最近两个月在做学习图像分割方面的学习,踩了无数的坑,也学到了很多的东西,想了想还是趁着国庆节有时间来做个总结,以后有这方面需要可以来看看。
神经网络被大规模的应用到计算机视觉中的分类任务中,说到神经网络的分类任务这里不得不提到CNN(卷积神经网络), 在我的认识中,CNN的分类是对整个训练图像对应的标签进行分类,而图像分割网络Unet是对图像的各个像素进行分类,在图像分类时像素 “0” 一般都代表着背景,其他的像素代表你自己需要训练分割的类别,比如在你进行第一个类别图像标注时,你可以用像素 “1” 代表你的第一类,用像素 “2” 代表第二个类,以此类推。当然,你也可以用其他的任意的像素表示自己的类别或者背景,这不重要,只是用像素 “0”、“1”、“2”等代表你的背景像素或者类别比较方便,训练起来消耗的时间也比较用其他像素短。
接下来,我们开始分析网络结构以及Pytorch版本的图像分割Unet。
1、Unet网络结构
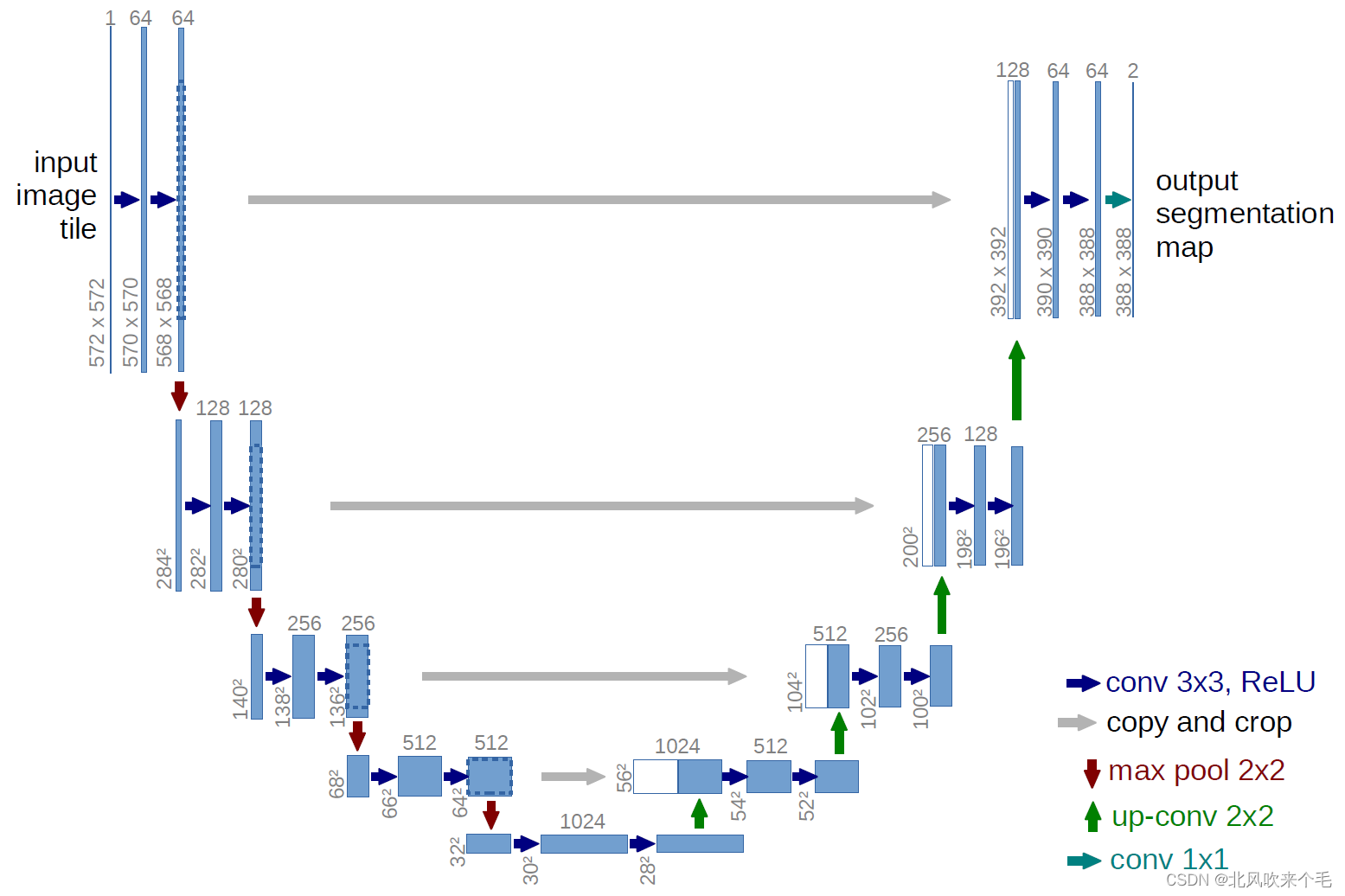
1.1 Unet网络结构
Unet网络可以分成两个结构:
(1)图像特征提取层:该层由卷积(Conv)、下采样(Pooling)构成,如图 1-1 左半部分,输入大小为 572x572x1(w,h,c) 的图像数据image到网络后先进行两次卷积得到C1(568x568x64),再进行下采样得到D1(284x284x64),继续对D1层进行进行两次卷积得到C2(280x280x128),对C2进行下采样得到D2(140x140x128),以此类推,后面分别计算出C3(136x136x256)、D3(68x68x256)、C4(64x64x512),D4(32x32x512)。至此,特征提取层结束。
(2)图像特征融合层:该层由卷积(Conv)、上采样(使用转置卷积或线性采样)、图像数据的拼接构成,首先一样的使用C4进行两次卷积得到C5(28x28x1024),再进行装置卷积或者线性采样得到U1(56x56x1024),此时再与C4进行拼接得到O1(56x56x1024),O1再进行两次卷积、上采样等操作,以此类推最后得到输出图像output(388x388x2)。至此整个Unet网络完成。
2、Pytorch版本代码实现
这里使用的是大佬的图像分割网络Unet进行学习的,bilibili链接:https://www.bilibili.com/video/BV11341127iK/?spm_id_from=333.999.0.0&vd_source=35b62865b997e4f1a87b1ab816f5296b
2.1 图像标注
这里使用开源图像标注工具labelme,命令行cmd使用命令 pip install labelme 进行安装,安装完成后在命令行中输入 labelme 打开工具进行标注。
