ai伪造论文实验数据
Many data scientists claim that around 80% of their time is spent on data preprocessing, and for good reason; collecting, annotating, and formatting data are crucial tasks in machine learning. This article will help you understand the importance of these tasks, as well as learn methods and tips from other researchers.
许多数据科学家声称,大约有80%的时间用于数据预处理,这是有充分理由的。 收集,注释和格式化数据是机器学习中的关键任务。 本文将帮助您了解这些任务的重要性,并向其他研究人员学习方法和技巧。
Below, we will highlight academic papers from reputable universities and research teams on various training data topics. The topics include the importance of high-quality human annotators, how to create large datasets in a relatively short time, ways to securely handle training data that may include private information, and more.
下面,我们将重点介绍来自知名大学和研究团队的有关各种培训数据主题的学术论文。 主题包括高质量人工注释者的重要性,如何在相对较短的时间内创建大型数据集,安全处理可能包含私人信息的培训数据的方式等。
1.人工注释者有多重要? (1. How Important are Human Annotators?)
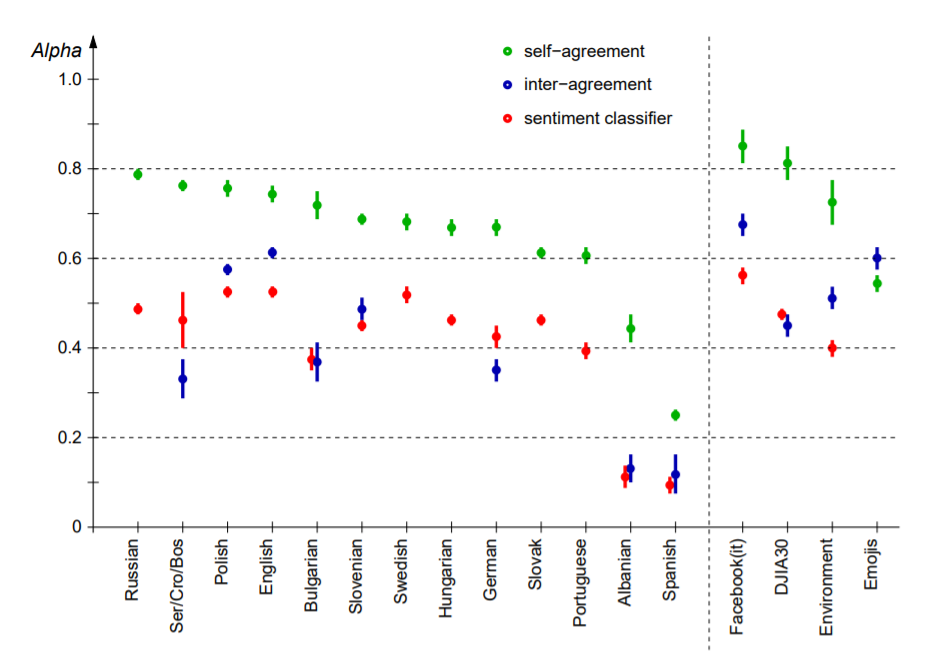
This paper presents a firsthand account of how annotator quality can greatly affect your training data, and in turn, the accuracy of your model. In this sentiment classification project, researchers from the Jožef Stefan Institute analyze a large dataset of sentiment-annotated tweets in multiple languages. Interestingly, the findings of the project state that there was no statistically major difference between the performance of the top classification models. Instead, the quality of the human annotators was the larger factor that determined the accuracy of the model.
本文提供了有关注释器质量如何极大地影响您的训练数据以及模型准确性的第一手资料。 在这个情感分类项目中,JožefStefan Institute的研究人员分析了多种带有多种语言的带有情感注释的推文的数据集。 有趣的是,该项目的发现表明,顶级分类模型的性能在统计上没有重大差异。 相反,人工注释者的质量是决定模型准确性的更大因素。
To evaluate their annotators, the team used both inter-annotator agreement processes and self- agreement processes. In their research, they found that while self-agreement is a good measure to weed out poor-performing annotators, inter-annotator agreement can be used to measure the objective difficulty of the task.
