lstm预测单词
Yo reader! I am Manik. What’s up?.
哟读者! 我是曼尼克 。 这是怎么回事?。
Hope you’re doing great and working hard for your goals. If not, it’s never late. Start now, at this very moment.
希望您做得很好,为实现目标而努力。 如果没有,那就永远不会晚。 从这一刻开始。
With this piece of information, you’ll walk away with a clear explanation on Sequence and Text processing for Deep Neural Networks which includes:
掌握了这些信息后,您将获得有关深度神经网络的序列和文本处理的清晰说明,其中包括:
- What’s one-hot Encoding? 什么是一键编码?
- OneHot Encoding with keras. 用keras进行OneHot编码。
- What are word embeddings and their advantage over One-Hot encoding? 什么是词嵌入及其相对于一键编码的优势?
- What are word embeddings trying to say? 单词嵌入试图说什么?
- A complete example of converting raw text to word embeddings in keras with an LSTM and GRU layer. 使用LSTM和GRU层在keras中将原始文本转换为单词嵌入的完整示例。
if you want to learn about LSTMs, you can go here
如果您想了解LSTM,可以点击这里
让我们开始吧。 (Let’s get started.)
“Yours and mine ancestors had run after a mastodons or wild boar, like an olympic sprinter, with a spear in hand covering themselves with leaves and tiger skin, for their breakfast” — History
“您和我的祖先曾像奥林匹克短跑运动员一样追捕过猛兽或野猪,手拿长矛遮住自己的叶子和老虎皮作为早餐” –历史
The above sentence is in textual form and for neural networks to understand and ingest it, we need to convert it into some numeric form. Two ways of doing that are One-hot encoding and the other is Word embeddings.
上面的句子是文本形式的,为了让神经网络理解和吸收它,我们需要将其转换为某种数字形式。 两种方法是一种热编码 ,另一种是Word嵌入 。
一站式 (One-Hot)
This is a way of representing each word by an array of 0s and 1. In the array, only one index has ‘1’ present and rest all are 0s.
这是一种用0和1的数组表示每个单词的方法。在该数组中,只有一个索引存在'1',其余所有索引均为0。
Example: The following vector represents only one word, in a sentence with 6 unique words.
示例:以下向量在一个包含6个唯一单词的句子中仅表示一个单词。

与numpy一热 (One-Hot with numpy)
Let’s find all the unique words in our sentence.
让我们找到句子中所有独特的词。
array(['Yours', 'a', 'after', 'an', 'ancestors', 'and', 'boar,',
'breakfast', 'covering', 'for', 'had', 'hand', 'in','leaves',
'like', 'mastodons', 'mine', 'olympic', 'or', 'run', 'skin,',
'spear', 'sprinter,', 'their', 'themselves', 'tiger', 'wild',
'with'], dtype='<U10')shape: (28,)
Now, give each of them an index i.e. create a word_index where each word has an index attached to it in a dictionary.
现在,给每个单词一个索引,即创建一个word_index,其中每个单词在字典中都附有一个索引。
You might have observed above in the code that 0 is not assigned to any word. It’s a reserved index in Keras(We’ll get here later).
您可能在上面的代码中注意到没有将0分配给任何单词。 这是Keras中的保留索引(稍后我们会在这里)。
{'Yours': 1, 'a': 2, 'after': 3, 'an': 4, 'ancestors': 5, 'and': 6, 'boar,': 7, 'breakfast': 8, 'covering': 9, 'for': 10, 'had': 11, 'hand': 12, 'in': 13, 'leaves': 14, 'like': 15, 'mastodons': 16, 'mine': 17, 'olympic': 18, 'or': 19, 'run': 20, 'skin,': 21, 'spear': 22, 'sprinter,': 23, 'their': 24, 'themselves': 25, 'tiger': 26, 'wild': 27, 'with': 28}
Now, let’s create one-hot encoding for them.
现在,让我们为它们创建一键编码。
Example output: This is how “yours” is represented.
输出示例:这就是“您的”的表示方式。
Yours [0. 1. 0. 0. 0. 0. 0. 0. 0. 0. 0. 0. 0. 0. 0. 0. 0. 0. 0. 0. 0. 0. 0. 0. 0. 0. 0. 0.]
