ai会取代程序员吗
I started doing some home baking recently. It started, like with a lot of other people, during the pandemic lockdown period when I got tired of buying the same bread from the supermarket every day. In all honesty, my bakes are passable, not very pretty but they please the family, which is good enough for me.
我最近开始做一些家庭烘焙。 与大批其他人一样,这是在大流行封锁时期开始的,那时我厌倦了每天从超市买同样的面包。 老实说,我的烘焙还算不错,虽然不是很漂亮,但它们却令整个家庭满意,这对我来说已经足够了。
Yesterday I stumbled on a YouTube video on how a factory makes bread in synchronised perfection and it broke a bit of my heart. All the hard work kneading dough amounts to nothing compared to spinning motors tumbling through a mechanised giant bucket. As I watch rows and rows of dough rising in unison spirals up the proofing carousel then slowly rolling into a constantly humming monstrous oven to become marching loaves of bread, something died in me.
昨天,我偶然发现了一个YouTube视频,内容涉及一家工厂如何同步制作面包,这让我很伤心。 与通过机械化的巨型铲斗翻滚的旋转电机相比,所有艰苦的揉捏生面团都不算什么。 当我看到成排的面团以一致的方式升起时,在发酵转盘上盘旋成螺旋形,然后慢慢地滚动进一个不断嗡嗡作响的巨大烤箱中,变成行进的面包,其中有些东西死在了我身上。
When the loaves zipped themselves into sealed bags and dumped themselves into packing boxes, I tell myself that they don’t have the same craftsmanship (in my mind) as someone who is making bread with love, for his family.
当面包将它们拉成密封袋并扔进包装箱时,我告诉自己,他们的手Craft.io(在我看来)与为自己的家人用爱做面包的人不同。
But deep inside me, I understand that if bread depended on human bakers only, it would be a whole lot more expensive, a lot more people would go hungry. Automation and manufacturing lines have had always fed the masses since they were invented. The pride of a human baker matters little compared to feeding all the ever growing populations of human beings.
但是在我内心深处,我了解到,如果仅依靠人类面包师做面包,那将贵很多,而且会有更多的人挨饿。 自从他们被发明以来,自动化和生产线一直满足大众的需求。 与养活所有不断增长的人类人口相比,人类面包师的骄傲意义不大。

Fortunately, I don’t bake in my day job. I’m in the software development industry, where the main activity involves software programmers (developers, engineers, coders – take your pick) writing code to build simple to complex software systems that control much of the world today (even bread factories are controlled by manufacturing control systems). Programmers who are also humans. Programmers who are highly sought-after craftsmen where their experience, talent and creativity is celebrated.
幸运的是,我没有烘烤日常工作。 我在软件开发行业中,主要活动涉及软件程序员(开发人员,工程师,编码人员-随您选择),编写代码以构建可控制当今世界大部分地区的简单到复杂的软件系统(甚至面包厂也受其控制)。制造控制系统)。 也是人类的程序员。 程序员是备受追捧的工匠,他们的经验,才华和创造力得到赞扬。
Just like bakers.
就像面包师一样。
Of course, this begs the question – are there software factories where software is churned out by machines, just like bread factories? Do they exist today?
当然,这引出了一个问题–是否有像面包厂一样由机器生产软件的软件厂? 它们今天存在吗?
生产线 (Manufacturing line)
Another perspective is that software development today is already a manufacturing line, with different software development teams working to take up different parts of the whole system to be assembled finally in well-organized processes.
另一个观点是,当今的软件开发已经是一条生产线,由不同的软件开发团队共同负责整个系统的不同部分,并最终以组织良好的流程进行组装。
In place of factory workers, we have programmers, in place of supervisors we have team leads and in place of a plant manager we have engineering managers. With a combination of outsourcing, offshoring, contracting as well as various manpower supplementary methods, companies can potentially ramp up software development as well.
我们有程序员,代替工厂工人,有团队领导,而不是主管,有工程经理,有工厂经理。 通过将外包,离岸外包,合同签订以及各种人力补充方法结合起来,公司还可以潜在地加快软件开发。

Naturally, no programmer likes to be called a factory worker. In fact, most of us prefer to be called software craftsman. But there is no denying that somewhere along the way, developing software in a large-scale environment is very much like a factory.
自然,没有程序员喜欢被称为工厂工人。 实际上,我们大多数人都喜欢被称为软件工匠 。 但是,不可否认的是,在大规模开发环境中开发软件非常像工厂。
Today, all large-scale software development happens in teams (doesn’t matter what it’s called or how much autonomy it’s given) and guided by some processes. Whether it’s agile, iterative, behaviour-driven, waterfall or any of the hybrid variants, most software development organizations have some sort of process and methodology. Larger organizations even have specialised teams to implement the processes and process models such as CMMI (Capability Maturity Model Integration) and ISO/IEC 12207.
