基于ai的预测
By Philong Duong, Senior Product Manager
高级产品经理Philong Duong
As a leading provider of AI-enabled predictive maintenance applications to the Department of Defense (DoD), C3.ai has had the privilege since 2017 of helping to transform the maintenance practices for more than 1,200 aircraft on seven different platforms in partnership with the U.S. Air Force, Army, and Defense Innovation Unit (DIU).
作为国防部(DoD)提供AI的预测性维护应用程序的领先提供商,C3.ai自2017年以来一直荣幸地与美国合作,帮助在七个不同平台上转变了1,200多架飞机的维护方法。空军,陆军和国防创新单位(DIU)。
After more than a decade on active duty with the U.S. Marine Corps as a helicopter and tiltrotor pilot, as well as a flight line maintenance officer, I joined C3.ai to help expand what I believe is a critical transformation. Let me explain why.
在美国海军陆战队服役超过十年后,我担任直升机和俯仰旋翼飞行员以及飞行路线维护官,之后我加入了C3.ai,以帮助扩大我认为是关键的转变。 让我解释一下原因。
DoD leadership emphasizes readiness — the measure of the military’s ability to meet the demands of the National Defense Strategy. In aviation, this is a product of both aircraft mission capability and aircrew proficiency/currency — a healthy aircraft combined with a well-trained crew makes for combat mission readiness. Unfortunately, some services have aircraft mission capability as low as 40 percent, which means that fewer than half the planes in the fleet are capable of flying required missions at any given time.
国防部领导层强调战备状态,这是衡量军队满足《国防战略》要求的能力的标准。 在航空领域,这是飞机任务能力与机组人员熟练程度/货币的结合的结果-一架健康的飞机加上训练有素的机组人员可以为战斗任务做好准备。 不幸的是,某些服务的飞机任务能力低至40%,这意味着机队中只有不到一半的飞机能够在任何给定时间执行所需的任务。
In my experience the reality is that the DoD consistently relies on its aircrews to compensate for lapses in aircraft health. Student pilots must demonstrate exceptional capabilities in stress management, decision-making, and aeronautical systems before they can become fleet aviators. Because of this, pilots generally are skilled enough to avert most catastrophes, but even the most savvy crews cannot compensate for every mechanical failure.
根据我的经验,国防部始终依靠其机组人员来弥补飞机健康状况的下降。 学生飞行员必须具备出色的压力管理,决策和航空系统能力,才能成为机队飞行员。 因此,飞行员通常具备足够的技能来避免大多数灾难,但是即使是最精明的机组人员也无法弥补每一次机械故障。
On my last deployment in 2014, my unit was hastily deployed to the Middle East to fight ISIS, the expanding insurgent Islamic State. Weeks after our arrival, we inherited decade-old tiltrotor MV-22s and immediately began flying combat missions. Unfortunately, the aircraft had endured years of compounding mechanical wear and tear; it was not uncommon to hear stories in the ready room of our peers facing system failures during their missions. During one particularly harrowing flight, Captain Steven Herrera was faced with fluctuating gearbox oil pressure while flying in Iraqi airspace, followed by a “gearbox oil pressure low” warning, and then a subsequent “complete loss of oil pressure” indication. Usually, such a series of warnings would serve as a clear signal of impending catastrophic failure. A less experienced crew might have landed the plane as quickly as possible in unfriendly territory, but Herrera and his crew were aware of a recent trend of faulty pressure transducers within the squadron. They continued flying and landed safely beyond the Iraqi border. Unfortunately, similar events are common, and they do not always end well. I have many dear friends who faced similar situations and did not survive their mechanical failures.
