猩猩艾艾吸烟
“A world perfectly fair in some dimensions would be horribly unfair in others.” - Kevin Kelly
“在某些方面完全公平的世界在其他方面则极其不公平。” -凯文·凯利
“Fairness” in Artificial Intelligence (AI) applications — both as a concept and a practice — is the focus of many organisations as they deploy new technologies for greater effectiveness and efficiencies. That machines are faster at processing large amounts of information and the notion that they are ‘more objective’ than humans, appear to make them an obvious choice for progressivity and seemingly impartial actors in ‘fairer’ decision-making.
人工智能(AI)应用程序中的“公平”(无论是概念还是实践)是许多组织在部署新技术以提高有效性和效率时所关注的焦点。 机器处理大量信息的速度更快,并且认为它们比人类更“客观”,这似乎使它们成为进步的明显选择,并且在“更公平”的决策中看似公正。
Yet, algorithmic based decisions have not come without their share of controversies — Australia’s recent ‘robo-debt’ government intervention which wrongly pursued thousands of welfare recipients; the UK’s ‘A-Levels fiasco’ of downgrading graduating grades based on historical data, its controversial visa application streaming tool; and concerns about Clearview AI’s facial recognition software for policing are raising new questions on the role of these technologies in society.
然而,基于算法的决策并非没有争议。澳大利亚最近的“机器人债务”政府干预错误地追求了成千上万的福利接收者。 英国的“ A级惨败”,根据历史数据,有争议的签证申请流工具降低了毕业成绩; 对Clearview AI的用于警务的面部识别软件的担忧,引发了有关这些技术在社会中的作用的新问题。
Risk assessments are part of the fabric of modern society, but what we are dealing with here is not just ‘scaling up’ human capacity for decision-making without the unwanted human biases and errors — we are also extolling the ‘virtues of objectivity’ under the guise of ‘fairness’ (which is inherently subjective!) and failing to recognise the many inter-relationships that are being unraveled through the use of these algorithms in our daily lives.
风险评估是现代社会结构的一部分,但是我们在这里处理的不只是“扩大”决策能力,而没有不必要的人为偏见和错误,我们还赞扬“客观性”冒充“公平”(本质上是主观的!)的幌子,并且没有意识到在我们的日常生活中通过使用这些算法所揭示的许多相互关系。
And it is these inter-relationships that are holding together the systems we find ourselves in.
这些相互关系将我们所处的系统联系在一起。
Issues around ‘trust’ when it comes to AI are multi-faceted — explaining how these technologies arrive at a particular outcome in decision making and its reliability is just part of the story! Understanding how our societal systems will change as a result of AI goes to the ‘trust’ that is within the relationships between people and their changing world.
关于AI的“信任”问题是多方面的-解释这些技术如何在决策中取得特定成果,其可靠性只是故事的一部分! 了解人类社会系统将如何随着人工智能的变化而走向人们与他们不断变化的世界之间关系的“信任”。
What we have really created is a way to disrupt these old structures and the perceptions on which they were founded. AI and other decision-making algorithms are forcing us to revisit the moral underpinnings of how we think about ‘fairness’ and its role in our society and where the trust really lies...
我们真正创造的是一种破坏这些旧结构和建立旧结构的方式。 人工智能和其他决策算法迫使我们重新审视我们对``公平''及其在社会中的作用以及信任真正所在的道德基础...
面对人工智能 (Facing up to AI)
“Against the infinity of the cosmos and the silent depths of nature, the human face shines out as the icon of intimacy” — John O’Dohohue
“面对宇宙的无边无际和大自然的寂静深度,人脸闪耀着亲密感的象征。” —约翰·奥多胡(John O'Dohohue)
The use of facial recognition in AI has received much attention in the media, particularly when it comes to human rights and privacy. In a recent article, the New York Times covered some of the many risks in using facial recognition technology including: its reliability and limitations; how it’s implemented and used; and the legal and moral challenges faced by society in navigating this ethical minefield.
