1 VGG网络总结
感觉就是再alex-net的基础上,研究了下如何加深网络来提高性能的。总体上也是五层卷积加上三层全链接,但是这五层卷积中都会以pooling来分割,且五层卷积尝试叠加多层卷积再一起,并且尝试以更小的核以及提高核的数量来提高网络的性能,比如alex-net的核的大小为11×11×96不等,vgg网络一般都是用3×3的核,但是她核的数量提高了很多,有3×3×256不等,来提高性能。即通过降低filter的大小,增加层数来达到同样的效果。
vgg的模型比alex-net大很多,训练出来的参数大概有500m,且训练时间长,幸好有训练好的参数可以使用,例如VGG-16,VGG-19等,这俩个效果还不错,且在网上可以下载使用。
2 VGG网络模型
总共探讨了一下几种类型,从A到E这几种类型,由上到下都是五层卷积加3层全链接。
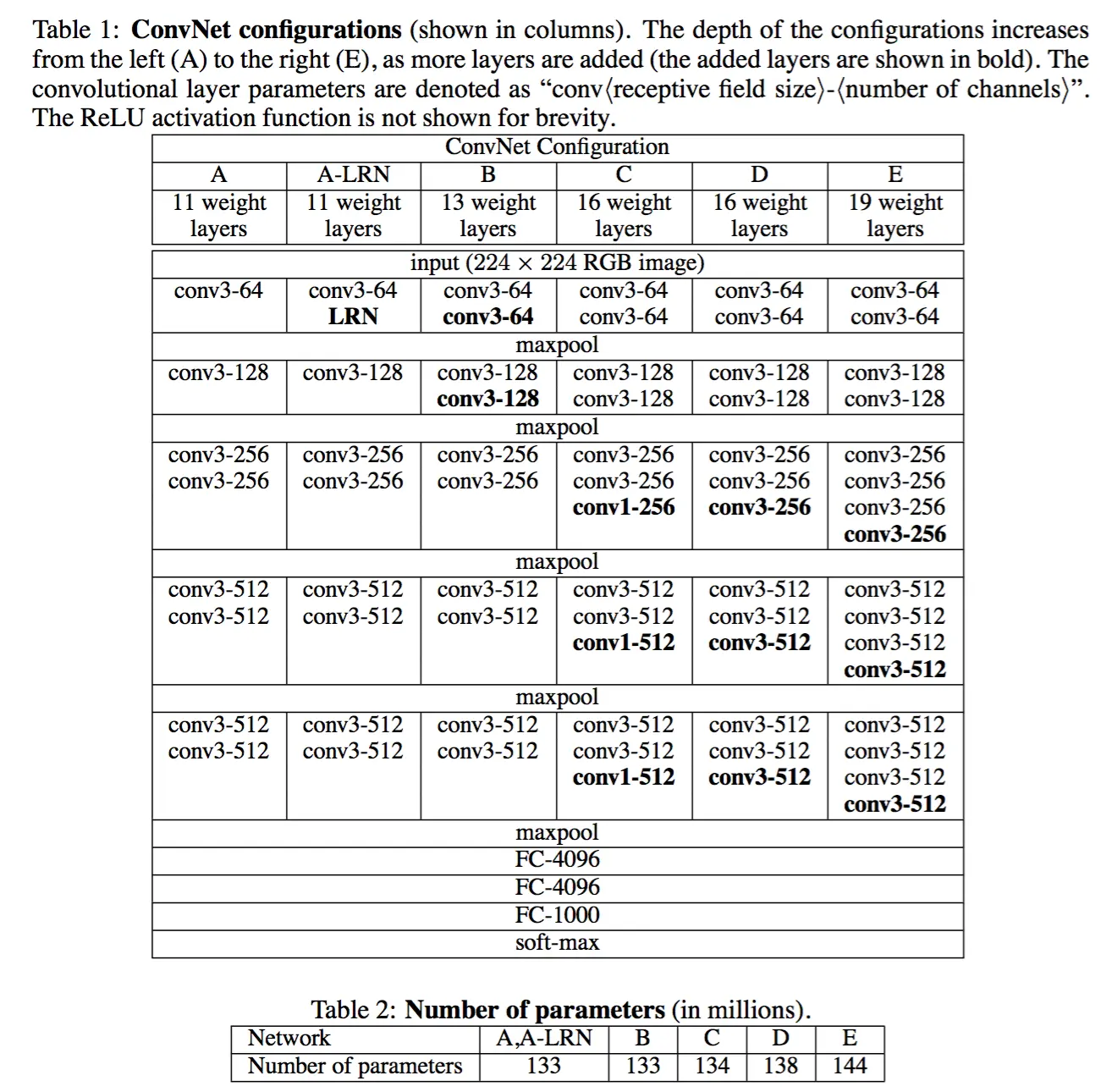
3 VGG网络的实现
来源:https://github.com/boyw165/tensorflow-vgg
这里你能训练自己的VGG模型,也能够加载已有的vgg模型来对图像进行分类,其中vgg19的模型的代码如下,写的很漂亮,把所有东西都放在这个类里面。
import numpy as np
import tensorflow as tf
_VGG_MEAN = [103.939, 116.779, 123.68]
class Vgg19:
"""
A VGG-19 Network implementation using TensorFlow library.
