基于端到端 语音合成
Healthcare has been one of the countless beneficiaries of the revolutionary advances that widespread computing has brought. Fast, efficient data organization, storage, and access that have greatly sped up the medical enterprise, yet many low hanging fruits remain hanging. Chief among those is the increased application of technologies that can process speech.
医疗保健已成为广泛计算带来的革命性进步的无数受益者之一。 快速,高效的数据组织,存储和访问极大地加快了医疗企业的发展速度,但仍有许多悬而未决的成果。 其中最主要的是增加了可以处理语音的技术的应用。
In this post, we’ll share with you how speech technology can improve healthcare in the three following ways. (1) Speech technology can be used to improve the efficiency of medical personnel. (2) Voice-based interactions for patients and hospital visitors can simplify access to information and speed up the registration process. Finally, (3) voice signal analysis can be used for earlier diagnosis and to help track the changes in medical conditions over time.
在本文中,我们将与您分享语音技术如何通过以下三种方式改善医疗保健。 (1)语音技术可用于提高医务人员的效率。 (2)患者和医院访客的基于语音的交互可以简化信息访问并加快注册过程。 最后,(3)语音信号分析可用于早期诊断并帮助跟踪医疗状况随时间的变化。
Let’s explore these three ways in detail.
让我们详细探讨这三种方式。
帮助医师 (Helping physicians)
Research has shown that medical personnel unsurprisingly spend a significant amount of their time interacting with their patients using their voice, but, surprisingly, they can spend even more time documenting these physician-patient sessions (Arndt et al., 2017). Taking notes, creating and updating medical records, and so on, take up a considerable amount of time. Physicians often make use of any resources available to them to speed up this process. For example, a physician can record the session with a patient to be able to revisit details of it later or employ a human scribe, that can either transcribe the session records or document the session directly as it unfolds. It is no secret, however, that the requirement of additional personnel with non-trivial training makes these options somewhat luxurious and unreliable.
研究表明,医务人员花费大量时间与他们的声音与患者互动并不奇怪,但令人惊讶的是,他们可以花更多的时间记录这些医患会议的内容(Arndt et al。,2017) 。 做笔记,创建和更新病历等都需要花费大量时间。 医师通常会利用他们可用的任何资源来加快此过程。 例如,医师可以记录与患者的会话,以便以后可以重访它的细节或雇用人类抄写员,后者可以抄录会话记录或在会话展开时直接记录该会话。 但是,秘密培训的额外人员需求使得这些选择有些奢侈和不可靠,这已不是秘密。
The first and most obvious remedy for this time sink is to use a digital dictation device including a speech-to-text system (introduced in our previous post) that automatically processes the recordings and prepares a draft transcription of the session, which the physician or a trained scribe can quickly post-edit. Speech-to-text can also be used to transcribe the dictated post-session recordings made by physicians. Processing medical speech is not without its challenges, though. While there are multiple products on the market that serve this use case already, it is useful to look at the challenges these systems are facing.
解决此问题的第一个也是最明显的补救方法是使用数字听写设备,该设备包括语音转文本系统(在我们之前的文章中介绍),该系统会自动处理录音并准备会议的录音草稿,医生或受过训练的抄写员可以快速进行后期编辑。 语音转文字还可以用于转录医师录制的指定的会后录音。 但是,处理医学演讲并非没有挑战。 尽管市场上已经有多种产品可以满足这种用例的需求,但是查看这些系统所面临的挑战很有用。
Like many other non-professional recordings, the audio quality in healthcare is very inconsistent. There’s often a background noise of varying amounts, and the speakers are also frequently unclear, specifically when speaking too fast or too far from the microphone. Good audio quality is usually not the primary concern of neither the patient nor the physician during a session. Thus, any speech recognition system that has been trained on clean audio will probably have a bad time trying to make sense of the vast possible range of acoustic conditions in medical recordings. Fortunately, special speech-to-text training techniques and data preparation can help create noise-robust systems that can provide much better recognition quality with such inconsistent inputs.