如今,所有大规模软件开发都是在团队中进行的(无论它叫什么名称或给予多少自治权),并受某些流程的指导。 无论是敏捷,迭代,行为驱动,瀑布式还是任何其他混合形式,大多数软件开发组织都具有某种过程和方法论。 较大的组织甚至拥有专门的团队来实施过程和过程模型,例如CMMI(能力成熟度模型集成)和ISO / IEC 12207 。
Some of these process models can get pretty serious. For example, CMMI which was developed by the Carnegie Mellon University (CMU) and the US Department of Defense, is required in many US government software development contracts.
这些过程模型中的一些模型可能会变得非常严肃。 例如, 许多美国政府软件开发合同都要求使用由卡耐基梅隆大学(CMU)和美国国防部开发的CMMI。
While the idea of software development as a manufacturing line is not very appealing, and even counter to the image of the cool tech startup development, once software gets to a certain scale, structure and processes are part and parcel of production.
尽管将软件开发作为生产线的想法不是很吸引人,甚至与酷技术启动开发的形象背道而驰,但是一旦软件达到一定规模,结构和流程便成为生产的一部分。

And as in baking bread, brewing beer and many other artisan jobs, making great software is a source of pride and joy. But when it comes to scale and production a process-oriented approach is critical to ensure quality, and more importantly, consistency.
就像在烤面包,酿造啤酒和许多其他工匠的工作中一样,制作出色的软件是自豪和喜悦的源泉。 但是,在规模和生产方面,面向流程的方法对于确保质量(更重要的是确保一致性)至关重要。
However, as much as we build in processes, it’s all still done by human beings and is prone to the famous ID-10T error. That’s why over time, we have moved over to continuous automated integration, testing and deployment. The idea is to reduce as much human error as possible when integrating multiple parts and components of the system we’re building by using scripts that automate the processes.
但是,尽管我们在流程中进行了很多构建,但这仍然是由人类完成的,并且容易发生著名的I D-10T错误 。 这就是为什么随着时间的推移,我们已转向持续不断的自动化集成,测试和部署。 这个想法是在使用自动化过程的脚本集成正在构建的系统的多个部分和组件时,尽可能减少人为错误。
This works remarkably well but obviously there is still a gap — the system is designed by humans, the different parts and components are written by humans, even the automated scripts are written by humans. The system is only as robust as the weakest link and we are that link.
这非常有效,但显然仍然存在差距-系统是由人设计的,不同的部件和组件是由人编写的,甚至自动化脚本也是由人编写的。 该系统仅与最弱的链接一样强大,而我们就是那个链接。
But what if every part of the process is automated?
但是,如果流程的每个部分都是自动化的怎么办?
自动化 (Automation)
In one of my favourite books, The Moon Is A Harsh Mistress (published 1966), Mike (short for Mycroft Holmes, Sherlock’s older brother) was an AI that “woke up”. Mike could understand classic programming but he could also understand Loglan (this is a real language) and English. Mannie (short for Manuel), the narrator and computerman (how cool is that name), in fact, interacts with him mainly in English. Mike does his own programming based on their interactions. Imagine that. And this is in from the mid-60s.
在我最喜欢的一本书《月亮是一个残酷的情妇》 (1966年出版)中,迈克(夏洛克的哥哥迈克罗夫特·福尔摩斯的缩写)是一台“醒来的”人工智能。 Mike可以理解经典编程,但也可以理解Loglan (这是一种真实的语言)和英语。 叙事者和计算机人 Mannie(Manuel的缩写)(实际上叫这个名字多么酷)实际上主要是用英语与他互动。 Mike根据他们的互动进行自己的编程。 设想。 这是从60年代中期开始的。
The idea that to program computers you only need to tell it your problems and it will create the necessary software to solve the programs is crazily compelling. In the 80s and 90s, there were several movements to create 4th generation and 5th generation languages (4GL and 5GL) that will do exactly that, and they were marketed heavily.
对计算机进行编程的想法只需要告诉它您的问题,并且它将创建必要的软件来解决该程序的想法令人信服。 在80年代和90年代,出现了几项创建第四代和第五代语言 (4GL和5GL)的动作,正是这些动作可以做到这一点,并且它们的销售量很大。

The intention was that eventually, actual coding wasn’t necessary, all it takes is to define the problem and the code will be generated to solve it. Naturally, the focus was then to define the problem is a much more precise way so that better code can be generated. To do that, you’d need to build models and have a user-friendly way describing the problem such that even non-software engineers can create software. My company then (like a lot of enterprises of its day) bought into it hook, line and sinker. To be honest, I fell for it too.
