ai人工智能方向
意见 (Opinion)
Four days ago, Hannah Kerner wrote
“Too many AI researchers think real-world problems are not relevant. The community’s hyperfocus on novel methods ignores what’s really important.”
“太多的AI研究人员认为现实世界中的问题不相关。 社区对新颖方法的过度关注忽略了真正重要的事情。”
This isn’t the first article (and won’t be the last) to discuss how the AI community seems to follow an agenda of its own. Large venues blatantly ignore application papers — works focused on applying current methods to real-world problems and the challenges of doing so (which are many). Proceedings are simply out of touch with reality. An extra 10% on object detection is far more valued than a 1% reduction in cancer deaths.
Ť他是不是第一篇文章(并不会是最后一次),讨论AI社区如何似乎遵循其自身的议程。 大型场所公然忽略了申请文件-致力于将当前方法应用于现实世界中的问题以及这样做的挑战(很多)。 程序与现实完全脱节。 在物体检测上多出10%的价值远比将癌症死亡人数减少1%的价值更大。
The AI community largely ignores the obvious: deep learning is an experimental science. Despite their formal roots, neural networks are massive non-linear systems that defy explanation. Although the growing body of work dedicated to their interpretation, neural networks remain as mysterious as ever. The only reliable tool we have to understand them is the scientific method — which is deeply rooted in experimentation.
AI社区在很大程度上忽略了显而易见的事实:深度学习是一门实验科学。 尽管具有正式的根源,但神经网络是无法解释的大规模非线性系统。 尽管致力于解释它们的工作越来越多,但神经网络仍然像以往一样神秘。 我们必须了解它们的唯一可靠工具是科学方法-它深深植根于实验中。
And here is the contradiction: despite being experimental in nature, the field rejects pure experimentation. The average neural network paper presents its novelties, attempts a formal proof, and does an ablation study. The end. This is as far as experimentation goes.
这是矛盾的: 尽管本质上是实验性的,但该领域拒绝纯粹的实验。 普通的神经网络论文介绍了它的新颖性,尝试了形式证明,并进行了消融研究。 结束 。 就实验而言。
Imagine if the civil engineering community decided to invent novel bridge designs, but opted to do all its validations on table-sized LEGO replicas. No expensive simulations nor trials using real building materials. Would you trust the newly proposed designs? Would you trust these experiments enough to invest millions of dollars in realizing them? I don’t think so.
想象一下,如果土木工程界决定发明新颖的桥梁设计,但选择对桌子大小的LEGO复制品进行所有验证。 使用真实的建筑材料无需进行昂贵的模拟或试验。 您相信新提出的设计吗? 您相信这些实验足以投入数百万美元来实现它们吗? 我不这么认为。
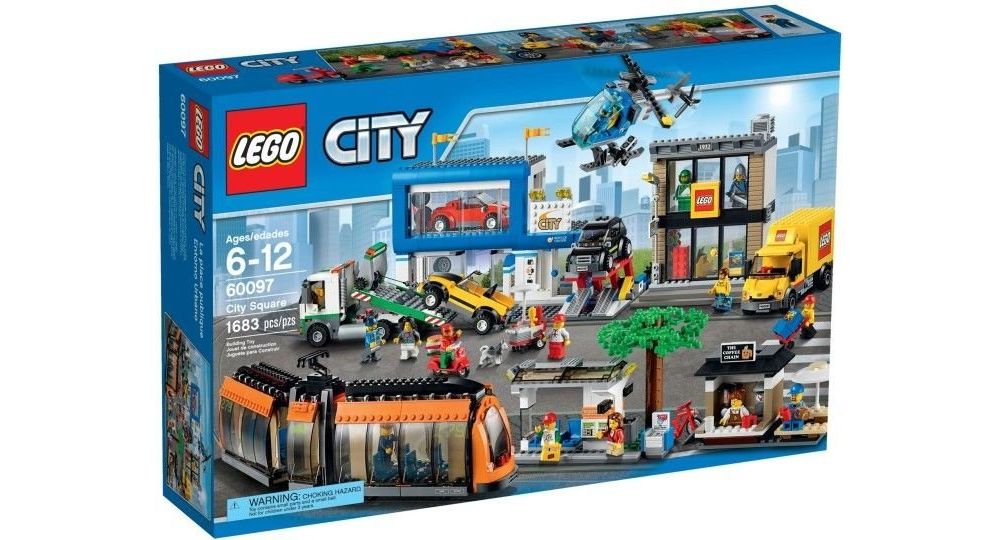
Simplified world models are useful for quick prototyping and trying ideas. They hint at good designs, even though they can’t prove them. For actual proof, you need the world. It is a two-step process.
