时间序列预测 深度学习
介绍 (Introduction)
In any company, there is an embedded desire to predict its future revenue and future sales. The basic recipe is:
在任何公司中,都存在着预测其未来收入和未来销售额的内在愿望。 基本配方是:
Collect historical data related to previous sales and use it to predict expected sales.
收集与以前的销售有关的历史数据,并用它来预测预期的销售。
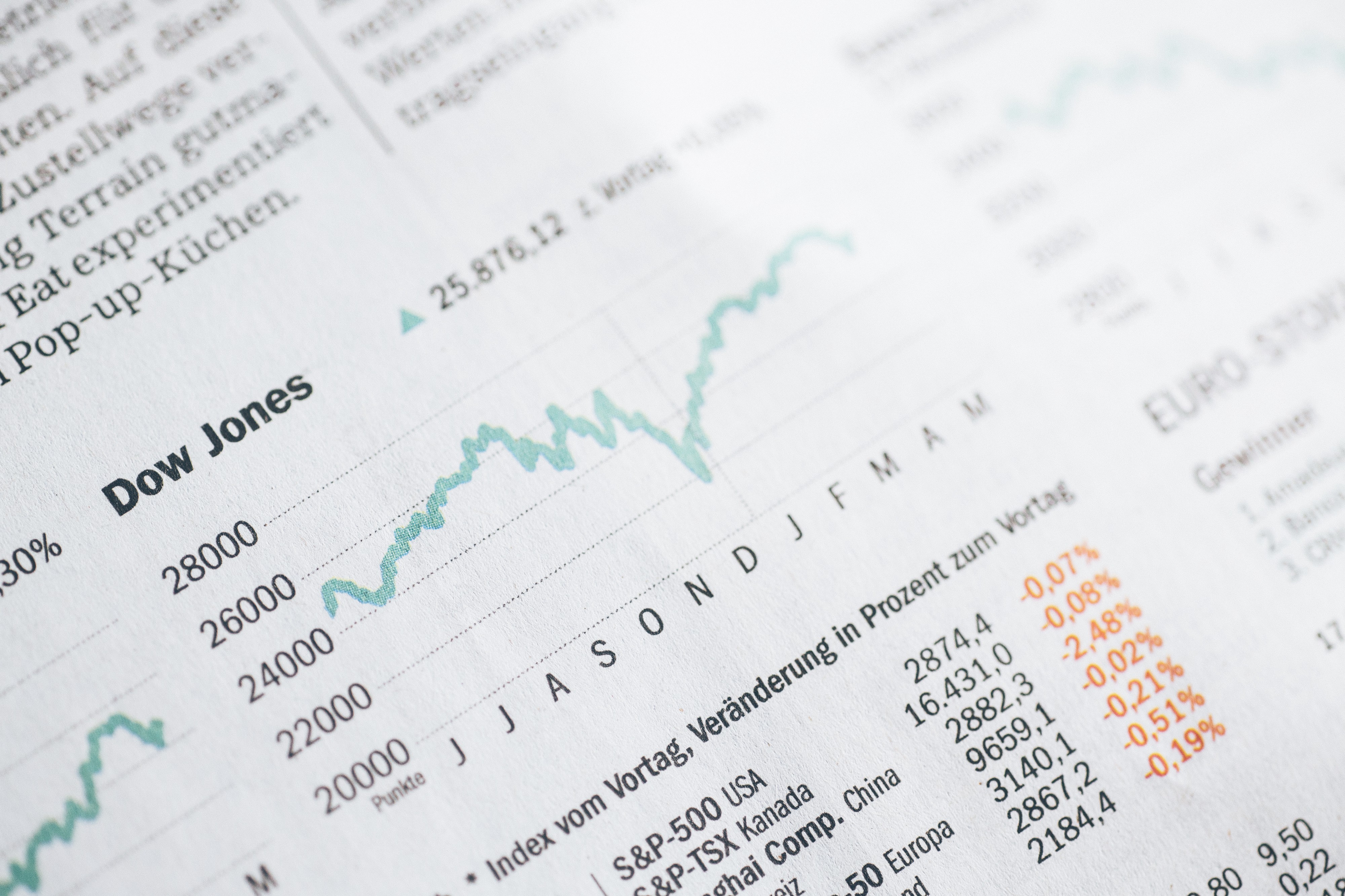
Over the last ten years, the rise of deep learning as the driving force behind all imaginable machine learning benchmarks revolutionized the field: be it in computer vision, language and so many others. Recently, one could argue that deep learning has restructured the potential future of sales forecasting by allowing models to encode for multiple time series in a single model as well as account for categorical variables. My goal today is:
在过去的十年中,深度学习的兴起成为所有可想象的机器学习基准背后的推动力,彻底改变了该领域:无论是在计算机视觉,语言还是许多其他领域。 最近,有人可能会争辩说,深度学习通过允许模型对单个模型中的多个时间序列进行编码并考虑分类变量,从而重构了销售预测的潜在未来。 我今天的目标是:
To walk you through the basic intuitions behind the main concepts and models for sales forecasting from a time-series perspective and discuss what kind of capabilities recent deep learning models could bring to the table.
从时间序列的角度向您介绍销售预测的主要概念和模型背后的基本直觉,并讨论最近的深度学习模型可以带来哪些功能。
阅读建议 (Reading Suggestions)
In case you feel like you need to brush up on the basics of sales forecasting and time-series, I recommend these 3 reads:
如果您觉得需要重新了解销售预测和时间序列的基础知识,我建议您阅读以下三篇文章:
Harvard business article on the fundamentals of sales forecasting.