目的是最终不需要实际的编码,只需要定义问题,然后生成代码来解决问题。 自然地,接下来的重点是以更精确的方式定义问题,以便生成更好的代码。 为此,您需要构建模型并采用一种用户友好的方式来描述问题,以便即使非软件工程师也可以创建软件。 然后,我的公司(像当时的许多企业一样)购买了钩,线和沉降片。 老实说,我也很喜欢。
It never really took off because … programming languages were already doing that. To make a programming language suitable for non-programmers you’d need to make it less rigid and more suitable for humans. But that would result in it being more fuzzy and less precise. In which case it can only create really simple, unambiguous software. To create more complex software you’d need someone who can not only define the problem but come up with the solution, write the code, make sure it runs and updates it as needed i.e. you need a programmer.
它从未真正起飞,因为……编程语言已经在这样做。 为了使编程语言适合非程序员,您需要使其僵化程度降低,更适合人类。 但这将导致它更加模糊和不精确。 在这种情况下,它只能创建非常简单,明确的软件。 要创建更复杂的软件,您需要一个不仅可以定义问题而且可以提出解决方案的人员,编写代码,确保其运行并根据需要对其进行更新,即您需要一名程序员。
Not that 4GL/5GL wasn’t useful, just that its promises for the death of the software development industry was greatly exaggerated. The project I was working on crashed and burned, losing millions along the way (though I can’t blame it entirely on 4GL, it was partly the reason too, overpromising on the capabilities but ultimately underdelivering on the results).
并不是说4GL / 5GL没什么用,只是它对软件开发行业灭亡的承诺被大大夸大了。 我正在从事的项目崩溃并被烧毁,一路上损失了数百万美元(尽管我不能完全将其归咎于4GL,这也是部分原因,对功能的承诺过高,但最终未能实现结果)。
软件创建软件 (Software creating software)
The 4GL/5GL wasn’t the answer partly because there’re still humans in the equation. What if we go deep and get software to create software? The developments in AI in the past few years, specifically machine learning, have resurfaced the idea. Machine learning is increasingly being used in a lot of software that’s written today. There is already machine learning software that is beating professional Go players, analysing markets, driving cars, and even creating music and art by learning from past masters. Is someone today creating some machine learning software that would write other software?
4GL / 5GL不能部分解决问题,因为方程式中仍然有人类。 如果我们深入研究并获得创建软件的软件怎么办? 人工智能在过去几年的发展,特别是机器学习,使这一想法浮出水面。 机器学习正越来越多地用于当今编写的许多软件中。 已经有机器学习软件击败专业围棋选手,分析市场,驾驶汽车,甚至通过向过往的大师学习来创作音乐和艺术。 今天有人在创建一些可以编写其他软件的机器学习软件吗?
Let’s break down what that means.
让我们解释一下这是什么意思。
There are a few types of software that creates other software. First, there is self-reproduction, which really means how a piece of software can reproduce itself. The idea is not new, in fact, John von Neumann theorized about self-reproducing automata in the 1940s.
有几种类型的软件可以创建其他软件。 首先,存在自我复制,这实际上意味着软件可以如何自我复制。 这个想法并不新鲜,实际上, 约翰·冯·诺伊曼 ( John von Neumann)在1940年代提出了关于自我复制自动机的理论 。
奎因 (Quines)
At the source code level, there are quines, which are programs that take no input and produces a copy of its own source code as its only output. Here’s a rather impressive quine called Qlobe created by Yusuke Endoh, a Ruby core member.
在源代码级别上,有quines,它们是不输入任何程序并产生其自身源代码的副本作为其唯一输出的程序 。 这是一个由Ruby核心成员Yusuke Endoh创建的名为Qlobe的令人印象深刻的藜 。
Run this in the command line:
在命令行中运行:
curl -fSSl https://gist.githubusercontent.com/shime/f0ebe84ca42c33b51d42/raw/5e74315dc6b6fe572f8a457536ad7eb17ad3f1e4/qlobe.rb > qlobe.rb; while true; do ruby qlobe.rb | tee temp.rb; sleep 0.1; mv -f temp.rb qlobe.rb; done
You’ll see a spinning globe of code.