简化的世界模型对于快速制作原型和尝试想法很有用。 他们暗示了好的设计,即使他们无法证明它们。 为了获得实际证明,您需要世界。 这是一个两步过程 。
Modern AI research is stuck in the first half: benchmarks. Real use cases are the other half — the missing half. ImageNet, COCO, CIFAR-10, and the sort are the LEGO of AI. They allow us to experiment with new ideas and dismiss bad ones. They are great tools. However, they are a means to an end, not the end itself.
现代AI研究停留在上半年:基准测试。 实际用例是另一半-缺少的另一半。 ImageNet,COCO,CIFAR-10等是AI的乐高。 它们使我们能够尝试新的想法并消除不良想法。 他们是很棒的工具。 但是,它们是达到目的的手段, 而不是目的本身 。
The message is not that current works are bad, far from so. The issue is the current disconnect between academia and the real world.
并不是说当前的工作是不好的,远不是这样。 问题是当前学术界与现实世界之间的脱节。
Consider the plot. It shows the recent progress on the COCO object detection benchmark. Each tiny dot is a different model — a new technique or a blend of existing ones. Leaders are highlighted in blue.
çonsider情节。 它显示了在COCO对象检测基准方面的最新进展。 每个小点都是不同的模型-一种新技术或现有技术的结合。 领导者以蓝色突出显示。
The chart shows a trajectory from 28.8 AP in January 2016 to a whopping 55.1 in July 2020. The progress is undeniable. According to the plot, EfficientDet D7x is the current best technique for object detection. Yet, I ask you: which of these models would you implement for an application?
图表显示了从2016年1月的28.8 AP到2020年7月的55.1的轨迹。进展是不可否认的。 根据该图,EfficientDet D7x是当前物体检测的最佳技术。 但是,我问你: 您将为应用程序实现哪些模型?
High are the odds that you won’t even answer, as you don’t know which app I am talking about or which are its requirements. Does it need to run in real-time? Will it run on mobile devices? How many classes does it have to identify? How tolerant are its users to wrong detections? Etc.
您甚至不愿回答的几率很高,因为您不知道我在谈论哪个应用程序,或者哪个是它的要求。 是否需要实时运行? 它将在移动设备上运行吗? 它必须识别多少个类? 用户对错误检测的容忍度如何? 等等。
Depending on the answers, none of the above are even worth considering, not even EfficientDet D7x. For instance, if the model has to run in real-time on a mobile phone, none of these models are even slightly tuned for performance. Worse, no guarantee exists that these models will yield consistent detections across sequential frames. I can’t even name an app that requires the highest quality detection available despite anything else — that has no other requirement besides high accuracy.
根据答案,以上都不是值得考虑的,甚至EfficientDet D7x都不值得考虑。 例如,如果该模型必须在手机上实时运行,则这些模型甚至都没有针对性能进行微调。 更糟糕的是,无法保证这些模型会在连续帧中产生一致的检测结果。 我什至无法命名一个需要最高质量检测的应用程序,尽管它有其他任何要求-除了高精度之外,它没有其他要求。
In other words, the metric the research community is chasing serves no one but the community itself.
换句话说,研究社区所追求的指标只为社区本身服务。
Back in 2015, increasing the depth of neural networks beyond a dozen layers was detrimental to performance. He et al., in the famous ResNet paper, hypothesized that connecting non-sequential layers by skip connections could scale capacity, as it would improve the gradient flow.
其他回在2015年,增加了神经网络的深度超出了十几层是不利的性能。 他等。 ,在著名的ResNet论文中 ,假设通过跳过连接来连接非顺序层可以扩展容量,因为这会改善梯度流。
In its first year, ResNet achieved terrific results on several benchmark competitions, such as ILSVRC and COCO. By now, you already realized this is just a hint that ResNet was a significant contribution — it is no proof.
在成立的第一年,ResNet在ILSVRC和COCO等多个基准测试比赛中取得了骄人的成绩。 到目前为止,您已经意识到这只是ResNet做出了重大贡献的暗示-尚无证据。
The definite proof of ResNet’s place on AI history is the enormous body of work built upon it. The striking aspect of ResNet is the number of unrelated problems it solves, not the competitions it won. And, to be fair, the real contribution is the skip idea, not the architecture itself.