在2014年的最后一次部署中,我的部队匆忙部署到中东,以对抗不断扩大的叛乱伊斯兰国ISIS。 抵达美国后数周,我们继承了具有十年历史的倾斜旋翼MV-22,并立即开始执行飞行任务。 不幸的是,飞机忍受了多年的机械磨损。 在我们的同龄人执行任务期间面临系统故障的情况下,经常听到他们在同伴间里的故事是很常见的。 在一次特别痛苦的飞行中,史蒂文·埃雷拉机长在伊拉克领空飞行时面临变速箱机油压力波动的情况,随后发出“齿轮箱机油压力低”警告,随后出现“机油压力完全损失”的提示。 通常,这样的一系列警告可以作为即将发生灾难性故障的明确信号。 经验不足的机组人员可能会尽快将飞机降落在不友好的地区,但是Herrera和他的机组人员意识到中队内部压力传感器故障的最新趋势。 他们继续飞行并安全降落在伊拉克边界之外。 不幸的是,类似的事件很普遍,而且并不总是以良好的结局。 我有许多亲爱的朋友,他们遇到过类似的情况,无法幸免于机械故障。
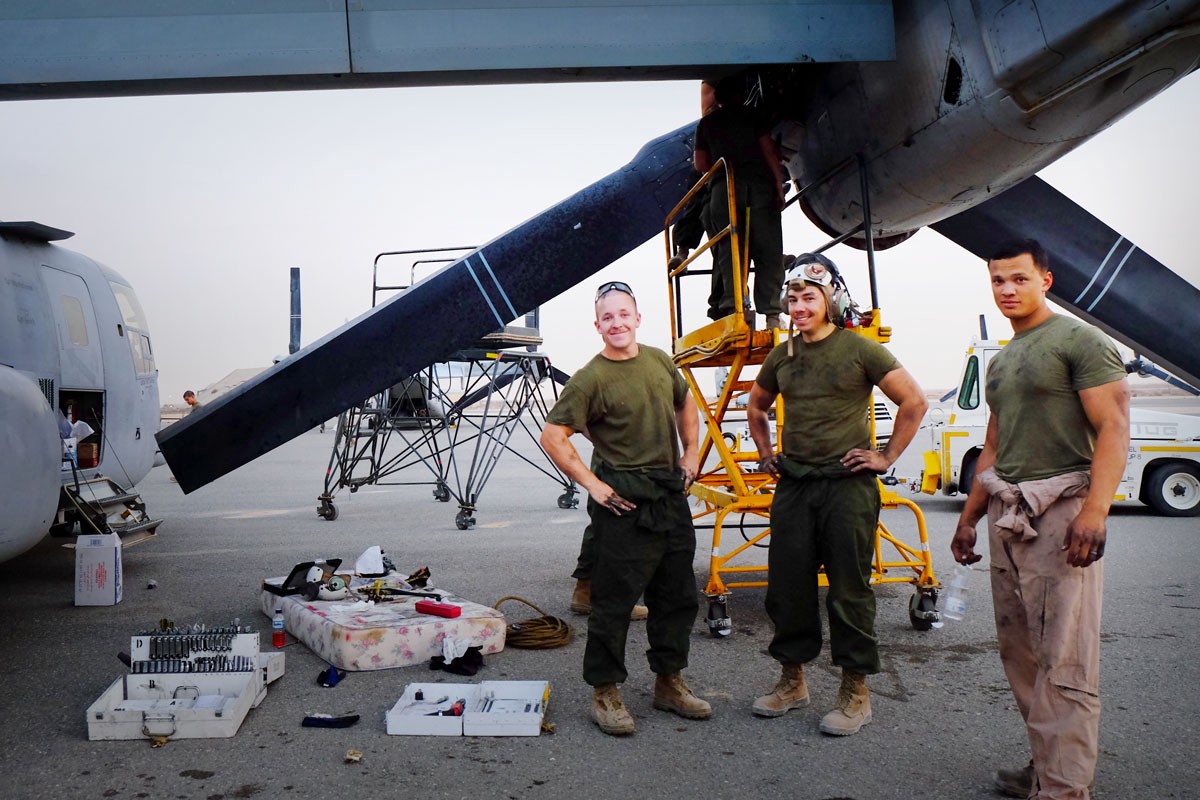
Flight line maintainers similarly are required to use their experience to compensate for a lack of analytics. During that same deployment, my unit consumed swashplate drive tubes at a rate four times greater than the fleet average in similar operational contexts. Because of our collective experience and our understanding of operational context and maintenance history, we were able to observe and fix the problem. Without this collective expertise, however, the cause of shortages would have gone undiagnosed for longer, negatively impacting mission capability. There were no sophisticated AI-enabled tools to help us predict, alert, or recommend appropriate investigations.