您会看到旋转的代码地球。

蠕虫 (Worms)
While quines are harmless fun, computer worms are not. Computer worms are standalone malware programs that self-reproduces and spreads across networks. The term “worm” was first used in the 1975 novel The Shockwave Rider by John Brunner. One of the first worms was the Morris worm, created by Robert Tappan Morris, a computer science graduate student from Cornell University in 1988. The Morris worm disrupted close to 10% of all computers on the Internet (not such a big number considering the size of the Internet then) in a virulent denial-of-service attack.
虽然藜无害,但计算机蠕虫却不是。 计算机蠕虫是独立的恶意软件程序,可以在网络中自我复制和传播。 “蠕虫”一词最早出现在1975年约翰·布鲁纳(John Brunner)的小说《冲击波骑士 》( The Shockwave Rider)中 。 Morris蠕虫是最早的蠕虫之一,它是1988年由康奈尔大学计算机科学专业的研究生Robert Tappan Morris创建的。Morris蠕虫破坏了Internet上近10%的所有计算机 (考虑到计算机的大小,这一数目并不是一个大数目)然后通过互联网进行激烈的拒绝服务攻击。
One of the most notorious worms is Stuxnet, which attacked industrial SCADA systems and is believe to have caused susbstantial damage to the Iran’s nuclear program. It is also believed to be a cyberweapon built jointly by US and Israel. I’ll let that sink in a while. State sponsored cyberattack on a nuclear facility.
最臭名昭著的蠕虫之一是Stuxnet ,它攻击了工业SCADA系统,并且据信已对伊朗的核计划造成了可观的破坏。 它也被认为是美国和以色列共同建造的网络武器。 我会让它沉没一会儿。 国家赞助对核设施的网络攻击。

Of course, self-reproduction is just a mechanism, it’s the payload that is malicious. Payloads can also be benign, though they are relatively rare. For example, the Welchia worm infects computers to combat the Blaster worm. It automatically downloads Microsoft security updates for Windows and reboots the computers, although it does that without any permissions. Obviously not everyone considers it friendly.
当然,自我复制只是一种机制,它是恶意的有效负载。 有效负载也可能是良性的,尽管它们相对较少。 例如, Welchia蠕虫感染计算机以对抗Blaster蠕虫 。 它会自动下载Windows的Microsoft安全更新,然后重新启动计算机,尽管这样做没有任何权限。 显然,并不是每个人都认为它友好。
基因编程 (Genetic programming)
What we talked about before is self-reproduction, which is basically software cloning a copy of itself. In the organic world, besides ameobas and other single cell animals, reproduction is more like producing offspring, creating newer generations of itself. This happens in software too!
我们之前所说的是自我复制,基本上是软件克隆其自身的副本。 在有机世界中,除了变形虫和其他单细胞动物外,繁殖更像是繁殖后代,从而创造了自己的后代。 这也发生在软件中!
Genetic programming (GP) is one way software can produce other software. GPs are population-based meta-heuristics that evolves programs that best solves a particular problem.
遗传编程(GP)是软件可以产生其他软件的一种方式。 GP是基于人群的元启发式算法,它可以开发出最能解决特定问题的程序。

Alan Turing was the first to propose evolving programs in his 1950 paper, Computing Machinery and Intelligence. However, the GP and in general, the field of evolutionary algorithms was first laid out in John Holland’s classic book, Adaptation in Natural and Artificial Systems, first published in 1975.
艾伦·图灵(Alan Turing)在其1950年的论文《 计算机技术与情报 》中率先提出了不断发展的程序。 但是,GP和一般而言,进化算法领域是在约翰·霍兰德(John Holland)于1975年首次出版的经典著作《 自然与人工系统的适应》中首次提出的。
GPs generally follow the principles of natural selection. These are the basic steps of a GP:
- Generate the initial population of programs randomly (first generation) 随机生成程序的初始种群(第一代)
- Evaluate the fitness of each individual program 评估每个程序的适用性
- Select the best programs for reproduction of the next generation 选择最好的程序以再现下一代
- Reproduce next generation programs from the previous generation of best solutions, through crossover and mutation mechanisms 通过交叉和变异机制重现上一代最佳解决方案中的下一代程序
- Replace the less-fit programs with the next generation 用下一代替换不合适的程序
- Repeat steps 2 to 5 until the termination condition is met, usually when a suitable program to the problem is found 重复步骤2到5,直到满足终止条件为止,通常是在找到适合该问题的程序时
Programs are represented in memory as tree structures in GP, so traditionally GP favours the use of programming languages that naturally uses tree structures. I’ve talked about another type variant of this, called genetic algorithms (GA) in another story. Both GP and GA are variants of a field broadly known as evolutionary algorithms. While concepts for either are very similar, GP’s main representation are programs while GA’s main representation is in raw data (often in strings).