ResNet在AI历史上的地位的确凿证明是建立在其之上的大量工作。 ResNet的显着方面是它解决的不相关问题的数量,而不是它赢得的竞争。 而且,公平地说,真正的贡献是跳过的想法,而不是架构本身。
Likewise, the Focal Loss paper stood the test of time because its contributions brought real improvements to other people’s work. The Attention paper follows the same route. Every day a new article shows how Attention improved some benchmark (and a focused loss made it even better).
同样, Focal Loss论文也经受了时间的考验,因为它的贡献为其他人的工作带来了真正的进步。 注意文件遵循相同的路线。 每天都有一篇新文章介绍Attention如何提高一些基准(而集中的损失使它变得更好)。
It is not the competitions that matter. It is the legacy. In fact, AlexNet won the ILSVRC in 2012, and ResNet won it in 2015. Can you name the 2013 and 2014 winners? What about the challenges held in 2016, 2017, and 2018? Are you even sure there was an ILSVRC for each year?
竞争并不重要。 这是遗产。 实际上,AlexNet在2012年赢得了ILSVRC,ResNet在2015年赢得了。您能说出2013年和2014年的获奖者吗? 那么2016年,2017年和2018年所面临的挑战又如何呢? 您甚至确定每年都有ILSVRC吗?
So you may ask, why is this happening? Why aren’t there better benchmarks or more useful metrics? How can we measure legacy or impact?
所以您可能会问,为什么会这样? 为什么没有更好的基准或更有用的指标? 我们如何衡量遗产或影响?
The sad truth is… we can’t. We could use citation or download counts, or maybe Reddit mentions or GitHub stars. However, these are all flawed metrics. For a fair comparison, we need to account for every detail while normalizing all bias out of the equation. It is insanely hard.
可悲的事实是……我们不能。 我们可以使用引文或下载次数,也可以使用Reddit提及或GitHub星级。 但是,这些都是有缺陷的指标。 为了进行公平的比较,我们需要考虑所有细节,同时将所有偏差归一化。 这太疯狂了。
For instance, to compare the legacy of Attention with the legacy of ResNet, we need to account for any successful use of these concepts, weigh their relative impacts, and normalize for age (ResNet is older) and reach (Attention is also relevant for text). It is readily apparent that quantifying these properties is a huge undertaking likely to be as flawed as any benchmark or metric. Ideas such as the journal’s impact factors don’t even scratch the surface of this issue.
例如,要将Attention的遗产与ResNet的遗产进行比较,我们需要考虑这些概念的任何成功使用,权衡它们的相对影响并针对年龄(ResNet年龄较大)和覆盖范围进行归一化(Attention也与文本有关) )。 显而易见,量化这些属性是一项艰巨的任务,可能会像任何基准或度量标准一样存在缺陷。 诸如期刊影响因素之类的观点甚至都没有触及这一问题的表面。
Some goals just can’t be quantified. Who was the most important person: Bach, regarded as the most influential composer of western music, or Shakespeare, regarded as the most influential dramatist? It makes no sense to compare their work, let alone their fields.
有些目标无法量化。 谁是最重要的人物:被认为是西方音乐最有影响力的作曲家巴赫 ,或被认为是最有影响力的戏剧家的莎士比亚 ? 比较他们的工作毫无意义,更不用说他们的领域了。
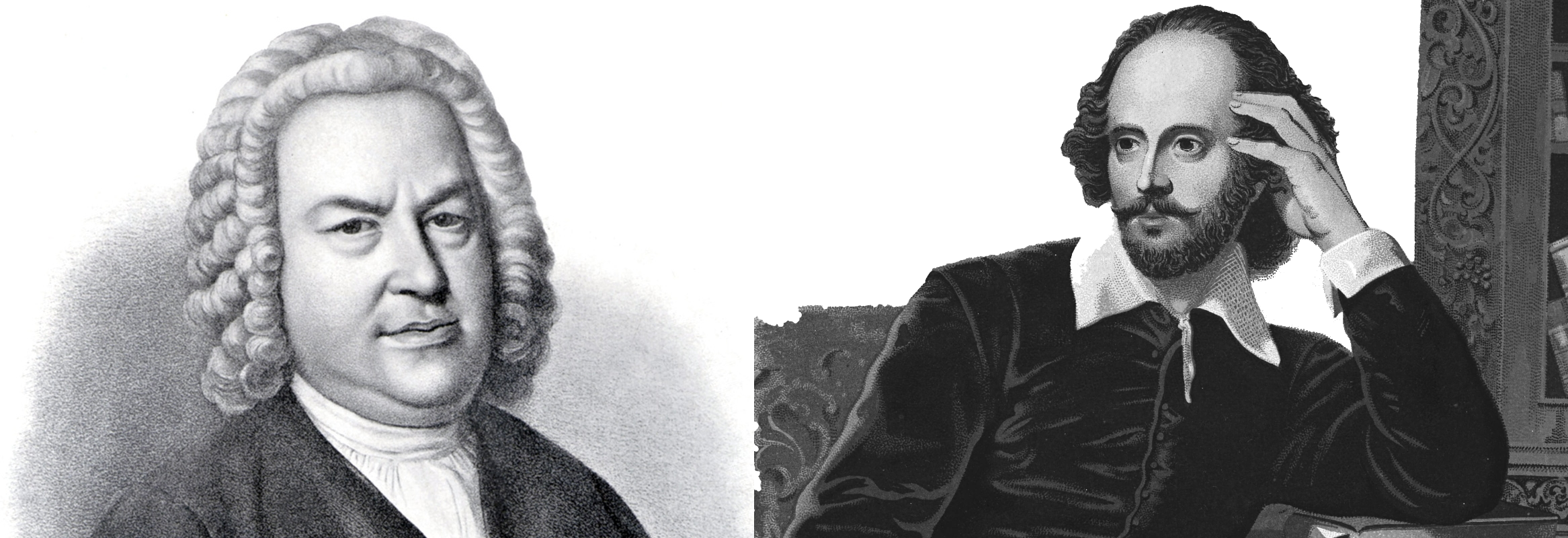
This brings us to a dead end. We can measure accuracy, we can measure speed, but we can’t measure impact. We all agree we need better science, but how can we tell one science is better than another? How can we measure the disconnect between research and reality?