同样,飞行路线维护者也需要利用他们的经验来弥补缺乏分析的不足。 在相同的部署中,我的单元在相同的操作环境中消耗的旋转斜盘驱动管的速度是机队平均水平的四倍。 由于我们的集体经验以及对操作环境和维护历史的了解,我们能够观察并解决问题。 但是,如果没有这种集体的专业知识,短缺的原因将无法得到诊断,时间更长,会对任务能力产生负面影响。 没有复杂的支持AI的工具可以帮助我们预测,警告或推荐适当的调查。
This is why the military requires a new class of near-real-time analytical tools that augment the experience and capabilities of aircrews and maintainers. Through aggregating, analyzing, and correlating all available telemetry, operational, and maintenance data, these tools could predict failures with enough lead time to inform downstream maintenance, operations, and supply-chain requirements and processes.
这就是为什么军方需要新型的近实时分析工具来增强机组人员和维修人员的经验和能力的原因。 通过汇总,分析和关联所有可用的遥测,操作和维护数据,这些工具可以用足够的交付时间来预测故障,以告知下游维护,操作以及供应链要求和流程。
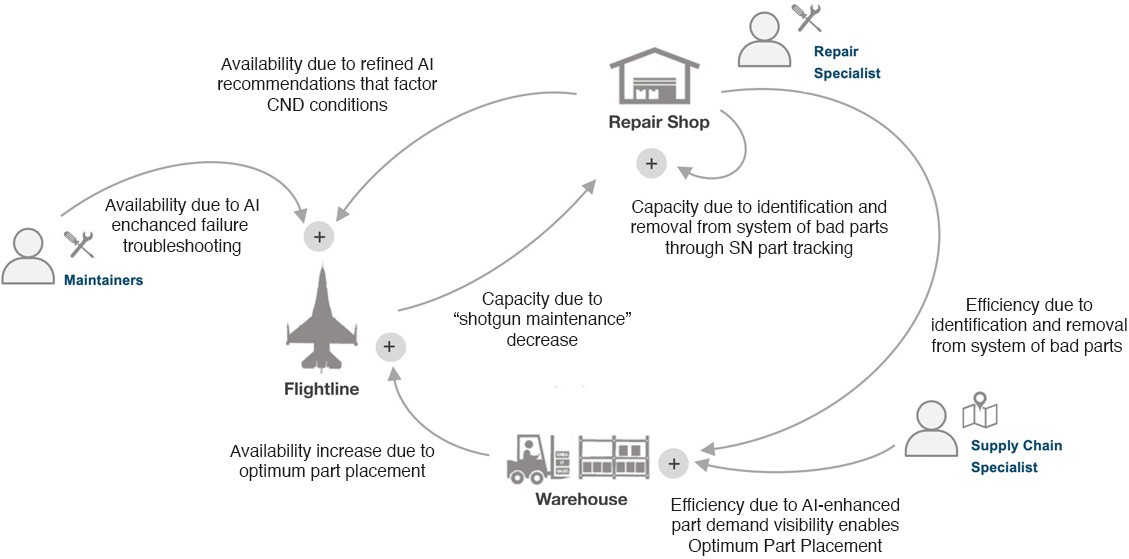
Identifying Barriers to Improvement
确定改进的障碍
The DoD spends $685 billion on sustainment annually, including $42 billion to support and maintain aviation. The department has long recognized the cost savings and flight safety improvements predictive maintenance can achieve. The 2007 Condition-Based Maintenance Plus (CBM+) policy for military departments and defense agencies includes efforts to shift equipment maintenance from unscheduled, reactive processes to more proactive and predictive methods driven by condition sensing and integrated, analysis-based decisions. Commercial airlines have been employing such methods for more than a decade.