The network takes an image of size 224x224 with RGB channels and returns
category scores of size 1000.
The network configuration:
- RGB: 224x224x3
- BGR: 224x224x3
- conv1: 224x224x64
- conv2: 112x112x128
- conv3: 56x56x256
- conv4: 28x28x512
- conv5: 14x14x512
- fc6: 25088(=7x7x512)x4096
- fc7: 4096x4096
- fc8: 4096x1000
"""
WIDTH = 224
"The fixed width of the input image."
HEIGHT = 224
"The fixed height of the input image."
CHANNELS = 3
"The fixed channels number of the input image."
model = {}
"The model storing the kernels, weights and biases."
model_save_path = None
"The model save path, especially for the training process."
model_save_freq = 0
"""
The frequency to save the model in the training process. e.g. Save the
model every 1000 iteration.
"""
learning_rate = 0.05
"Learning rate for the gradient descent."
_inputRGB = None
_inputBGR = None
_inputNormalizedBGR = None
_conv1_1 = None
_conv1_2 = None
_pool1 = None
_conv2_1 = None
_conv2_2 = None
_pool2 = None
_conv3_1 = None
_conv3_2 = None
_conv3_3 = None
_conv3_4 = None
_pool3 = None
_conv4_1 = None
_conv4_2 = None
_conv4_3 = None
_conv4_4 = None
_pool4 = None
_conv5_1 = None
_conv5_2 = None
_conv5_3 = None
_conv5_4 = None
_pool5 = None
_fc6 = None
_relu6 = None
_fc7 = None
_relu7 = None
_fc8 = None
_preds = None
"The predictions tensor, shape of [?, 1000]"
_loss = None
_optimizer = None
_train_labels = None
"The train labels tensor, a placeholder."
def __init__(self,
model=None,
model_save_path=None,
model_save_freq=0):
"""
:param model: The model either for back-propagation or
:param model_save_path: The model path for training process.
:param model_save_freq: Save the model (in training process) every N
iterations.
forward-propagation.