为了评估其注释者,团队使用了注释者间协议过程和自我协议过程。 在他们的研究中,他们发现,虽然自我约定是清除表现不佳的注释者的好方法,但注释者之间的共识可以用来衡量任务的客观难度。
Research Paper: Multilingual Twitter Sentiment Classification: The Role of Human Annotators
研究论文 : 多语言Twitter情感分类:人类注释者的作用
Authors / Contributors: Igor Mozetic, Miha Grcar, Jasmina Smailovic (all authors from the Jozef Stefan Institute)
作者/撰稿人: Igor Mozetic,Miha Grcar,Jasmina Smailovic(所有作者均来自Jozef Stefan Institute)
Date Published / Last Updated: May 5, 2016
发布日期/最近更新日期: 2016年5月5日
2.机器学习数据收集调查 (2. A Survey On Data Collection for Machine Learning)
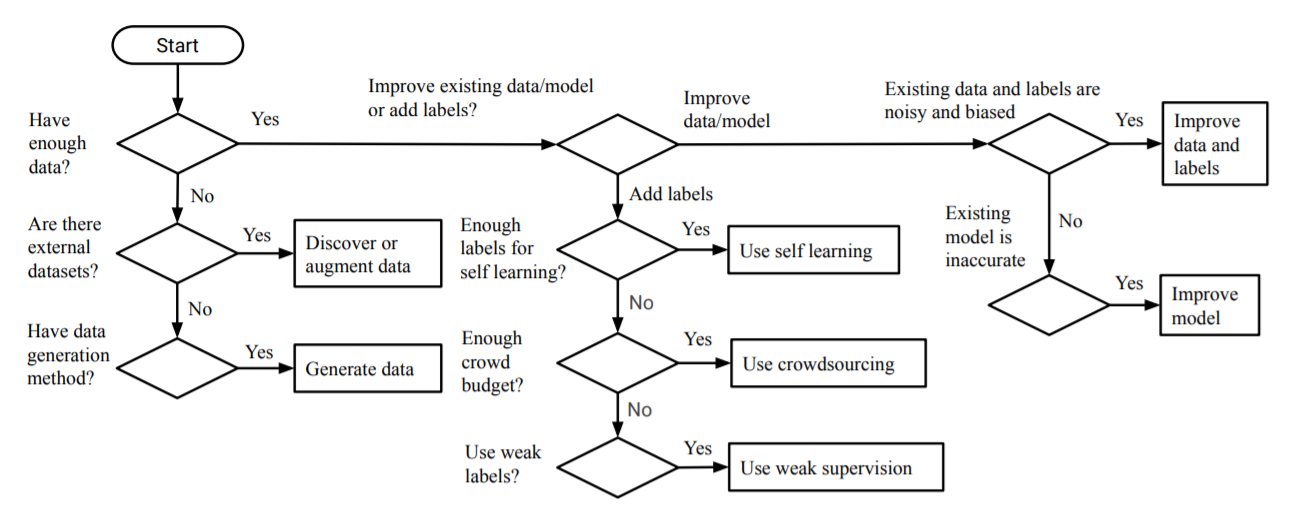
From a research team at the Korean Advanced Institute of Science and Technology, this paper is perfect for beginners looking to get a better understanding of the data collection, management, and annotation landscape. Furthermore, the paper introduces and explains the processes of data acquisition, data augmentation, and data generation.
本文由韩国高级科学技术研究院的研究团队提供,非常适合希望对数据收集,管理和注释领域有更好了解的初学者。 此外,本文还介绍并解释了数据获取,数据扩充和数据生成的过程。
For those new to machine learning, this paper is a great resource to help you learn about many of the common techniques to create high-quality datasets used in the field today.