像许多其他非专业录音一样,医疗保健中的音频质量也非常不一致。 通常会有不同数量的背景噪音,而且扬声器也经常不清楚,特别是当说话速度太快或离麦克风太远时。 在会议期间,良好的音频质量通常不是患者和医生都不会关注的主要问题。 因此,任何已经接受过清晰音频训练的语音识别系统都可能会花费大量时间来尝试理解医疗记录中的声学条件。 幸运的是,特殊的语音到文本训练技术和数据准备可以帮助创建噪声健壮的系统,这些系统在输入不一致的情况下可以提供更好的识别质量。
Also, medical language is highly specialized, with a vast vocabulary, acronyms and other forms of expression not found in common language. Commercial speech-to-text systems are often trained to cover as many application domains as possible. Therefore, they are called general domain systems. Using a general domain system on a narrow domain like the medical one often results in a noticeable drop in recognition accuracy. Fortunately, we can use domain adaptation to adjust our general domain speech-to-text system to a narrower domain or, given enough data, even train a domain-specific system. These systems trade off accuracy on the general domain for much better accuracy on the domain of interest. Given the vast size of medical vocabulary, it can sometimes make sense to focus on a particular medical specialisation such as, e.g., radiology or dermatology.
同样,医学语言是高度专业化的,具有大量的词汇,首字母缩写词和其他表达形式,这些语言是普通语言所没有的。 商业语音转文本系统通常经过培训,以覆盖尽可能多的应用领域。 因此,它们被称为通用域系统。 在医学等狭窄领域上使用通用领域系统通常会导致识别准确性明显下降。 幸运的是,我们可以使用域自适应将我们的通用域语音转文本系统调整为一个较窄的域,或者在给定足够数据的情况下,甚至可以训练特定于域的系统。 这些系统会权衡通用域的精度,以达到所关注域更好的精度。 鉴于医学词汇量巨大,有时将重点放在特定医学专长上是有意义的,例如放射学或皮肤病学。
While speech-to-text is a significant boost on its own, we can make further improvements by building on top of it. We can use speech-to-text as a stepping stone to build smarter downstream applications that can deal with the recognised text in a useful manner. For example, it would be useful to extract structured information from the raw transcript, and try to prepare draft medical records, which often follow a regular structure. Spoken language understanding tools (also introduced in our previous post) can be used to extract information from raw transcripts, starting from recognising highly regular patterns in the text such as medicine dosage and standardised measurements, to classifying and extracting more nebulous entities such as medical history, symptom descriptions, a chief complaint by the patient, etc. The extracted structural information can then be used to prepare the actual record drafts, a template where the physician must fill in the missing pieces and check the rest.
虽然语音转文字本身是一个巨大的进步,但我们可以在此基础上进一步改进。 我们可以使用语音转文本作为垫脚石,构建更智能的下游应用程序,以有用的方式处理已识别的文本。 例如,从原始成绩单中提取结构化信息,并尝试准备通常遵循常规结构的病历草案将非常有用。 可以使用口语理解工具(也可以在我们以前的文章中介绍)可以从原始成绩单中提取信息,从识别文本中高度规则的模式(例如药物剂量和标准化测量)到分类和提取更模糊的实体(例如病史) ,症状描述,患者的主诉等。提取的结构信息可用于准备实际的记录草稿,医生必须在其中填写缺失的部分并检查其余部分的模板。
对患者和访客的协助 (Assistance for patients and visitors)
It’s not only doctors and other medical personnel that speech technologies can help. A clever combination of speech recognition, speech synthesis and chatbot technologies can result in a more useful virtual assistant in hospitals, which is capable of helping people with common questions and problems. Eventually, this can optimise the workload of hospital personnel and reduce the time people have to wait in queues for information and registration. Such a hospital assistant can, for example, help with directions or guide through the proper hospital processes for getting to where the visitor or patient has or wants to be.
语音技术不仅可以帮助医生和其他医务人员。 语音识别,语音合成和聊天机器人技术的巧妙结合可以在医院中提供更有用的虚拟助手,从而能够帮助有常见问题的人。 最终,这可以优化医院人员的工作量,并减少人们在队列中等待信息和注册的时间。 这样的医院助手可以,例如,帮助指导或指导适当的医院程序,以到达访客或患者想要或想要去的地方。
Beyond procedural help in the hallways and corridors of a hospital, speech technologies can also have a positive impact on the quality of life of patients with disabilities. Speech recognition and speech synthesis together can provide a practical substitute for the mostly visual interface of a modern computer, enabling access to many of the benefits of modern computing (Hawley, 2002). Among the benefits, we can mention the example of a person with vision impairments who can enjoy voice feedback from a computer relaying the information that would be traditionally presented on the screen. Another example can be a person with limited mobility who can interact with and issue speech commands to modern virtual assistants, which can automatically perform a range of useful tasks, thanks to modern connectivity enabled household objects.