国防部每年在维持方面的支出为6,850亿美元,其中包括用于支持和维护航空业的420亿美元。 该部门早已认识到可以节省成本并提高飞行安全性,从而实现预测性维护。 针对军事部门和国防机构的2007年基于状态的维护增强(CBM +)政策包括将设备维护从计划外的React性过程转变为由状态感测和基于分析的综合决策驱动的更主动和更具预测性的方法。 商业航空公司已经采用这种方法已有十多年了。
But the military’s progress toward enacting these policies has been slow. I observed first-hand how the following hampered adoption of modern technology:
但是军方在制定这些政策方面的进展缓慢。 我亲眼观察了以下因素如何阻碍现代技术的采用:
High demand for digital transformation, but efforts are fragmented and siloed: Competing interests and antiquated policies stifle adoption of effective software despite clear demand and myriad examples in the commercial sector. Disparate data sources are owned by disconnected stakeholders. Supply chain and maintenance policies and processes are insulated from each other.
尽管对数字化转型的需求很高,但是努力却是零散的和孤立的:尽管在商业领域有明确的需求和无数的例子,但竞争激烈的利益和过时的政策扼杀了有效软件的采用。 互不相关的利益相关者拥有不同的数据源。 供应链和维护策略与流程相互隔离。
Status quo is not truly AI/ML: Through my interactions with leaders in the Army, Air Force, and Navy, it became clear that adopted solutions, policies, and procedures over the last 20 years have focused on basic aggregation of historical data. Outside of prototypes and trials, there is no use of AI/ML to draw predictive insight from near-real-time sensor data in concert with historical maintenance data to inform maintenance and inventory practices.
现状并不是真正的AI / ML :通过我与陆军,空军和海军领导人的互动,很明显,过去20年采用的解决方案,政策和程序都集中在历史数据的基本汇总上。 除了原型和试验之外,没有使用AI / ML结合历史维护数据从近实时传感器数据中获取预测见解,从而为维护和库存实践提供依据。
What Predictive Solutions Require
预测解决方案需要什么
I joined C3.ai to build systems that help overcome these barriers to adoption, deliver mission capability to warfighters, and ultimately save lives. In my experience, the following capabilities are required to provide the DoD with accurate, AI-enabled predictive maintenance recommendations:
我加入C3.ai来构建有助于克服采用这些障碍,为战士提供任务能力并最终挽救生命的系统。 以我的经验,为国防部提供准确的,启用AI的预测性维护建议需要以下功能:
Platform as a service: Software should leverage today’s unique intersection between elastic cloud computing, big data, internet of things, and AI/ML advances to provide a scalable, unbounded platform that incorporates both current and future solutions.
平台即服务:软件应利用弹性云计算,大数据,物联网和AI / ML先进技术之间的当今独特交汇点,以提供可扩展的,不受限制的平台,该平台融合了当前和未来的解决方案。
True prediction in near real-time: Software should allow AI/ML models to be trained on structured and unstructured data such as maintenance history, high-frequency telemetry data, and handwritten logs. Those models can be leveraged to predict and forecast subsystem failures and supply requirements.
几乎实时的真实预测:软件应允许AI / ML模型在结构化和非结构化数据(例如维护历史记录,高频遥测数据和手写日志)上进行训练。 这些模型可用于预测和预测子系统故障和供应需求。
Rich models that provide AI-driven, action-oriented insights: Software should provide a rich user interface that offers customizable alerts for individual use cases to highlight issues and drive next steps. Software should be allowed to proactively aid maintainers and leadership in making decisions.
丰富的模型可提供AI驱动的,面向操作的见解:软件应提供丰富的用户界面,该界面可为各个用例提供可自定义的警报,以突出显示问题并推动下一步。 应该允许软件积极地帮助维护者和领导者进行决策。
Software that enables and complements highly trained technicians: Software should enhance, not usurp human judgment. A solution should complement the expertise of trained maintenance professionals and inform their decision-making by detecting anomalies and aligning maintenance and supply efforts.
