国内quora
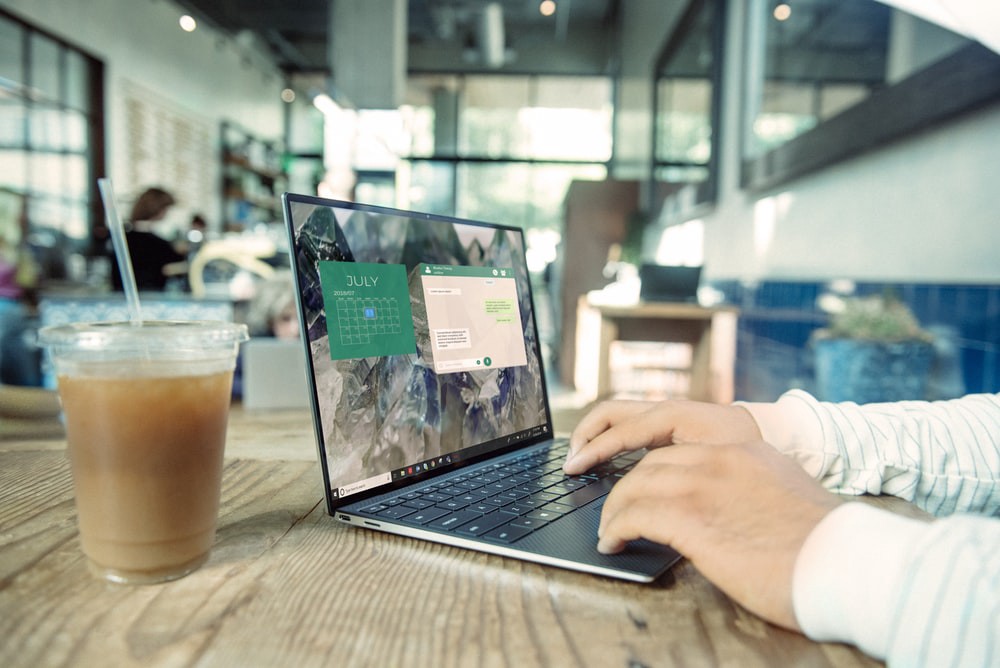
Quora insincere question classification was a challenge organized by kaggle in the field of natural language processing. The main aim the challenge was to figure out the toxic and divisive content. It is binary classification problem where class 0 represented insincere question and class 1 otherwise. This blog would specifically deal with the data modelling part.
Quora真诚的问题分类是kaggle在自然语言处理领域组织的一项挑战。 挑战的主要目的是找出有毒和分裂性的内容。 这是二进制分类问题,其中类别0表示不诚实的问题,否则类别1。 该博客将专门处理数据建模部分。
预处理: (Preprocessing:)
In the first step we shall read the data using pandas. This code snippet would read the file into a pandas data frame.
第一步,我们将使用熊猫读取数据。 此代码段会将文件读入pandas数据框。
train=pd.read_csv(‘/kaggle/input/quora-insincere-questions-classification/train.csv’)
test_df=pd.read_csv(‘/kaggle/input/quora-insincere-questions-classification/test.csv’)
sub=pd.read_csv(‘/kaggle/input/quora-insincere-questions-classification/sample_submission.csv’)
We can know the shape of the data using the shape method.
我们可以使用shape方法知道数据的形状。
Initially we would try to divide the training dataset into 2 parts which are train and validation. To do so we can take help of sklearn. The following code snippet would help us achieve it.
最初,我们尝试将训练数据集分为训练和验证两部分。 为此,我们可以寻求sklearn的帮助。 以下代码段将帮助我们实现这一目标。
train_df,val_df=train_test_split(train,test_size=0.1)
In the first step we would try to fill the question that contain null values. To do so we can use the fillna method. The code snippet below would do the same.
第一步,我们将尝试填充包含空值的问题。 为此,我们可以使用fillna方法。 下面的代码段将执行相同的操作。
train_x=train_df['question_text'].fillna('__na__').values
val_x=val_df['question_text'].fillna('__na__').values
test_x=test_df['question_text'].fillna('__na__').values
Now it is the time to choose some of the important parameters. These are embedd_size,max_features and max_len. Here embedd_size represents the word vector size of each word we are going to represent, whereas max_features tells about the number of top frequency words which we shall consider. For example if we consider max_feature to be 50000 it would imply we shall take the 50000 most occurring words into consideration while converting them into vectors. Similarly max_len would imply starting from the beginning how many words we would. For example max_len 100 would mean we shall consider the only first 100 words. The following are the parameters chosen for the same.
现在是时候选择一些重要参数了。 这些是embedd_size , max_features和max_len 。 这里的embedd_size表示我们将要表示的每个单词的单词向量大小,而max_features讲述了我们将要考虑的最高频率单词的数量。 例如,如果我们认为max_feature为50000,则意味着在将它们转换为向量时,应考虑50000个最常出现的单词。 类似地, max_len表示从头开始会有多少个单词。 例如max_len 100意味着我们将只考虑前100个字。 以下是为其选择的参数。
embedd_size=300
max_features=50000
max_len=100
Now consider the following code snippet.