除了在医院的走廊和走廊中提供程序上的帮助外,语音技术还可以对残疾患者的生活质量产生积极影响。 语音识别和语音合成一起可以为现代计算机的大部分视觉界面提供实用的替代,从而能够获得现代计算机的许多好处(Hawley,2002) 。 在这些好处中,我们可以举出一个有视力障碍的人的例子,该人可以享受来自计算机的语音反馈,该计算机可以中继传统上显示在屏幕上的信息。 另一个例子是行动不便的人,他可以与现代虚拟助手互动并向其发出语音命令,这要归功于具有现代连通性的家用对象,该虚拟助手可以自动执行一系列有用的任务。
Most of these helpful use cases require integration between speech technology, which can provide voice interfaces, and the actual systems doing something useful, that can’t yet be accessed by either the visitor or the patient. While there are some existing integrations out there today, the number of research and commercial interests in this space should ensure that we’ll see such applications increasingly often in the future.
这些有用的用例中的大多数都需要语音技术与可以提供语音接口的实际系统之间的集成,而实际系统正在做一些有用的事情,访客或患者都无法访问。 尽管今天有一些现有的集成,但是在这个领域中的研究和商业利益的数量应该确保我们将来会越来越频繁地看到这种应用。
诊断与分析 (Diagnosis and analysis)
The frontier of speech technology in health goes beyond assistance. A highly active and perspective area of speech technology research is diagnosing various physical and mental disorders that affect patient’s speech and other vocal expressions. A diverse set of technologies is used for diagnostic purposes, and a significant part of them do not only consider the linguistic contents of speech, i.e., what is being said, but pay more attention to its paralinguistic features, i.e., how it’s being said. Both the contents and the manner of speech can provide important clues to determine a more accurate diagnosis.
语音技术在健康领域的前沿已超越了援助。 语音技术研究的高度活跃和具前景的领域正在诊断影响患者语音和其他声音表达的各种身体和精神疾病。 各种各样的技术都用于诊断目的,其中很大一部分不仅考虑语音的语言内容(即正在说的是什么),还更加注意其副语言功能(即如何说的)。 内容和讲话方式都可以提供重要的线索来确定更准确的诊断。
Quite obviously, speech technologies can be used to diagnose, classify and track speech disorders. These technologies can be used to identify acute speech disorders establishing initial diagnosis without the need for expensive and potentially painful medical procedures. Likewise, keeping track of how pronunciation changes over time can help keep a non-perceptual record of how a disorder is progressing or receding. Providing or improving diagnosis by using just an audio recording does not require expensive medical equipment. Adding to this, it helps avoid subjective perceptual judgments by the clinician and, most importantly for the patient, it is painless and easy (Wu et al., 2018).
很明显,语音技术可用于诊断,分类和跟踪语音障碍。 这些技术可用于识别建立初始诊断的急性言语障碍,而无需昂贵且可能痛苦的医疗程序。 同样,跟踪发音随时间变化的方式可以帮助保持对疾病如何进展或消退的非感知记录。 仅使用音频记录来提供或改善诊断不需要昂贵的医疗设备。 除此之外,它有助于避免临床医生的主观感知判断,并且对患者而言最重要的是,它无痛且容易(Wu等人,2018) 。
Similar methods can be applied to track and provide early detection of problems during child development, where an early diagnosis of a speech disorder can help provide effective early intervention to reduce potential problems later. One simple implementation would be a convenient mobile application that a parent can use to record their child speaking a set of carefully chosen benchmark words, which can then be analysed and compared to a set of ground-truth recordings from known healthy recordings (Kothalkar et al., 2018).
类似的方法可用于跟踪和提供儿童发育过程中的问题的早期检测,其中语音障碍的早期诊断可帮助提供有效的早期干预,以减少以后的潜在问题。 一个简单的实现是便捷的移动应用程序,父母可以使用它来记录他们的孩子说出一组精心选择的基准词,然后可以对其进行分析,并将其与来自已知健康记录的一系列真实记录进行比较(Kothalkar等人。,2018) 。
Speech signal analysis could also prove itself useful for early diagnosis of neurodegenerative conditions, some of which are tricky to diagnose early as the symptoms are subtle and could be explained by several other conditions. Prominent examples, where speech signal analysis has shown promise in early detection are amyotrophic lateral sclerosis (An et al., 2018), Parkinson’s disease (Tsanas et al., 2012) and brain injury (Falcone et al., 2013). Many of the markers used today rely on self-assessment or performance of tasks that are judged perceptually by a human observer, but these conditions affecting the brain usually become noticeable to a human only after significant brain damage has already occurred. Statistical analysis of speech recordings, however, has shown to be more sensitive than human ear to early symptoms (An et al., 2018).