哈佛商业文章中有关销售预测的基础知识。
TDS article by @Marco Peixeiro from on @Towards Data Science (really comprehensive and instructive).
@Marco Peixeiro在@Towards Data Science上发表的TDS文章 (非常全面且具有指导意义)。
“Time Series Forecasting Principles with Amazon Forecast”. They do a thorough job of explaining how Sales Forecasting works, as well as what are the challenges and problems one might encounter in the field.
“使用Amazon Forecast进行时间序列预测的原理” 。 他们做了详尽的工作,解释了“销售预测”的工作原理以及该领域可能遇到的挑战和问题。
销售预测问题 (The Sales Forecasting Problem)
Sales forecasting is all about using historical data to inform decision making.
销售预测是关于使用历史数据来指导决策的全部。
A simple forecasting cycle looks like this:
一个简单的预测周期如下所示:

On its core, this is a time series problem: given some data in time, we want to predict the dynamics of that same data in the future. To do this, we require some trainable model of these dynamics.
从根本上讲,这是一个时间序列问题:给定一些及时的数据,我们希望将来预测相同数据的动态。 为此,我们需要一些动态的可训练模型。
According to Amazon’s time series forecasting principles, forecasting is a hard problem for 2 reasons:
根据Amazon的时间序列预测原则 ,由于以下两个原因,预测是一个难题:
- Incorporating large volumes of historical data, which can lead to missing important information about the past of the target data dynamics. 合并大量历史数据可能会导致丢失有关目标数据动态变化的过去的重要信息。
- Incorporating related yet independent data (holidays/events, locations, marketing promotions) 整合相关但独立的数据(节假日/活动,位置,营销促销)
Besides these, one of the central aspects of sales forecasting is that accuracy is key:
除此之外,销售预测的主要方面之一是准确性是关键:
- If the forecast is too high it may lead to over-investing and therefore losing money. 如果预测值太高,则可能导致过度投资,从而造成资金损失。
- If the forecast is too low it may lead to under-investing and therefore losing opportunity. 如果预测值太低,则可能导致投资不足,从而失去机会。
Incorporating exogenous factors like the weather, time and spatial location could be beneficial for a prediction. In this medium piece by Liudmyla Taranenko, she mentions a great example discussing how on-demand ride services like UBER, Lyft or Didi Chuxing must take into account factors like weather conditions (like humidity and temperature), time of the day or day of the week to do its demand forecasting. Therefore, good forecasting models should have mechanisms that enable them to account for such factors.
结合天气,时间和空间位置等外在因素可能有助于预测。 在这种媒介片由Liudmyla Taranenko ,她提到了一个很好的例子,讨论如何点播喜欢UBER,Lyft或迪迪楚星程服务,必须考虑多种因素,如天气条件(如温度和湿度)的一天或一天的时间一周进行需求预测。 因此,良好的预测模型应具有使它们能够考虑这些因素的机制。
In sum, what do we know so far?
总而言之,到目前为止我们知道什么?
- We know that forecasting is a hard problem where accuracy really matters. 我们知道,预测是一个很难解决的问题,其中准确性至关重要。
- We know that there are exogenous factors that come into play that are hard to account for. 我们知道,有些外在因素在起作用,很难解释。
What we don’t know yet is:
我们还不知道的是:
- What are the traditional forecasting methods and why they might succumb to these challenges. 传统的预测方法是什么,为什么它们会屈服于这些挑战。
- How is it that deep learning methods could help, and what are some of the prospects to replace traditional models. 深度学习方法将如何提供帮助,以及替代传统模型的前景如何?
预测模型的类型 (Types of Forecasting Models)
According to this article featured in the Harvard business review, there are three types of Forecasting techniques:
根据《哈佛商业评论》上的这篇文章 ,有三种类型的预测技术:
Qualitative techniques: usually involve expert opinion or information about special events.
定性技术 :通常涉及专家意见或有关特殊事件的信息。
Time series analysis and projection: involve historical data, finding structure in the dynamics of the data like cyclical patterns, trends and growth rates.
时间序列分析和预测 :涉及历史数据,在数据动态中寻找结构,例如周期性变化,趋势和增长率。
Causal models: these models involve the relevant causal relationships that may include pipeline considerations like inventories or market survey information. They can incorporate the results of a time series analysis.
因果模型 :这些模型涉及相关的因果关系,其中可能包括管道方面的考虑,例如库存或市场调查信息。 他们可以合并时间序列分析的结果。
We will focus on the time series analysis approach which has been the driving force behind traditional forecasting methods and it can give a comprehensive layout of the forecasting landscape.
我们将重点关注时间序列分析方法,该方法一直是传统预测方法的推动力,并且可以对预测格局进行全面布局。
时间序列方法 (Time Series Approach)
A time series is a sequence of data points taken at successive, equally-spaced points in time that can be used to predict the future. A time series analysis model involves using historical data to forecast the future. It looks in the dataset for features such as trends, cyclical fluctuations, seasonality, and behavioral patterns.
时间序列是在连续的等间隔时间点获取的数据点序列,可用于预测未来。 时间序列分析模型涉及使用历史数据来预测未来。 它在数据集中查找诸如趋势,周期性波动,季节性和行为模式等特征。
The three key general ideas that are fundamental to consider, when dealing with a sales forecasting problem tackled from a time series perspective, are:
在处理从时间序列角度解决的销售预测问题时,需要考虑的三个基本基本概念是:
- Repeating patterns 重复图案
- Static patterns 静态模式
- Trends 发展趋势
Now we’ll look into each of these factors