./{}_x.jpg".format(i),x)
cv2.imwrite("./{}_y.jpg".format(i),y)
cv2.imwrite("./{}_M.jpg".format(i),M)
non_maxima=NMS(M,x,y)
result=double_threshold(non_maxima, 0.3, 0.8)
cv2.imwrite("./{}".format(i),result*255)
四、Seam Carving
个人比较喜欢这个,可以很方便的对图片的尺寸进行修改
https://karthikkaranth.me/blog/implementing-seam-carving-with-python/
流程
- smooth
- “energy” as gradient magnitude
- 在8邻域内,找到一条能量最小的缝,将其去除
公式3.2:
- 重复2-3 ,直到满足条件
代码实现
- smooth
由于算子用的是sobel,可以
def smooth(im, gauss_kernel):
W, H = im.shape
k_size = gauss_kernel.shape[0]
new_gray = np.zeros([W - k_size + 1, H - k_size + 1])
for i in range(W - k_size):
for j in range(H - k_size):
new_gray[i, j] = np.sum(im[i: i+k_size, j: j+k_size] * gauss_kernel)
return new_gray
- energy
这里加了padding = 1
def gradients(im, x_kernel, y_kernel,padding=1):
W, H = im.shape
if(x_kernel.shape[0] != y_kernel.shape[0]):
print("x_kernel.shape[0] != y_kernel.shape[0]")
exit(1)
k_size = x_kernel.shape[0]
out_row = W - k_size + 2*padding + 1
out_col = H - k_size + 2*padding + 1
padded_input = np.pad(im, padding, mode='constant')
dx = np.zeros([out_row, out_col])
dy = np.zeros([out_row, out_col])
energy_map = np.zeros([out_row, out_col])
for i in range(out_row):
for j in range(out_col):
dx[i, j] = np.sum(padded_input[i: i+k_size, j: j+k_size] * x_kernel)
dy[i, j] = np.sum(padded_input[i: i+k_size, j: j+k_size] * y_kernel)
energy_map[i, j] = np.sqrt(np.square(dx[i, j]) + np.square(dy[i, j]))
return energy_map
- 计算各个seam的能量
计算各个列的能量,M的最后一行为 公式3.2 的求和。
def calculate_seam(img):
energy_map = cv2.cvtColor(img, cv2.COLOR_BGR2GRAY)
energy_map = gradients(energy_map, np.array([[-1, 0, 1], [-2, 0, 2], [-1, 0, 1]])
, np.array([[1, 2, 1], [0, 0, 0], [-1, -2, -1]]))
rows, cols = energy_map.shape
M = energy_map.copy()
backtrack = np.zeros_like(M, dtype=np.int32)
for i in range(1, rows):
for j in range(0, cols):
if j == 0:
idx = np.argmin(M[i - 1, j:j + 2])
backtrack[i, j] = idx + j
min_energy = M[i - 1, idx + j]
else:
idx = np.argmin(M[i - 1, j - 1:j + 2])
backtrack[i, j] = idx + j - 1
min_energy = M[i - 1, idx + j - 1]
M[i, j] += min_energy
return M, backtrack
找到能量最小的一列,将其去除。
def carve_column(img):
rows, cols, _ = img.shape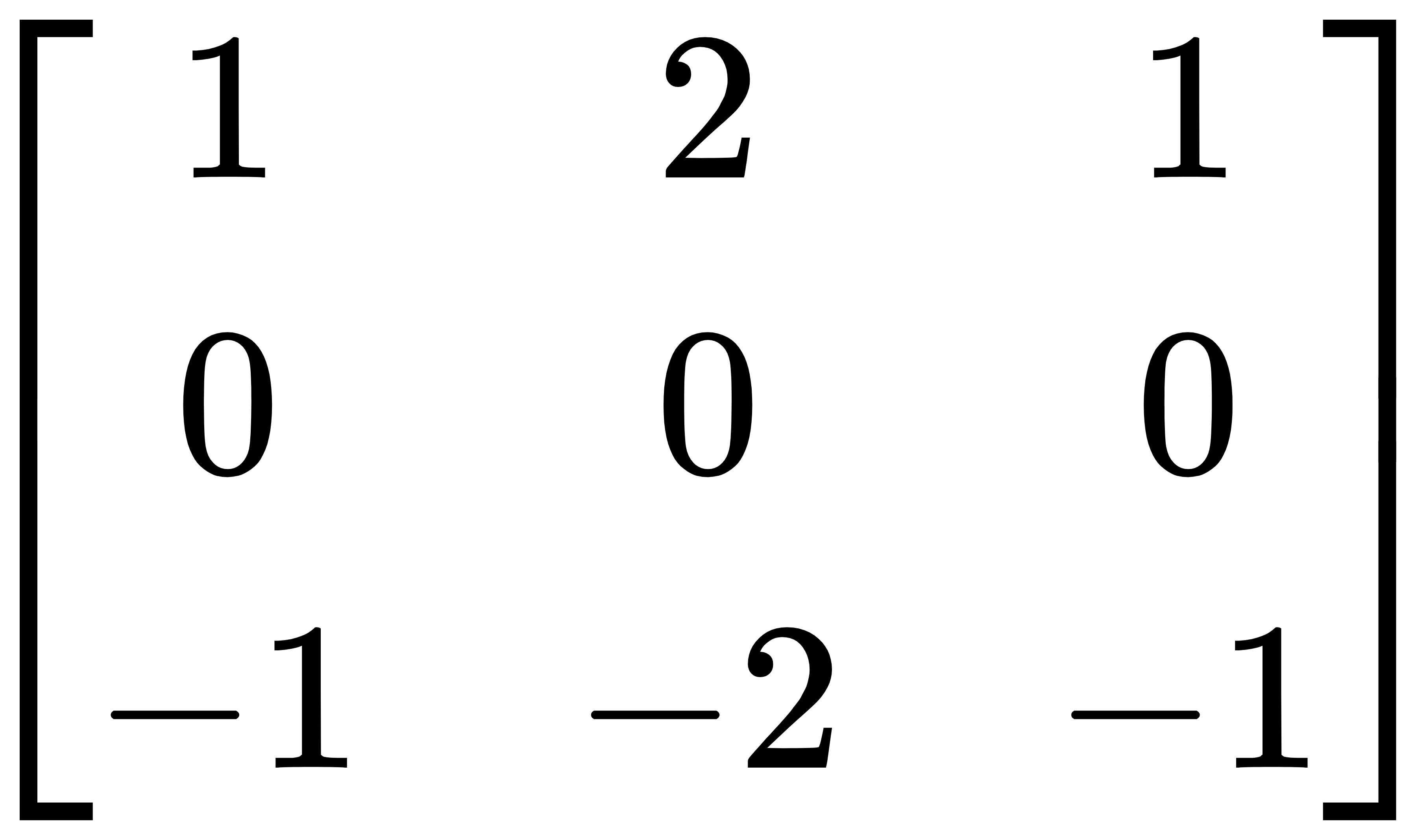
M, backtrack = calculate_seam(img)
mask = np.ones((rows, cols), dtype=np.bool_)
# mask = np.zeros_like(img) #需要imshow的话,可以用该mask,或者直接对原图像进行操作
j = np.argmin(M[-1])
for i in reversed(range(rows)):
mask[i, j] = False
# mask[i, j, 1] = 255
j = backtrack[i, j]
mask = np.stack([mask] * 3, axis=2)
img = img[mask].reshape((rows, cols - 1, 3))
return img
- 以上步骤,已经完成column的一列去除。
时间复杂度还是很高的,也能通过一次计算能量图后,将前几个低能量的列直接去除。