pca人脸
In my previous article, we took a graphical approach to understand how Principal Component Analysis works and how it can be used for data compression. If you are new to this concept, I strongly suggest you read my previous article before proceeding. I have provided the link below:
在我的上一篇文章中,我们采用了一种图形化的方法来了解主成分分析的工作原理以及如何将其用于数据压缩。 如果您是这个概念的新手,我强烈建议您在继续之前阅读我的上一篇文章。 我提供了以下链接:
In this article, we will learn how PCA can be used to compress a real-life dataset. We will be working with Labelled Faces in the Wild (LFW), a large scale dataset consisting of 13233 human-face grayscale images, each having a dimension of 64x64. It means that the data for each face is 4096 dimensional (there are 64x64 = 4096 unique values to be stored for each face). We will reduce this dimension requirement, using PCA, to just a few hundred dimensions!
在本文中,我们将学习如何使用PCA压缩现实数据集。 我们将使用“野生标签脸”(LFW),这是一个大型数据集,包含13233张人脸灰度图像,每幅图像的尺寸为64x64 。 这意味着每个面的数据为4096维(每个面存储64x64 = 4096个唯一值)。 我们将使用PCA将尺寸要求降低到几百个尺寸!
介绍 (Introduction)
Principal component analysis (PCA) is a technique for reducing the dimensionality of datasets, exploiting the fact that the images in these datasets have something in common. For instance, in a dataset consisting of face photographs, each photograph will have facial features like eyes, nose, mouth. Instead of encoding this information pixel by pixel, we could make a template of each type of these features and then just combine these templates to generate any face in the dataset. In this approach, each template will still be 64x64 = 4096 dimensional, but since we will be reusing these templates (basis functions) to generate each face in the dataset, the number of templates required will be small. PCA does exactly this. Let’s see how!
主成分分析(PCA)是一种利用数据集中的图像有共同点的事实来降低数据集维数的技术。 例如,在由脸部照片组成的数据集中,每张照片将具有面部特征,例如眼睛,鼻子,嘴巴。 除了可以逐个像素地编码此信息外,我们可以为这些特征的每种类型制作一个模板,然后将这些模板组合在一起以生成数据集中的任何人脸。 在这种方法中,每个模板仍将是64x64 = 4096尺寸,但是由于我们将重复使用这些模板(基本函数)以生成数据集中的每个面,因此所需的模板数量将很少。 PCA正是这样做的。 让我们看看如何!
笔记本 (Notebook)
You can view the Colab Notebook here:
您可以在此处查看Colab笔记本:
数据集 (Dataset)
Let’s visualize some images from the dataset. You can see that each image has a complete face, and the facial features like eyes, nose, and lips are clearly visible in each image. Now that we have our dataset ready, let’s compress it.
让我们可视化数据集中的一些图像。 您可以看到每个图像都具有完整的脸部,并且在每个图像中都清晰可见了诸如眼睛,鼻子和嘴唇等面部特征。 现在我们已经准备好数据集,让我们对其进行压缩。

压缩 (Compression)
PCA is a 4 step process. Starting with a dataset containing n dimensions (requiring n-axes to be represented):
PCA是一个四步过程。 从包含n个维度的数据集开始(需要表示n个轴):
Step 1: Find a new set of basis functions (n-axes) where some axes contribute to most of the variance in the dataset while others contribute very little.
步骤1 :找到一组新的基函数( n轴),其中一些轴对数据集中的大部分方差有贡献,而另一些轴则贡献很小。
Step 2: Arrange these axes in the decreasing order of variance contribution.
步骤2 :以方差贡献的降序排列这些轴。
Step 3: Now, pick the top k axes to be used and drop the remaining n-k axes.
步骤3 :现在,选择要使用的前k个轴,然后删除其余的nk个轴。
Step 4: Now, project the dataset onto these k axes.
步骤4 :现在,将数据集投影到这k个轴上。
These steps are well explained in my previous article. After these 4 steps, the dataset will be compressed from n-dimensions to just k-dimensions (k<n).
我的上一篇文章对这些步骤进行了很好的解释。 经过这4个步骤,数据集将从n维压缩为仅k维( k < n )。
第1步 (Step 1)
Finding a new set of basis functions (n-axes), where some axes contribute to most of the variance in the dataset while others contribute very little, is analogous to finding the templates that we will combine later to generate faces in the dataset. A total of 4096 templates, each 4096 dimensional, will be generated. Each face in the dataset can be represented as a linear combination of these templates.
