不使用机器学习的机器视觉
Marketing is an important aspect of any successful company. In simple words, marketing refers to the business’s activities related to buying and selling a product or service.
营销是任何成功公司的重要方面。 简而言之,营销是指与购买或出售产品或服务有关的企业活动。
以客户为中心 (Customer Centricity)
Customer centricity is putting your customer first and at the core of your business to provide the best possible experience and build long-term relationships.
以客户为中心将客户放在第一位,并将业务放在核心位置,以提供最佳的体验并建立长期的合作关系。
An organization with a customer-centric mindset has customer experience as a core value that helps in the decision-making process for leadership, reflects the beliefs that employees support, and provides clarity to current and potential customers.
一个以客户为中心的组织将客户体验作为核心价值,这有助于领导层的决策过程,反映员工支持的信念,并向当前和潜在客户提供清晰的信息。
以客户为中心的营销 (Customer-Centric Marketing)
Customer-centric marketing is an approach that prioritizes customers’ needs and interests in all decisions related to advertising, selling, and promoting products and services.
以客户为中心的营销是一种在与广告,销售以及促销产品和服务有关的所有决策中将客户的需求和兴趣放在优先位置的方法。
For successful customer-centric marketing, you need a deep understanding of the question: why do your customers need your product or service? The goal is not business growth alone; it is growth driven by convincing customers to believe that whatever your company provides can improve some aspect of their work or life.
为了成功进行以客户为中心的营销,您需要对以下问题有深刻的理解:为什么客户需要您的产品或服务? 我们的目标不只是业务增长。 通过说服客户相信公司提供的任何产品都可以改善他们工作或生活的某些方面来驱动增长。
机器学习-人工智能的子集 (Machine Learning — A Subset of Artificial Intelligence)
Artificial Intelligence (AI) — is a computer science field focused on making machines seem like they possess human intelligence. It is called “artificial” because humans create it, and it does not exist naturally.
人工智能(AI) —是一门计算机科学领域,致力于使机器看起来好像具有人类智能。 之所以称为“人工”,是因为人类创造了它,并且它不是自然存在的。
Machine Learning (ML) is a subset of AI. ML algorithms are computer-implementable instructions that take a dataset as input. They find out patterns within the dataset that were previously undiscovered.
机器学习(ML)是AI的子集。 ML算法是计算机可执行的指令,将数据集作为输入。 他们在数据集中找出以前未被发现的模式。
Supervised ML is when these patterns lead to some labels on the data, and your ML model learns how to map each unique pattern to the most appropriate label. This type of ML is used for predicting labels for unlabeled data.
监督式ML是这些模式在数据上导致一些标签的时候,您的ML模型学习如何将每个唯一的模式映射到最合适的标签。 这种类型的ML用于预测未标记数据的标签。
Unsupervised ML is when your model presents the discovered patterns to the users to provide insights.
无监督的ML是指您的模型将发现的模式呈现给用户以提供见解。
ML models improve their performance over time as they encounter more and more data. They improve by experience, just like a human would when indulging in a new activity through careful observation and self-correction.
随着ML模型遇到越来越多的数据,它们的性能会随着时间的推移而提高。 它们会随着经验的增长而提高,就像人类通过仔细观察和自我纠正沉迷于新活动时一样。
以客户为中心的营销的机器学习 (Machine Learning For Customer-Centric Marketing)
As competition gets tougher, consumers now have more choices of businesses to engage with, making ML evaluative to efficiently reaching and engaging your customers.
随着竞争的加剧,消费者现在可以选择更多的业务选择,从而使ML能够有效地吸引和吸引客户。
All business owners have noticed the growing hype around AI in marketing. AI applications in marketing include chatbots, content creation, programmatic advertising and a lot more.
所有企业主都注意到,在市场上围绕AI的炒作越来越多。 营销中的AI应用程序包括聊天机器人,内容创建,程序化广告等等。
With so many AI applications in marketing, you should never lose sight of what is essential in implementing effective and optimized marketing strategy i.e. deep and clear understanding of your customers.