在人工智能中使用面部识别已引起媒体的广泛关注,尤其是在人权和隐私方面。 在最近的一篇文章中,《纽约时报》介绍了使用面部识别技术的许多风险,其中包括:可靠性和局限性; 如何实现和使用; 以及社会在这个道德雷区中所面临的法律和道德挑战。
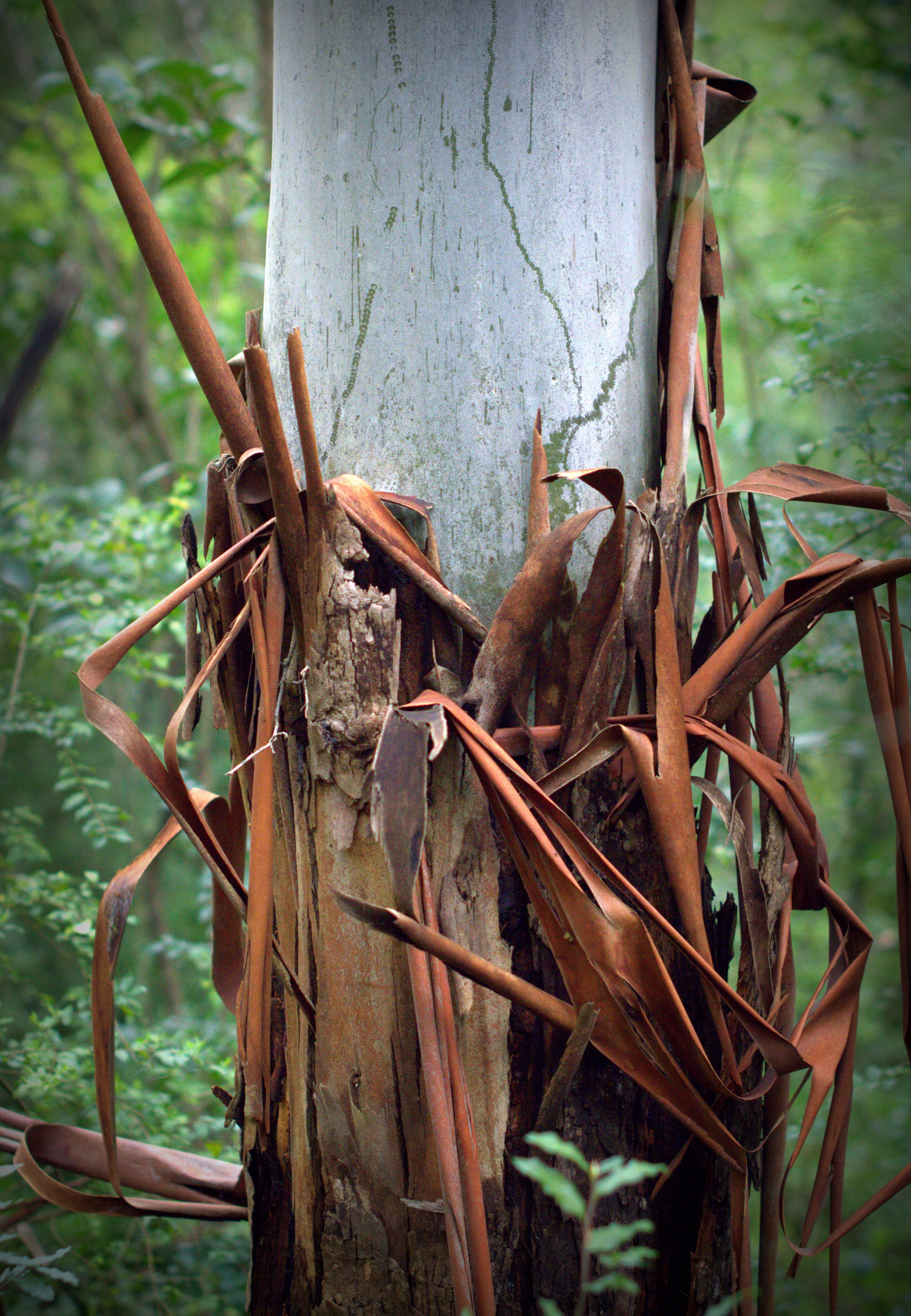
Calls for more transparency in AI applications, though critically important in understanding hidden biases and uncovering the underlying values in the algorithmic design, only scrape at the surface of deeper societal issues around justice and fairness.