"""
self.model = self._init_empty_model() if not model else model
self.model_save_path = model_save_path
self.model_save_freq = model_save_freq
self._train_labels = tf.placeholder(tf.float32,
[None, 1000])
self._inputRGB = tf.placeholder(tf.float32,
[None,
Vgg19.WIDTH,
Vgg19.HEIGHT,
Vgg19.CHANNELS])
red, green, blue = tf.split(3, 3, self._inputRGB)
self._inputBGR = tf.concat(3, [
blue,
green,
red,
])
self._inputNormalizedBGR = tf.concat(3, [
blue - _VGG_MEAN[0],
green - _VGG_MEAN[1],
red - _VGG_MEAN[2],
])
self._conv1_1 = self._conv_layer(self._inputNormalizedBGR, "conv1_1")
self._conv1_2 = self._conv_layer(self._conv1_1, "conv1_2")
self._pool1 = self._max_pool(self._conv1_2, 'pool1')
self._conv2_1 = self._conv_layer(self._pool1, "conv2_1")
self._conv2_2 = self._conv_layer(self._conv2_1, "conv2_2")
self._pool2 = self._max_pool(self._conv2_2, 'pool2')
self._conv3_1 = self._conv_layer(self._pool2, "conv3_1")
self._conv3_2 = self._conv_layer(self._conv3_1, "conv3_2")
self._conv3_3 = self._conv_layer(self._conv3_2, "conv3_3")
self._conv3_4 = self._conv_layer(self._conv3_3, "conv3_4")
self._pool3 = self._max_pool(self._conv3_4, 'pool3')
self._conv4_1 = self._conv_layer(self._pool3, "conv4_1")
self._conv4_2 = self._conv_layer(self._conv4_1, "conv4_2")
self._conv4_3 = self._conv_layer(self._conv4_2, "conv4_3")
self._conv4_4 = self._conv_layer(self._conv4_3, "conv4_4")
self._pool4 = self._max_pool(self._conv4_4, 'pool4')
self._conv5_1 = self._conv_layer(self._pool4, "conv5_1")
self._conv5_2 = self._conv_layer(self._conv5_1, "conv5_2")
self._conv5_3 = self._conv_layer(self._conv5_2, "conv5_3")
self._conv5_4 = self._conv_layer(self._conv5_3, "conv5_4")
self._pool5 = self._max_pool(self._conv5_4, 'pool5')
self._fc6 = self._fc_layer(self._pool5, "fc6")
self._relu6 = tf.nn.relu(self._fc6)
self._fc7 = self._fc_layer(self._relu6, "fc7")
self._relu7 = tf.nn.relu(self._fc7)
self._fc8 = self._fc_layer(self._relu7, "fc8")
self._preds = tf.nn.softmax(self._fc8, name="prediction")
self._loss = tf.nn.softmax_cross_entropy_with_logits(self._fc8,
self._train_labels)
self._optimizer = tf.train \
.GradientDescentOptimizer(self.learning_rate) \
.minimize(self._loss)
@property
def inputRGB(self):
"""
The input RGB images tensor of channels in RGB order.
Shape must be of [?, 224, 224, 3]
"""
return self._inputRGB
@property
def inputBGR(self):
"""
The input RGB images tensor of channels in BGR order.
Shape must be of [?, 224, 224, 3]
"""
return self._inputBGR
@property
def preds(self):
"""
The prediction(s) tensor, shape of [?, 1000].
"""
return self._preds
@property
def train_labels(self):
"""
The train labels tensor, shape of [?, 1000].
"""
return self._train_labels
@property
def loss(self):
"""
The loss tensor.
"""
return self._loss
@property
def optimizer(self):
"""
The optimizer tensor, used for the training.
"""
return self._optimizer
def _avg_pool(self, value, name):
return tf.nn.avg_pool(value, ksize=[1, 2, 2, 1], strides=[1, 2, 2, 1],
padding='SAME', name=name)
def _max_pool(self, value, name):