在市场营销中有如此多的AI应用程序,您永远都不应忽视实施有效和优化的营销策略的基本要素,即对客户的深刻而清晰的了解。
Once your ML model is well trained, it can quickly categorize any new data inputs and predict likely outcomes. You can generate deeper customer insights and make better behavioral predictions.
一旦您的ML模型经过了良好的训练,就可以快速对所有新数据输入进行分类并预测可能的结果。 您可以产生更深刻的客户见解,并做出更好的行为预测。
These can be related to your prospects’ and customers’ probability of converting on certain campaigns, increasing their frequency of purchase, churn or lapse, or something more specific.
这些可能与您的潜在客户和客户转换某些广告系列,增加其购买,流失或失误的频率或更具体的内容有关的可能性有关。
Leveraging ML in your marketing strategy is no longer a luxury. Rather, it has become a necessity.
在您的营销策略中使用ML不再是奢侈。 相反,它已成为必需品。
Let’s take a look at ML-driven insights marketers are using to come up with best customer-centric marketing strategies.
让我们看看营销人员正在使用ML驱动的见解来提出最佳的以客户为中心的营销策略。
行为洞察力和预测 (Behavioral Insights and Predictions)
Which behavioral patterns of your customers led them to take a particular action in the past?
过去,您的客户的哪种行为方式使他们采取了特定措施?
Did the customers who ended their relationship with you developed behavioral patterns significantly different from those who remained loyal to you?
终止与您的关系的客户的行为模式是否与仍然忠于您的客户形成了明显的差异?
It is not easy for a human mind to figure out and study all these patterns. The mathematics behind all this is very complex.
人的头脑很难弄清楚并研究所有这些模式。 所有这些背后的数学非常复杂。
Therefore, your ML model takes care of this task. It can detect which piece of information about a customer has put how much weight on their decision to take a particular action, such as churning.
因此,您的ML模型可以完成此任务。 它可以检测到有关客户的哪些信息对他们采取特定行动(例如搅动)的决定有多大的影响。
It is important to anticipate your customer’s actions before they take them, especially when the action is irreversible. A lost customer is an irreversible loss. You should never have to begin facing the loss and then start thinking about reducing and preventing it.
重要的是在客户采取行动之前就对其进行预测,尤其是当这些行动不可逆转时。 失去客户是不可挽回的损失。 您永远不必开始面对损失,然后开始考虑减少和防止损失。
Supervised ML algorithms can discover predictive patterns hidden deep in your customer data.
监督的ML算法可以发现隐藏在客户数据深处的预测模式。
You can use them to find out which prospects are most likely to become your customers by training your model on the data of previous prospects who were successfully converted into customers and the prospects who were lost.
您可以通过使用模型将成功转换为客户的先前潜在客户和丢失的潜在客户的数据训练模型,从而使用它们找出最有可能成为您的客户的潜在客户。
Once your model is trained, your model can take potential customers’ data as input and predict the extent to which a new lead best “looks like” leads who were successfully converted into customers in the past.
一旦对模型进行了训练,您的模型就可以将潜在客户的数据作为输入,并预测在过去成功转换为客户的最佳“看起来”新潜在客户的程度。
Similarly, you can use supervised ML to predict if a customer is likely to churn. You can train your model on data of those customers who are already lost and those who are still active.
同样,您可以使用监督式ML来预测客户是否可能流失。 您可以根据已经丢失的客户和仍然活跃的客户的数据训练模型。
Your model can then take data of your current customers as input and figure out if any customer’s data shows patterns similar to those in data of lost customers.
然后,您的模型可以将当前客户的数据作为输入,并确定是否有任何客户的数据显示出与丢失客户的数据相似的模式。
The anticipation of your customers’ actions would allow you to come up with the most effective marketing campaigns according to the point they are at in their customer journey.
预期客户的行为将使您能够根据客户在客户旅程中所处的点来提出最有效的营销活动。
基于角色的见解和预测 (Persona-Based Insights and Predictions)
Although predicting your customer’s next move is very helpful in reaching the right people at the right time, it is not where the road to truly optimized marketing ends.
尽管预测客户的下一步行动对在正确的时间接触合适的人非常有帮助,但这并不是真正优化营销之路的终点。
To interact effectively with your customers, you need to know them as real people. You would then be able to provide hyper-personalized experiences that evoke emotional responses.