现在考虑以下代码片段。
tokenizer=Tokenizer(num_words=max_features)
tokenizer.fit_on_texts(list(train_df))
train_x=tokenizer.texts_to_sequences(train_x)
val_x=tokenizer.texts_to_sequences(val_x)
test_x=tokenizer.texts_to_sequences(test_x)
Here we would take the most 50000 frequent words into account in the first line. The second line would convert each word into a unique number based on where it appears in the sentence. The text_to_sequence method in the third,fourth and fifth line would convert each sentence to the numbers. For example to convert “India won the match” we shall lookup the integer assigned to each word in the previous method fit_on_texts and change the sentence accordingly.
在第一行中,我们将考虑最多50000个常用词。 第二行将根据单词在句子中的位置将每个单词转换为唯一的数字。 第三,第四和第五行中的text_to_sequence方法会将每个句子转换为数字。 例如,要转换“ 印度赢得比赛 ”,我们将在先前的方法fit_on_texts中查找分配给每个单词的整数,并相应地更改句子。
train_x=pad_sequences(train_x,maxlen=max_len)
val_x=pad_sequences(val_x,maxlen=max_len)
test_x=pad_sequences(test_x,maxlen=max_len)
The above code snippet would ensure each sentence is converted to a particular length. This is done so that while giving this sequences in batches they would fit into a particular length. Hence it is required to pad or truncate some of the sequences.
上面的代码片段将确保将每个句子转换为特定的长度。 这样做是为了在批量分配此序列的同时,使其适合特定的长度。 因此,需要填充或截断某些序列。
造型: (Modelling:)
We shall take a bidirectional lstm in order to build this classification model. Before that we have to convert the text into numbers. We did the initial step of it in the last paragraph where each word was converted into unique integers. In the next step we shall convert the words into vectors using the keras embedding layer. We also have the option of using any pretrained word embedding but here we have chosen the embedding layer to learn the word embedding while training. Embedding layer assigns random vectors to the words initially but learns the word embedding for each as the training of the model goes on. The below snippet tells us about the whole modelling strategy.
我们将采用双向lstm来建立此分类模型。 在此之前,我们必须将文本转换为数字。 我们在最后一个段落中完成了它的第一步,其中每个单词都被转换为唯一的整数。 在下一步中,我们将使用keras嵌入层将单词转换为向量。 我们还可以选择使用任何预训练的单词嵌入,但是在这里,我们选择了嵌入层来在训练时学习单词嵌入。 嵌入层最初会为单词分配随机向量,但是随着模型的训练的进行,每个单词都将学习单词嵌入。 下面的代码段向我们介绍了整个建模策略。
inp=Input(shape=(max_len))
x=Embedding(max_features,embedd_size)(inp)
x=Bidirectional(LSTM(128,return_sequences=True))(x)
x=GlobalMaxPool1D()(x)
x=Dense(16,activation='relu')(x)
x=Dropout(0.2)(x)
x=Dense(1,activation='sigmoid')(x)
model=Model(inputs=inp,outputs=x)
model.compile(loss='binary_crossentropy',optimizer='adam',metrics=['accuracy'])
After the embedding we shall use the bidirectional lstm. Here the parameter return sequence=True implies we would like to get the output of each hidden state. The deafult one is false which means we want the output of the final state only. Similarly the GlobalMaxPool1D implies that for each sentence vector we shall take the highest value only. Dropout layer is added to deal with the overfitting. The dense layer with the sigmoid activation function will output a value between 0 and 1. The compile method builds the model where no training has been performed yet.
嵌入之后,我们将使用双向lstm。 在这里,参数return sequence = True表示我们希望获取每个隐藏状态的输出。 默认值是假的,这意味着我们只需要最终状态的输出。 类似地, GlobalMaxPool1D意味着对于每个句子向量,我们将仅取最大值。 添加了辍学层以应对过度拟合。 具有S型激活函数的密集层将输出0到1之间的值。编译方法将在尚未进行训练的情况下构建模型。
In the compile method we had only defined the architecture and initialized it. Now we have to tune the parameters so as to get optimal model. To do so we pass the training data through the model. The fit method passes the data through model and computes the loss. Also based on the loss it does the backpropagation and tunes the parameters. The following code snippet does the same.
在编译方法中,我们仅定义了架构并对其进行了初始化。 现在我们必须调整参数以获得最佳模型。 为此,我们通过模型传递训练数据。 拟合方法通过模型传递数据并计算损失。 同样基于损耗,它进行反向传播并调整参数。 以下代码段执行相同的操作。
model.fit(train_x,train_out,batch_size=256,epochs=2,validation_data=(val_x,val_out))
After training for 2 epochs the model had achieved an accuracy of 93%. We can change the parameters and do hyperparameter tuning to get a better model.
在训练了2个时期后,该模型的准确率达到了93%。 我们可以更改参数并进行超参数调整以获得更好的模型。
The performance metric for this competition is F1 score. As the dataset is imbalanced.
这项比赛的表现指标是F1分数。 由于数据集不平衡。
To predict the model we can write
为了预测模型,我们可以写
pred_y=model.predict([test_x],batch_size=256)
The whole code can be found out at https://github.com/mohantyaditya/quora-insincere-classification/blob/master/quora%20insincere.ipynb
整个代码可以在https://github.com/mohantyaditya/quora-insincere-classification/blob/master/quora%20insincere.ipynb中找到
This was a pretty basic approach to the problem. We can try out with the pre trained embedding vectors to get better result.
这是解决该问题的非常基本的方法。 我们可以尝试使用预训练的嵌入向量以获得更好的结果。
Gain Access to Expert View — Subscribe to DDI Intel
获得访问专家视图的权限- 订阅DDI Intel
国内quora