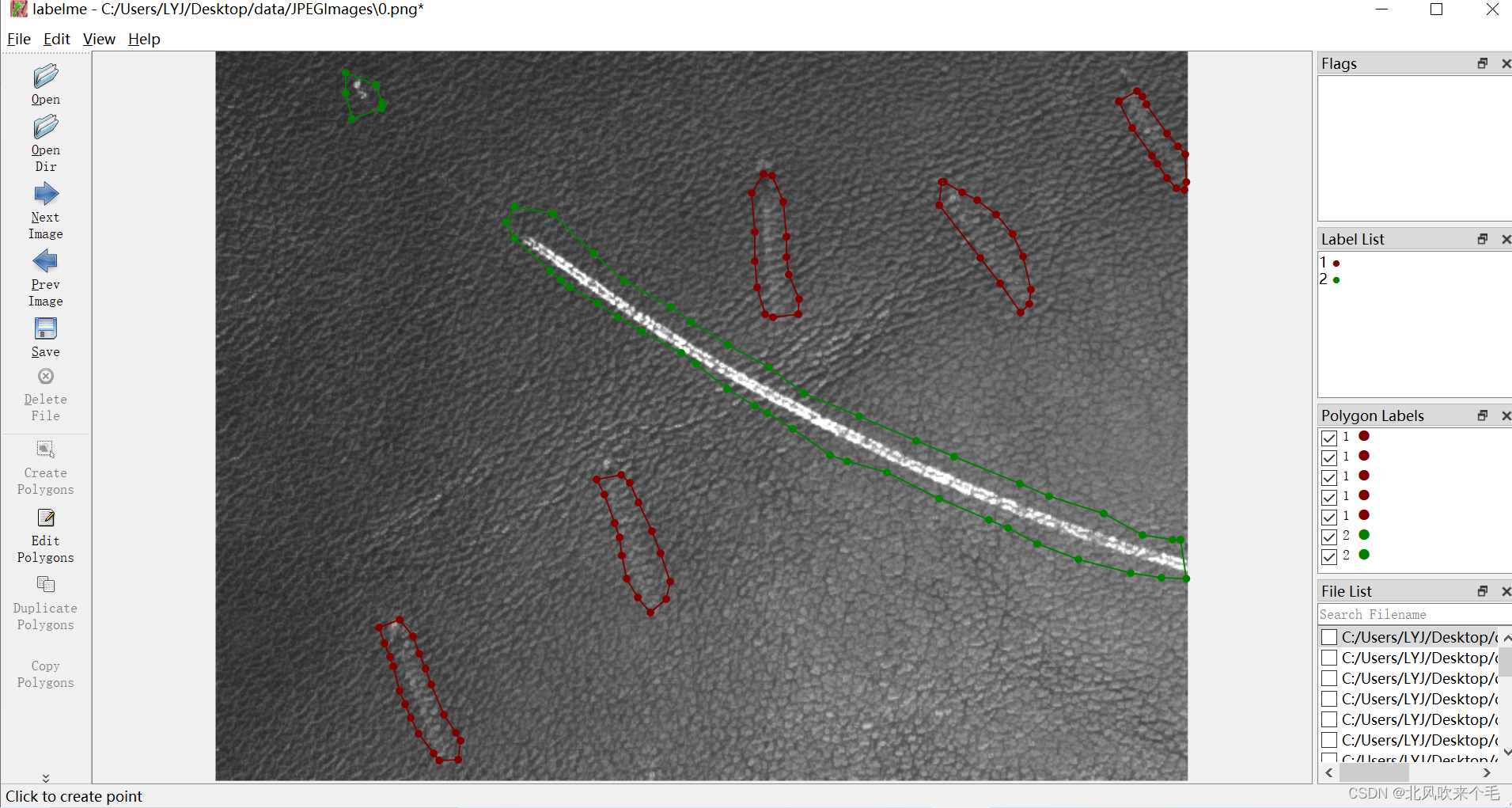
标注完成保存之后会生成 .json 文件,在该标签图像路径输入 labelme_json_to_dataset + 你 .json文件名就可以生成标签文件,如图2-2 至 图2-3显示,则成功标注该图像。其中img为标注原图、label为标签图像、label_names.txt文本文件里面是标注的类别以及背景类、label_viz为标注原图与标签图像融合之后得到的图像。
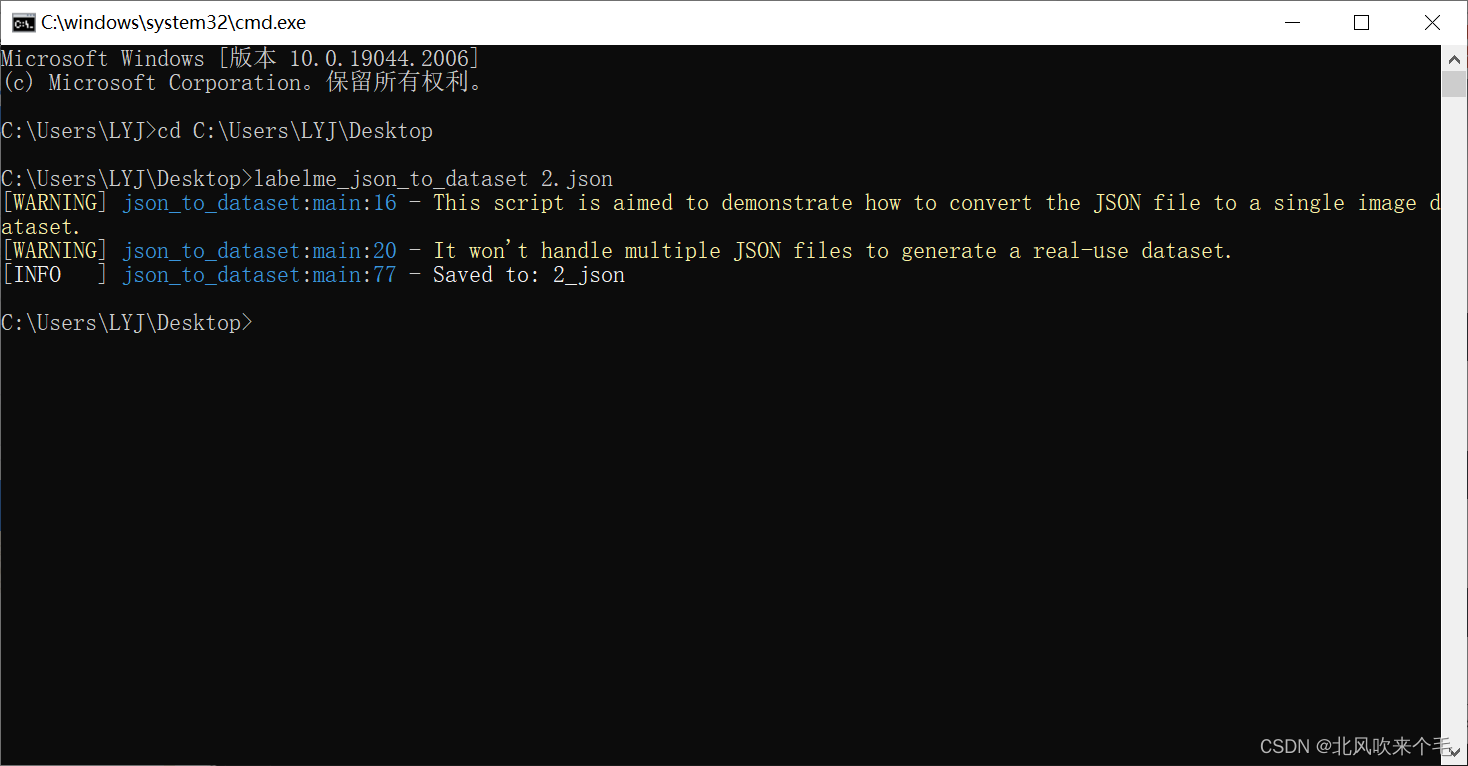
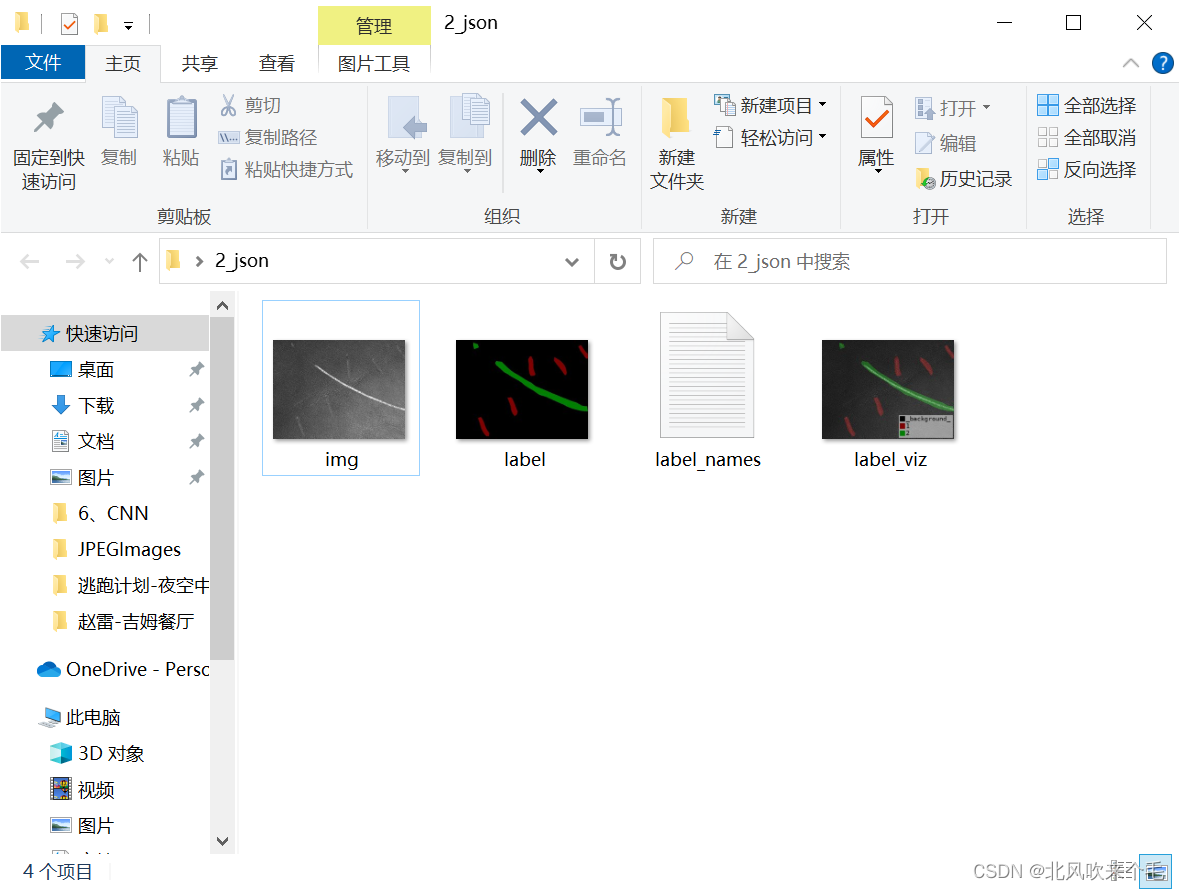
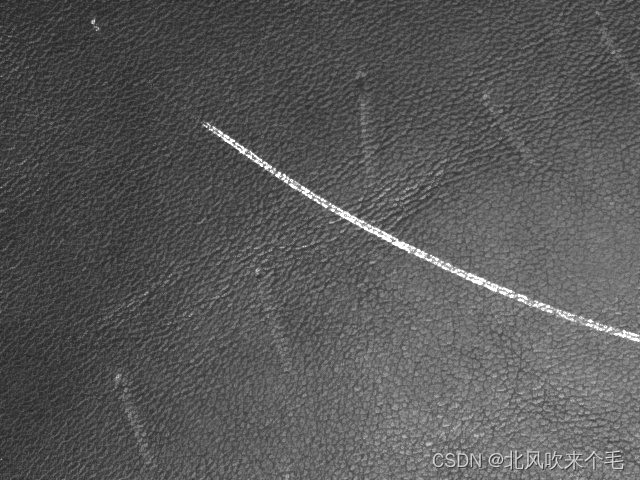
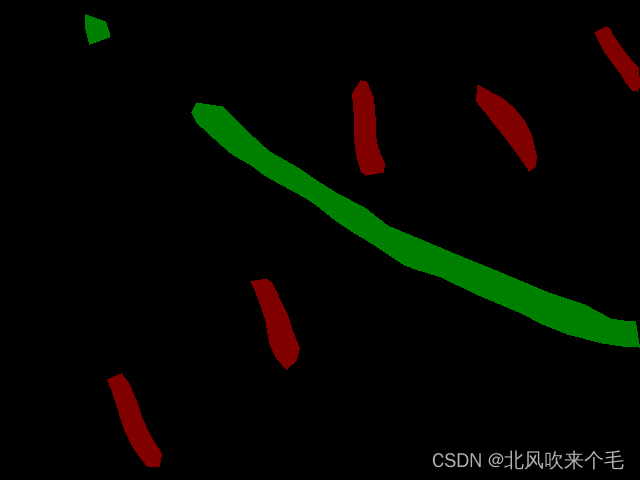
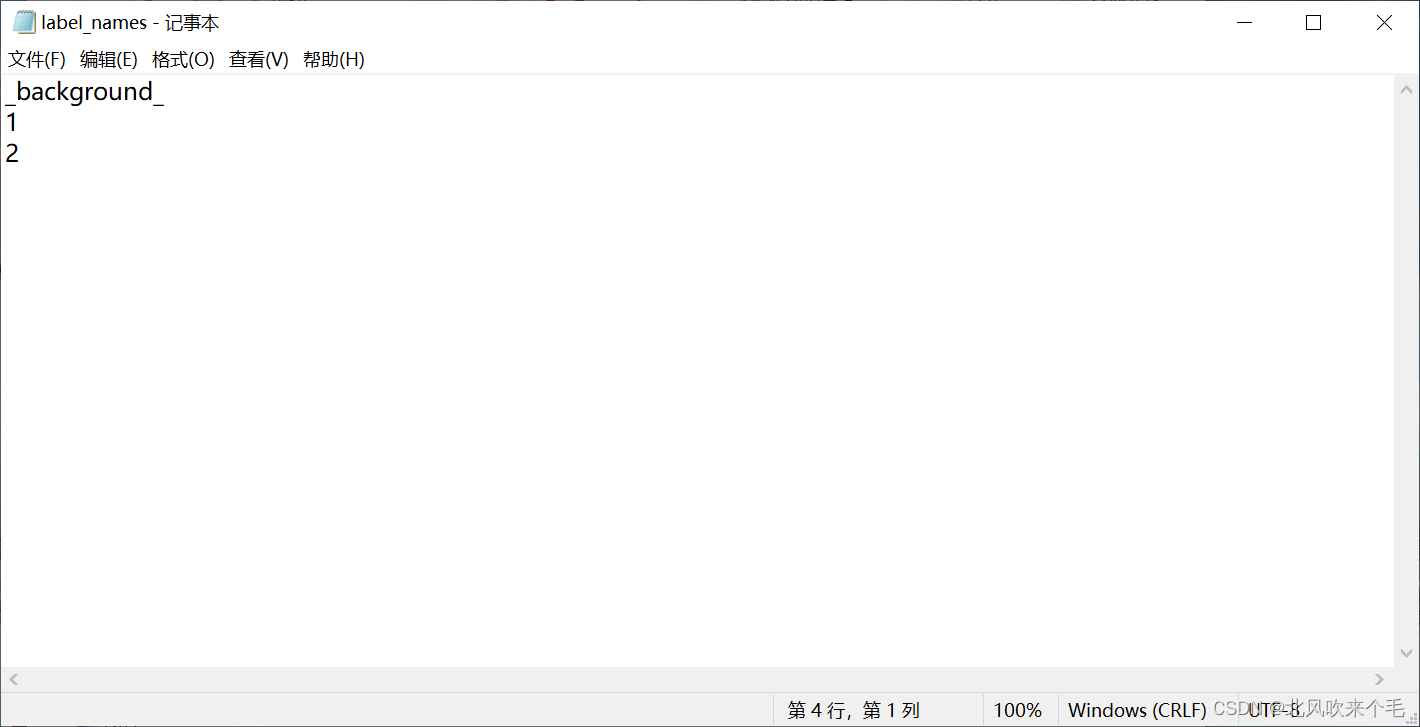
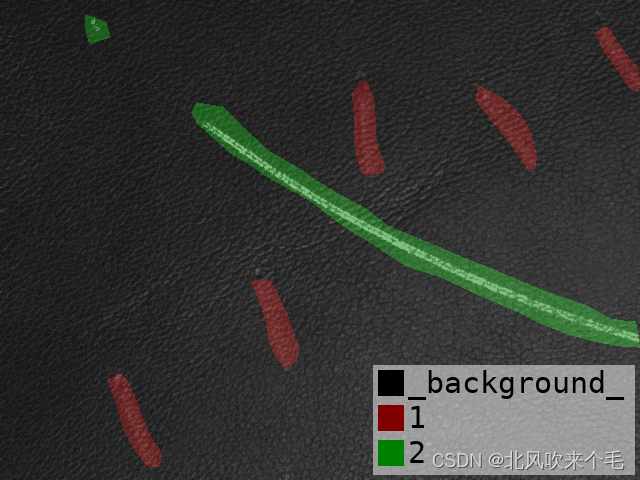
当标注的图像比较多时,使用labelme工具自带的解析器一个一个标签图像的生成会浪费很多时间,因此我自己写了一个代码来自动使用labelme的解析器,以下代码能够批量的生成标签图像。其中image路径为.json和标注原图的路径,JPEGImages为生成的训练图像路径,SegmentationClass为生成的标签图像路径。
json_to_dataset.py
from __future__ import print_function
import argparse
import glob
import math
import json
import os
import os.path as osp
import shutil
import numpy as np
import PIL.Image
import PIL.ImageDraw
import cv2
import time
def json_to_dataset(json_path, image_path, label_path):
if osp.isdir(label_path):
shutil.rmtree(label_path)
#print(label_path)
os.makedirs(label_path)
image_path_list = []
json_path_list = []
for file_path in os.listdir(json_path): # 0.png - 10.png
#print(file_path)
if file_path.endswith(".png"):
