2020年ai顶会时间表
Editor’s Note: AI is routinely cited as one of the biggest factors expected to disrupt business in the future — but how pervasive is Enterprise AI already? In the following post Roger Magoulas and Steve Swoyer interpret a December 2019 survey on the topic, analyzing the current landscape and the most common challenges to AI adoption.
编者注: 人工智能通常被认为是预计将来会扰乱业务的最大因素之一,但是企业AI的普及程度如何? 在接下来的文章中,Roger Magoulas和Steve Swoyer解释了有关该主题的2019年12月的一项调查,分析了当前的形势以及采用AI的最常见挑战。
Last year, when we felt interest in artificial intelligence (AI) was approaching a fever pitch, we created a survey to ask about AI adoption. When we analyzed the results, we determined the AI space was in a state of rapid change, so we eagerly commissioned a follow-up survey to help find out where AI stands right now. The new survey, which ran for a few weeks in December 2019, generated an enthusiastic 1,388 responses. The update sheds light on what AI adoption looks like in the enterprise — hint: deployments are shifting from prototype to production — the popularity of specific techniques and tools, the challenges experienced by adopters, and so on. There’s a lot to bite into here, so let’s get started. Key survey results:
去年,当我们对人工智能(AI)的兴趣日益升温时,我们进行了一项调查,询问AI的采用情况。 当我们分析结果时 ,我们确定AI空间处于快速变化的状态,因此我们急切地进行了一项后续调查,以帮助了解AI目前的状况。 新调查于2019年12月进行了几周,获得了1,388份热情洋溢的回复。 该更新揭示了AI在企业中的采用情况-提示:部署正从原型转移到生产-特定技术和工具的普及,采用者所面临的挑战等等。 这里有很多东西可以咬,所以让我们开始吧。 主要调查结果:
The majority (85%) of respondent organizations are evaluating AI or using it in production.1 Just 15% are not doing anything at all with AI.
大部分(85%)的受访组织正在评估AI或将其用于生产中。 1只有15%的人根本没有对AI做任何事情。
- More than half of respondent organizations identify as “mature” adopters of AI technologies: that is, they’re using AI for analysis or in production. 超过一半的受访组织将AI技术视为“成熟”的采用者:也就是说,他们将AI用于分析或生产。
- Supervised learning is the most popular ML technique among mature AI adopters, while deep learning is the most popular technique among organizations that are still evaluating AI. 监督学习是成熟的AI采用者中最流行的ML技术,而深度学习是仍在评估AI的组织中最流行的技术。
- Though a problem, the lack of ML and AI skills isn’t the biggest impediment to AI adoption. Almost 22% of respondents identified a lack of institutional support as the most significant issue. 尽管存在问题,但缺乏机器学习和AI技能并不是采用AI的最大障碍。 几乎22%的受访者认为缺乏机构支持是最重要的问题。
- Few organizations are using formal governance controls to support their AI efforts. 很少有组织使用正式的治理控制来支持其AI工作。
The takeaway: AI adoption is proceeding apace. Most companies that were evaluating or experimenting with AI are now using it in production deployments. It’s still early, but companies need to do more to put their AI efforts on solid ground. Whether it’s controlling for common risk factors — bias in model development, missing or poorly conditioned data, the tendency of models to degrade in production — or instantiating formal processes to promote data governance, adopters will have their work cut out for them as they work to establish reliable AI production lines.
要点:人工智能的采用正在Swift发展。 大多数评估或试验AI的公司现在都在生产部署中使用它。 现在还为时过早,但是公司需要做更多的事情才能将其AI努力置于坚实的基础上。 无论是控制常见风险因素(模型开发中的偏见,数据缺失或条件差,模型在生产中的退化趋势),还是实例化正式流程以促进数据治理,采用者都将在工作时为他们削减工作量。建立可靠的AI生产线。
分析:当今AI采用的状态 (Analysis: The State of AI Adoption Today)
More than half of respondent organizations are in the “mature” phase of AI adoption (using AI for analysis/production), while about one-third are still evaluating AI.2 This is close to a mirror image of last year’s AI survey results, when 54% of respondent organizations were evaluating AI and just 27% were in the “mature” adoption phase. This year, about 15% of respondent organizations are not doing anything with AI, down ~20% from our 2019 survey.
超过一半的受访组织处于采用AI的“成熟”阶段(使用AI进行分析/生产),而大约三分之一的组织仍在评估AI。 2这与去年的AI调查结果非常相似 ,当时54%的受访组织正在评估AI,而只有27%处于“成熟”采用阶段。 今年,约15%的受访组织没有对AI做任何事情,比我们2019年的调查下降了约20%。
The upshot is that 85% of organizations are using AI, and (of these) most are using it in production. It seems as if the experimental AI projects of 2019 have borne fruit. But what kind?