一口热喀拉拉邦的例子 (One-hot keras example)
text_to_matrix is the method used to return one-hot encoding.
text_to_matrix是用于返回一键编码的方法。
You can see that, to represent a word, we are actually wasting a lot of memory to just set 0s(sparse matrix). These one-hot encodings also doesn’t reflect any relation between similar words. They are just representation of some word with ‘1’. Two similar words such as “accurate” and “exact” might be at very different positions in one-hot encodings.
您可以看到,为了表示一个单词,我们实际上是在浪费大量内存来仅将0设置为(稀疏矩阵)。 这些一键编码也不能反映相似词之间的任何关系。 它们只是某个带有“ 1”的单词的表示。 在“一键编码”中,两个类似的词(例如“准确”和“精确”)可能位于非常不同的位置。
What if we can represent a word with less space and have a meaning of its representation with which we can learn something.
如果我们可以用更少的空间来表示一个单词,并且具有可以用来学习某些东西的表示含义,该怎么办。
词嵌入 (Word Embeddings)
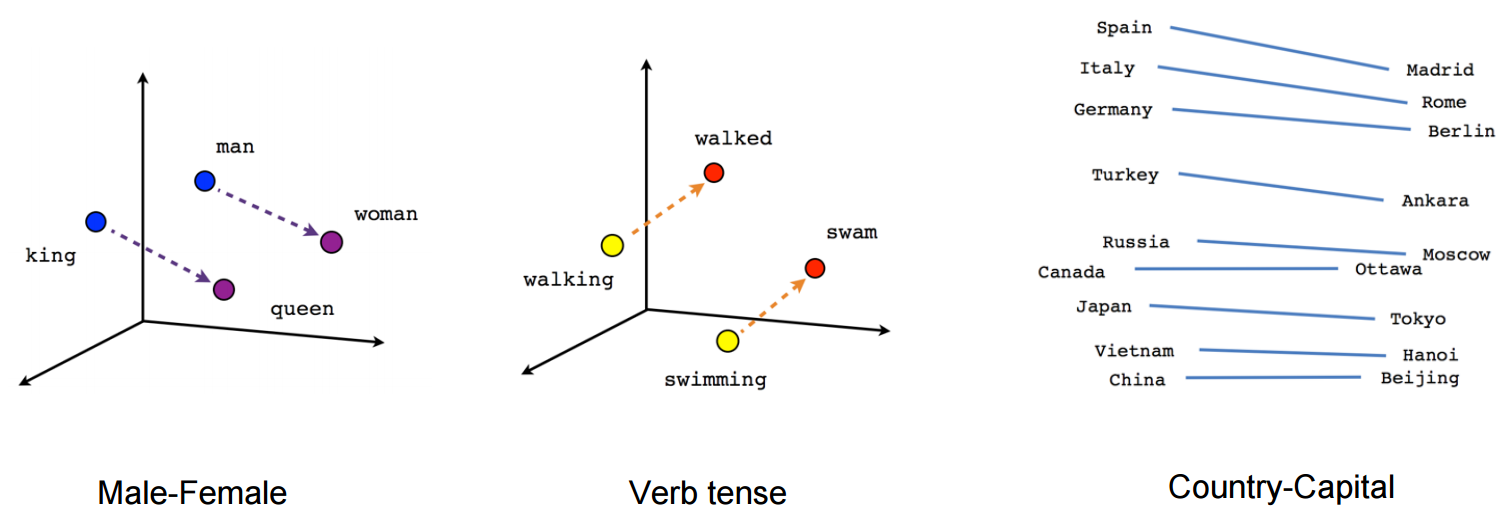
- Word embeddings also represent words in an array, not in the form of 0s and 1s but continuous vectors. 词嵌入还表示数组中的词,而不是0和1的形式,而是连续的向量。
- They can represent any word in few dimensions, mostly based on the number of unique words in our text. 它们可以在几个维度上表示任何单词,主要是基于文本中唯一单词的数量。
- They are dense, low dimensional vectors 它们是密集的低维向量
- Not hardcoded but are “learned” through data. 不进行硬编码,而是通过数据“学习”。
单词嵌入试图说什么? (What are word embeddings trying to say?)
- Geometric relationship between words in a word embeddings can represent semantic relationship between words. Words closer to each other have a strong relation compared to words away from each other. 词嵌入中的词之间的几何关系可以表示词之间的语义关系。 与彼此远离的单词相比,彼此靠近的单词具有很强的关系。
- Vectors/words closer to each other means the cosine distance or geometric distance between them is less compared to others. 向量/词彼此靠近意味着它们之间的余弦距离或几何距离小于其他向量。
- There could be vector “male to female” which represents the relation between a word and its feminine. That vector may help us in predicting “king” when “he” is used and “Queen” when she is used in the sentence. 可能存在向量“男对女”,代表一个单词与其女性味之间的关系。 当在句子中使用“他”时,该向量可以帮助我们预测“国王”,而在句子中使用“女王”时,该向量可以帮助我们预测。
单词嵌入的外观如何? (How word Embeddings look like?)