image_name = file_path.split(".")[0] # 0 - 10
json_name = os.path.join(json_path, image_name + ".json") # C:/Users/yiyaxin/Desktop/C++/pytorch-UNet-master/data/image\0.json
image_path_list.append(os.path.join(json_path , file_path)) # ['C:/Users/yiyaxin/Desktop/C++/pytorch-UNet-master/data/image\\0.png']
json_path_list.append(json_name) # ['C:/Users/yiyaxin/Desktop/C++/pytorch-UNet-master/data/image\\0.json']
# print(image_path_list)
# print(json_path_list)
for i in range(len(image_path_list)):
# 读取原图像
image = cv2.imread(image_path_list[i])
h, w = image.shape[:2]
# 生成与原图像大小的一样的标签图像
mask = np.zeros([h, w, 1], np.uint8)
# 打开json文件
with open(json_path_list[i], "r") as f:
label = json.load(f)
# 提取json文件中的 shapes
label = label["shapes"]
for label in label:
category = label["label"] # 标签
points = label["points"] # 标记的点
#print(category, points_array)
points_array = np.array(points, dtype=np.int32)
# 填充
mask = cv2.fillPoly(mask, [points_array], category_types.index(category))
# 保存原图像至 JPEGImages
cv2.imwrite(os.path.join(image_path, image_path_list[i].split("\\")[-1]), image)
# 保存标签图像至 SegmentationClass
cv2.imwrite(os.path.join(label_path, image_path_list[i].split("\\")[-1]), mask)
print("Pictures has been saved!")
