微软语音 文本到语音
Disclaimer: we will not train neural nets in this example but rather use pre-trained models.
免责声明:在此示例中,我们不会训练神经网络,而是使用预先训练的模型。
TLDR: clone my repository with --recurse-submodule
and download weights. Or skip the first section.
TLDR :使用--recurse-submodule
克隆我的存储库并下载weights 。 或跳过第一部分。
介绍 (Introduction)
I decided to build a small, efficient, and open-sourced version of a voice assistant. Mostly for fun. The basic setup for voice assistant would require three components, every of which is not limited in complexity inside.
我决定构建一个小型,高效且开源的语音助手版本。 主要是为了娱乐。 语音助手的基本设置将需要三个组件,每个组件的内部复杂性均不受限制。
- Speech to text — something that will understand what you say to it. 文字对话-可以理解您所说的话。
- Textual chatbot — something that will decide what to answer to you 文字聊天机器人-可以决定如何回答您的问题
- Text to speech — something that will make you hear the output instead of reading it. 文字转语音-使您听到而不是阅读输出的内容。
That’s it? Oh boy, you cannot imagine how much it is!
而已? 天哪,你无法想象它多少钱!
任何文本到语音框架的结构 (Structure of any Text-to-Speech framework)
The modern framework consists of a recurrent neural network that will convert text into spectrogram frames and another network that will convert spectrogram into sound. The first neural network is called Text-to-speech (not text-to-spectrogram, unfortunately), the second one is the vocoder.
现代框架包括一个递归神经网络,它将文字转换为声谱图框架;另一个网络将光谱图转换为声音。 第一个神经网络称为“文本到语音”(不幸的是,不是“文本到频谱图”),第二个神经网络是声码器。
Q: Why we just don’t cut audios letter by letter and combine them to make words?A: There is a technique like that and it was used not so long ago. The neural net approach produces better results and way easier to maintain.
问:为什么我们不逐个字母地剪切音频并将它们组合成单词? 答:有一种类似的技术,不久前才使用。 神经网络方法产生更好的结果,并且维护方法更容易。
我们为什么使用声码器 (Why do we use vocoder)
The problem is that we cannot train our neural net in one step. The sentence “London is the capital of Great Britain” has only 38 characters. But 2.5 seconds of the voice it corresponds to, will have far more data inside. To be exact, with the default sample rate of 44100Hz (to represent all 20kHz that we hear we need at least twice that number of measures in sound), it will have an array of 110250 numbers. It is almost 3000 times longer than the sentence.
问题在于我们不能一步一步地训练神经网络。 句子“伦敦是英国的首都”只有38个字符。 但是它所对应的语音的2.5秒将在其中包含更多数据。 确切地说,默认采样率为44100Hz(要表示我们听到的所有20kHz,我们至少需要声音数量的两倍),它将具有110250个数字数组。 它几乎比句子长3000倍。
For neural nets, shrinking space is easy but expanding is somewhat hard. For example, you can always find the sum of matrix given matrix, but finding a matrix given its sum is only possible if you know something about the original matrices. Good if they only consisted of the same numbers. As on the image on the left.
对于神经网络而言,缩小空间很容易,但是很难扩展。 例如,您总是可以找到给定矩阵的矩阵总和,但是只有在您了解原始矩阵的情况下,才能找到给定矩阵总和的矩阵。 如果它们仅包含相同的数字,则很好。 如左图所示。
So engineers came up with a solution for this. They used a representation of sound known as a spectrogram.
因此,工程师为此提出了一个解决方案。 他们使用声音的表示法称为频谱图。

On a spectrogram, each line represents some frequency and each column represents timeframe. This way each pixel shows how loud was sound at the given frequency at the given time. The average MEL-spectrogram is a matrix 80x200 for 2.5 seconds of speech. And in this case, we care about the length of the spectrogram which is 200. 200 is way closer to 38 characters that we need to represent in speech.
在频谱图上,每行代表某个频率,每列代表时间范围。 这样,每个像素可以显示在给定时间以给定频率发出的声音有多响。 平均MEL频谱图是一个80x200的矩阵,持续2.5秒的语音。 在这种情况下,我们关心的是声谱图的长度200。200更接近我们需要在语音中表示的38个字符。
The generation of a spectrogram from a wav file is an easy and fast process. That’s why it’s so commonly used. Converting it back is not so obvious, but there are many approaches to that.