语音信号分析还可以证明自己对神经退行性疾病的早期诊断很有用,其中一些症状很难诊断,因为症状很细微,并且可以由其他几种疾病进行解释。 语音信号分析在早期发现中显示出希望的突出例子是肌萎缩性侧索硬化(An等,2018) ,帕金森氏病(Tsanas等,2012)和脑损伤(Falcone等,2013) 。 当今使用的许多标记物都依赖于人类观察者在感知上判断的自我评估或任务的执行,但是影响大脑的这些条件通常只有在严重的脑部损伤已经发生之后才对人类显而易见。 然而,语音记录的统计分析已显示比人耳对早期症状更敏感(An等人,2018) 。
It has been shown that even subtle mental disorders can be analysed using both the contents of patient’s speech and the manner of speech (Cummins et al., 2015). For example, research shows that depression notably affects the patient’s vocabulary, and this change can be statistically measured to bolster the clinician’s confidence. On the other hand, subtle pronunciation differences can also betray the speaker’s mood and state of mind to not just a perceptive human listener, but also to statistical machine analysis.
已经表明,即使是细微的精神障碍,也可以使用患者的言语内容和言语方式进行分析(Cummins等人,2015) 。 例如,研究表明,抑郁症会显着影响患者的词汇量,可以通过统计学方法测量这种变化以增强临床医生的信心。 另一方面,细微的发音差异也可能使说话者的情绪和心态不仅向听觉敏锐的听众出示,而且还会向统计机器分析出卖。
While many of these methods promise early, easy and, most importantly, objective diagnosis for a whole range of medical conditions, currently they are also limited to mostly research laboratories and curated medical datasets. In other words, it’s still mostly the future, but early signs give us cautious hope that these technologies might soon deliver substantial real benefits for doctors and patients.
尽管这些方法中的许多方法都有望对整个医学状况进行早期,简便且最重要的是客观诊断,但目前它们还仅限于大多数研究实验室和精选的医学数据集。 换句话说,这仍然主要是未来,但是早期迹象给我们谨慎的希望,这些技术可能很快为医生和患者带来实质性的好处。