找到一组新的基函数( n轴),其中一些轴对数据集中的大部分方差有贡献,而另一些轴所占的比例很小,这类似于查找我们稍后将组合以在数据集中生成人脸的模板。 总共将生成4096个模板,每个4096维。 数据集中的每个面Kong都可以表示为这些模板的线性组合。
Please note that the scalar constants (k1, k2, …, kn) will be unique for each face.
请注意,每个面的标量常数(k1,k2,…,kn)将是唯一的。
第2步 (Step 2)
Now, some of these templates contribute significantly to facial reconstruction while others contribute very little. This level of contribution can be quantified as the percentage of variance that each template contributes to the dataset. So, in this step, we will arrange these templates in the decreasing order of variance contribution (most significant…least significant).
现在,这些模板中的一些对面部重构的贡献很大,而其他模板则贡献很少。 这种贡献水平可以量化为每个模板对数据集贡献的方差百分比。 因此,在此步骤中,我们将按方差贡献的降序排列这些模板(最高有效…最低有效)。
第三步 (Step 3)
Now, we will keep the top k templates and drop the remaining. But, how many templates shall we keep? If we keep more templates, our reconstructed images will closely resemble the original images but we will need more storage to store the compressed data. If we keep too few templates, our reconstructed images will look very different from the original images.
现在,我们将保留前k个模板,并删除其余的模板。 但是,我们应该保留多少个模板? 如果我们保留更多的模板,我们的重建图像将与原始图像非常相似,但是我们将需要更多的存储空间来存储压缩数据。 如果我们保留的模板太少,则重建的图像看起来将与原始图像有很大不同。
The best solution is to fix the percentage of variance that we want to retain in the compressed dataset and use this to determine the value of k (number of templates to keep). If we do the math, we find that to retain 99% of the variance, we need only the top 577 templates. We will save these values in an array and drop the remaining templates.
最好的解决方案是固定我们要保留在压缩数据集中的方差百分比,并用它来确定k的值(要保留的模板数量)。 如果我们做数学运算,我们发现要保留99%的方差,我们只需要前577个模板。 我们将这些值保存在数组中,然后删除其余模板。
Let’s visualize some of these selected templates.
让我们可视化其中一些选定的模板。

Please note that each of these templates looks somewhat like a face. These are called as Eigenfaces.
请注意,这些模板中的每个模板看起来都有些像脸。 这些称为本征面。
第4步 (Step 4)
Now, we will construct a projection matrix to project the images from the original 4096 dimensions to just 577 dimensions. The projection matrix will have a shape (4096, 577), where the templates will be the columns of the matrix.
现在,我们将构建一个投影矩阵,将图像从原始的4096维投影到只有577维。 投影矩阵将具有形状(4096,577) ,其中模板将是矩阵的列。
Before we go ahead and compress the images, let’s take a moment to understand what we really mean by compression. Recall that the faces can be generated by a linear combination of the selected templates. As each face is unique, every face in the dataset will require a different set of constants (k1, k2, …, kn) for the linear combination.
在继续压缩图像之前,让我们花一点时间来理解压缩的真正含义。 回想一下,可以通过所选模板的线性组合来生成面。 由于每个面都是唯一的,因此数据集中的每个面对于线性组合都将需要一组不同的常数(k1,k2,…,kn)。
Let’s start with an image from the dataset and compute the constants (k1, k2, …, kn), where n = 577. These constants along with the selected 577 templates can be plugged in the equation above to reconstruct the face. This means that we only need to compute and save these 577 constants for each image. Instead of doing this image by image, we can use matrices to compute these constants for each image in the dataset at the same time.
让我们从数据集中的图像开始,计算常数(k1,k2,…,kn),其中n =577。这些常数以及所选的577个模板可以插入上面的等式中以重建脸部。 这意味着我们只需要为每个图像计算并保存这577个常量。 代替逐个图像地处理,我们可以使用矩阵同时为数据集中的每个图像计算这些常数。
Recall that there are 13233 images in the dataset. The matrix compressed_images contains the 577 constants for each image in the dataset. We can now say that we have compressed our images from 4096 dimensions to just 577 dimensions while retaining 99% of the information.
回想一下,数据集中有13233张图像。 矩阵compressed_images包含数据集中每个图像的577个常量。 现在我们可以说,我们已将图像从4096尺寸压缩到了577尺寸,同时保留了99%的信息。
压缩率 (Compression Ratio)
Let’s calculate how much we have compressed the dataset. Recall that there are 13233 images in the dataset and each image is 64x64 dimensional. So, the total number of unique values required to store the original dataset is 13233 x 64 x 64 = 54,202,368 unique values.