为了与客户进行有效互动,您需要了解他们是真实的人。 然后,您将能够提供唤起情感React的超个性化体验。
If you can show your audience that you understand well their reasons to interact with you, whether they are an early prospect or a loyal customer, the relationship and trust between you and your customers grow stronger.
如果您可以向受众群体表明您很好地理解了与您互动的原因,无论他们是早期潜在客户还是忠实的客户,您与客户之间的关系和信任就会增强。
市场细分 (Market Segmentation)
Customer segmentation is to divide your customers into segments. A customer from one segment is significantly different from a customer of another segment, based on the data used to carry out the segmentation process.
客户细分是将客户划分为多个细分。 根据用于执行细分过程的数据,一个细分市场的客户与另一细分市场的客户存在显着差异。
Unsupervised ML comes into play here. Clustering algorithms, such as K-Means, are part of unsupervised ML, used to discover hidden patterns within an unlabeled dataset and group data-points that are significantly similar.
无监督的ML在这里起作用。 聚类算法(例如K-Means)是无监督ML的一部分,用于发现未标记的数据集中的隐藏模式以及与数据点显着相似的组。
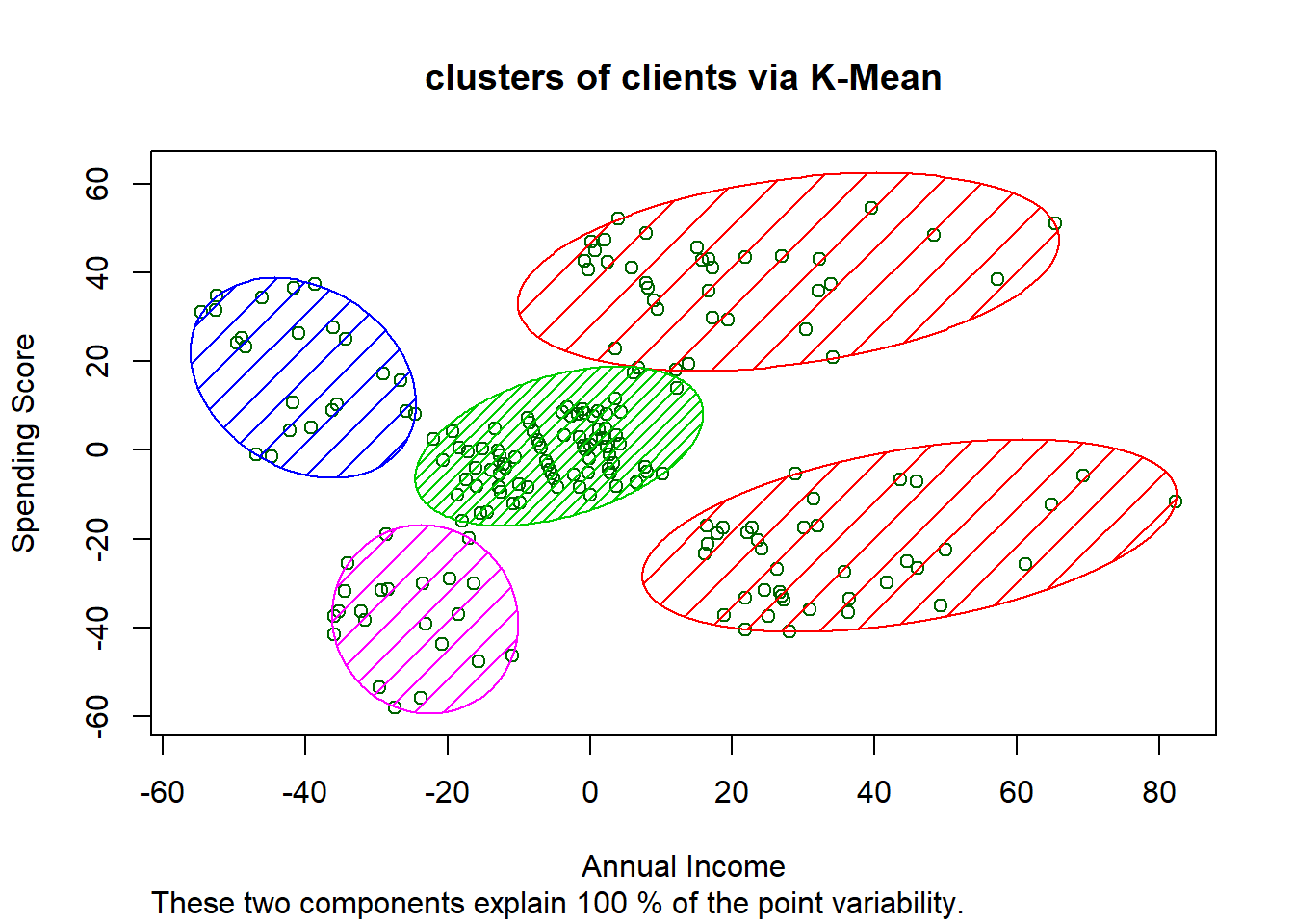
The figure above visualizes a very simple example of cluster formation using ML. The dataset included two pieces of information (attributes) about each customer.
