重点(Top highlight)
环境(ENVIRONMENT)
“We are the first generation to be able to end poverty and the last generation that can take steps to avoid the worst impacts of climate change. Future generations will judge us harshly if we fail to uphold our moral and historical responsibilities. “– Ban Ki-moon, Former-Secretary General UN.
“我们是能够消除贫困的第一代人,也是可以采取措施避免气候变化最严重影响的最后一代人。 如果我们不履行我们的道德和历史责任,后代将严厉地审判我们。 “ –联合国前秘书长潘基文。
Do you know that climate change caused nearly 70% of all the extreme weather events in the last 20 years?
您是否知道在过去20年中,气候变化导致了近70%的极端天气事件?
Global warming refers to an increase in the average long-term temperature of Earth’s climate. The expansion of physical factors, such as CO2, CH4, and N20, has triggered a substantial shift. The higher emission of greenhouse gases has led the Earth’s temperature to rise sharply since 1990.
全球变暖是指地球气候的长期平均温度升高。 物理因素(例如CO2,CH4和N20)的膨胀引发了重大变化。 自1990年以来,温室气体排放量增加,导致地球温度急剧上升。
Compared to 100 years ago, the average temperature of Earth is 1 degree Celsius higher. Climate scientists fear that in the next 200 years, the average global temperature will rise by almost 6 degrees Celsius.
与100年前相比,地球的平均温度高出1摄氏度。 气候科学家担心,在未来200年中,全球平均温度将上升近6摄氏度。
To handle global warming, countries need an effective action plan. For this, they’re heavily relying on real-time data analytics. Predictive analytics offers considerable promise in predicting global warming trends.
为了应对全球变暖,各国需要有效的行动计划。 为此,他们严重依赖实时数据分析。 预测分析为预测全球变暖趋势提供了可观的前景。
There is a reason why climate scientists are increasingly leveraging data analytics and machine learning. According to research, these models are more affordable and accurate in the following scenarios:
气候科学家越来越多地利用数据分析和机器学习是有原因的。 根据研究,在以下情况下,这些模型更实惠且更准确:
- When there are large amounts of data, but traditional statistics are not significant enough to model systems. 当有大量数据时,但是传统统计数据不足以对系统进行建模。
- When there are good models, it costs an arm and leg to compute them through conventional production methods. 当有好的模型时,通过传统的生产方法来计算它们会花费很多时间。
Climate scientists have used data analytics to identify pollutant sources, classify crop cover, and calibrate satellite sensors. Deep learning, one of the critical branches of machine learning, could help with super-resolution, pattern recognition, and forecasting in global warming, and compile data of environmental imagery to speed up data analytics in the field.
气候科学家已经使用数据分析来识别污染物来源,对农作物的覆盖范围进行分类并校准卫星传感器。 深度学习是机器学习的重要分支之一,可以帮助解决全球变暖带来的超分辨率,模式识别和预测,并编译环境图像数据以加快现场数据分析的速度。
Both public and private sector organizations have been creating cutting-edge tools and technologies to fight against global warming. Voluminous amounts of data of various variables, such as carbon emissions, forest cover, sea levels, and temperature change, are stored and analyzed in real-time. These tools can identify the correlation between variables, recommend actionable insights, and generate patterns and predictions. In this way, appropriate proactive precautions or actions can be addressed timely.
公共和私营部门组织都在创造尖端的工具和技术来对抗全球变暖。 实时存储和分析各种变量的大量数据,例如碳排放量,森林覆盖率,海平面和温度变化。 这些工具可以识别变量之间的相关性,推荐可行的见解,并生成模式和预测。 这样,可以及时解决适当的主动预防措施或行动。
做出更好的预测 (Making better predictions)
The push to adopt data analytics builds on the work performed in climate informatics, a field developed in 2011 that merges climate science and data analytics. This discipline covers a wide range of topics, including enhancing prediction of extreme events, such as floods, paleoclimatology — reimagining historical climate conditions by using data extracted from things, such as climate downscaling, ice cores, and utilizing large-scale models to make predictions on a hyper-local level and study the socio-economic effects of climate and weather.
推动采用数据分析的基础是在气候信息学领域开展的工作,该领域于2011年开发,将气候科学和数据分析融合在一起。 该学科涵盖了广泛的主题,包括增强对洪水,古气候等极端事件的预测-通过使用从诸如降尺度,冰芯之类的事物中提取的数据来重新构想历史气候条件,并利用大规模模型进行预测在超地方层面上研究气候和天气的社会经济影响。
Data analytics can generate hidden and valuable insights from the massive amounts of problematic climate and global warming simulations created by climate modeling.