呼吁提高AI应用程序的透明度,尽管在理解隐藏的偏见和揭示算法设计中的潜在价值方面至关重要,但只能涉及围绕正义和公平的更深层次的社会问题。
To view ‘transparency’, in this context, as a window into the decision making process from data to output, is to miss how interfacing AI with society is altering our current systems.
在这种情况下,将“透明度”视为从数据到输出的决策过程的一个窗口,就是想念AI与社会的互动如何改变我们当前的系统。
无论是算法还是基础数据,都没有足够的“创可贴解决方案”来解决本质上是系统范围的问题。
AI transparency and the toolkits and guidelines for building trust in these technologies do not go far enough in providing insights into how our systems are being affected across many different contexts such as social, economic, financial, political and educational amongst others.
人工智能的透明度以及建立对这些技术的信任的工具包和指南在提供洞察力以了解我们的系统如何在社会,经济,金融,政治和教育等许多不同环境中受到影响的程度还远远不够。
Take for example, using AI to analyse a job applicant’s facial movements to determine their suitability for employment in a particular industry or the claim that AI is able to predict a job applicants propensity for job-hopping — both which evoke sentiments similar to early eugenics!
例如,使用AI分析求职者的面部动作以确定他们在特定行业中的适合性,或者声称AI能够预测求职者的跳槽倾向-两者都唤起了类似于早期优生学的情感!
While the algorithms and underlying data may be the focus of much scrutiny, and questions on ethics and human rights come to the fore — what’s been largely missing is a deeper understanding of why the problems that these technologies seek to address are occurring, and how these ‘automated solutions’ actually affect the resilience and performance of these industries.
尽管算法和基础数据可能是许多审查的重点,并且有关道德与人权的问题应运而生,但在很大程度上缺少的是对这些技术为何试图解决的问题以及如何解决这些问题有更深入的了解。 “自动化解决方案”实际上会影响这些行业的弹性和性能。
Algorithmic transparency alone cannot comprehensively examine the inter-relationships within these contexts, or how they are changing as a result of these technologies. Why? Because historical data and a rules-based ethical framework cannot accommodate a continually evolving world, especially when not everything can be measured, and much in our world is ‘trans-contextual’ and learning or responding to the changing conditions around us.
仅算法透明性不能全面检查这些上下文中的相互关系,或者它们由于这些技术而如何变化。 为什么? 因为历史数据和基于规则的道德框架无法容纳一个不断发展的世界,尤其是在无法衡量所有事物的情况下,尤其是在我们的世界中,很多事情都是“跨上下文的”,并且学习或应对我们周围不断变化的状况。
At the heart of the matter lies many questions of how these technologies shape our society, what powers of control we have in directing these changes and how we perceive and think about fairness. Do we really want to go down the path we’ve prescribed for ourselves?
问题的核心是许多问题,这些技术如何塑造我们的社会,我们在指导这些变化方面拥有什么控制权,以及我们如何看待和思考公平问题。 我们真的要沿着我们为自己规定的道路走下去吗?