对于那些不熟悉机器学习的人来说,本文是一个非常有用的资源,可以帮助您了解创建当今该领域中使用的创建高质量数据集的许多常用技术。
Research Paper: A Survey on Data Collection for Machine Learning
研究论文 : 机器学习数据收集调查
Authors / Contributors: Yuji Roh, Geon Heo, Steven Euijong Whang (all authors from KAIST)
作者/撰稿人 :余宇治,姜,,史蒂文欧钟(所有来自KAIST的作者)
Date Published / Last Updated: August 12th, 2019
发布日期/最后更新日期: 2019年8月12日
3.使用弱监督来标记大量数据 (3. Using Weak Supervision to Label Large Volumes of Data)
For many machine learning projects, sourcing and annotating large datasets takes up substantial amounts of time. In this paper, researchers from Stanford University propose a system for the automatic creation of datasets through a process called “data programming”.
对于许多机器学习项目,大型数据集的获取和注释会占用大量时间。 在本文中,斯坦福大学的研究人员提出了一种通过称为“数据编程”的过程自动创建数据集的系统。

The above table was taken directly from the paper and shows precision, recall, and F1 scores using data programming (DP) in comparison to the distant supervision ITR approach.
上表直接取自本文,与远程监管ITR方法相比,该表显示了使用数据编程(DP)的准确性,召回率和F1得分。
The proposed system employs weak supervision strategies to label subsets of the data. The resulting labels and data will likely have a certain level of noise. However, the team then removes noise from the data by representing the training process as a generative model, and presents ways to modify a loss function to ensure it is “noise-aware”.
所提出的系统采用弱监督策略来标记数据的子集。 产生的标签和数据可能会具有一定程度的噪音。 但是,该团队然后通过将训练过程表示为一个生成模型来从数据中去除噪声,并提出修改损失函数以确保其“感知噪声”的方法。
Research Paper: Data Programming: Creating Large Training Sets, Quickly
研究论文 : 数据编程:快速创建大型训练集
Authors / Contributors: Alexander Ratner, Christopher De Sa, Sen Wu, Daniel Selsam, Christopher Ré (all authors from Stanford University)
作者/撰稿人:亚历山大·拉特纳(Alexander Ratner),克里斯托弗·德萨(Christopher De Sa),吴森(Sen Wu),丹尼尔·塞尔萨姆(Daniel Selsam),克里斯托弗·雷(ChristopherRé)(斯坦福大学的所有作者)
Date Published / Last Updated: January 8, 2017
发布日期/最后更新日期: 2017年1月8日
4.如何使用半监督知识转移来处理个人身份信息(PII) (4. How to Use Semi-supervised Knowledge Transfer to Handle Personally Identifiable Information (PII))
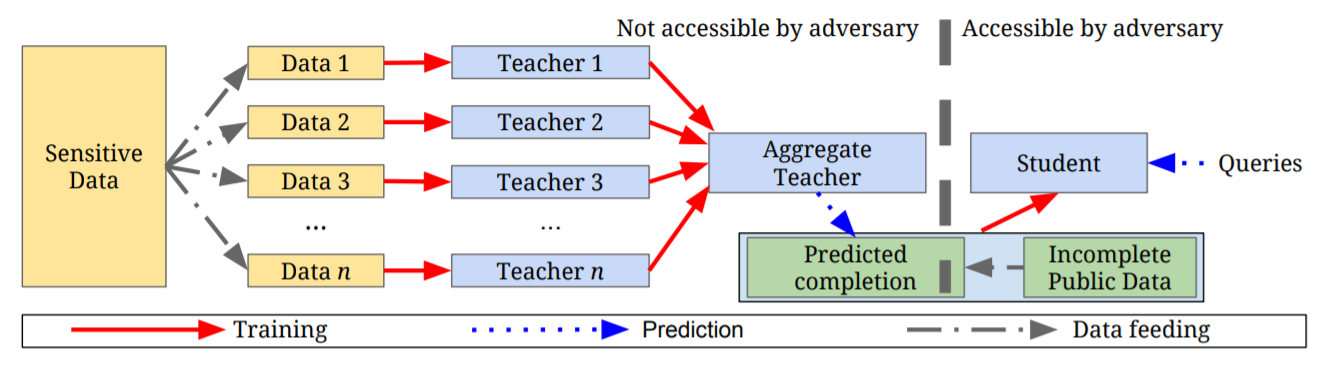
From researchers at Google and Pennsylvania State University, this paper introduces an approach to dealing with sensitive data such as medical histories and private user information. This approach, known as Private Aggregation of Teacher Ensembles (PATE), can be applied to any model and was able to achieve state-of-the-art privacy/utility trade-offs on the MNIST and SVHN datasets.
