pca 主成分分析
TL;DR: PCA cannot handle categorical variables because it makes linear assumptions about them. Nonlinear PCA addresses this issue by warping the feature space to optimize explained variance. (Key points at bottom.)
TL; DR: PCA无法处理分类变量,因为它对它们进行了线性假设。 非线性PCA通过扭曲特征空间以优化解释的方差来解决此问题。 (关键点在底部。)
Principal Component Analysis (PCA) has been one of the most powerful unsupervised learning techniques in machine learning. Given multi-dimensional data, PCA will find a reduced number of n uncorrelated (orthogonal) dimensions, attempting to retain as much variance in the original dataset as possible. It does this by constructing new features (principle components) as linear combinations of existing columns.
主成分分析(PCA)是机器学习中最强大的无监督学习技术之一。 给定多维数据,PCA将发现数量减少的n个不相关(正交)维,尝试在原始数据集中保留尽可能多的方差。 它通过将新功能(原理组件)构造为现有列的线性组合来实现。
However, PCA cannot handle nominal — categorical, like state — or ordinal — categorical and sequential, like letter grades (A+, B-, C, …) — columns. This is because a metric like variance, which PCA explicitly attempts to model, is an inherently numerical measure. If one were to use PCA on data with nominal and ordinal columns, it would end up making silly assumptions like ‘California is one-half New Jersey’ or ‘A+ minus four equals D’, since it must make those kinds of relationships to operate.
但是,PCA无法处理名义(类别,如状态)或排序(类别和顺序),如字母等级(A +,B-,C等)的列。 这是因为PCA明确尝试建模的类似方差的度量标准是固有的数字度量。 如果要在具有标称和序数列的数据上使用PCA,最终将做出愚蠢的假设,例如“加利福尼亚州是新泽西州的一半”或“ A +减去四等于D”,因为它必须使这种关系起作用。
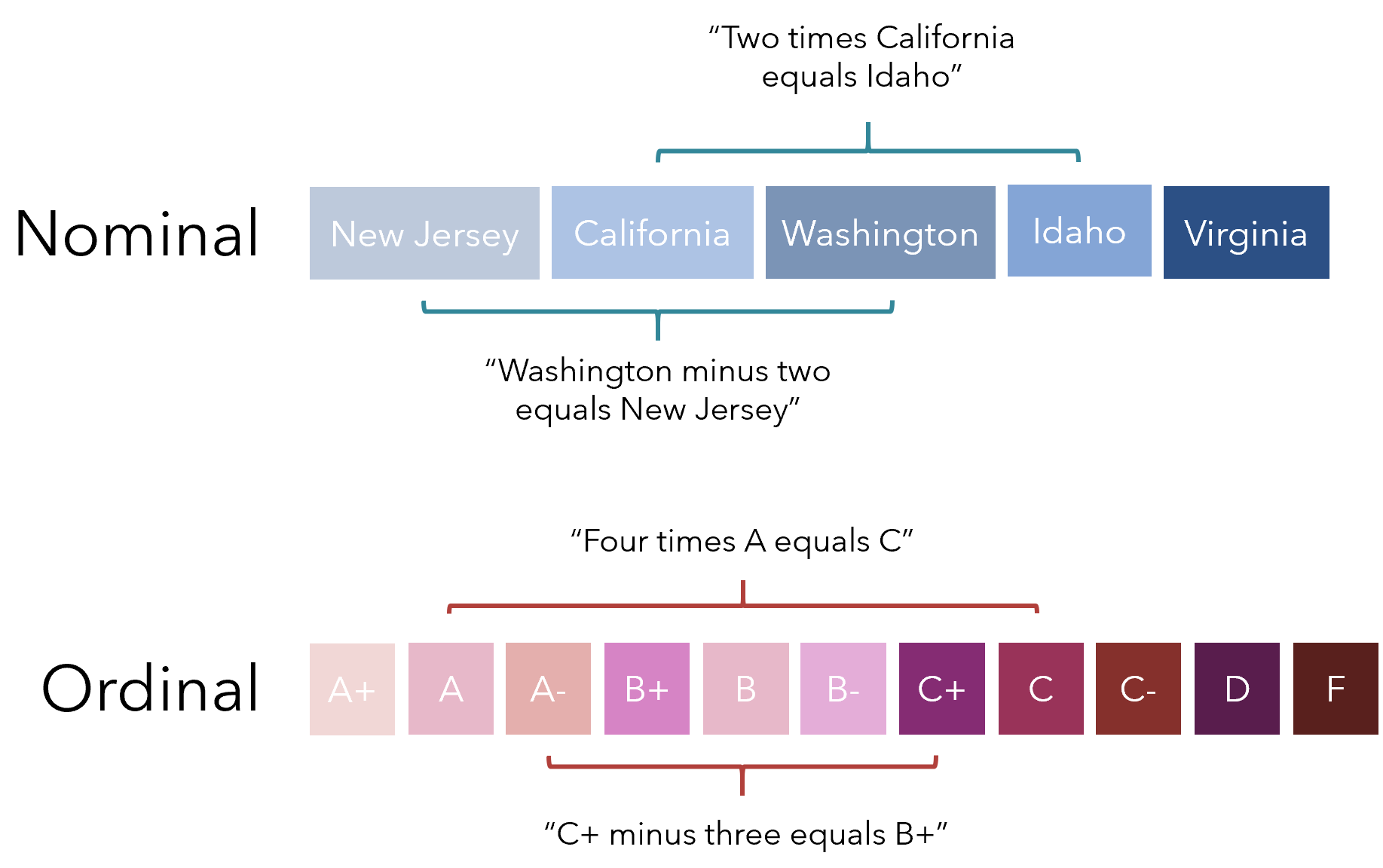
Rephrased in relation to a mathematical perspective, PCA relies on linear relationships, that is, the assumption that the distance between “