让我们计算一下我们对数据集的压缩量。 回想一下,数据集中有13233张图像,每个图像都是64x64尺寸。 因此,存储原始数据集所需的唯一值总数为13233 x 64 x 64 = 54,202,368个唯一值 。
After compression, we store 577 constants for each image. So, the total number of unique values required to store the compressed dataset is 13233 x 577 = 7,635,441 unique values. But, we also need to store the templates to reconstruct the images later. Therefore, we also need to store 577 x 64 x 64 = 2,363,392 unique values for the templates. Therefore, the total number of unique values required to store the compressed dataset is7,635,441 + 2,363,392 = 9,998,883 unique values.
压缩后,我们为每个图像存储577个常数。 因此,存储压缩数据集所需的唯一值总数为13233 x 577 = 7,635,441个唯一值。 但是,我们还需要存储模板以稍后重建图像。 因此,我们还需要为模板存储577 x 64 x 64 = 2,363,392个唯一值。 因此,存储压缩数据集所需的唯一值总数为7,635,441 + 2,363,392 = 9,998,883个唯一值 。
We can calculate the percentage compression as:
我们可以将压缩百分比计算为:
重建图像 (Reconstruct the Images)
The compressed images are just arrays of length 577 and can’t be visualized as such. We need to reconstruct it back to 4096 dimensions to view it as an array of shape (64x64). Recall that each template has a dimension of 64x64 and that each constant is a scalar value. We can use the equation below to reconstruct any face in the dataset.
压缩的图像只是长度为577的数组,因此无法可视化。 我们需要将其重构回4096尺寸,以将其视为形状数组(64x64)。 回想一下,每个模板的尺寸为64x64,并且每个常量都是标量值。 我们可以使用下面的公式重建数据集中的任何面Kong。
Again, instead of doing this image by image, we can use matrices to reconstruct the whole dataset at once, with of course a loss of 1% variance.
同样,我们可以使用矩阵一次重建整个数据集,而不是逐个图像地进行处理,当然损失1%。
Let’s look at some reconstructed faces.
让我们看一些重构的面Kong。


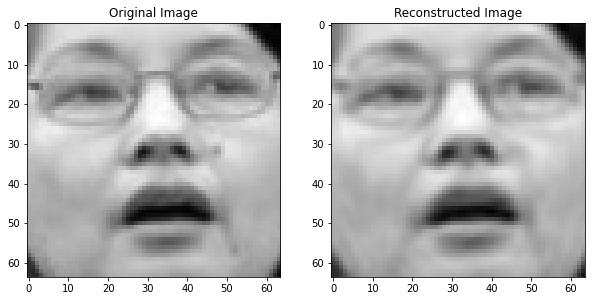
We can see that the reconstructed images have captured most of the relevant information about the faces and the unnecessary details have been ignored. This is an added advantage of data compression, it allows us to filter unnecessary details (and even noise) present in the data.
我们可以看到,重建的图像已经捕获了有关面部的大多数相关信息,而不必要的细节已被忽略。 这是数据压缩的另一个优点,它使我们可以过滤数据中存在的不必要的细节(甚至噪声)。
那是所有人! (That’s all folks!)
If you made it till here, hats off to you! In this article, we learnt how PCA can be used to compress Labelled Faces in the Wild (LFW), a large scale dataset consisting of 13233 human-face images, each having a dimension of 64x64. We compressed this dataset by over 80% while retaining 99% of the information. You can view my Colab Notebook to understand the code. I encourage you to use PCA to compress other datasets and comment the compression ratio that you get.
如果您做到了这里,就向您致敬! 在本文中,我们了解了如何使用PCA来压缩野外标记的面部(LFW),LFW是由13233张人脸图像组成的大规模数据集,每张图像的尺寸为64x64 。 我们将此数据集压缩了80%以上,同时保留了99%的信息。 您可以查看我的Colab笔记本以了解代码。 我鼓励您使用PCA压缩其他数据集并注释所获得的压缩率。
If you have any suggestions, please leave a comment. I write articles regularly so you should consider following me to get more such articles in your feed.
如果您有任何建议,请发表评论。 我会定期撰写文章,因此您应该考虑关注我,以便在您的供稿中获取更多此类文章。
If you liked this article, you might as well love these:
如果您喜欢这篇文章,您可能会喜欢这些:
Visit my website to learn more about me and my work.
访问我的网站以了解有关我和我的工作的更多信息。
翻译自: https://towardsdatascience.com/face-dataset-compression-using-pca-cddf13c63583
pca人脸