数据分析可以从大量有问题的气候和气候模型创建的全球变暖模拟中生成隐藏且有价值的见解。
One of the earliest climate change simulations developed at Princeton University in the 1960s, these models represent ice, cryosphere, land, oceans, and atmosphere. Despite the agreement on basic scientific assumptions, Claire Monteleoni, a computer science professor from the University of Colorado, Boulder, is not satisfied with their accuracy, especially for long-term forecasts. She said, “There’s a lot of uncertainty. They don’t even agree on how precipitation will change in the future.”
作为普林斯顿大学在1960年代开发的最早的气候变化模拟之一,这些模型代表了冰,冰冻圈,陆地,海洋和大气。 尽管在基本科学假设上达成了共识,但来自科罗拉多大学博尔德分校的计算机科学教授克莱尔·蒙特莱奥尼(Claire Monteleoni)对它们的准确性并不满意,尤其是对于长期预报。 她说:“存在很多不确定性。 他们甚至不同意未来的降水量如何变化。”
Monteleoni has used data analytics to combine the predictions of around 30 climate models for making better predictions.
Monteleoni使用数据分析来组合大约30个气候模型的预测,以便做出更好的预测。
数据分析如何帮助应对全球变暖? (How is data analytics helping against global warming?)
Data analytics can counter the threat of global warming. One of the prominent examples is the work done by Climate Central, an independent non-profit organization. They developed Surging Seas, an interactive map that shows the information on the rising sea levels in the U.S. Open the map; you can observe accurate sea levels in different areas, view historical data, action plans, and flood warnings. The tool predicts that Miami Beach will go underwater due to rising sea levels shortly.
数据分析可以应对全球变暖的威胁。 著名的例子之一是独立的非营利组织Climate Central所做的工作。 他们开发了Surging Seas ,这是一个交互式地图,显示了美国境内不断上升的海平面信息。 您可以观察不同区域的准确海平面,查看历史数据,行动计划和洪水预警。 该工具预测,由于海平面上升,迈阿密海滩将很快进入水下。
Illegal logging is one of the primary factors causing deforestation. Rainforest Connection (RFCx) utilizes data analytics and cell phones to control deforestation. They created acoustic monitoring systems to safeguard a rainforest area, enabling them to improve their responses via real-time alerts. RFCx works with TensorFlow, a machine learning framework, to analyze forest sounds in real-time and identify sounds that resemble logging trucks, chainsaws, and similar illegal activity noises to locate forest issues.