Below is a single row of embedding matrix representing the word ‘the’ in 100 dimensions from a text having 100K unique words.
下面是单行嵌入矩阵,表示具有100K个唯一单词的文本在100个维度中代表单词“ the” 。
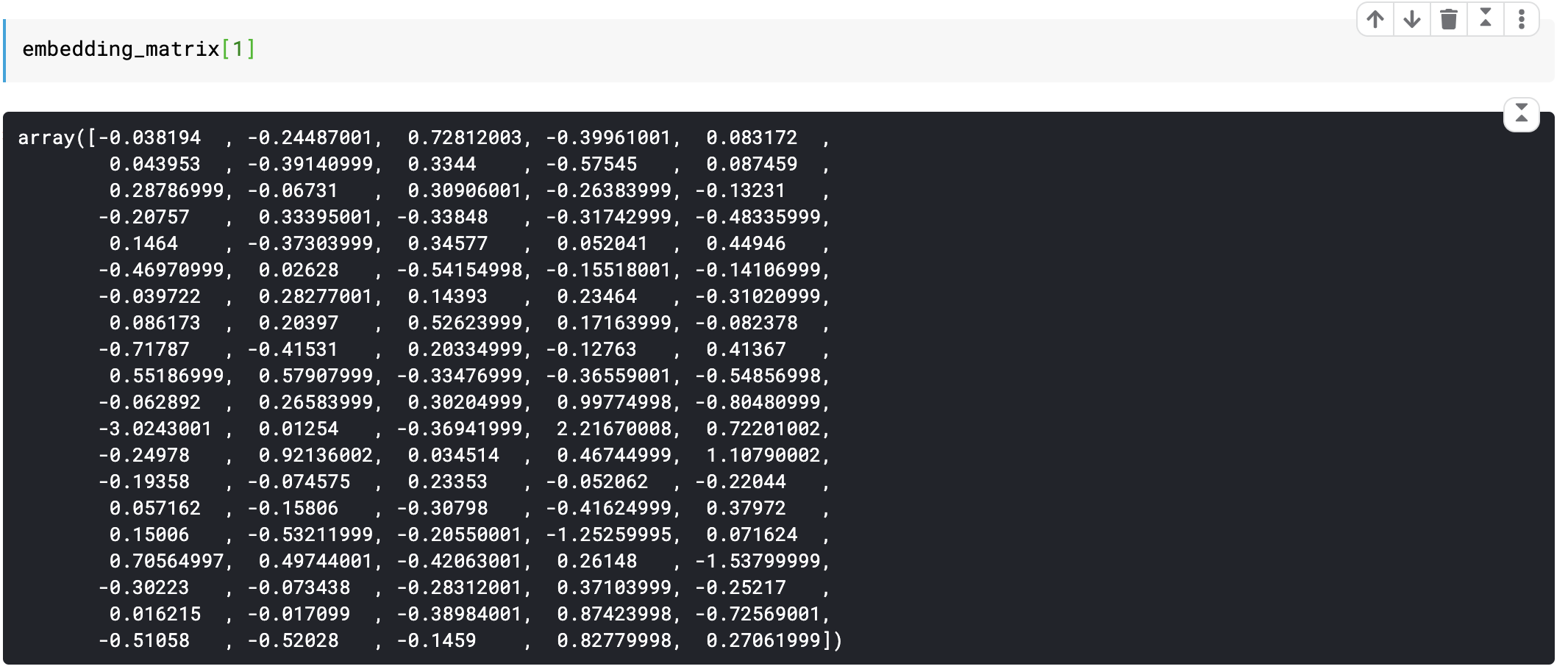
Such matrices are learned from data and can represent any text with millions of words in 100, 200, 1000 or more dimensions (The same would require 1MM dimensions if one-hot encoding is used).
此类矩阵可从数据中学习,并且可以表示100、200、1000或更多个维度中具有数百万个单词的任何文本(如果使用一键编码,则同样需要1MM维度)。
Let’s see how to create embeddings of our text in keras with a recurrent neural network.