从wav文件生成频谱图是一个简单而快速的过程。 这就是为什么它如此常用。 将其转换回原位不是很明显,但是有很多方法可以实现。
文字规范化 (Text normalization)
In order to make our speech synthesis pronounce words as it should, we need to normalize text as well. Definition by wikipedia says the following:
为了使语音合成按原样发音,我们还需要对文本进行规范化。 维基百科的定义如下:
As part of a text-to-speech (TTS) system, the text normalization component is typically one of the first steps in the pipeline, converting raw text into a sequence of words, which can then be passed to later components of the system, including word pronunciation, prosody prediction, and ultimately waveform generation.
作为文本语音转换 ( TTS )系统的一部分, 文本规范化组件通常是管道中的第一步,将原始文本转换为单词序列,然后可以将其传递给系统的后续组件,包括单词发音,韵律预测以及最终的波形生成。
In other words, it will make your “USA” and “$1.20” sound like “you-as-ay” and “wan dollar and twenty cents”. Normalization is crucial. And it’s almost an unlimited field for improvement.
换句话说,它将使您的“ USA”和“ $ 1.20”听起来像“ you-as-ay”和“ wan dollar and 20. cents”。 规范化至关重要。 而且这几乎是无限的改进领域。
让我们弄脏双手吧! (Let’s get our hands dirty!)
I will run an open-sourced framework called “Fastspeech”.
我将运行一个名为“ Fastspeech”的开源框架。
Why fastspeech? Well, because it fast.
为什么要快语? 好吧,因为它很快。
And it’s good too. My target device is CPU and I want it to run faster than real-time. These two constraints narrow the list of frameworks significantly.
这也很好。 我的目标设备是CPU,我希望它的运行速度快于实时。 这两个限制大大缩小了框架的范围。
Disclaimer 2: I want to warn you before you choose this framework. This framework is supposed to distill bigger boys like Google’s Tacotron. So you won’t be able to train it on your dataset right away. You will need to train Tacotron first, and use speech it produces to train this network.
免责声明2:在您选择此框架之前,我想警告您。 该框架应该能够提炼像Google的Tacotron这样的大男孩。 因此,您将无法立即在数据集上对其进行训练。 您将需要首先训练Tacotron,并使用其产生的语音来训练该网络。
Everything you need to know about fastspeech can be found in the abstract of original paper.
您需要了解的有关快速语音的所有信息都可以在原始论文的摘要中找到。
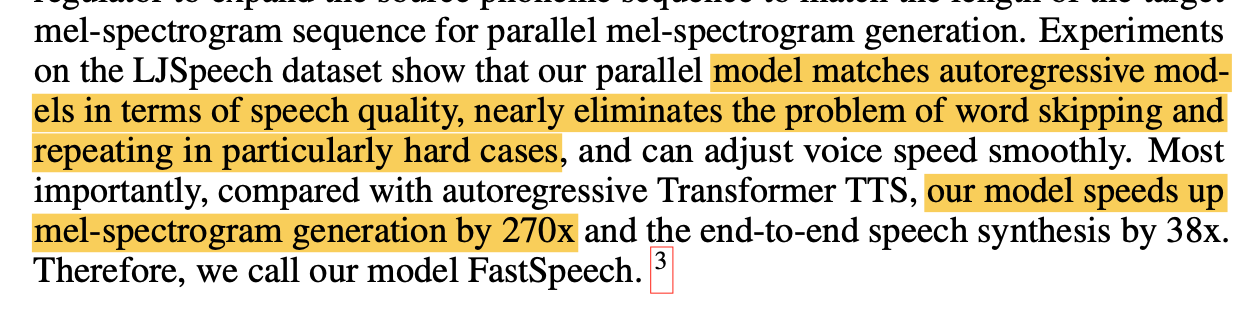
Sounds promising!
听起来很有前途!
A nice implementation of this paper was found here. Let’s clone it.
$ git clone https://github.com/xcmyz/FastSpeech
$ cd FastSpeech
$ pip3 install -r requirements.txt
Download weights from here and put them in a folder calledmodel_new
. You will need to create it inside yourFastSpeech
directory.