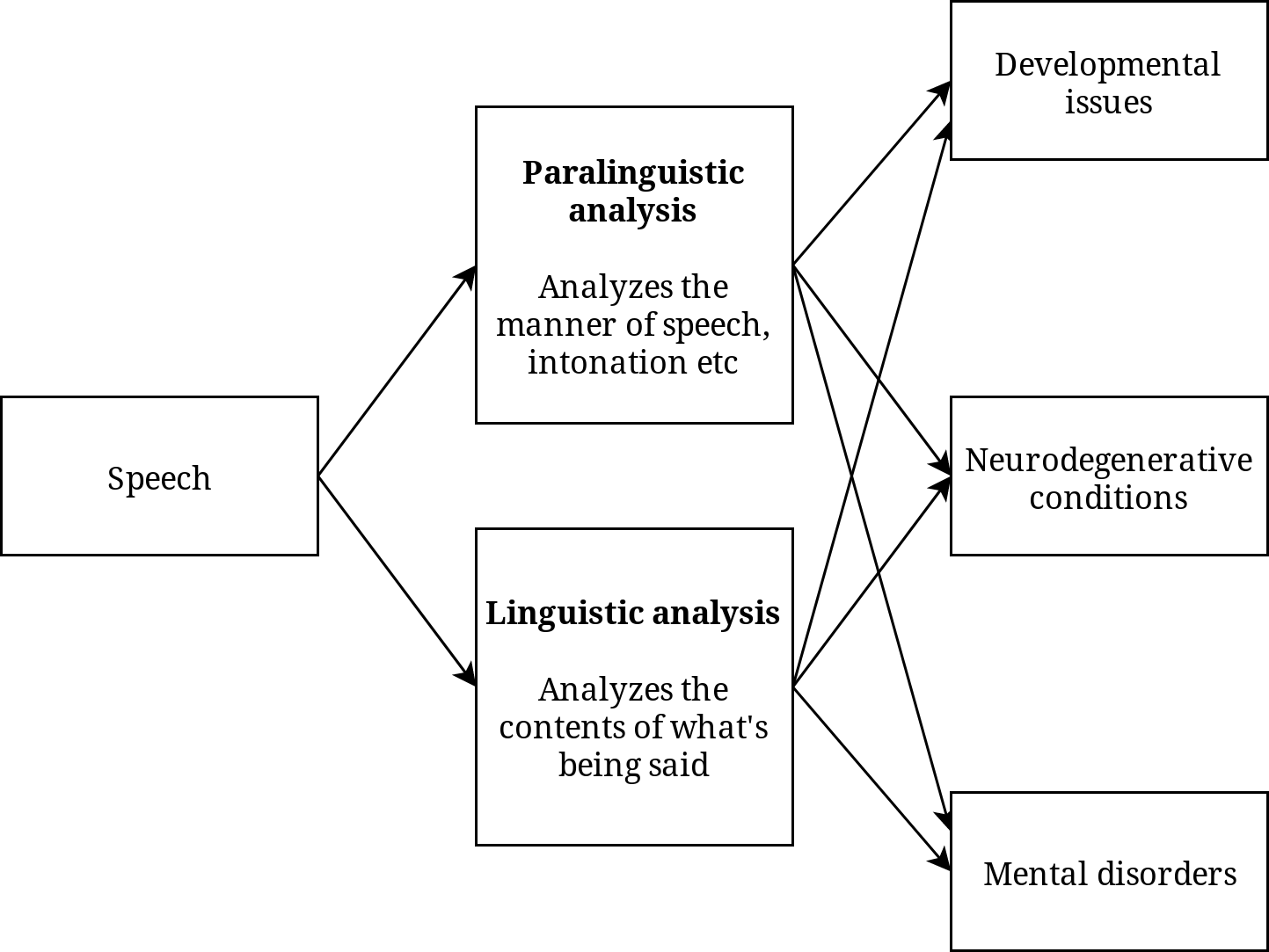
In this blog post, we have described just a few promising avenues, where speech technology can help both the medical practitioner and the patient. While many of the above-mentioned technologies have been tested only in research and experimental settings and thus can be considered somewhat pre-mature, the growing number of these technologies also points towards the potential for improvement on multiple levels in healthcare by employing these technological advances.
在此博客文章中,我们仅描述了一些有希望的途径,其中语音技术可以帮助医生和患者。 尽管许多上述技术仅在研究和实验环境中进行过测试,因此可以认为还为时过早,但通过采用这些技术进步,这些技术的数量不断增加,也表明在医疗保健多个层面上都有改进的潜力。
It should be noted that the medical industry is one with high stakes and moral responsibility. Medical data is often highly sensitive, and particular care should be taken to protect the privacy of the patient. The technologists and researchers bringing these improvements to life should also pay attention to not overselling and carefully indicating where the new technology still falls short. The improvements here should help and facilitate the job of a medical expert, but can’t substitute for it.
应该指出的是,医疗行业是一个利益攸关且具有道德责任的行业。 医疗数据通常是高度敏感的,应特别注意保护患者的隐私。 实现这些改进的技术人员和研究人员也应注意不要过度销售,并仔细指出新技术的不足之处。 此处的改进应有助于并促进医学专家的工作,但不能替代它。
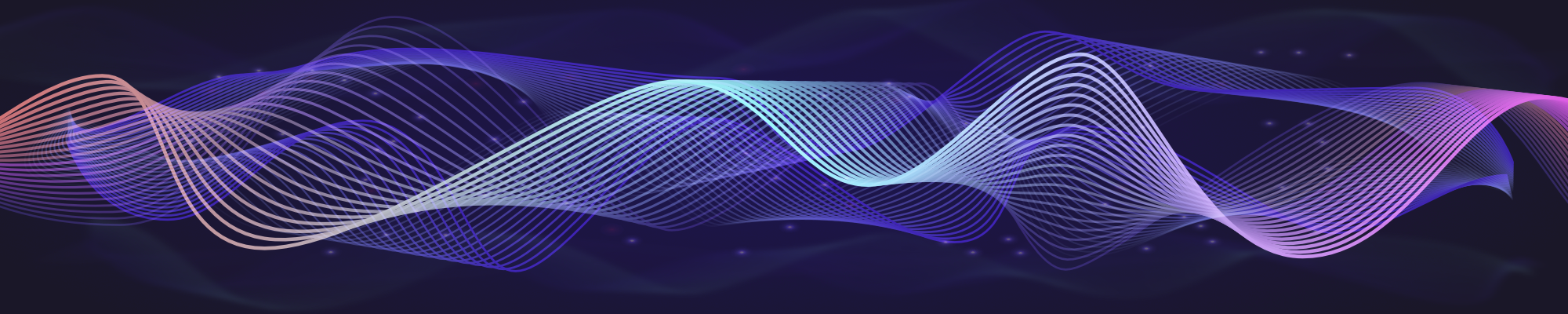
翻译自: https://medium.com/language-tech/voice-based-applications-for-e-health-befdadb1fd31
基于端到端 语音合成