让我们看看如何使用递归神经网络在keras中创建文本的嵌入。
将原始数据转换为嵌入的步骤如下: (Steps to follow to convert raw data to embeddings:)
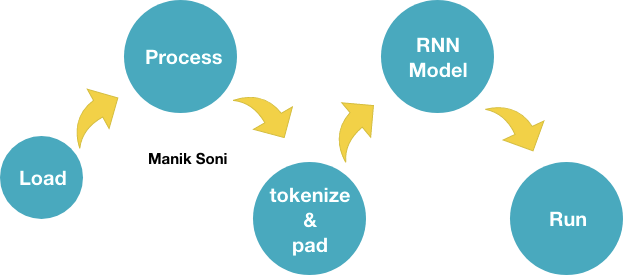
- Load text data in array. 将文本数据加载到数组中。
- Process the data. 处理数据。
- Convert the text to sequence and using the tokenizer and pad them with keras.preprocessing.text.pad_sequences method. 将文本转换为序列,并使用标记器,并使用keras.preprocessing.text.pad_sequences方法对其进行填充。
Initialise a model with Embedding layer of dimensions (max_words, representation_dimensions, input_size))
使用尺寸 (最大字数,表示尺寸 ,输入尺寸)的嵌入层初始化模型
max_words: It is the no. of unique words in your data
max_words :否。 数据中的独特单词
representation_dimension: It is the no. of dimensions in which you want to represent a word. Usually, it is number of (unique words)^(1/4)
presentation_dimension :否。 您想要代表一个单词的维度。 通常,它是(唯一词)^(1/4)的数量
input_size: size of your padded sequence(maxlen)
input_size:填充序列的大小( maxlen )
5 . Run the model
5。 运行模型
Let’s follow the above steps for IMDB raw data. All the code below is present in my Kaggle notebook.
让我们按照上述步骤处理IMDB原始数据。 我的Kaggle笔记本中包含以下所有代码。
步骤1.必要的进口 (Step 1. Necessary imports)
步骤2.加载文本数据。 (Step 2. Load the text data.)
loading the text data with pandas.
用熊猫加载文本数据。
步骤3:处理数据。 (Step 3: Process the data.)
Marking 1 for positive movie review and 0 for negative review.
标记1表示正面电影评论,标记0表示负面电影评论。
步骤4:创建和填充序列。 (Step 4: Creating and padding the sequence.)
Creating an instance of keras’s Tokenizer class and padding the sequence to ‘maxlen’.
创建keras的Tokenizer类的实例,并将序列填充到' maxlen '。
步骤5.初始化我们的模型 (Step 5. Initialise our model)
A simple recurrent neural network with embedding as first layer.
一个简单的以嵌入为第一层的递归神经网络。
步骤6:运行模型! (Step 6: Run the model!)
产出 (Outputs)
使用GRU: (With GRU:)
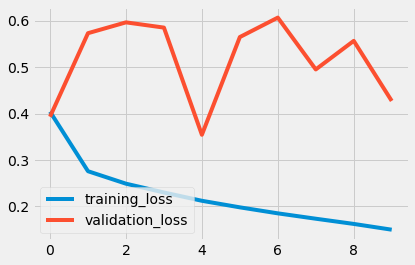
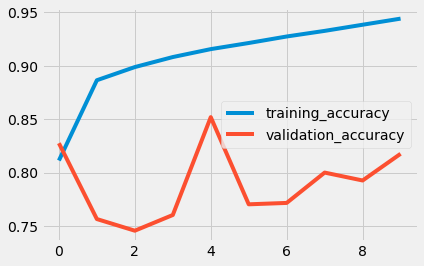
使用LSTM: (With LSTM:)
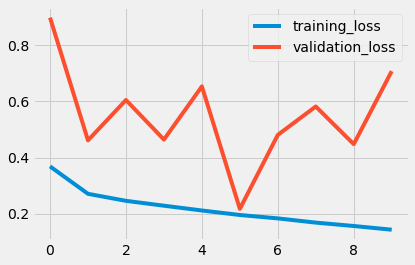
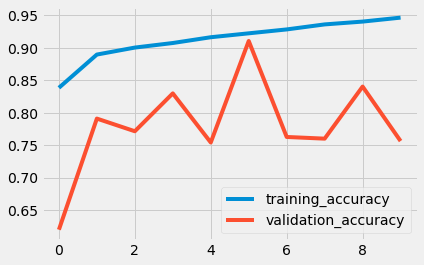
lstm预测单词