if __name__=='__main__':
json_path = "C:/Users/yiyaxin/Desktop/C++/pytorch-UNet-master/data/image"
image_path = "C:/Users/yiyaxin/Desktop/C++/pytorch-UNet-master/data/JPEGImages"
label_path = "C:/Users/yiyaxin/Desktop/C++/pytorch-UNet-master/data/SegmentationClass"
# 标签种类
category_types = ["Background", "1", "2"]
json_to_dataset(json_path, image_path, label_path)
至此,图像的标注完成。
2.2 图像预处理加载图像数据
这里大佬先找到图像的最长边,然后用黑色像素来填充另外一边形成的高和宽相等的图像来进行训练,生成大小长宽相等的图像之后再把图像大小重置为256x256进行训练,比如标注的图像大小为640x480,则找到图像的最长边640,另外一边长为480的边则用黑色像素填充为640,最后得到的标注图像大小为640x640,再把图像大小重置为256x256,标签图像同理。图像预处理代码如下:
utils.py
from PIL import Image
def keep_image_size_open(path, size=(256, 256)):
img = Image.open(path)
temp = max(img.size)
mask = Image.new('P', (temp, temp))
mask.paste(img, (0, 0))
;
mask = mask.resize(size)
#mask.save(path)
return mask
def keep_image_size_open_rgb(path, size=(256, 256)):
img = Image.open(path)
temp = max(img.size)
mask = Image.new('RGB', (temp, temp))
mask.paste(img, (0, 0))
mask = mask.resize(size)
#mask.save(path)
return mask
if __name__ == '__main__':
image_path = "C:/Users/yiyaxin/Desktop/C++/pytorch-UNet-master/data/JPEGImages/0.png"
label_path = "C:/Users/yiyaxin/Desktop/C++/pytorch-UNet-master/data/SegmentationClass/0.png"
image1 = keep_image_size_open_rgb(image_path)
print(image1.mode)
print(image1.size)
image1.show('test1')
image2 = keep_image_size_open(label_path)
print(image2.mode)
print(image2.size)
#image2.save('C:/Users/yiyaxin/Desktop/C++/pytorch-UNet-master/data/JPEGImages/PASS2022_04_29_11_16_49_924.jpg')
image2.show('test2')
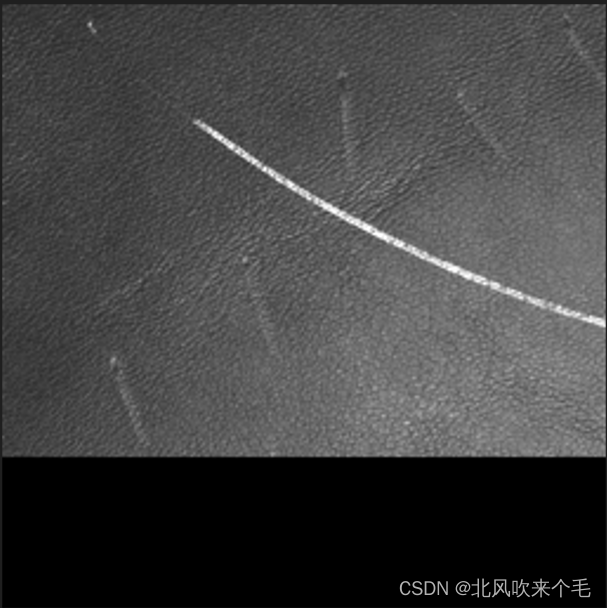
若是训练的图像是类似与下图中图像,背景占比较大,而我们需要分类的像素是图像的某个特征点,则我们可以使用opencv的查找轮廓函数进行图像的特征提取,如不进行提取的话,背景多余的干扰影响会很大,导致要训练更多的次数才能把图像中的类别给分割出来,并且效果很一般,这时可以使用opencv中的特征查找、特征提取函数进行提取特征。提取图像特征代码如下:
image_corp.py
import cv2
from PIL import Image
import os
def get_picture_path(file_path):
image_path_list = []
for i in os.listdir(file_path):
image_path = i.split(".")
if image_path[-1] != "json":
image_crop(os.path.join(file_path, i), i)
print(image_path_list)
def image_crop(image_path, image_name):
img = Image.open(image_path)
image = cv2.imread(image_path)
image_copy = image.copy()
image = cv2.cvtColor(image, cv2.COLOR_BGR2GRAY)
ret, thresh = cv2.threshold(image, 200, 255,cv2.THRESH_BINARY)
contours,hierarchy=cv2.findContours(thresh,cv2.RETR_TREE,cv2.CHAIN_APPROX_SIMPLE)
for i in range(len(contours)):
area = cv2.contourArea(contours[i])
#print(area)
if 111208.0 <area< 1308417.0:
print(area)
#image_copy=cv2.drawContours(image_copy,contours[i],-1,(0,255,0),2) # img为三通道才能显示轮廓 cv2.FILLED
x, y, w, h = cv2.boundingRect(contours[i])
cv2.rectangle(image_copy, (x-20,y-20), (x+w+20,y+h+20), (255,0,0), 2)
img = img.crop((x, y, x+w, y+h))
else:
continue
img.save(os.path.join("C:/Users/yiyaxin/Desktop/C++/pytorch-UNet-master/data/image", image_name))
#cv2.imwrite("C:/Users/yiyaxin/Desktop/PASS2022_04_29_11_08_20_749_test.jpg", image_copy)
#cv2.imshow("thresh", image_copy)
if __name__ =='__main__':
file_path = 'C:/Users/yiyaxin/Desktop/bls/20220513/8X2.5-BIAOMIAN-ng'
get_picture_path(file_path)
cv2.waitKey(0)
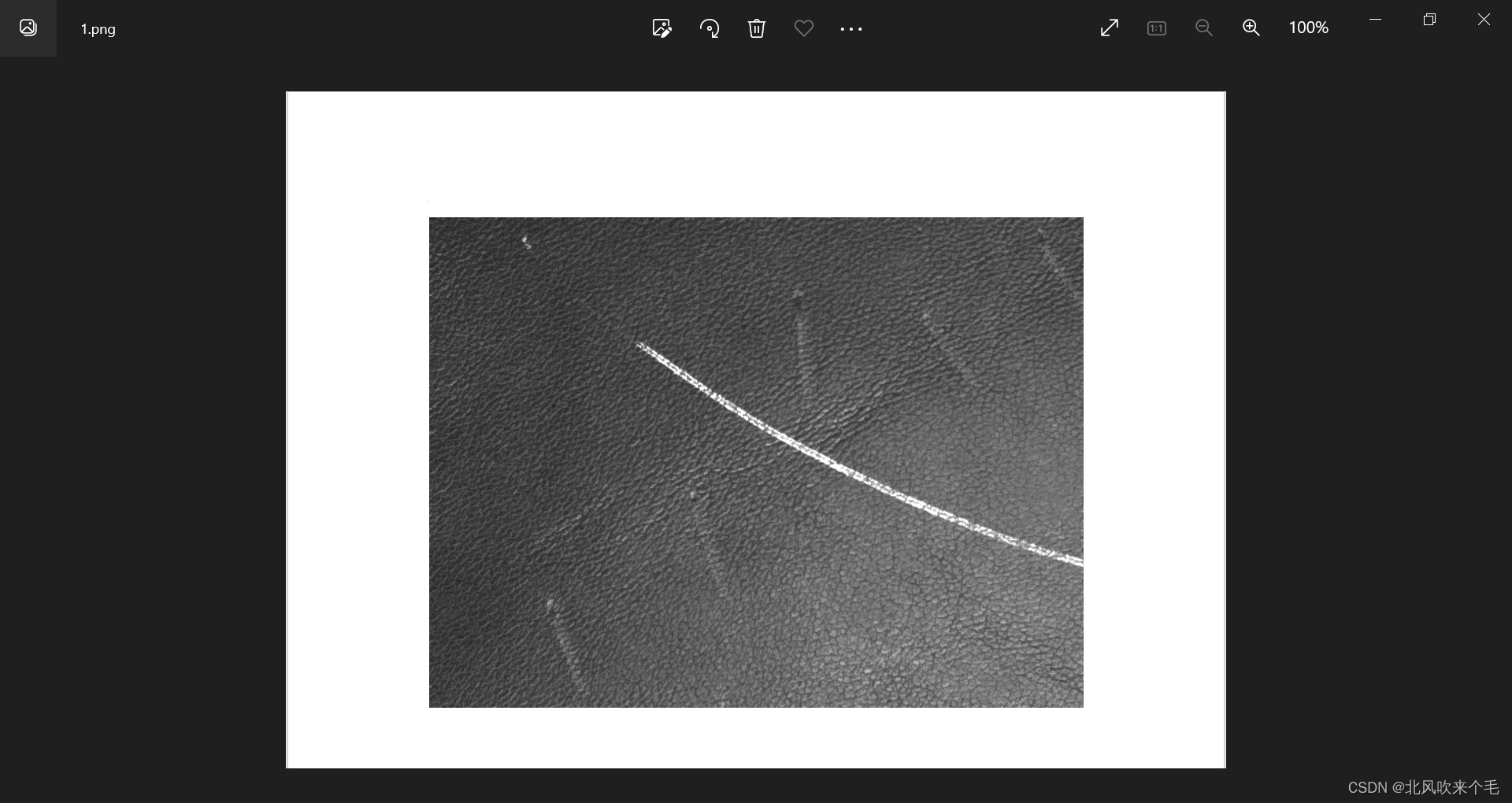
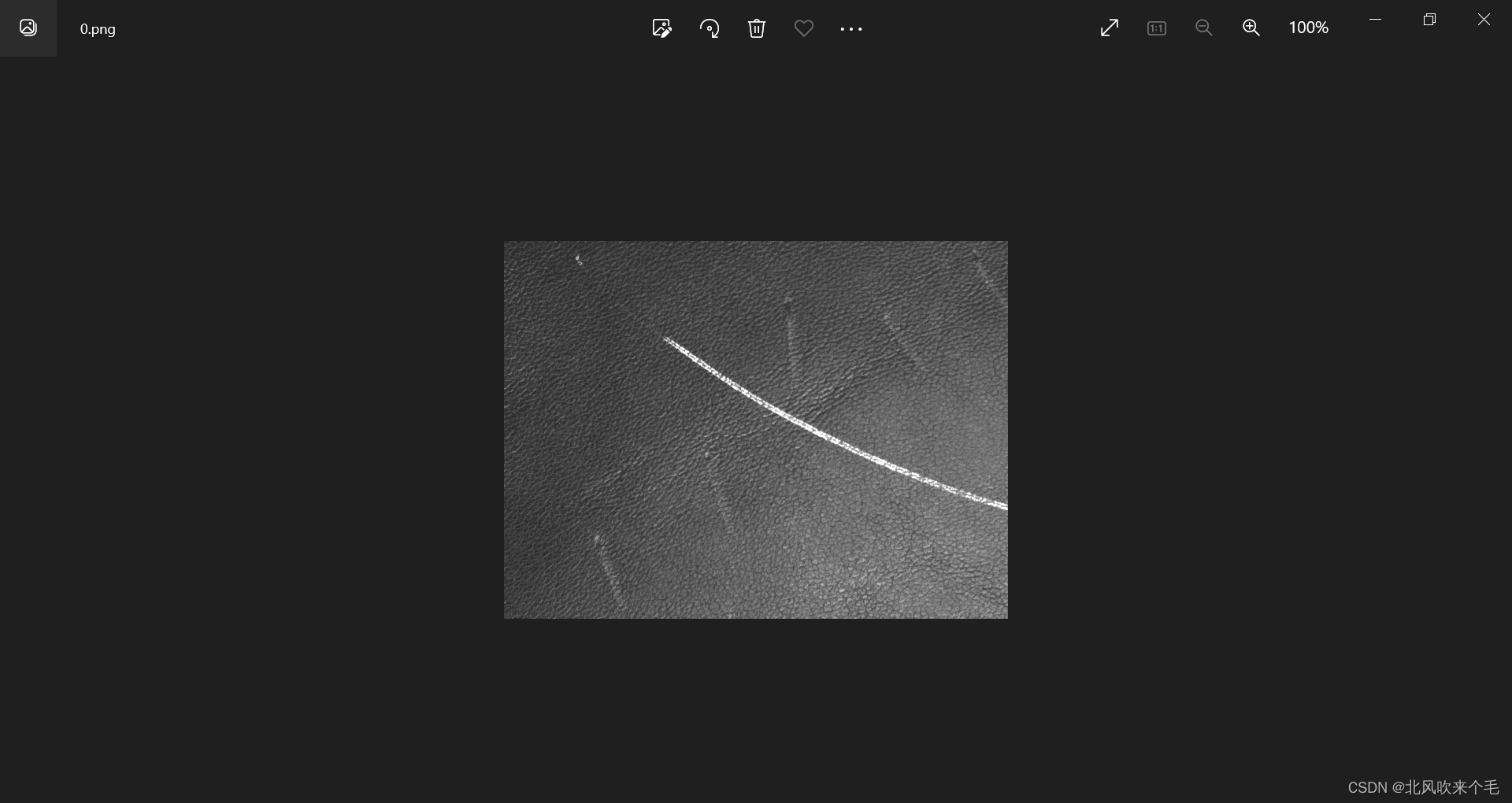
