[1]Howard A G, Zhu M, Chen B, et al. Mobilenets: Efficient convolutional neural networks for mobile vision applications[J]. arXiv preprint arXiv:1704.04861, 2017.
[2]Sandler M, Howard A, Zhu M, et al. Mobilenetv2: Inverted residuals and linear bottlenecks[J]. 2018 IEEE/CVF Conference on Computer Vision and Pattern Recognition (CVPR)[C], 2018: 4510-4520.
[3]Hu J, Fan C, Jiang H, et al. Boosting Light-Weight Depth Estimation Via Knowledge Distillation[J]. arXiv preprint arXiv:2105.06143, 2021.
[4]Wedel A, Badino H, Rabe C, et al. B-spline modeling of road surfaces with an application to free-space estimation[J]. IEEE transactions on Intelligent transportation systems 10(4), 2009:572-583
[5]Alvarez, J.M., Salzmann, M., Barnes, N. Learning appearance models for road detection[A]. In IEEE Intelligent Vehicles Symposium (IV)[C], New York, 2013, pp. 423-429.
[6]L. Caltagirone, M. Bellone, L. Svensson, and M. Wahde. Lidar camera fusion for road detection using fully convolutional neural networks[J]. Robotics and Autonomous Systems, 2019(111): 125-131.
[7]H. Liu, Y. Yao, Z. Sun, X. Li, K. Jia, and Z. Tang. Road segmentation with image-lidar data fusion in deep neural network[J]. Multimedia Tools and Applications, 2020, 79(47):35503-35518.
[8]Z. Chen, J. Zhang, and D. Tao. Progressive lidar adaptation for road detection[J]. IEEE/CAA Journal of Automatica Sinica, 2019, 6(3): 693-702.
[9]Scharwächter T, Franke U. Low-level fusion of color, texture and depth for robust road scene understanding[A]. Intelligent Vehicles Symposium IEEE[C]. 2015:599-604.
[10]Ramos S, Gehrig S, Pinggera P, et al. Detecting unexpected obstacles for self-driving cars: Fusing deep learning and geometric modeling[A]. 2017 IEEE Intelligent Vehicles Symposium (IV)[C]. 2017: 1025-1032.
参考文献.
最新推荐文章于 2023-07-25 16:24:47 发布
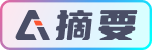