上图显示了使用ML形成簇的非常简单的示例。 数据集包含有关每个客户的两条信息(属性)。
Based on the values of these attributes, each customer occupies their unique position within the two-dimensional representation above. It is two dimensional because there are two attributes.
基于这些属性的值,每个客户在上述二维表示中占据其唯一位置。 这是二维的,因为有两个属性。
The algorithm’s task is to figure out how to group these data-points into a number explicitly provided by the users of the algorithm.
该算法的任务是弄清楚如何将这些数据点分组为算法用户明确提供的数字。
The resulting groups, or clusters, could form the foundation of unbiased, truly data-driven personas. As you move forward and collect more data, rerunning the clustering algorithms may reveal new groups amongst your customers that emerge.
由此产生的组或群集可以构成无偏见,真正由数据驱动的角色的基础。 随着您前进并收集更多数据,重新运行群集算法可能会在出现的客户中揭示新的群体。
Therefore, you would be able to update your knowledge and refresh your messaging, creative, and other personalization efforts to always stay relevant as your customer base evolves.
因此,您将能够更新您的知识并刷新您的消息传递,创意和其他个性化工作,从而始终随着客户群的发展而保持相关性。
Let us tell you an interesting example that would clear how clustering can allow customer-centricity in marketing. Burrow, a disruptive direct-to-consumer furniture brand, employs ML-driven personas to identify what color couches their audience segments see in targeted ads.
让我们告诉您一个有趣的示例,该示例将阐明群集如何使营销以客户为中心。 Burrow是一家具有破坏性的直接面向消费者的家具品牌,它采用ML驱动的角色来识别其受众群体在目标广告中看到的颜色。
They discovered that older customers, living in single-family homes, with children were more inclined to buy dark-colored couches.
他们发现,住在独户住宅中且有孩子的年长顾客更倾向于购买深色沙发。
The younger customers, living in apartments, having few or no children were likely to buy light-colored couches.
居住在公寓中,几乎没有孩子或没有孩子的年轻顾客很可能会购买浅色的沙发。
These insights allowed Burrow to push creative that reflected these attributes to the audiences that possessed them.
这些见解使Burrow能够将反映这些属性的创意推向拥有它们的受众。
结论—使用机器学习可为公司节省数百万美元 (Conclusion — Use Of Machine Learning is Saving Millions For Companies)
Although many complex AI applications would need more years or even decades to develop fully, the democratization of ML is allowing marketing teams to generate predictive customer insights with no need of spending millions on expensive consultants or hire large data science teams.
尽管许多复杂的AI应用程序需要花费甚至数年甚至数十年的时间才能完全开发,但ML的民主化使营销团队无需花费数百万美元聘请昂贵的顾问或雇用大型数据科学团队即可生成预测性客户见解。
It is time for you to explore this option.
现在是您探索此选项的时候了。
翻译自: https://towardsdatascience.com/how-customer-centric-marketers-use-machine-learning-387df1a33850
不使用机器学习的机器视觉