众所周知,在Pytorch中加载自己的训练图像时重写Dataset中类中的初始化函数(init)、长度函数(len)和加载图像函数(getitem),在大佬的代码里,初始化函数是找到图像的路径,长度函数则是返回图像数据的数量,getitem函数里面则是先把图像处理成长宽相等的图像,再重置大小为256x256。接着再使用pytorch中的transforms把图像数据和标签数据转换成向量的形式,传入网络训练。加载图像代码如下:
data.py
import os
import numpy as np
import torch
from torch.utils.data import Dataset
from utils import *
from torchvision import transforms
transform = transforms.Compose([
transforms.ToTensor()
])
class MyDataset(Dataset):
def __init__(self, path):
self.path = path
self.name = os.listdir(os.path.join(path, 'SegmentationClass'))
def __len__(self):
#print("len(name)", len(self.name))
return len(self.name)
def __getitem__(self, index):
segment_name = self.name[index] # xx.png
segment_path = os.path.join(self.path, 'SegmentationClass', segment_name)
image_path = os.path.join(self.path, 'JPEGImages', segment_name)
#print("segment_name: ", segment_name)
#print("image_path: ", image_path)
segment_image = keep_image_size_open(segment_path)
image = keep_image_size_open_rgb(image_path)
# print(image.size)
segment_image = np.array(segment_image)
# print(image.shape[1])
for i in range(segment_image.shape[1]):
print(np.array(segment_image[i]))
# for j in range(segment_image.shape[0]):
# print(np.array(segment_image[i][j]))
return transform(image), torch.Tensor(np.array(segment_image))
if __name__ == '__main__':
#from torch.nn.functional import one_hot
data = MyDataset('C:/Users/yiyaxin/Desktop/C++/pytorch-UNet-master/data')
print("image: ", data[0][0].shape)
print("label:", data[0][1].shape)
#out=one_hot(data[0][1].long())
#print("one_hot:", out.shape)
2.3 Unet网络搭建
在这一步中,分别构建卷积层类、下采样类、上采样类、Unet网络类。其中卷积层包括两次卷积函数,使用Pytorch中的BatchNorm2d函数进行数据归一化、Dropout2d函数进行数据的随机丢弃,目的是防止数据过大而产生过拟合,使用的激活函数为LeakyRelu()函数。下采样使用卷积函数Conv2d和BatchNorm2d及LeakyRelu()函数,上采样使用卷积函数Conv2d使图像通道变为原来的一半,接着使用转置卷积函数ConvTranspose2d或者使用线性采样函数interpolate进行上采样,最后再使用cat函数进行图像的拼接。接着再按照论文中的Unet网络结构进行搭建Unet类,这里可以用不用sigmoid或softmax等激活都无所谓,其中num_classes为图像预测的类别,完成网络的搭建后可以测试以下,比如输入(1,3,256,256)大小的图像数据,若经过网络计算后输出的图像数据依然是(1,3,256,256)大小的图像数据,则搭建的网络没有问题。
至此,Unet网络的搭建完成。搭建Unet网络代码如下:
net.py
import torch
from torch import nn
from torch.nn import functional as F
class Conv_Block(nn.Module):
def __init__(self,in_channel,out_channel):
super(Conv_Block, self).__init__()
self.layer=nn.Sequential(
nn.Conv2d(in_channel,out_channel,3,1,1,padding_mode='reflect',bias=False),
nn.BatchNorm2d(out_channel),
nn.Dropout2d(0.3),
nn.LeakyReLU(),
nn.Conv2d(out_channel, out_channel, 3, 1, 1, padding_mode='reflect', bias=False),
nn.BatchNorm2d(out_channel),
nn.Dropout2d(0.3),
nn.LeakyReLU()
)
def forward(self,x):
return self.layer(x)
class DownSample(nn.Module):
def __init__(self,channel):
super(DownSample, self).__init__()
self.layer=nn.Sequential(
nn.Conv2d(channel,channel,3,2,1,padding_mode='reflect',bias=False),
nn.BatchNorm2d(channel),
nn.LeakyReLU()
)
def forward(self,x):
return self.layer(x)
class UpSample(nn.Module):
def __init__(self,channel):
super(UpSample, self).__init__()
self.layer=nn.Conv2d(channel,channel//2,1,1)
self.up =torch.nn.ConvTranspose2d(channel,channel,2,2)
def forward(self,x,feature_map):
out=self.layer(self.up(x))
return torch.cat((out,feature_map),dim=1)
class UNet(nn.Module):
def __init__(self,num_classes):
super(UNet, self).__init__()
self.c1=Conv_Block(3,64)
self.d1=DownSample(64)