return tf.nn.max_pool(value, ksize=[1, 2, 2, 1], strides=[1, 2, 2, 1],
padding='SAME', name=name)
def _conv_layer(self, value, name):
with tf.variable_scope(name):
filt = self._get_conv_filter(name)
conv = tf.nn.conv2d(value, filt, [1, 1, 1, 1], padding='SAME')
conv_biases = self._get_bias(name)
bias = tf.nn.bias_add(conv, conv_biases)
relu = tf.nn.relu(bias)
return relu
def _fc_layer(self, value, name):
with tf.variable_scope(name):
shape = value.get_shape().as_list()
dim = 1
for d in shape[1:]:
dim *= d
x = tf.reshape(value, [-1, dim])
weights = self._get_fc_weight(name)
biases = self._get_bias(name)
fc = tf.nn.bias_add(tf.matmul(x, weights), biases)
return fc
def _get_conv_filter(self, name):
return tf.Variable(self.model[name][0], name="filter")
def _get_bias(self, name):
return tf.Variable(self.model[name][1], name="biases")
def _get_fc_weight(self, name):
return tf.Variable(self.model[name][0], name="weights")
def _init_empty_model(self):
self.model = {
"conv1_1": [np.ndarray([3, 3, 3, 64]),
np.ndarray([64])],
"conv1_2": [np.ndarray([3, 3, 64, 64]),
np.ndarray([64])],
"conv2_1": [np.ndarray([3, 3, 64, 128]),
np.ndarray([128])],
"conv2_2": [np.ndarray([3, 3, 128, 128]),
np.ndarray([128])],
"conv3_1": [np.ndarray([3, 3, 128, 256]),
np.ndarray([256])],
"conv3_2": [np.ndarray([3, 3, 256, 256]),
np.ndarray([256])],
"conv3_3": [np.ndarray([3, 3, 256, 256]),
np.ndarray([256])],
"conv3_4": [np.ndarray([3, 3, 256, 256]),
np.ndarray([256])],
"conv4_1": [np.ndarray([3, 3, 256, 512]),
np.ndarray([512])],
"conv4_2": [np.ndarray([3, 3, 512, 512]),
np.ndarray([512])],
"conv4_3": [np.ndarray([3, 3, 512, 512]),
np.ndarray([512])],
"conv4_4": [np.ndarray([3, 3, 512, 512]),
np.ndarray([512])],
"conv5_1": [np.ndarray([3, 3, 512, 512]),
np.ndarray([512])],
"conv5_2": [np.ndarray([3, 3, 512, 512]),
np.ndarray([512])],
"conv5_3": [np.ndarray([3, 3, 512, 512]),
np.ndarray([512])],
"conv5_4": [np.ndarray([3, 3, 512, 512]),
np.ndarray([512])],
"fc6": [np.ndarray([25088, 4096]),
np.ndarray([4096])],
"fc7": [np.ndarray([4096, 4096]),
np.ndarray([4096])],
"fc8": [np.ndarray([4096, 1000]),
np.ndarray([1000])]}
return self.model
- 1
- 2
- 3
- 4
- 5
- 6
- 7
- 8
- 9
- 10
- 11
- 12
- 13
- 14
- 15
- 16
- 17
- 18
- 19
- 20
- 21
- 22
- 23
- 24
- 25
- 26
- 27
- 28
- 29
- 30
- 31
- 32
- 33
- 34
- 35
- 36
- 37
- 38
- 39
- 40
- 41
- 42
- 43
- 44
- 45
- 46
- 47
- 48
- 49
- 50
- 51
- 52
- 53
- 54
- 55
- 56
- 57
- 58
- 59
- 60
- 61
- 62
- 63
- 64
- 65
- 66
- 67
- 68
- 69
- 70
- 71
- 72
- 73
- 74
- 75
- 76
- 77
- 78
- 79
- 80
- 81
- 82
- 83
- 84
- 85
- 86
- 87
- 88
- 89
- 90
- 91
- 92
- 93
- 94
- 95
- 96
- 97
- 98
- 99
- 100
- 101
- 102
- 103
- 104
- 105
- 106
- 107
- 108
- 109
- 110
- 111
- 112
- 113
- 114
- 115
- 116
- 117
- 118
- 119
- 120
- 121
- 122
- 123
- 124
- 125
- 126
- 127
- 128
- 129
- 130
- 131
- 132
- 133
- 134
- 135
- 136
- 137
- 138
- 139
- 140
- 141
- 142
- 143
- 144
- 145
- 146
- 147
- 148
- 149
- 150
- 151
- 152
- 153
- 154
- 155
- 156
- 157
- 158
- 159
- 160
- 161
- 162
- 163
- 164