好,更好,最好… (Good, Better, Best…)
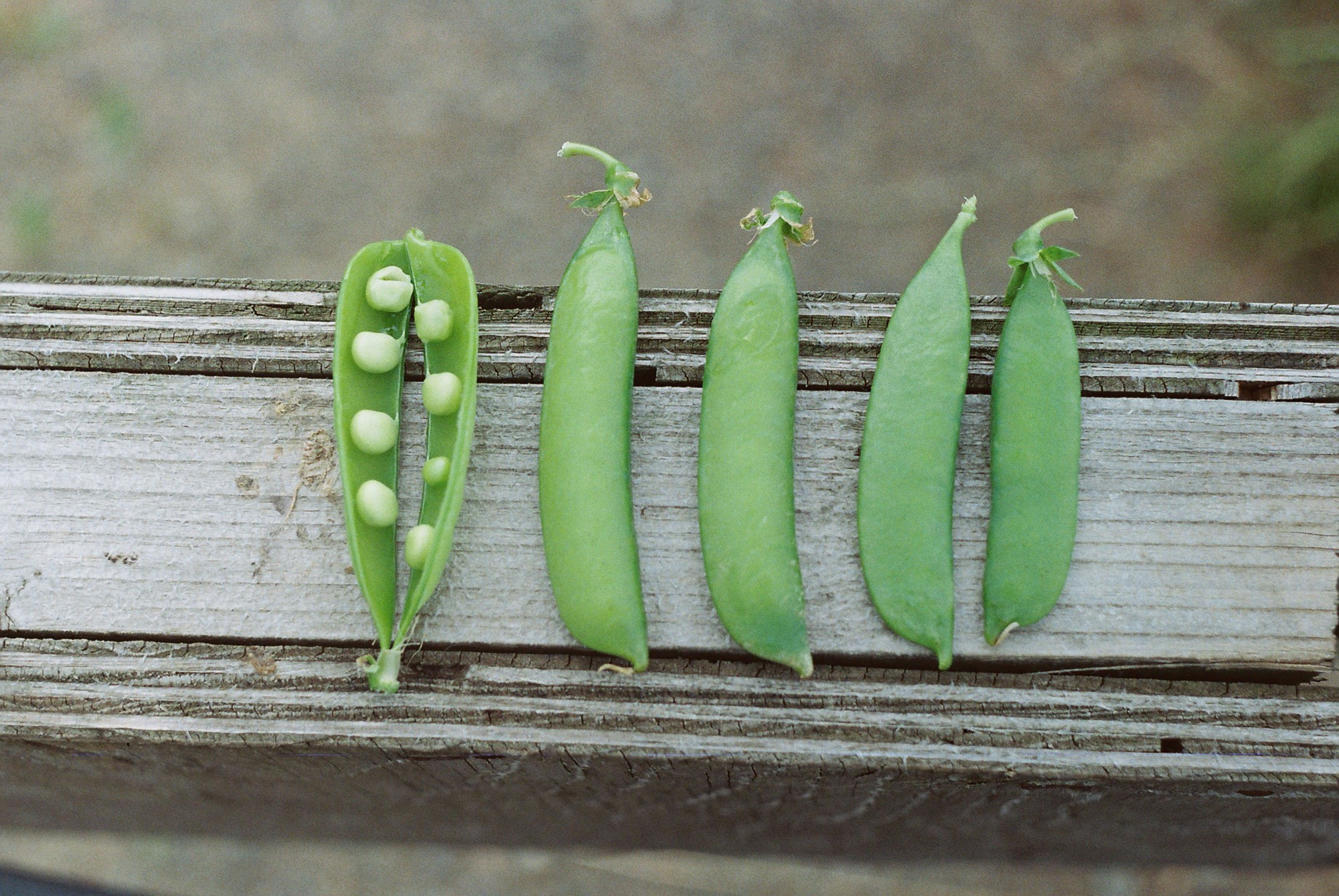
“We can be fully human without being in complete control of our world” — Douglas Rushkoff, Team Human
“我们可以成为完全的人类,而不必完全控制我们的世界” —人类团队Douglas Rushkoff
The business of decision-making is fraught with judgement — choosing between various alternatives, discriminating between different features and weighing up multiple possibilities — are all in the hope that any actions taken as a result of these decisions will achieve the desired outcome.
决策事务充满判断力-在各种选择之间进行选择,在不同功能之间进行区分并权衡多种可能性-都是希望这些决策所采取的任何行动都能取得理想的结果。
Underlying this human desire to predict and therefore ‘control’ outcomes to a pre-determined future lies the implicit assumption that these decisions will shift the system (be it an organisation or a society) to a ‘better’ state.
这种人类预测并因此“控制”结果到预定未来的愿望的基本隐含假设是,这些决定会将系统(无论是组织还是社会)转变为“更好”的状态。
These ideas are not new — one historical example being the desire for ‘improving humanity’, which gave birth to “Eugenics”(improving the genetic composition of the human race). First originating during the time of Plato (around 400 BC) and later developed after being inspired by Darwinism in the early 1900s, eugenics is supposed to literally mean “good creation”.