从此处下载权重并将其放在名为model_new
的文件夹中。 您将需要在FastSpeech
目录中创建它。
A quick tour around the project:
快速浏览该项目:
- eval.py — script you will run to run speech on predefined texts eval.py-您将运行的脚本以在预定义的文本上运行语音
- train.py — script you will run to train your network train.py-您将运行以训练网络的脚本
- text —the folder that has a text normalizer. It’s not the best, but enough for now. 文本-具有文本规范化器的文件夹。 这不是最好的,但到目前为止已经足够了。
- waveglow — you can delete this folder right away. waveglow-您可以立即删除此文件夹。
- model_new — place your weights here. model_new —在这里放置您的重量。
I will use MelGAN instead of waveglow. MelGAN sounds just as bad as waveglow on this dataset but at least it’s fast.
我将使用MelGAN代替waveglow。 在此数据集上,MelGAN听起来和waveglow一样糟糕,但至少它很快。
# Do it in your FastSpeech directory
$ git clone https://github.com/seungwonpark/melgan
Now let’s change waveglow onto melgan in our code. In order to do this, we need to add a new method to utils.py
:
现在,让我们在代码中将waveglow更改为melgan。 为此,我们需要向utils.py
添加一个新方法:
from melgan.model import generator
def get_melgan():
if not torch.cuda.is_available():
melgan = generator.Generator(hparams.num_mels)
checkpoint = torch.hub.load_state_dict_from_url('https://github.com/seungwonpark/melgan/releases/download/v0.3-alpha/nvidia_tacotron2_LJ11_epoch6400.pt', map_location="cpu")
melgan.load_state_dict(checkpoint["model_g"])
melgan.eval(inference=True)
else:
melgan = torch.hub.load('seungwonpark/melgan', 'melgan')
melgan = melgan.to(device)
return melgan
Now in eval.py
we will need to change the vocoder onto our new one:
现在在eval.py
我们需要将声码器更改为新的声码器:

Here’s a copy-pastable format:
这是可复制复制的格式:
if __name__ == "__main__":
# Test
melgan = utils.get_melgan()
parser = argparse.ArgumentParser()
parser.add_argument('--step', type=int, default=135000)
parser.add_argument("--alpha", type=float, default=1.0)
args = parser.parse_args()
model = get_DNN(args.step)
data_list = get_data()
for i, phn in enumerate(data_list):
mel, mel_cuda = synthesis(model, phn, args.alpha)
if not os.path.exists("results"):
os.mkdir("results")
waveform = melgan(mel.unsqueeze(0)).squeeze().detach().cpu().numpy()
audio.tools.save_audio(waveform, "results/"+str(args.step)+"_"+str(i)+".wav")
print("Done", i + 1)
s_t = time.perf_counter()
for i in range(100):
for _, phn in enumerate(data_list):
_, _, = synthesis(model, phn, args.alpha)
print(i)
e_t = time.perf_counter()
print((e_t - s_t) / 100.)
To get rid of GPU dependencies, find and replace .cuda()
with .to(device)
and specify location of weights (bold font) on load in function get_DNN
:
要摆脱对GPU的依赖关系,请在函数get_DNN
找到.cuda()
并将其替换为.cuda()
.to(device)
并在加载时指定权重(粗体)的位置:
model.load_state_dict(torch.load(os.path.join(hp.checkpoint_path, checkpoint_path), map_location=device)['model'])
Now we need to add save_audio
method to audio/tools.py
to match the code written. And a low-pass filter to get rid of high-frequency noise which we will definitely encounter.
现在,我们需要在audio/tools.py
添加save_audio
方法以匹配编写的代码。 还有一个低通滤波器可以消除我们一定会遇到的高频噪声。
from scipy import signal
def low_pass(audio):
sos = signal.butter(3, 7000, 'lp', fs=hparams.sampling_rate, output='sos')
return signal.sosfilt(sos, audio)
def save_audio(audio, out_filename, filter=True):
if filter:
audio = low_pass(audio)
audio_path = out_filename
write(audio_path, hparams.sampling_rate, audio)
Compare your results with my repository if something doesn’t work.
如果不起作用,请将您的结果与我的存储库进行比较。
Now try to run your synthesizer!
现在尝试运行您的合成器!
$ python3 eval.py
If it produces something the output below, go and check your results
folder, there would be your speech samples!
如果它在下面的输出中产生了某些东西,请检查您的results
文件夹,其中将有您的语音样本!

Now to make framework call more convenient, we will modify our eval file. I will implement it in object-oriented way as we have weights of neural nets that take some time to load.