self.c2=Conv_Block(64,128)
self.d2=DownSample(128)
self.c3=Conv_Block(128,256)
self.d3=DownSample(256)
self.c4=Conv_Block(256,512)
self.d4=DownSample(512)
self.c5=Conv_Block(512,1024)
self.u1=UpSample(1024)
self.c6=Conv_Block(1024,512)
self.u2 = UpSample(512)
self.c7 = Conv_Block(512, 256)
self.u3 = UpSample(256)
self.c8 = Conv_Block(256, 128)
self.u4 = UpSample(128)
self.c9 = Conv_Block(128, 64)
self.out=nn.Conv2d(64,num_classes,3,1,1)
print("num_classes: ", num_classes)
def forward(self,x):
R1=self.c1(x)
#print(R1.size())
R2=self.c2(self.d1(R1))
R3 = self.c3(self.d2(R2))
R4 = self.c4(self.d3(R3))
R5 = self.c5(self.d4(R4))
#print(R5.size())
O1=self.c6(self.u1(R5,R4))
O2 = self.c7(self.u2(O1, R3))
O3 = self.c8(self.u3(O2, R2))
O4 = self.c9(self.u4(O3, R1))
return self.out(O4)
#return F.log_softmax(self.out(O4),dim=1)
if __name__ == '__main__':
x=torch.randn(1,3,256,256)
net=UNet(5)
print("shape: ", net(x).shape)
2.4 图像训练与预测
搭建好Unet网络之后就可以开始训练了,这里最需要注意的是背景也为一类,也就是说如果你的标注图像标注的是2个类别,那么进行训练时的类别是3类,传入的num_classes参数应该为3,首先把图像加载到网络里进行训练,再进行反向传播就可以了,这里使用的优化器是自适应Adam,使用的损失函数为多分类损失函数交叉熵损失函数CrossEntropyloss函数,如果只想进行二分类也可以只用BCE损失函数,不过网络之后的激活函数要换成sigmoid激活函数。训练函数代码如下:
train.py
import os
import tqdm
from torch import nn, optim
import torch
from torch.utils.data import DataLoader#数据集加载器
from data import *
from net import *
from torchvision.utils import save_image
import os
os.environ['CUDA_DEVICE_ORDER'] = 'PCI_BUS_ID'
os.environ['CUDA_LAUNCH_BLOCKING'] = '1'
device = torch.device('cuda')
weight_path = 'C:/Users/yiyaxin/Desktop/C++/pytorch-UNet-master/params/unet.pth'#权重地址
data_path = r'C:/Users/yiyaxin/Desktop/C++/pytorch-UNet-master/data'#数据集地址
save_path = 'C:/Users/yiyaxin/Desktop/C++/pytorch-UNet-master/train_image'#训练时保存的图像地址
if __name__ == '__main__':
num_classes = 2+ 1 # +1是背景也为一类
data_loader = DataLoader(MyDataset(data_path), batch_size=2, shuffle=True)#加载数据集,batch_size批次,根据自身电脑的情况进行修改
net = UNet(num_classes).to(device)#实例化Unet网路
if os.path.exists(weight_path):#判断权重是否存在
net.load_state_dict(torch.load(weight_path))
print('successful load weight!')
else:
print('not successful load weight')
opt = optim.Adam(net.parameters())
#opt = optim.SGD(net.parameters(), lr=0.01, momentum=0.9)
loss_fun = nn.CrossEntropyLoss() # nn.BCELoss()
epoch = 1
while epoch < 100:
for i, (image, segment_image) in enumerate(tqdm.tqdm(data_loader)):
#print("标签种类:", segment_image.max(), segment_image.min())
# print(image.size)
# print(segment_image.size)
image, segment_image = image.to(device), segment_image.to(device)
if hasattr(torch.cuda, 'empty_cache'):
torch.cuda.empty_cache()
out_image = net(image)
train_loss = loss_fun(out_image, segment_image.long())
#print("train_loss:", train_loss)
opt.zero_grad()
try:
train_loss.backward()
except RuntimeError as e:
print("异常:", e)
opt.step()
if i % 5 == 0:
print(f'\t{epoch}-{i}-train_loss===>>{train_loss.item()}')
_image = image[0]
_segment_image = torch.unsqueeze(segment_image[0], 0) * 255
_out_image = torch.argmax(out_image[0], dim=0).unsqueeze(0) * 255
img = torch.stack([_segment_image, _out_image], dim=0)
save_image(img, f'{save_path}/{i}.png')
if epoch % 20 == 0:#每20次保存一次权重
torch.save(net.state_dict(), weight_path)
#torch.save(net, weight_path)
print('save successfully!')