- 165
- 166
- 167
- 168
- 169
- 170
- 171
- 172
- 173
- 174
- 175
- 176
- 177
- 178
- 179
- 180
- 181
- 182
- 183
- 184
- 185
- 186
- 187
- 188
- 189
- 190
- 191
- 192
- 193
- 194
- 195
- 196
- 197
- 198
- 199
- 200
- 201
- 202
- 203
- 204
- 205
- 206
- 207
- 208
- 209
- 210
- 211
- 212
- 213
- 214
- 215
- 216
- 217
- 218
- 219
- 220
- 221
- 222
- 223
- 224
- 225
- 226
- 227
- 228
- 229
- 230
- 231
- 232
- 233
- 234
- 235
- 236
- 237
- 238
- 239
- 240
- 241
- 242
- 243
- 244
- 245
- 246
- 247
- 248
- 249
- 250
- 251
- 252
- 253
- 254
- 255
- 256
- 257
- 258
- 259
- 260
- 261
- 262
- 263
- 264
- 265
- 266
- 267
- 268
- 269
- 270
- 271
- 272
- 273
- 274
- 275
- 276
- 277
- 278
- 279
- 280
- 281
- 282
- 283
- 284
- 285
- 286
- 287
- 288
- 289
- 290
- 291
- 292
- 293
- 294
- 295
- 296
- 297
- 298
- 299
- 300
- 301
- 302
- 303
- 304
- 305
- 306
- 307
- 308
- 309
- 310
- 311
- 312
- 313
- 314
- 315
- 316
- 317
- 318
- 319
- 320
- 321
- 322
- 323
- 324
- 325
- 326
- 327
- 328
- 329
- 330
- 331
- 332
- 333
- 334
- 1
- 2
- 3
- 4
- 5
- 6
- 7
- 8
- 9
- 10
- 11
- 12
- 13
- 14
- 15
- 16
- 17
- 18
- 19
- 20
- 21
- 22
- 23
- 24
- 25
- 26
- 27
- 28
- 29
- 30
- 31
- 32
- 33
- 34
- 35
- 36
- 37
- 38
- 39
- 40
- 41
- 42
- 43
- 44
- 45
- 46
- 47
- 48
- 49
- 50
- 51
- 52
- 53
- 54
- 55
- 56
- 57
- 58
- 59
- 60
- 61
- 62
- 63
- 64
- 65
- 66
- 67
- 68
- 69
- 70
- 71
- 72
- 73
- 74
- 75
- 76
- 77
- 78
- 79
- 80
- 81
- 82
- 83
- 84
- 85
- 86
- 87
- 88
- 89
- 90
- 91
- 92
- 93
- 94
- 95
- 96
- 97
- 98
- 99
- 100
- 101
- 102
- 103
- 104
- 105
- 106
- 107
- 108
- 109
- 110
- 111
- 112
- 113
- 114
- 115
- 116
- 117
- 118
- 119
- 120
- 121
- 122
- 123
- 124
- 125
- 126
- 127
- 128
- 129
- 130
- 131
- 132
- 133
- 134
- 135
- 136
- 137
- 138
- 139
- 140
- 141
- 142
- 143
- 144
- 145
- 146
- 147
- 148
- 149
- 150
- 151
- 152
- 153
- 154
- 155
- 156
- 157
- 158
- 159
- 160
- 161
- 162
- 163
- 164
- 165
- 166
- 167
- 168
- 169
- 170
- 171
- 172
- 173
- 174
- 175
- 176
- 177
- 178
- 179
- 180
- 181
- 182
- 183
- 184
- 185
- 186
- 187
- 188
- 189
- 190
- 191
- 192
- 193
- 194
- 195
- 196
- 197
- 198
- 199
- 200
- 201
- 202
- 203
- 204
- 205
- 206
- 207
- 208
- 209
- 210
- 211
- 212
- 213
- 214
- 215
- 216
- 217
- 218
- 219
- 220
- 221
- 222
- 223
- 224
- 225
- 226
- 227
- 228
- 229
- 230
- 231
- 232
- 233
- 234
- 235
- 236
- 237
- 238
- 239
- 240
- 241
- 242
- 243
- 244
- 245
- 246
- 247
- 248
- 249
- 250
- 251
- 252
- 253
- 254
- 255
- 256
- 257
- 258
- 259
- 260
- 261
- 262
- 263
- 264
- 265
- 266
- 267
- 268
- 269
- 270
- 271
- 272
- 273
- 274
- 275
- 276
- 277
- 278
- 279
- 280
- 281
- 282
- 283
- 284
- 285
- 286
- 287
- 288
- 289
- 290
- 291
- 292
- 293
- 294
- 295
- 296
- 297
- 298
- 299
- 300
- 301
- 302
- 303
- 304
- 305
- 306
- 307
- 308
- 309
- 310
- 311
- 312
- 313
- 314
- 315
- 316
- 317
- 318
- 319
- 320
- 321
- 322
- 323
- 324
- 325
- 326
- 327
- 328
- 329
- 330
- 331
- 332
- 333
- 334
4 其他
http://www.cnblogs.com/xuanyuyt/p/5743758.html
这篇博客对为什么使用3×3的核解释的比较清楚,以及使用浅层模型训练来的参数对深层模型的前几层进行初始化也有解释。