这使我们陷入死胡同。 我们可以测量准确性,可以测量速度,但是不能测量影响。 我们都同意我们需要更好的科学,但是我们怎样才能说一门科学比另一门科学更好呢? 我们如何衡量研究与现实之间的脱节?
We want to move forward with AI, but we don’t know which direction forward is nor how far have we walked.
我们想通过AI向前迈进,但我们不知道前进的方向,也不知道走了多远。
This isn’t a problem with AI alone. We want better governments, better health care, better education, but how can we really quantify these things? So far, the least unsuccessful approach (and the most popular one) is surrogate metrics. A surrogate is a substitute — something we use in-place of something else. For instance, the COCO AP score.
Ť他不是单独AI的问题。 我们想要更好的政府,更好的医疗保健,更好的教育,但是我们如何真正量化这些东西呢? 到目前为止,最不成功的方法(也是最受欢迎的方法)是替代指标。 替代品是替代品-我们用其他替代品代替。 例如,COCO AP分数。
We can’t measure the progress of AI, but we can measure how accurate the current object detection methods are. Since object detection is a part of AI, if we make any progress on it, we can expect also to be making progress on AI. Before COCO, we used ImageNet Top-5 results… until we nailed it, so we moved to a more challenging problem. Hopping from problem to problem, we hope also to be making progress towards better AI.
我们无法衡量AI的进度,但是我们可以衡量当前对象检测方法的准确性。 由于对象检测是AI的一部分,因此,如果我们在它上面取得任何进展,我们也可以期望它也在AI方面取得进展。 在COCO之前,我们使用ImageNet Top-5的结果…直到确定了结果,所以我们提出了一个更具挑战性的问题。 从一个问题跳到另一个问题,我们也希望朝着更好的AI迈进。
We do this all the time. We can’t train detection models to improve their AP, but we can teach them to reduce the L2 loss of the bounding box coordinates. Losses are surrogates for undifferentiable metrics. The L2 loss is not AP, but we know that a low L2 loss correlates with a higher AP, so it works.
我们一直在这样做。 我们无法训练检测模型来提高其AP,但可以教他们减少边界框坐标的L2损失。 损失是无法区分的指标的替代物。 L2损耗不是AP,但我们知道L2损耗低与AP越高相关,因此它可以工作。
Once upon a time, literacy rates were the dominant metric for progress in education in many countries. Some decades later, literacy was high enough for the focus to shift towards higher school completion rates. Then, higher college attendance. I don’t know if degrees are as correlated with education as we think they are — or if high schools teach what they should, but these are the metrics we target today.
曾几何时,识字率是许多国家教育进步的主要指标。 几十年后,识字率高到足以使重点转向更高的学业完成率。 然后,上大学。 我不知道学位与教育是否像我们认为的那样相关-还是中学教他们应该做什么,但这是我们今天的目标。
In a sense, there is no right direction to tackle such problems. Therefore, by definition, all routes are wrong. The only way we can increase our odds of picking a less-wrong path is by following as many paths as we can. Using AI terminology, we need to use a larger batch-size and sample as many distributions as we can.