epoch += 1
测试代码就是使用图像预处理时的函数加载图像到训练好的网络里进行分类,由于网络输出的是(1,3,256,256)大小的图像数据,所有只需要把这个图像数据进行降维成(1,256,256)大小的图像数据,接着把图像数据的像素转换成没有重复数据的矩阵就可以知道它预测出来的类别了,想要使用opencv查看的话需要把图像数据转换成(256,256,1)的形式进行保存或者显示,预测分类图像的代码如下:
test.py
import os
import cv2
import numpy as np
import torch
from net import *
from utils import *
from data import *
from torchvision.utils import save_image
from PIL import Image
os.environ['CUDA_DEVICE_ORDER'] = 'PCI_BUS_ID'
os.environ['CUDA_LAUNCH_BLOCKING'] = '1'
net=UNet(3).cuda()
weights='C:/Users/yiyaxin/Desktop/C++/pytorch-UNet-master/params/unet.pth'
if os.path.exists(weights):
net.load_state_dict(torch.load(weights))
#net.load(weights)
print('successfully')
else:
print('no loading')
#_input='C:/Users/yiyaxin/Desktop/C++/pytorch-UNet-master/test/PASS2022_04_29_11_16_49_924.jpg'
_input = 'C:/Users/yiyaxin/Desktop/C++/pytorch-UNet-master/test/0.jpg'
img=keep_image_size_open_rgb(_input)
#img = Image.open(_input)
#img = img.convert("RGB")
img_data=transform(img).cuda() # (3, 256, 256)
img_data=torch.unsqueeze(img_data,dim=0) # (1, 3, 256, 256)
print("img_data.size: ", img_data.shape)
net.eval()
out=net(img_data) # 网络输出 (1, 2, 256, 256)
out=torch.argmax(out,dim=1) # (1, 256, 256)
out=torch.squeeze(out,dim=0) # (256, 256)
out=out.unsqueeze(dim=0) # (1, 256, 256)
print(set((out).reshape(-1).tolist()))
out=(out).permute((1,2,0)).cpu().detach().numpy() # (256, 256, 1)[
cv2.imwrite('C:/Users/yiyaxin/Desktop/C++/pytorch-UNet-master/result/result.png',out)
cv2.imshow('out',out*255.0)
cv2.waitKey(0)
上面的代码显示的只是像素为0或255(黑或白)的图像,若想看的它的类别的话可以使用以下的代码进行显示:
label_path = 'C:/Users/yiyaxin/Desktop/C++/pytorch-UNet-master/result/ret.jpg'
#label_path = 'C:/Users/yiyaxin/Desktop/C++/pytorch-UNet-master/data/SegmentationClass'
label = np.asarray(Image.open(label_path), dtype=np.float32)
np.save("test.npy",label)
img3 = np.load("test.npy")
print("img3.shape", img3.shape)
print(set((img3).reshape(-1).tolist()))
plt.imshow(img3)
plt.show()
若是想使用opencv中的查找轮廓函数显示分割结果,可以使用下面的代码进行显示:
image1 = cv2.imread('C:/Users/yiyaxin/Desktop/C++/pytorch-UNet-master/result/ret.jpg')
print(set((image1).reshape(-1).tolist()))
image2 = cv2.imread('C:/Users/yiyaxin/Desktop/C++/pytorch-UNet-master/test/0.jpg')