结果是85%的组织正在使用AI,(其中)大多数组织在生产中使用AI。 似乎2019年的实验性AI项目已取得成果。 那是什么
Figure 3. Where AI projects are being used within companies
图3.公司内部使用AI项目的位置
The bulk of AI use is in research and development — cited by just under half of all respondents — followed by IT, which was cited by just over one-third. (Respondents were encouraged to make multiple selections.) Another high-use functional area is customer service, with just under 30% of share. Two functional areas — marketing/advertising/PR and operations/facilities/fleet management — see usage share of about 20%. Clearly respondent organizations see the value of AI in a raft of different functional organizations, and the flat results from last year show a consistency to that pattern.
AI的大部分使用在研发中(被所有受访者中的将近一半引用),其次是IT,仅三分之一以上。 (鼓励受访者做出多项选择。)另一个高功能功能领域是客户服务,所占份额不到30%。 市场营销/广告/公关和运营/设施/车队管理这两个职能领域-占有约20%的使用份额。 显然,受访组织认为AI在众多不同职能组织中的价值,而去年取得的平坦结果表明了这种模式的一致性。
人工智能的普遍挑战 (Common Challenges to AI Adoption)
The acquisition and retention of AI-specific skills remains a significant impediment to adoption in most organizations. This year, slightly more than one-sixth of respondents cited difficulty in hiring/retaining people with AI skills as a significant barrier to AI adoption in their organizations. This is down, albeit slightly, from 2019, when 18% of respondents blamed an AI skills gap for lagging adoption.
在大多数组织中,获取和保留AI专用技能仍然是采用该技术的重要障碍。 今年,略多于六分之一的受访者将雇用/保留具有AI技能的人员的困难视为其组织采用AI的重大障碍。 与2019年相比,这有所下降,尽管有所下降,当时有18%的受访者将AI技能的差距归咎于落后的采用率。
Figure 4. Bottlenecks to AI adoption
图4.采纳AI的瓶颈
Believe it or not, a skills gap isn’t the biggest impediment to AI adoption. In 2020, as in 2019, a plurality of respondents — almost 22% — identified a lack of institutional support as the biggest problem. In both 2019 and 2020, the AI skills gap actually occupied the №3 slot; this year, it trailed “Difficulties in identifying appropriate business use cases,” which was cited by 20% of respondents. A more detailed look at the bottleneck data shows executives selecting an unsupportive culture less often (15%) than the practitioners and managers (23%) who responded to the survey.
信不信由你,技能差距并不是采用AI的最大障碍。 与2019年一样,2020年的受访者(将近22%)认为缺乏机构支持是最大的问题。 在2019年和2020年,人工智能技能差距实际上占据了№3位置; 今年,它落后于“确定合适的业务用例的难度”,有20%的受访者引用了该术语。 对瓶颈数据进行更详细的研究后发现,与回答调查的从业人员和管理人员(23%)相比,高管选择不支持文化的频率(15%)更少。
By a 2:1 margin, respondents in companies that are evaluating AI are much more likely to cite an unsupportive culture as the primary bulwark to AI adoption. This disparity is striking — and intriguing. Is it just the case that late-adopters are ipso facto more resistant to — less open to — AI? By contrast, AI adopters are about one-third more likely to cite problems with missing or inconsistent data. We saw in our “State of Data Quality in 2020” survey that ML and AI projects tend to surface latent or hidden data quality issues, with the result that organizations that are using ML and AI are more likely to identify issues with the quality or completeness of their data. The logic in this case partakes of garbage-in, garbage out: data scientists and ML engineers need quality data to train their models. Companies evaluating AI, by contrast, may not yet know to what extent data quality can create AI woes.