程序在GP中以树结构形式在内存中表示,因此传统上GP倾向于使用自然使用树结构的编程语言。 我在另一个故事中谈到了这种类型的另一种变体,称为遗传算法(GA) 。 GP和GA都是该领域的变体,被广泛称为进化算法 。 尽管两者的概念非常相似,但GP的主要表示形式是程序,而GA的主要表示形式是原始数据(通常是字符串)。
GPs are fascinating not only because it is something we as programmers did not code, but is evolved through generations. It’s also because it’s software that can continually change and adapt to the environment. It’s software not only creating software but literally giving birth to software offspring.
GP令人着迷,不仅因为它是我们作为程序员没有编写过的东西,而且是几代人不断发展的东西。 这也是因为它是可以不断变化并适应环境的软件。 它不仅是软件的创造软件,而且实际上是软件后代的诞生。
深度神经网络 (Deep neural networks)
Deep neural networks (or deep learning) is a type of machine learning algorithm based on artificial neural networks. The word “deep” refers to the multiple layers in a neural network. Deep neural networks (DNN) has been getting a lot of attention in recent years, with astonishing results and sometimes a bit of hype. I won’t delve too deeply (pun intended) into the how it works here but I’ve written a couple of stories on it, including one that shows how someone can build a neural network from ground up.
深度神经网络(或深度学习)是一种基于人工神经网络的机器学习算法。 术语“深层”是指神经网络中的多层。 近年来,深度神经网络(DNN)受到了广泛关注,其结果令人惊讶,有时还会大肆宣传。 我不会太深入地研究它的工作原理,但是我已经写了一些故事,其中包括一些故事,展示了人们如何从头开始构建神经网络 。
Neural networks aren’t new, the concepts date all the way to the 1950s, with many breakthroughs in the 1980s and 1990s. What really accelerated things were the breakthroughs and availability of computational power and growth of data. To use more buzzwords, cloud and big data made DNNs possible.
神经网络并不是什么新鲜事物,其概念一直可以追溯到1950年代,在1980年代和1990年代有了许多突破。 真正加速的是计算能力的突破和可用性以及数据的增长。 要使用更多的流行词,云和大数据使DNN成为可能。
In 2014, DeepMind, a UK artificial intelligence company started a project called AlphaGo to build a deep neural network to compete in Go. By March 2016, it played Lee Sedol, one of the best Go players in the world and won 4 out of 5 games (using 1,920 CPUs and 280 GPUs). That 1 loss was the last time AlphaGo lost to a human being in an official game.
2014年, 英国人工智能公司DeepMind启动了一个名为AlphaGo的项目,目的是构建一个深度神经网络来与Go竞争。 到2016年3月,它扮演了Lee Sedol,这是世界上最好的围棋选手之一,并在5场比赛中赢得4场比赛(使用1,920个CPU和280个GPU)。 那一次的损失是AlphaGo在正式比赛中最后一次输给一个人。

In May 2017, AlphaGo played 3 games with Ke Jie, the world No 1 ranked Go player and won all 3, after which it was awarded professional 9-dan by the Chinese Weiqi Association.
2017年5月,AlphaGo与世界排名第一的围棋选手柯洁(Ke Jie)踢了3场比赛,并赢得了所有3场比赛,此后它被中国围棋协会授予职业9丹。
DNNs has been used in many many other fields, from image and speech recognition, to natural language processing and financial fraud detection. Self-driving vehicles (cars, buses, trucks), as well as autonomous robots (both ground-based and drones), depend heavily on DNNs. Cancer researchers use DNNs to detect cancer cells, DNNs has been used to generate music and art, even black and white photos have been recolored using DNNs.
DNN已用于许多其他领域,从图像和语音识别到自然语言处理和财务欺诈检测。 无人驾驶汽车(汽车,公共汽车,卡车)以及自动驾驶机器人(基于地面的机器人和无人驾驶飞机)在很大程度上取决于DNN。 癌症研究人员使用DNN来检测癌细胞,DNN已被用于生成音乐和艺术作品,甚至黑白照片也已使用DNN重新着色。
But can DNNs be used to create software?
但是DNN可以用于创建软件吗?
There are some attempts to generate source code but I don’t think that is really necessary. What we want is performed by the DNN models already — the DNN models are the software.
有一些尝试生成源代码的尝试,但是我认为这不是真正必要的。 我们想要的已经由DNN模型执行-DNN模型是软件。
Let me explain what I mean.
让我解释一下我的意思。
该模型可能是软件 (The model is probably the software)
In structured programming, we use sequence, selection (if-then-else) and iteration (for, while) to describe rules that processes data to produce results. Very often these rules are bundled into functions, which takes data input and produces results.
