情感分析朴素贝叶斯
Millions of tweets are posted every second. It helps us know how the public is responding to a particular event. To get the sentiments of tweets, We can use the Naive Bayes classification algorithm, which is simply the application of Bayes rule.
每秒发布数百万条推文。 它可以帮助我们了解公众如何响应特定事件。 为了获得推文的情感,我们可以使用朴素贝叶斯分类算法,这只是贝叶斯规则的应用。
贝叶斯规则 (Bayes Rule)
Bayes rule is merely describing the probability of an event on prior knowledge of the occurrence of another event related to it.
贝叶斯规则仅是根据与之相关的另一个事件的发生的先验知识来描述事件的概率。
Then the probability of occurrence of event A given that event B has already occurred is
假设事件B已经发生,则事件A发生的概率为
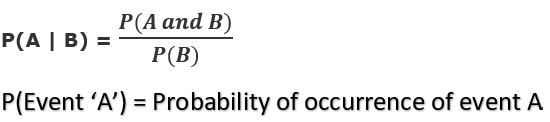
And for the probability of occurrence of event B given that event A has already occurred is
对于事件B发生的概率,假设事件A已经发生,则为
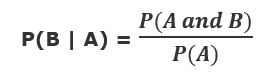
Using both these equations, we can rewrite them collectively as
使用这两个等式,我们可以将它们统一重写为
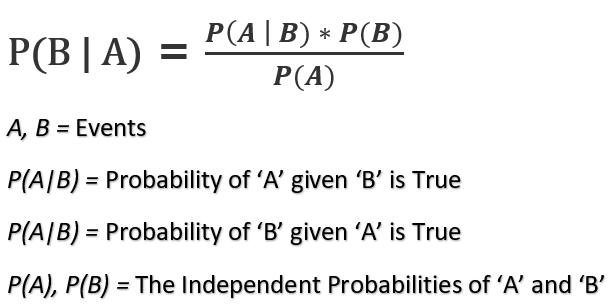
Let’s take a look at tweets and how we are going to extract features from them
让我们看一下推文以及我们如何从中提取功能
We will be having two corpora of tweets, positive and negative tweets.
我们将有两种推文,正面和负面推文。
Positive tweets: ‘I am happy because I am learning NLP,’ ‘I am happy, not sad.’
积极的推文 :“我很高兴,因为我正在学习NLP”,“我很高兴,而不是悲伤”。
Negative tweets: ‘I am sad, I am not learning NLP,’ ‘I am sad, not happy.’
负面推文 :“我很难过,我没有学习NLP”,“我很难过,不开心”。
前处理 (Preprocessing)
We need to preprocess our data so that we can save a lot of memory and reduce the computational process.
我们需要对数据进行预处理,以便节省大量内存并减少计算过程。
- Lowercase: We will convert all the text to lower case. so, that the words like Learning and leaning can be taken as same words