非法采伐是造成森林砍伐的主要因素之一。 Rainforest Connection(RFCx)利用数据分析和手机来控制森林砍伐。 他们创建了声音监控系统来保护雨林地区,从而使他们能够通过实时警报来改善响应。 RFCx与机器学习框架TensorFlow一起实时分析森林的声音,并识别类似于伐木车,链锯和类似非法活动噪音的声音来定位森林问题。
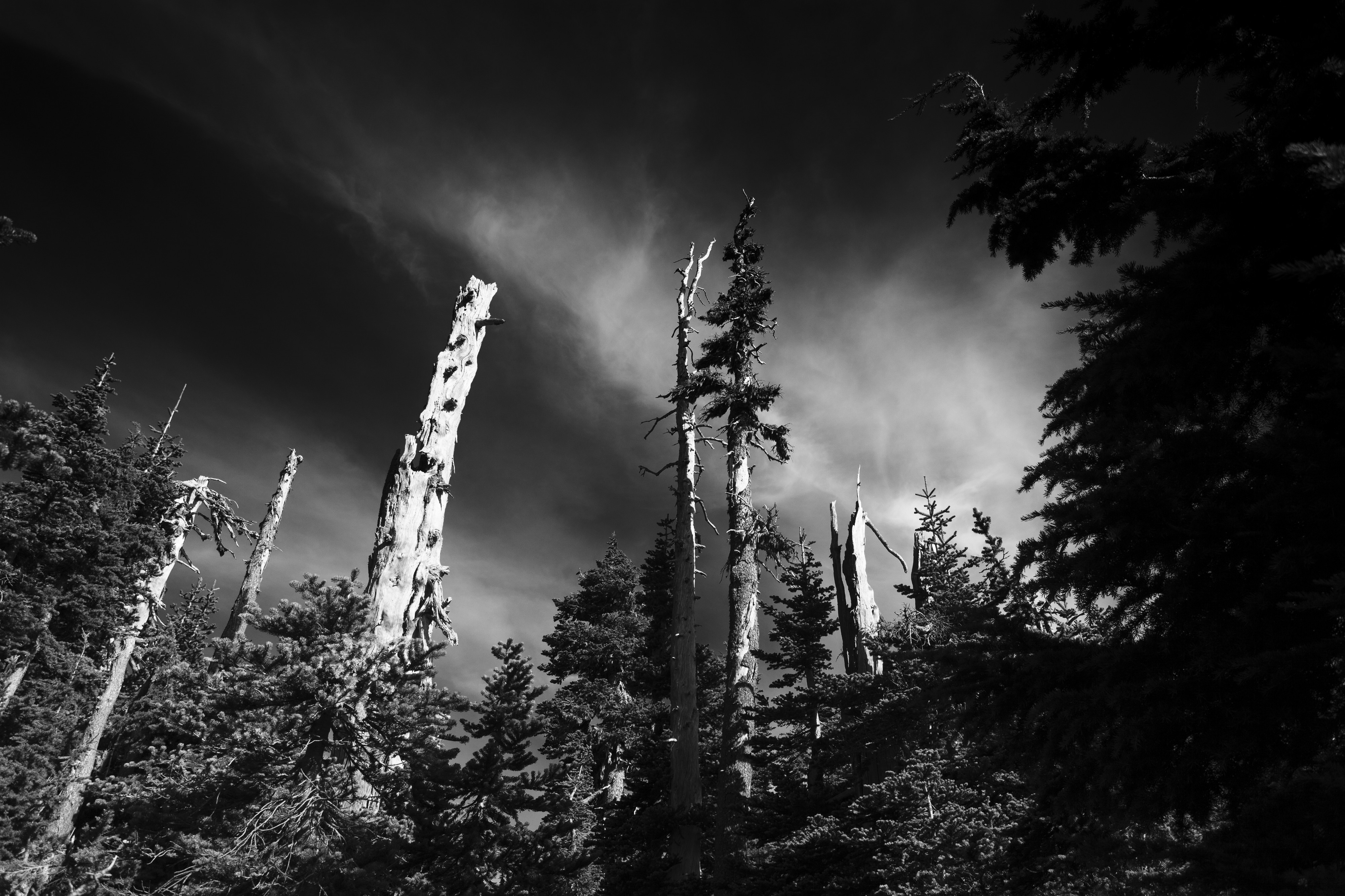
Maria Uriarte, a professor of Environment Biology from Columbia’s University, and Tian Zheng, a Statistics professor at the Data Science Institute, used data analytics and AI to examine Hurricane Maria’s impact on the El Yunque National Forest in Puerto Rico. The study’s motivation was to find how tropical storms influence tree species’ distribution and notice their influence on global warming and climate change.
哥伦比亚大学环境生物学教授Maria Uriarte和数据科学研究所统计学教授Tian Zheng使用数据分析和AI检验了飓风Maria对波多黎各El Yunque国家森林的影响。 该研究的目的是发现热带风暴如何影响树种的分布并注意到它们对全球变暖和气候变化的影响。
Due to Hurricane Maria‘s extensive damage, the only viable way to find the affected species was to go through countless high-resolution images. Nevertheless, a particular dilemma existed: how to distinguish one species from another when only a green mass was visible in a large area?
由于飓风玛丽亚遭受了广泛的破坏,找到受影响物种的唯一可行方法是浏览无数的高分辨率图像。 但是,仍然存在一个特殊的难题:当在大范围内仅可见绿色块时,如何将一个物种与另一个物种区分开?
They used AI and data analytics to analyze the high-resolution images and compared them with a dataset — it identified each tree species in given plots and mapped them accordingly. The ground data from fixed fields helped them determine how different species looked from above in the aerial images.
他们使用AI和数据分析来分析高分辨率图像,并将其与数据集进行比较-识别给定地块中的每种树种并相应地对其进行映射。 固定场的地面数据帮助他们确定了航空图像中从上方看不同物种的方式。
Figuring out how modern storms affect the composition and distribution of forests is necessary for global warming. When a hurricane damages forests, it forces the vegetation to decompose, emitting more significant amounts of CO2 into the atmosphere.
弄清楚现代风暴如何影响森林的组成和分布是全球变暖的必要条件。 当飓风破坏森林时,它迫使植被分解,向大气中排放大量二氧化碳。
As trees grow again in the storm’s aftermath, they store less carbon due to their smaller size. Hence, as climate change causes more storms, lesser carbon will be stored and more will be released—ultimately worsening global warming.