现在,为了使框架调用更方便,我们将修改我们的eval文件。 由于神经网络的权重需要一些时间来加载,因此我将以面向对象的方式实现它。
class TTS:
def __init__(self, step=135000):
self.vocoder = utils.get_melgan()
self.model = get_DNN(step)
def get_spec(self, string, alpha=1.0):
text_norm = text.text_to_sequence(string, hp.text_cleaners)
with torch.no_grad():
mel, mel_cuda = synthesis(self.model, text_norm, alpha)
return mel
def inv_spec(self, mel):
waveform = self.vocoder(mel.unsqueeze(0)).squeeze().detach().cpu().numpy()
return waveform
def run(self, string, alpha=1.0):
mel = self.get_spec(string, alpha)
waveform = self.inv_spec(mel)
return waveform
To run synthesis on arbitrary sentence I added corresponding parameter to argparser.
为了对任意句子进行综合,我向argparser添加了相应的参数。
if __name__ == "__main__":
# Test
parser = argparse.ArgumentParser()
parser.add_argument('text', type=str)
parser.add_argument('--step', type=int, default=135000)
parser.add_argument("--alpha", type=float, default=1.0)
args = parser.parse_args()
tts = TTS(args.step)
waveform = tts.run(args.text, args.alpha)
if not os.path.exists("results"):
os.mkdir("results")
audio.tools.save_audio(waveform, "results/" + str(hash(args.text)) + ".wav")
Hasher on the filename will help to save the same audios into the same files. Now let’s run and see how it works.
文件名上的哈希器将有助于将相同的音频保存到相同的文件中。 现在开始运行,看看它是如何工作的。
$ python fastspeech.py "London is the capital of Great Britain"
故障排除 (Troubleshooting)
Produced sound is never good with open-sourced models. Let’s see what kind of problems we have to address and solve them.
使用开源模型产生的声音永远都不好。 让我们看看我们必须解决和解决什么样的问题。
第一个样本 (First sample)
This one is quite clear, though we hear some high-frequency noise. This noise is clearly a problem of the vocoder. To eliminate that, you need to use a more sophisticated vocoder. Authors of MelGAN were able to produce clear sound in their product. But they didn’t tell their secret sauce. I guess you will need to train the vocoder for longer. The authors of MelGAN trained it for 2 weeks on an Nvidia V100 — server-class GPU. It was the best available back then.
尽管我们听到了一些高频噪声,但这一点很清楚。 这种噪声显然是声码器的问题。 为了消除这种情况,您需要使用更复杂的声码器。 MelGAN的作者能够在其产品中产生清晰的声音。 但是他们没有告诉他们秘密的调味料。 我想您将需要训练声码器更长的时间。 MelGAN的作者在Nvidia V100(服务器级GPU)上进行了2周的培训。 那是当时最好的。
第二样本 (Second sample)
Words “Durian” and “Synthesis” were not in the training set. We can hear that they sound worse than the rest of the sentence. It’s definitely a problem of the fastspeech itself and called overfitting. Distillation doesn’t matter here as when we use Tacotron to produce dataset, we use the same words as in the training set.
训练集中没有单词“ Durian”和“ Synthesis”。 我们可以听到它们听起来比句子的其余部分更糟。 这绝对是fastspeech本身的问题,称为过拟合。 此处的蒸馏无关紧要,因为当我们使用Tacotron产生数据集时,我们使用与训练集中相同的词。
Note that when you train generative models, you generally want to overfit a little bit. Every TTS model is generative by definition.
请注意,在训练生成模型时,通常需要稍微拟合一点。 根据定义,每个TTS模型都是生成的。
第三样本 (Third sample)
This is a successful sample. The goal of the sample was to put intonation and pause correctly, which it did. This is the beauty of fastspeech — it rarely makes mistakes here.
这是一个成功的示例。 样本的目的是正确插入语调并暂停。 这就是快语的美–在这里很少犯错误。
第四样本 (Fourth sample)
ModUle. This is a problem of the normalizer. In some languages like in Russian, you cannot really use normalizer without word stress. But in English, it’s more or less obvious, so you can run TTS without word stress and come to terms with getting mistakes like this one.
模块。 这是归一化器的问题。 在某些语言(如俄语)中,没有单词重音就无法真正使用规范化器。 但是用英语来说,它或多或少是显而易见的,因此您可以在没有单词重音的情况下运行TTS,并且会遇到诸如此类的错误。
享受语音合成的乐趣! 还有更多文章。 (Have fun with speech synthesis! There are more articles to come.)
微软语音 文本到语音