支持并补充训练有素的技术人员的软件:软件应增强而不是篡改人类的判断力。 解决方案应补充经过培训的维护专业人员的专业知识,并通过检测异常并调整维护和供应工作来告知他们的决策。
C3.ai Develops Readiness Application
C3.ai开发就绪应用程序
In October 2017, C3.ai signed a prototype development contract with the DIU to develop a predictive maintenance solution for the U.S. Air Force. The resulting application — C3.ai™ Readiness — since has been deployed for use with the E-3 Sentry, the C-5M Galaxy, F-16 Fighting, and F-35 Lightning II aircraft fleets.
2017年10月,C3.ai与DIU签署了原型开发合同,以为美国空军开发预测性维护解决方案。 由此产生的应用程序-C3.ai™Readiness-已部署到与E-3 Sentry,C-5M Galaxy,F-16 Fighting和F-35 Lightning II机队一起使用。
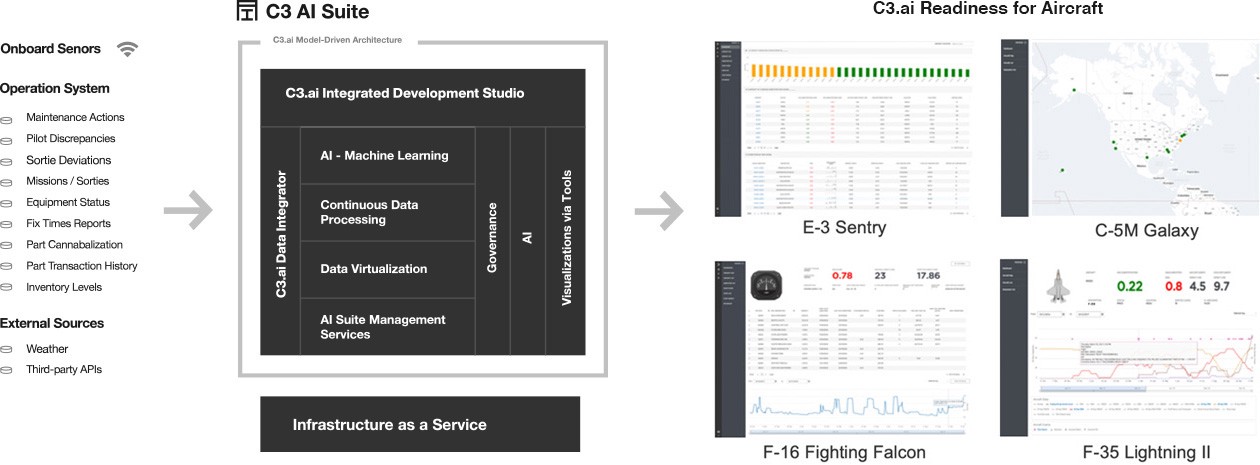
C3.ai Readiness integrates and unifies data from aircraft telemetry sensors, maintenance, and operational systems to monitor near-real time subsystem health. The application then predicts subsystem failures, provides root cause analysis, and forecasts part demand to ensure that maintenance can be executed at the right time and place, with the right parts at hand. The DoD benefits from reduced unscheduled maintenance, optimized maintenance schedules, reduced down time awaiting parts, and reduced in-flight aborts and precautionary emergency landings.
C3.ai Readiness集成并统一了来自飞机遥测传感器,维护和操作系统的数据,以监控近实时子系统的运行状况。 然后,该应用程序可以预测子系统故障,提供根本原因分析并预测零件需求,以确保可以在正确的时间和地点使用正确的零件进行维护。 国防部受益于减少计划外的维护,优化的维护计划,减少了等待零件的停机时间以及减少了飞行中止和预防性紧急着陆。
After the successful completion of the prototype phase of the E-3, C-5M, F-16, and F-35 platforms, C3.ai signed a production contract vehicle valued at $95 million with the DIU to use the application across the Department of Defense’s aircraft fleet.
在成功完成E-3,C-5M,F-16和F-35平台的原型阶段后,C3.ai与DIU签署了价值9500万美元的生产合同工具,以便在国防部范围内使用该应用程序国防部的机队。
We’ve since provided the ability to predict 40 percent of unscheduled maintenance events for fixed-wing, rotary-wing, and tiltrotors alike. Additionally, the model leverages the C3.ai multi-factor Mean Time Between Failure module to also surface root-cause analysis and component level insights. The marriage between failure prediction, root-cause analysis, and inventory optimization gives maintainers the ability to inform maintenance efforts and ensure minimal down-time.