这些想法并不是什么新鲜事物,一个历史例子就是对“改善人类”的渴望,它催生了“优生学”(改善人类的基因组成)。 优生学最初起源于柏拉图时期(约公元前400年),后来在1900年代早期受到达尔文主义的启发而发展起来,它的字面意思是“好的创造力” 。
Contrary to popular belief, Darwinism does not fully explain the phenomenon of evolution— Mendel’s research into the hereditary and variation in peas, along with William Bateson’s interpretation of Mendelian principles, suggest that it could not explain ‘new species’. Interestingly, at around the same time, English Philosopher, G. E. Moore, in his Principia Ethica (1903) contended that “good” could not be defined.
与普遍的看法相反,达尔文主义不能完全解释进化现象-孟德尔对豌豆的遗传和变异的研究以及威廉·贝特森对孟德尔原理的解释表明,它不能解释“新物种” 。 有趣的是,大约在同一时间,英国哲学家通用·摩尔( GE Moore )在其《伦理学原理》 ( Principia Ethica) (1903)中主张,“好”是无法定义的。
These insights are important when using AI technologies in ‘selecting’ features or, in the example of recruitment and employment, choosing humans for a particular job or task. What this means is that what is ‘good’ and what is ‘fair’, is not only open to interpretation — but that even if these could be agreed on, the outcome that’s been engineered may not be as robust as we may have thought!
在将AI技术用于“选择”功能时,或者在招聘和雇用示例中,为特定工作或任务选择人员时,这些见解非常重要。 这意味着什么是“好”和什么是“公平”,不仅可以进行解释,而且,即使可以达成共识,经过精心设计的结果也可能没有我们想象的那么健壮!
In his book, “Out of Control”, Kevin Kelly talks about how “a little touch of randomness… actually creates long term stability”. So what might appear to be sub-optimal choices, could actually be critical elements in ensuring the resilience of systems through diversification! Moreover, Kelly emphasises the importance of ‘symbiosis’ (mutually beneficial interactions) in relationships, noting that in “one mutual relationship, evolution could jump past a million years of individual trial and error.”
凯文·凯利(Kevin Kelly)在他的《失控》一书中谈到了“一点点随机性……实际上创造了长期稳定性”。 因此,看似次优的选择实际上可能是通过多元化确保系统弹性的关键要素! 此外,凯利(Kelly)强调了关系中“共生”(互惠互利)的重要性,并指出在“一种相互关系中,进化可能会跨越一百万年的反复试验。 ”
What AI applications are as yet unable to capture are elements of ‘mutual learning’ or ‘symmathesy’, as Nora Bateson refers to, which are dependent on the many contexts and the responses within that environment. It is within these ‘learnings’ that evolution and adaptation is occurring.
正如诺拉·贝特森(Nora Bateson)所提到的,至今仍无法捕获的AI应用程序是“相互学习”或“交响乐”的要素,这些要素取决于该环境中的许多上下文和响应。 正是在这些“学习”中发生了进化和适应。
In our earlier example of AI recruitment, determining the optimal facial features, which in turn supposedly pre-determines personality traits, misses the inter-relationships and learnings that happen within an organisation. Not only that, the opportunities for innovation and growth within the organisational ecosystem are also limited.
在我们较早的AI招聘示例中,确定最佳面部特征(据称预先确定了人格特征)会错过组织内部发生的相互关系和学习。 不仅如此,组织生态系统内创新和增长的机会也很有限。
AI technologies may be able to optimise for what we think are ‘best case scenarios’, but may be dismissing key attributes and features that are essential for our long term viability — whether it be organisation, industry or nation.
人工智能技术可能能够针对我们认为的“最佳情况”进行优化,但可能忽略了对于我们的长期生存能力至关重要的关键属性和功能,无论是组织,行业还是国家。
Phoensight is an international consultancy dedicated to supporting the interrelationships between people, public policy and technology, and is accredited by the International Bateson Institute to host and conduct Warm Data Labs.
Phoensight是一家致力于支持人,公共政策和技术之间的相互关系的国际咨询公司,并获得了国际Bateson Institute的认可,可以托管和开展暖数据实验室。
翻译自: https://medium.com/phoensight/ai-ai-on-the-wall-whos-the-fairest-of-them-all-5e3983d6bd39
猩猩艾艾吸烟