从某种意义上说,没有正确的方向来解决这些问题。 因此,根据定义,所有路线都是错误的。 我们增加选择一条错误路径的几率的唯一方法是尽可能多地遵循路径。 使用AI术语,我们需要使用更大的批处理大小,并尽可能采样分布。
This means we have to broaden our focus beyond “accuracy” and “speed” also to include things such as “robustness” or “coherence.” On top, we need to move from hand-picked benchmarks to the real-world.
这意味着我们必须将重点扩展到“准确性”和“速度”之外,还应包括“稳健性”或“一致性”之类的内容。 最重要的是,我们需要从精心挑选的基准过渡到现实世界 。
Giving my personal account studying breast cancer detection algorithms, it is easy to think the field is solved. Recent works have achieved super-human scores on the topic, yet, you don’t see these algorithms being applied at any hospital. For a simple reason: it doesn’t work.
摹艾尔文我的个人账户学习乳腺癌检测算法,很容易联想到现场解决。 最近的 工作在该主题上都取得了超人的成绩,但是,您看不到这些算法在任何医院都得到应用。 原因很简单:它不起作用。
I know this sounds a bit of a stretch, but it is pretty simple: if you train on dataset A, the algorithm doesn’t work on dataset B, even though it is the same thing: mammograms. There is no known technique today that can be trained on one dataset and work well on others without fine-tuning. You have to build a dataset for each machine/hospital to get any useful results. Metric-wise, the field is solved. In practice, it hardly even began.
我知道这听起来有些牵强,但这很简单:如果在数据集A上进行训练,该算法就无法在数据集B上运行,即使它是同一件事:乳房X光照片。 如今,没有一种已知技术可以在一个数据集上进行训练,而无需进行微调就可以很好地在其他数据集上工作。 您必须为每台机器/医院建立一个数据集,以获得任何有用的结果。 从度量角度来看,该字段已解决。 实际上,它甚至还没有开始。
On top of that, algorithms provide little to no insight into their answers. Put yourself on the doctors’ shoes: would you tell someone they have cancer because a machine said so? You won’t; you will give the images a second look. If the AI isn’t trusted, it will never be used.
最重要的是,算法几乎不能完全了解其答案。 穿上医生的鞋:你会告诉某人患有癌症的原因是因为一台机器这么说吗? 你不会的 您将再次查看图像。 如果不信任AI,将永远不会使用它。
So far, the dominating metric for published works is the AUC score. It tells you how well the algorithm classifies mammograms as benign and malignant. It doesn’t tell you how robust it is to other datasets or if any of it is explainable. In other words, it answers almost nothing about: is it useful?
到目前为止,已发表作品的主要指标是AUC分数。 它告诉您算法将乳房X线照片分类为良性和恶性的程度。 它并没有告诉您它对其他数据集的稳健性或其中的任何可解释性。 换句话说,它几乎没有回答:它有用吗?
There is no right path to advance AI, but there surely are very wrong ones. It doesn’t take much time in the industry to realize how inapplicable most of the literature is and how the real pressing issues are clearly neglected. As I said at the beginning, this article’s message is not that current works are bad. The point is the current disconnect between academia and the real world. We are too narrowly focused on accuracy.
推进人工智能没有正确的道路,但是肯定有非常错误的道路。 在行业中不需要花费很多时间就可以认识到大多数文献是多么不适用,以及如何真正地忽略了紧迫的问题。 就像我在开始时说的那样, 本文的意思并不是说当前的作品是不好的。 关键是当前学术界与现实世界之间的脱节。 我们过于狭accuracy地关注准确性。
Quoting Hannah Kerner again, “The community’s hyperfocus on novel methods ignores what’s really important.” In my opinion, what is really important is advancing our society — and we expect to do so by improving AI. However, we will only be able to do so properly when we embrace real societal problems. Problems way more complicated than accurate object detection.
再次引用Hannah Kerner的话: “社区对新颖方法的过度关注忽略了真正重要的事情。” 我认为,真正重要的是促进我们的社会发展,我们希望通过改善AI来实现。 但是,只有在我们面对真正的社会问题时,我们才能正确地做到这一点。 问题的方法比精确的对象检测更为复杂。
Thanks for reading :)
谢谢阅读 :)
翻译自: https://towardsdatascience.com/ai-is-heading-the-wrong-direction-dc758f7e870
ai人工智能方向