在结构化编程中 ,我们使用顺序,选择(if-then-else)和迭代(for,while)来描述处理数据以产生结果的规则。 这些规则通常捆绑在函数中,函数接受数据输入并产生结果。
DNN models use multiple layers in multiple DNNs (many DNNs are assembled from different types of neural networks) to do the same. DNNs train models using massive amounts of data and once these models have been trained, they can be used to predict results, given new data. This means DNN models are functions that takes data input and products results too. In fact, DNN models can approximate and mimic any function!
DNN模型在多个DNN中使用多层(许多DNN是由不同类型的神经网络组装而成)来完成的。 DNN使用大量数据训练模型,一旦对这些模型进行训练,就可以使用给定的新数据来预测结果。 这意味着DNN模型是需要数据输入和乘积结果的函数。 实际上,DNN模型可以近似和模拟任何功能!
The difference is that while structured code can be traced and followed, it is very difficult to trace the data flow through the different layers of a DNN model. This is because there are just too many parameters to be reasonably walked through. For example, LeNet-5 (published in 1998), one of the simplest convolutional neural network (CNN), has about 60,000 parameters. VGG-16 (published in 2014), a more recent CNN has about 138 million parameters!
区别在于,虽然可以跟踪和遵循结构化代码,但要跟踪通过DNN模型的不同层的数据流非常困难。 这是因为有太多参数无法合理遍历。 例如,最简单的卷积神经网络(CNN)之一LeNet-5 (于1998年发布) 具有约60,000个参数 。 VGG-16(于2014年发布)是最新的CNN,具有约1.38亿个参数!

In addition, the process to train models is stochastic and therefore the parameter values of the various nodes within the layers might be different even if we use the same training data!
此外,训练模型的过程是随机的,因此即使我们使用相同的训练数据,各层内各个节点的参数值也可能不同!
Sure, after the training, DNNs are deterministic, meaning if you pass in the same data each time, the same results will be reproduced. However, many DNNs are designed to return a probabilistic result.
当然,在训练之后,DNN是确定性的,这意味着,如果您每次传递相同的数据,则将再现相同的结果。 但是,许多DNN旨在返回概率结果。
This might be confusing so let me give you an example. Let’s say we designed a DNN and train it with lots and lots of cat photos so that it can identify if a given photo has a cat.
这可能会令人困惑,所以让我举一个例子。 假设我们设计了一个DNN并用很多猫照片训练它,以便它可以识别给定的照片中是否有猫。
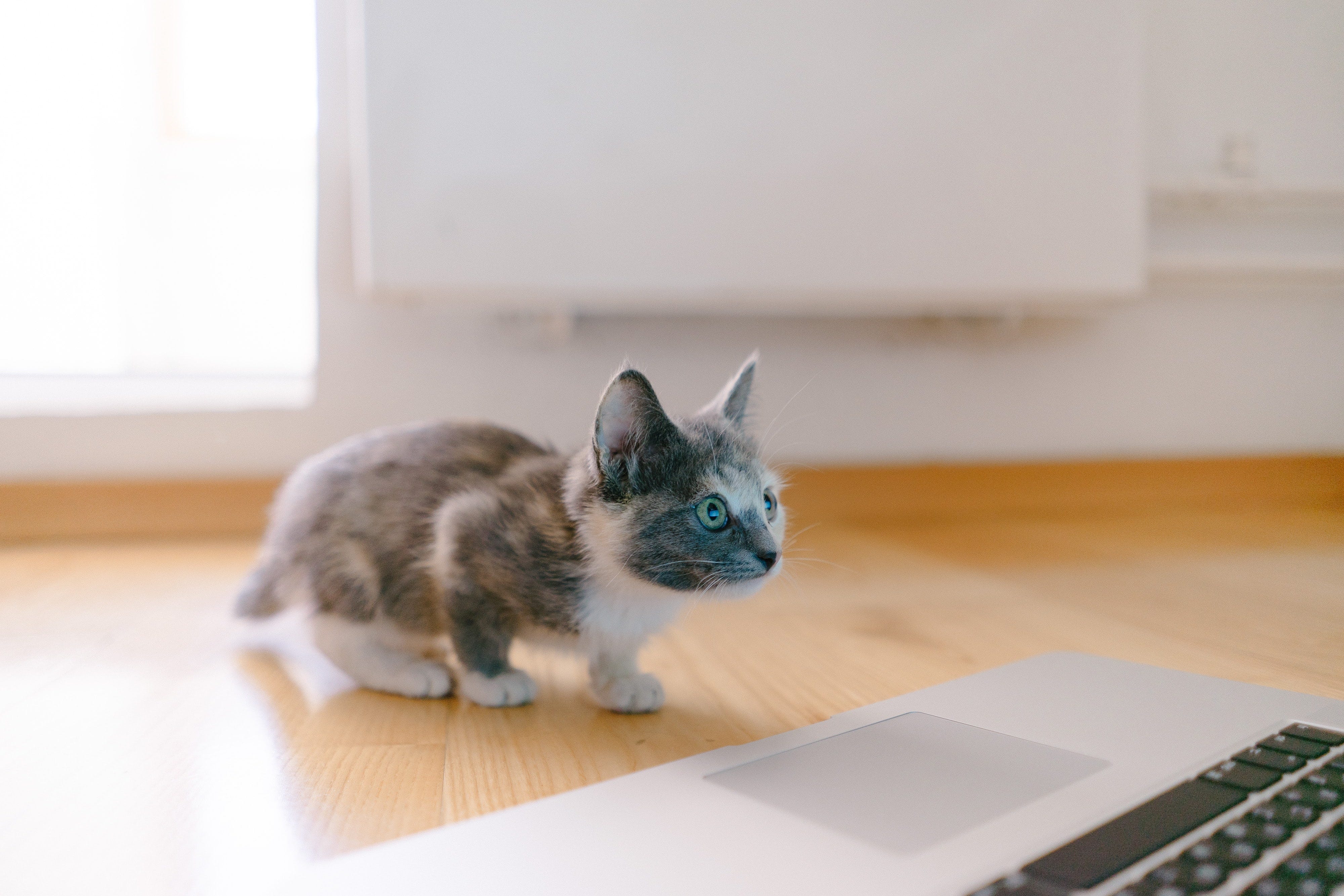
Next we give it a picture. What will be DNN model return? It will be a probability of whether a cat is in the photo or not.
接下来我们给它一张图片。 DNN模型将返回什么? 可能是照片中是否有猫。