#image2 = keep_image_size_open_rgb('C:/Users/yiyaxin/Desktop/C++/pytorch-UNet-master/test/PASS2022_04_29_11_08_46_933.png')
# image2 = cv2.cvtColor(np.array(image2), cv2.COLOR_RGB2BGR)
image2 = cv2.resize(image2, (256, 256))
image3 = image2.copy()
# print(image3.shape)
image1=cv2.cvtColor(image1,cv2.COLOR_BGR2GRAY)
# #print(image1.shape)
ret,thresh=cv2.threshold(image1,0,255,0)
#cv2.imshow('imageshow',thresh) # 显示返回值image,其实与输入参数的thresh原图没啥区别
contours,hierarchy=cv2.findContours(thresh,cv2.RETR_TREE,cv2.CHAIN_APPROX_SIMPLE)
#print(contours)
for i in range(len(contours)):
area = cv2.contourArea(contours[i])
#print(area)
if area < 10:
continue
print(area)
image1=cv2.drawContours(image2,contours[i],-1,(0,255,0),2) # img为三通道才能显示轮廓 cv2.FILLED
# # 核心拼接代码
image = np.concatenate([image3, image2], axis=1)
cv2.imshow('drawimg',image)
cv2.waitKey(0)
cv2.destroyAllWindows()
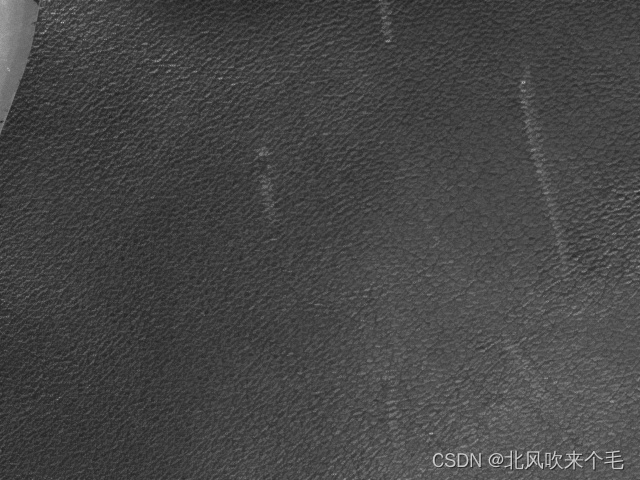
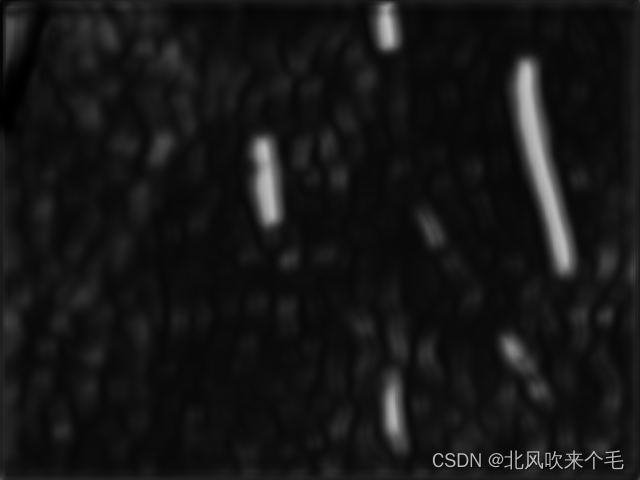
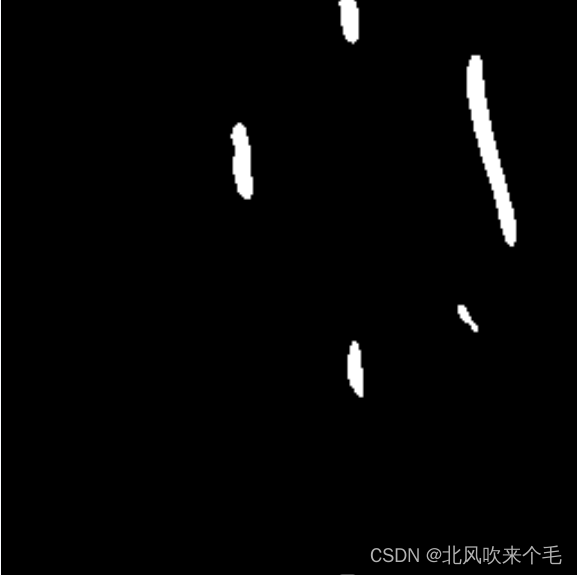
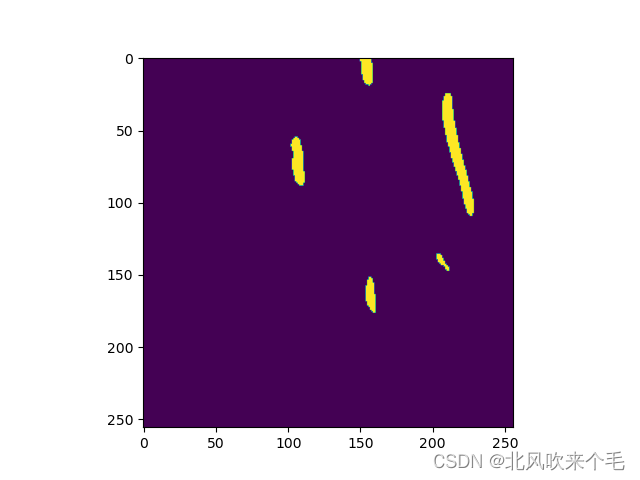
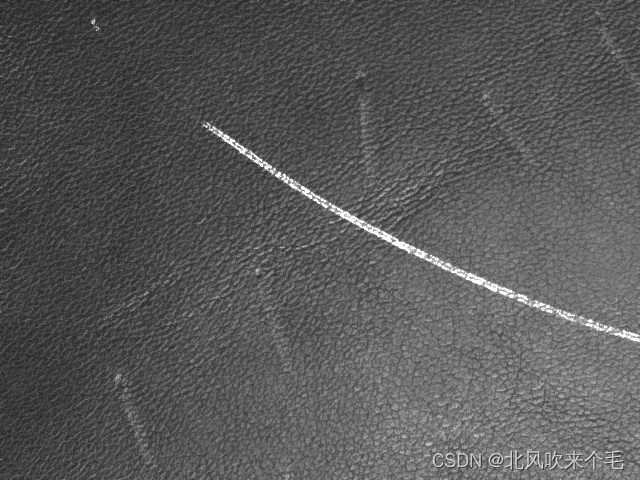
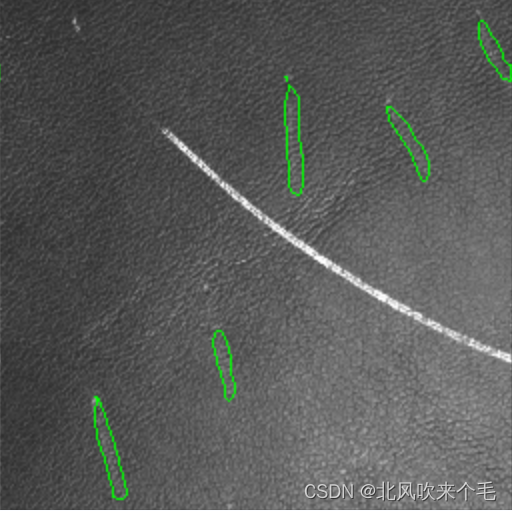
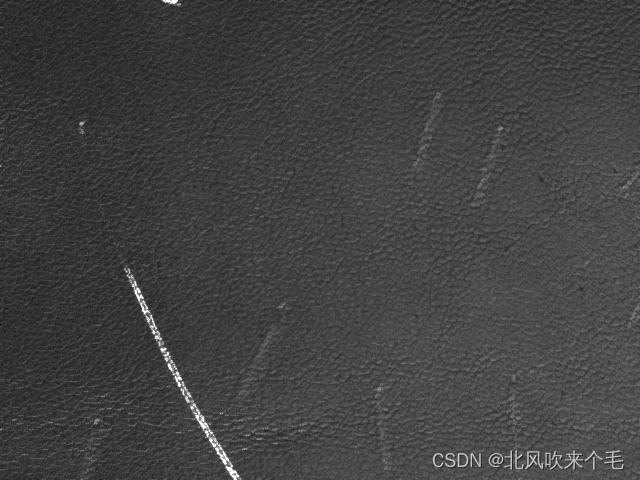
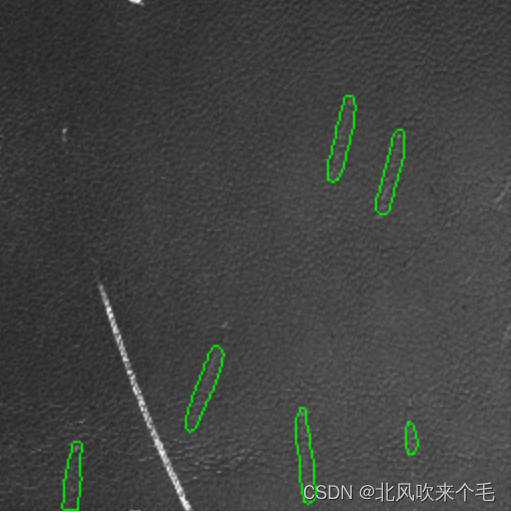
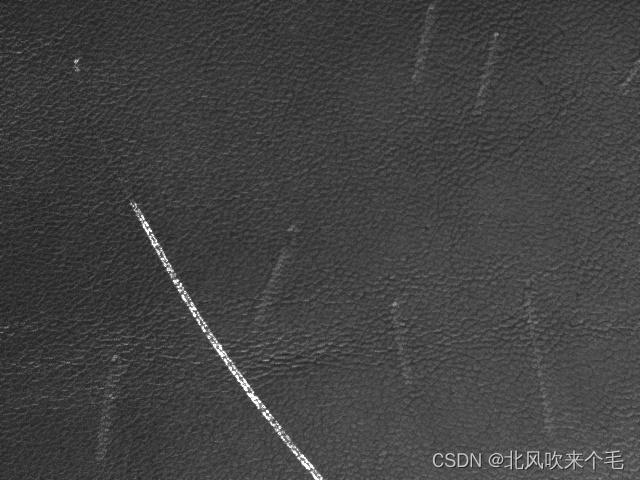
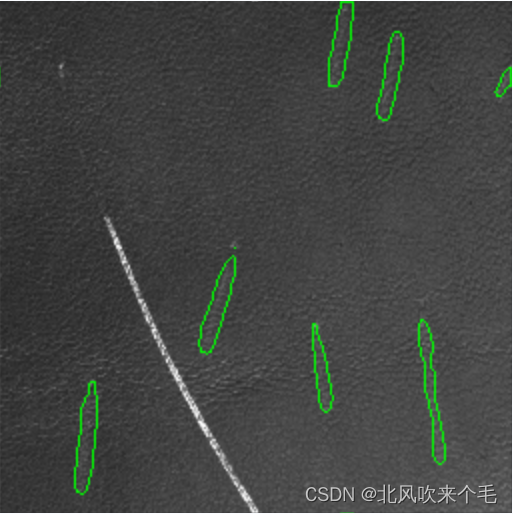
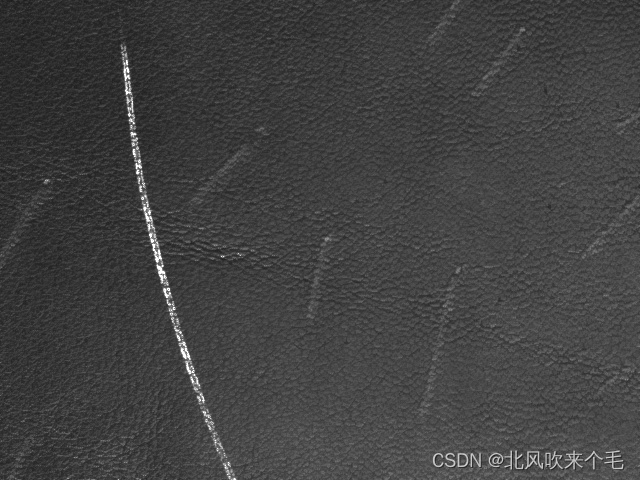
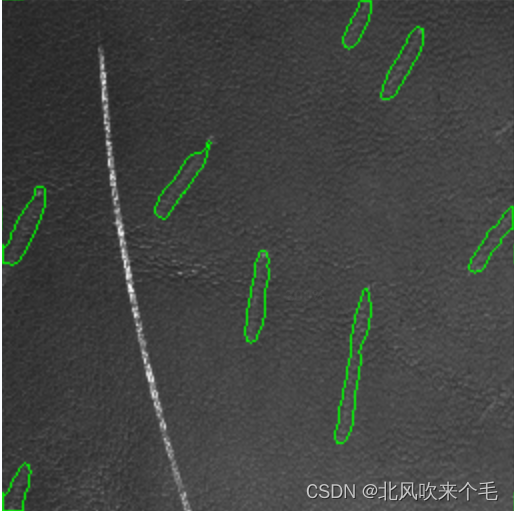
至此,Unet分割网络项目完成。
3、项目总结
这次的Unet分割网络主要可以分为四个步骤:
一、图像预处理:安装labelme工具进行标注,进行把图像预处理为等高的256x256x3的图像数据,重写Pytorch中Dataset中的加载图像数据函数。
二、搭建Unet网络:首先构建卷积类、下采样类、上采样类,其中num_classes为自己标注的类别加一,因为背景像素也是一个类别,如果只进行二分类,则使用的激活函数为sigmoid,损失函数为BCE损失函数,若进行多分类则可以使用激活函数为softmax,损失函数为交叉熵损失函数CrossEntorpyLoss函数。上采样可以使用转置卷积函数或者线性采样函数,在上采样的最后要进行图像数据的拼接。
三、训练和预测:按照官方标准的训练测试函数构建。
最后,分析一下这个网络的优缺点,在我学习中看来,Unet网络对于大图像特征的分割还是比较不错的,可以使用较少的训练图像和较少的训练次数就能够得到很好的分类结果,网络搭建起来也是比较简单的,特别是熟悉Pytorch的话搭建起来超级方便。最大的缺点我觉得是对于图像的特征提取不够好,这个或许是跟本身的网络结构有问题,由于它对图像的特征提取并没有那么好,因此在训练背景像素干扰比较大,图像也比较大,想要分类的图像比较细致的话结果并没有那么理想,对于这种图像需要训练的次数还是比较多的,而且分割出来的图像特征干扰还是比较多的。第二个就是对于类别特别多的图像有时候根本分割不出全部的图像类别,会损失掉一两个的图像特征,对于这点以我目前的知识还没想到是什么原因导致的。
下次我会给大家带来C++版的基于libtorch的Unet图像分割网络分析和代码,以及在实现过程中我所踩过的坑。
欢迎大家对此项目提出您最宝贵的建议,并在此处留言,指正我在文章内出现的错误或者与我交流您对于Unet分割网络的宝贵见解。