谷歌和宾夕法尼亚州立大学的研究人员介绍了一种处理敏感数据(例如病史和私人用户信息)的方法。 这种方法被称为教师合奏私人聚集(PATE),可以应用于任何模型,并且能够在MNIST和SVHN数据集上实现最新的隐私/实用性权衡。
However, as Data Scientist Alejandro Aristizabal states in his article, one major issue with PATE is that the framework requires the student model to share its data with the teacher models. In this process, privacy is not guaranteed. Therefore, Aristizabal proposes an additional step that adds encryption to the student model’s dataset. You can read about this process in his article, Making PATE Bidirectionally Private, but please make sure you read the original research paper first.
但是,正如数据科学家Alejandro Aristizabal在他的文章中指出的那样,PATE的一个主要问题是该框架要求学生模型与教师模型共享其数据。 在此过程中,不能保证隐私。 因此,Aristizabal提出了一个额外的步骤,该步骤将加密添加到学生模型的数据集中。 您可以在他的文章PATE Bidirectionally Private中阅读有关此过程的信息,但是请确保您首先阅读了原始研究论文。
Research Paper: Semi-Supervised Knowledge Transfer for Deep Learning From Private Training Data
研究论文 : 从私人培训数据进行深度学习的半监督知识转移
Authors / Contributors: Nicolas Papernot (Pennsylvania State University), Martin Abadi (Google Brain), Ulfar Erlingsson (Google), Ian Goodfellow (Google Brain), Kunal Talwar (Google Brain)
作者/贡献者: Nicolas Papernot(宾夕法尼亚州立大学),Martin Abadi(谷歌大脑),Ulfar Erlingsson(谷歌),Ian Goodfellow(谷歌大脑),Kunal Talwar(谷歌大脑)
Date Published / Last Updated: March 3, 2017
发布日期/最后更新日期: 2017年3月3日
5.用于半监督学习和转移学习的高级数据增强 (5. Advanced Data Augmentation for Semi-supervised Learning and Transfer Learning)
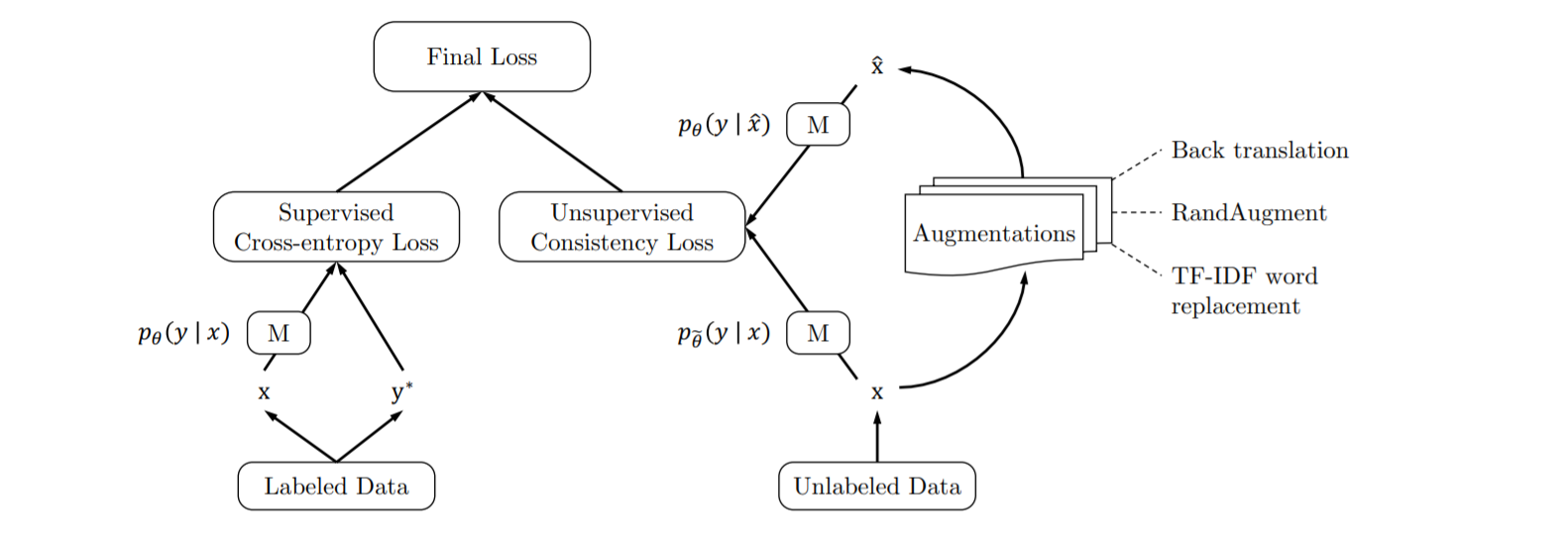
One of the largest problems facing data scientists today is getting access to training data. It can be argued that one of the biggest problems of deep learning is that most models require large amounts of labeled data in order to function with a high degree of accuracy. To help combat these issues, researchers from Google and Carnegie Mellon University have come up with a framework for training models on substantially lower amounts of data.