So how do we determine the accuracy of the model? We test it again with a whole bunch of photos we (as human beings) determine to be cat photos and we see how many it got correct or wrong. The correctness of the response is again determined by us. We can say if the probability is > 80% (or any number, really) it has a cat.
那么我们如何确定模型的准确性呢? 我们用一堆我们(作为人类)确定是猫的照片再次测试它,然后看看有多少张照片正确或错误。 响应的正确性再次由我们确定。 我们可以说,如果概率> 80%(或任何数字,实际上),它就有一只猫。
Let’s say we test it with 1,000 more cat photos and the model comes 90% of the time correct. What does that really mean? Well, we can decrease the correctness threshold and say that if probability result is > 70%, it means it is a cat, and then suddenly the model becomes 95% correct. Or if we train the model again with even more photos, and model becomes 97% correct. Or 85% correct, depending on the photos. Or 88% if we use a different set of 1,000 cat photos.
假设我们再使用1000张猫照片进行测试,并且该模型有90%的时间正确。 那个的真实意义是什么? 好吧,我们可以降低正确性阈值,并说,如果概率结果> 70%,则意味着它是一只猫,然后突然该模型变为95%正确。 或者,如果我们使用更多照片再次训练模型,则模型将变为97%正确。 或85%正确,取决于照片。 如果我们使用另一组1000张猫照片,则为88%。
We don’t really know. The results are probabilistic, despite the model being deterministic.
我们真的不知道。 尽管模型是确定性的,但结果是概率性的。
它是如何做到的? (How does it do it?)
Wait, there’s a bit more than that. We know machine learning models like DNN models predict results. But actually we don’t really know how it does that. After training a model, we know it produces certain results, but we don’t know exactly why. It’s a black box.
等等,还有更多。 我们知道像DNN模型这样的机器学习模型可以预测结果。 但是实际上我们并不真正知道它是如何做到的。 训练模型后,我们知道它会产生某些结果,但我们不知道为什么。 这是一个黑匣子。
But if it’s deterministic, we should know right?
但是,如果是确定性的,我们应该知道吗?
Let’s start with a simple example. Let’s say we have a mathematical function f(x,y)
where x = 1
and y = 2
that takes in 2 numbers, 1
and 2
and returns a third number 3
.
让我们从一个简单的例子开始。 假设我们有一个数学函数f(x,y)
,其中x = 1
和y = 2
接受两个数字1
和2
并返回第三个数字3
。

The function that immediately comes to mind is a simple addition, we take 1
and add 2
and we get 3
. But is that the only function? Actually no, we don’t really know what else happens in the function, so the if the function takes 1
and adds 100
then adds 2
and then subtracts 100
the function still returns 3
. For all purposes, we can’t tell the difference. And there can be an infinite number of such functions, as long as we take in 1
and 2
and return 3
.