随着风暴过后树木的再次生长,由于树木较小,它们储存的碳更少。 因此,随着气候变化引发更多的风暴,更少的碳将被存储,更多的碳将被释放,最终加剧了全球变暖。
Data analytics can play a pivotal role in creating more livable and sustainable cities. It can enhance a city’s energy efficiency by processing data collected from IoT equipment, such as smart meters. In this way, it can forecast energy demand.
数据分析在创建更宜居,更可持续的城市中可以发挥关键作用。 通过处理从智能电表等物联网设备收集的数据,它可以提高城市的能源效率。 这样,它可以预测能源需求。
Smart solutions can empower authorities to simulate potential zoning laws, build flood plains, and work on disaster preparedness and urban planning. A sustainable city’s administration can envision a state-of-the-art analytics dashboard that shows real-time data on energy use, water availability, weather, and traffic to make cities more livable and efficient.
聪明的解决方案可以使主管部门模拟潜在的分区法律,建造洪泛区,并进行备灾和城市规划工作。 可持续城市的管理部门可以构想一个最新的分析仪表板,该仪表板显示有关能源使用,水的可利用性,天气和交通的实时数据,以使城市更加宜居和高效。
In China, the Green Horizon project (developed by IBM) can monitor pollution sources, forecast air pollution, and create potential plans. For instance, it can suggest whether it is better to close specific power plants or limit drivers to minimize pollution levels within a particular area. IBM is working on another system to aid cities with predicting future heat waves.
在中国,“绿色地平线”项目(由IBM开发)可以监视污染源,预测空气污染并制定潜在计划。 例如,它可以建议关闭特定的发电厂还是限制驱动程序以最小化特定区域内的污染水平是否更好。 IBM正在开发另一个系统,以帮助城市预测未来的热浪。
It would simulate the climate at the urban scale and study a diverse set of strategies to test how they ease heat waves. For instance, data analytics and machine learning models could determine the best areas to plant new trees to enhance optimal tree cover and reduce heat from the pavement.
它将模拟城市规模的气候,并研究各种策略来测试它们如何缓解热浪。 例如,数据分析和机器学习模型可以确定种植新树木的最佳区域,以增强最佳树木覆盖率并减少人行道产生的热量。
没有数据分析的世界 (A world without data analytics)
Without data analytics, plans, or policies for tackling global warming becomes one-dimensional. Hypothetically, possible scenarios include:
没有数据分析,解决全球变暖的计划或政策将变成一维。 假设地,可能的情况包括:
- The calculations for reducing carbon emissions could be affected. Consider a system where companies unanimously agree to enforce a law that requires carbon emissions to minimize carbon emissions produced by industrial plants, cars, and other sources by 3% in the next ten years. However, the actual requirement was to cut carbon emissions by 6%, and thus flawed calculations will exacerbate global warming. 减少碳排放的计算可能会受到影响。 考虑一个系统,在该系统中,公司一致同意执行一项法律,该法律要求碳排放量在未来十年内将工厂,汽车和其他来源产生的碳排放量减少3%。 但是,实际要求是将碳排放量减少6%,因此,错误的计算将加剧全球变暖。
- Glaciers are melting rapidly, and sea levels are rising faster than ever, placing coastal regions under serious risk. If predictive analytics is not applied, the relevant authorities could fail to take proactive housing relocation and rehabilitation planning steps. 冰川正在Swift融化,海平面上升的速度比以往任何时候都要快,这使沿海地区面临严重的风险。 如果不应用预测分析,则有关当局可能无法采取主动的房屋搬迁和恢复计划步骤。
最后的想法 (Final thoughts)
At present, there’s no doubt that data analytics is redefining climate change policies. Today, it has become a core component of plans and procedures designed to address and predict global warming trends. Data analytics’ potential for analyzing large amounts of complex climate data, identifying hidden correlations, and displaying real-time insights is essential.
目前,毫无疑问,数据分析正在重新定义气候变化政策。 今天,它已成为旨在解决和预测全球变暖趋势的计划和程序的核心组成部分。 数据分析在分析大量复杂气候数据,识别隐藏的相关性以及显示实时洞察力方面的潜力至关重要。
However, data analytics can only do so much. Ultimately, it’s up to the concerned authorities to implement it for the greater good.
但是,数据分析只能做很多事情。 最终,要实现更大的利益,取决于有关当局。
Remember, there’s no planet B.
记住,没有行星B。
翻译自: https://medium.com/swlh/the-future-of-global-warming-lies-in-data-analytics-63d255d6b62c