以2:1的比例,正在评估AI的公司中的受访者更有可能将无支持的文化作为采用AI的主要堡垒。 这种差距令人震惊,而且很有趣。 只是后来者就在事实上更能抵抗AI了-对AI的开放性更低了吗? 相比之下,AI使用者采用数据丢失或不一致的问题的可能性要高大约三分之一。 我们在“ 2020年数据质量状况”调查中看到,机器学习和人工智能项目倾向于发现潜在的或隐藏的数据质量问题,结果是使用机器学习和人工智能的组织更有可能确定质量或完整性方面的问题他们的数据。 在这种情况下,逻辑包括垃圾的输入,垃圾的输出 :数据科学家和ML工程师需要高质量的数据来训练他们的模型。 相比之下,评估AI的公司可能尚不知道数据质量会在多大程度上造成AI问题。
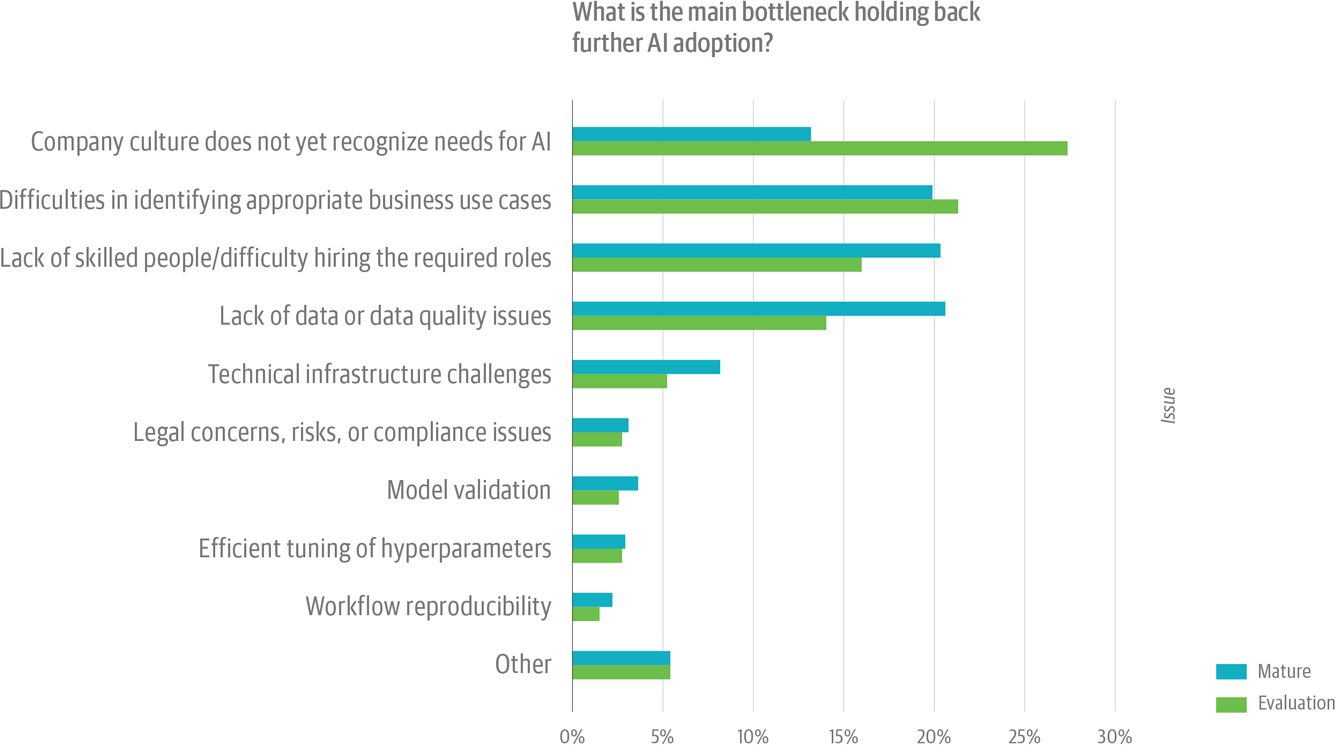
Figure 5. Bottlenecks to AI adoption with AI maturity level
图5. AI成熟度级别采用AI的瓶颈
学得更快。 深入挖掘。 看得更远。 (Learn faster. Dig deeper. See farther.)
Join the O’Reilly online learning platform. Get a free trial today and find answers on the fly, or master something new and useful.
加入O'Reilly在线学习平台。 立即获得免费试用版,即时找到答案,或者掌握一些新的有用的知识。
Roger Magoulas is a data science expert and was formerly VP of Radar at O’Reilly.
Roger Magoulas 是一位数据科学专家,曾担任O'Reilly的Radar副总裁。
Stephen Swoyer is a researcher and analyst with more than 20 years of industry experience. His research has focused on business intelligence (BI), data warehousing, and analytics, along with the larger data management segment, for 15 years. Swoyer’s work combines a deep interest in BI and analytic technology with an alertness to the needs and priorities of the people who must use (or, often as not, dis-use) it. He’s a recovering philosopher and occasionally guest lectures on Kierkegaard, Nietzsche, and Heidegger at both Penn State and Vanderbilt.
Stephen Swoyer 是一位拥有超过20年行业经验的研究人员和分析师。 他的研究专注于商业智能(BI),数据仓库和分析以及较大的数据管理领域,已有15年的历史。 Swoyer的工作结合了对BI和分析技术的浓厚兴趣,以及对必须使用(或经常不使用)它的人们的需求和优先级的警觉性。 他是一个正在恢复中的哲学家,偶尔在宾州州立大学和范德比尔特大学就基尔凯郭尔,尼采和海德格尔做客座演讲。
翻译自: https://medium.com/oreillymedia/ai-adoption-in-the-enterprise-2020-e2263f781647
2020年ai顶会时间表