马上想到的功能是一个简单的加法,我们取1
并加2
,得到3
。 但这是唯一的功能吗? 实际上不,我们真的不知道该函数还会发生什么,因此,如果该函数取1
并加100
,然后加2
,然后减100
,则该函数仍返回3
。 出于所有目的,我们无法说出区别。 只要我们接受1
和2
并返回3
,就可以有无数个这样的函数。
Now let’s say there is a mathematical function f(d)
that can take in photos as an input and produces a probability whether there is a cat in it. Just as in our previous example, there could be any number of such functions that does this.
现在,假设有一个数学函数f(d)
可以将照片作为输入,并产生其中是否有猫的概率。 就像在前面的示例中一样,可以有许多这样的函数来执行此操作。

The DNN model we described above approximates f(d)
by taking in the same input and producing the same output, but we don’t actually know which function it actually approximates. And we cannot say for sure it replicates any function either, unless we provide and test the finite set of data. But if we do that we don’t really need the DNN model anymore, do we?
我们上面描述的DNN模型通过吸收相同的输入并产生相同的输出来近似f(d)
,但实际上我们不知道它实际上近似哪个函数。 而且,除非我们提供并测试有限的数据集,否则我们不能肯定地说它会复制任何函数。 但是,如果这样做,我们真的不再需要DNN模型了,对吧?
So we end up with generated software which results and rules are no longer deterministic. This sounds totally useless except that DNN models can approximate any function and predict anything given suitable algorithms, lots of processing power and enough data.
因此,我们最终得到了生成的软件,其结果和规则不再是确定性的。 这听起来完全没用,只是DNN模型可以在适当的算法,大量处理能力和足够数据的情况下近似任何功能并预测任何内容。
This is its super power.
这是它的超级力量。
这到底是什么意思? (What does that all mean?)
Now that we’ve done a bit of a tour, let’s get back to our original question — are machines going to replace programmers, will we lose our jobs?
现在,我们已经进行了一些浏览,让我们回到最初的问题—机器将取代程序员,我们会丢掉工作吗?
So far as I can see nothing has taken any programmers’ job yet. If anything, it has intensified the need for more and better programmers. But what kind of programmers will be needed? That’s not a straightforward answer.
据我所知,到目前为止,还没有任何工作完成任何程序员的工作。 如果有的话,它加剧了对更多更好的程序员的需求。 但是将需要什么样的程序员? 这不是一个简单的答案。
The current programming paradigm, which is structured and deterministic has been with us a long time, and will continue to be with us in the forseeable future. But much of the new exciting work will be in the field creating new machine learning algorithms, curating data pipelines, training and nurturing models.
当前的结构化和确定性的编程范式已经存在了很长时间,并且在可预见的将来将继续存在。 但是,许多令人兴奋的新工作将是在该领域中创建新的机器学习算法,管理数据管道,培训和培养模型。
New roles will come about to deal with the new probabilistic programming paradigm. For example, in software development, quality control activities are a critical part of the overall process. But how do you ensure a probabilistic function will always perform as needed? How do we ensure that we never miss a cat in a photo? Or say there’s a cat where there isn’t one?
将出现新角色来应对新的概率编程范例。 例如,在软件开发中,质量控制活动是整个过程的关键部分。 但是,如何确保概率函数将始终根据需要执行? 我们如何确保我们不会错过照片中的猫? 还是说有一只猫没有一只猫?
Genetic programs and DNN models are generated software. DNN models are generated without even source code and we don’t necessarily understand its rules and logic. That causes problems we don’t know how to resolve yet. For example, if an error comes about when the software runs, who is responsible? And how do we assure that it won’t happen again? This is not hypothetical. Tesla’s semi-autonomous Autopilot feature has been found to be partially responsible for fatal accidents.
遗传程序和DNN模型是生成的软件。 DNN模型是在没有源代码的情况下生成的,我们不一定了解其规则和逻辑。 这导致了我们尚不知道如何解决的问题。 例如,如果在软件运行时发生错误,谁负责? 我们如何确保它不会再次发生? 这不是假设。 特斯拉的半自动驾驶仪功能被认为是致命事故的部分原因 。

As programmers, we need to navigate this new landscape and adapt to it. But as programmers, we probably will. After all, our industry is all about constant change.
作为程序员,我们需要浏览这个新环境并适应它。 但是作为程序员,我们可能会。 毕竟,我们的行业是关于不断变化的。
翻译自: https://towardsdatascience.com/are-machines-going-to-replace-programmers-995072159365
ai会取代程序员吗