此后,我们提供了对固定翼,旋翼和倾转旋翼等类似飞机的40%计划外维护事件进行预测的功能。 此外,该模型还利用C3.ai多因素平均故障间隔时间模块来显示根本原因分析和组件级见解。 故障预测,根本原因分析和库存优化之间的结合使维护人员能够通知维护工作并确保最少的停机时间。
Conclusion
结论
No matter how much emphasis we place on mission capability, aviation remains an inherently dangerous activity, particularly combat aviation. Manned aviation mishaps have spiked nearly 40 percent since 2013, and 133 service members have been killed over the last seven years. The military often requires its aviators to fly complex aircraft at the edge of the safety envelope, while also relying on its pilots to make snap decisions to compensate for in-flight failures as a result of suboptimal maintenance programs. These antiquated practices must be transformed. The DoD needs to continue leveraging AI-enabled technologies like C3.ai Readiness to provide a unified, scalable, and predictive solution to increase mission capability and reduce reliance on pilot heroics to bring broken aircraft home.
无论我们多么重视任务能力,航空都是固有的危险活动,特别是战斗航空。 自2013年以来,载人航空事故已激增近40%,在过去七年中有133名服务人员被杀。 军方经常要求其飞行员在安全护罩的边缘飞行复杂的飞机,同时还依靠飞行员做出Swift的决定,以补偿由于次优维护计划而导致的飞行中故障。 这些过时的做法必须转变。 国防部需要继续利用C3.ai Readiness等支持AI的技术来提供统一,可扩展和可预测的解决方案,以提高任务能力并减少对飞行员英雄的依赖,以使破碎的飞机回家。
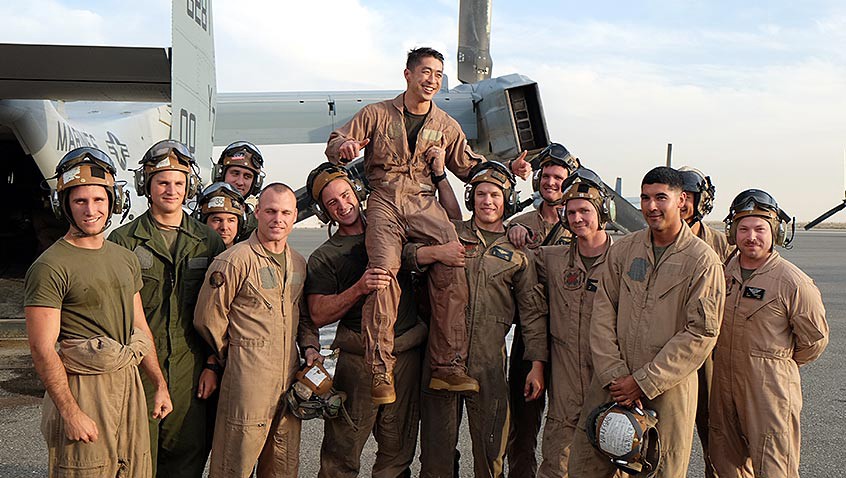
Philong Duong is a senior product manager at C3.ai. He holds an MBA from Columbia University and a bachelor’s in computer science from Elon University. Philong is a 15-year United States Marine Corps veteran and currently holds the rank of Major in the Selected Marine Corps Reserves. He co-founded and currently remains on the board of The Wingman Foundation, the nation’s first non-profit dedicated to serving families of fallen naval aviators and maintainers.
Philong Duong是C3.ai的高级产品经理。 他拥有哥伦比亚大学的MBA学位和埃隆大学的计算机科学学士学位。 Philong是一位在美国海军陆战队服役15年的老兵,目前在选定的海军陆战队后备队中担任少校职务。 他是Wingman Foundation的共同创立者,目前仍是该公司的董事会成员。WingmanFoundation是美国第一个致力于为坠落的海军飞行员和维修人员的家庭提供服务的非营利组织。
基于ai的预测