当今数据科学家面临的最大问题之一是获得培训数据。 可以说,深度学习的最大问题之一是,大多数模型需要大量的标记数据才能以较高的精度运行。 为了帮助解决这些问题,谷歌和卡内基梅隆大学的研究人员提出了一个框架,该框架可用于对大量数据进行模型训练。
The team proposes using advanced data augmentation methods to efficiently add noise to unlabeled data samples used in semi-supervised learning models. Amazingly, this framework was able to achieve incredible results. The team states that on the IMDB text classification dataset, their method was able to outperform state-of-the-art models by training on only 20 labeled samples. Furthermore, on the CIFAR-10 benchmark, their method outperformed all previous approaches.
该团队建议使用高级数据增强方法来将噪声有效地添加到半监督学习模型中使用的未标记数据样本中。 令人惊讶的是,该框架能够实现令人难以置信的结果。 该团队指出,在IMDB文本分类数据集上,他们的方法仅对20个带有标签的样本进行了训练,就能够胜过最新模型。 此外,在CIFAR-10基准测试中,他们的方法优于所有以前的方法。
Research Paper: Unsupervised Data Augmentation for Consistency Training
研究论文 : 用于一致性训练的无监督数据增强
Authors / Contributors: Qizhe Xie1,2 , Zihang Dai1,2 , Eduard Hovy2 , Minh-Thang Luong1 , Quoc V. Le1 (1Google Research, Brain Team, 2Carnegie Mellon University)
作者/撰稿人 :谢启哲1,2,戴子行1,2,爱德华·霍维2,Minh-Thang Luong1,Quoc V. Le1(1 Google Research,Brain Team,2卡内基梅隆大学)
Date Published / Last Updated: September 30th, 2019
发布日期/最后更新日期: 2019年9月30日
Hopefully these machine learning papers focusing on training data and data processing tasks helped you learn something new that you can apply to your own projects. For more machine learning articles please view our top stories below, and please be sure to follow me on Medium.
希望这些专注于训练数据和数据处理任务的机器学习论文可以帮助您学习可以应用于自己的项目的新知识。 有关更多机器学习的文章,请在下面查看我们的热门文章,请确保在Medium上关注我 。
Original article reposted with permission.
原始文章经许可重新发布。
翻译自: https://medium.com/datadriveninvestor/5-essential-papers-on-ai-training-data-aba8ea359f79
ai伪造论文实验数据