A solution to the opioid crisis has become somewhat elusive, as America continues to struggle to combat the crisis. One of the biggest hurdles is finding ways to manage a patient’s pain without creating a dependence on opioids. Another hurdle is how to manage or reduce prescription dosages for patients who already have a dependence on opioids. Two of the ways the healthcare system is attempting to overcome these hurdles are opioid risk assessments, the identification of patients at risk of misusing opioids or overdose, and opioid tapering plans, the gradual reduction of opioid dosages to curb withdrawal symptoms. Though these have proven effective, they are not foolproof. These practices can be made more accurate and helpful to healthcare providers through machine learning — a promising catalyst for such advancement.
随着美国继续努力抗击阿片类药物危机,解决阿片类药物危机的方法变得有些难以捉摸。 最大的障碍之一是找到在不产生阿片类药物依赖的情况下控制患者疼痛的方法。 另一个障碍是如何为已经依赖阿片类药物的患者管理或减少处方药剂量。 医疗保健系统试图克服这些障碍的两种方法是阿片类药物风险评估,确定有滥用阿片类药物或过量用药风险的患者以及阿片类药物逐渐减少计划,逐步减少阿片类药物剂量以遏制戒断症状。 尽管已证明这些方法有效,但并非万无一失。 通过机器学习可以使这些做法更加准确,对医疗保健提供者有所帮助,这是推动此类进步的有希望的催化剂。
Maybe for you, a solution to staying fit during quarantine has become elusive. Let’s say you found the next thing to try and stay fit: Zumba. One day, though, you trip over your coffee table during a workout and end up with a broken wrist, and you’ve never broken anything before. You find the pain to be unbearable, so, when you get to a doctor, you describe the pain as being unmatched. Consequently, you are prescribed hydrocodone, commonly known as Vicodin: a relatively potent opioid considering you have never taken opioids. You take your first dosage, and it is instant relief. Little do you know, that first pill could spell a future of opioid misuse. You take the medication, but you have work to do. The pain resurfaces, so you take another dosage before you should. What’s the worst that could happen? You continue to do this and go through the medication before your refill date. You choose to turn to other sources to ease your pain and get your medication, whether that be other healthcare providers or an illicit seller on the street. This doesn’t mean you are an addict, but these are signs of opioid use disorder (OUD): a physical and mental dependence on opioids.
也许对您而言,在隔离过程中保持健康的解决方案变得捉襟见肘。 假设您找到了下一件适合保持健康的东西:尊巴舞。 不过,有一天,您在锻炼过程中绊倒了咖啡桌,手腕骨折了,而您之前从未摔破任何东西。 您发现疼痛难以忍受,因此,去看医生时,您形容这种疼痛是无法弥补的。 因此,您需要开处方氢可酮 ,俗称维可丁:考虑到您从未服用过阿片类药物,因此是一种相对有效的阿片类药物。 您服用第一剂,立即缓解。 您几乎不知道,第一种药可能会导致滥用阿片类药物的未来。 您正在服药,但是您有工作要做。 疼痛重新出现,因此您应该先服用另一剂。 可能发生的最坏情况是什么? 您将继续执行此操作,并在补充日期之前检查药物。 您选择求助于其他来源以减轻痛苦并获得药物治疗,无论是其他医疗保健提供者还是街头的非法卖家。 这并不意味着您会上瘾,但这是阿片类药物使用障碍(OUD)的征兆:阿片类药物在身心上的依赖性。
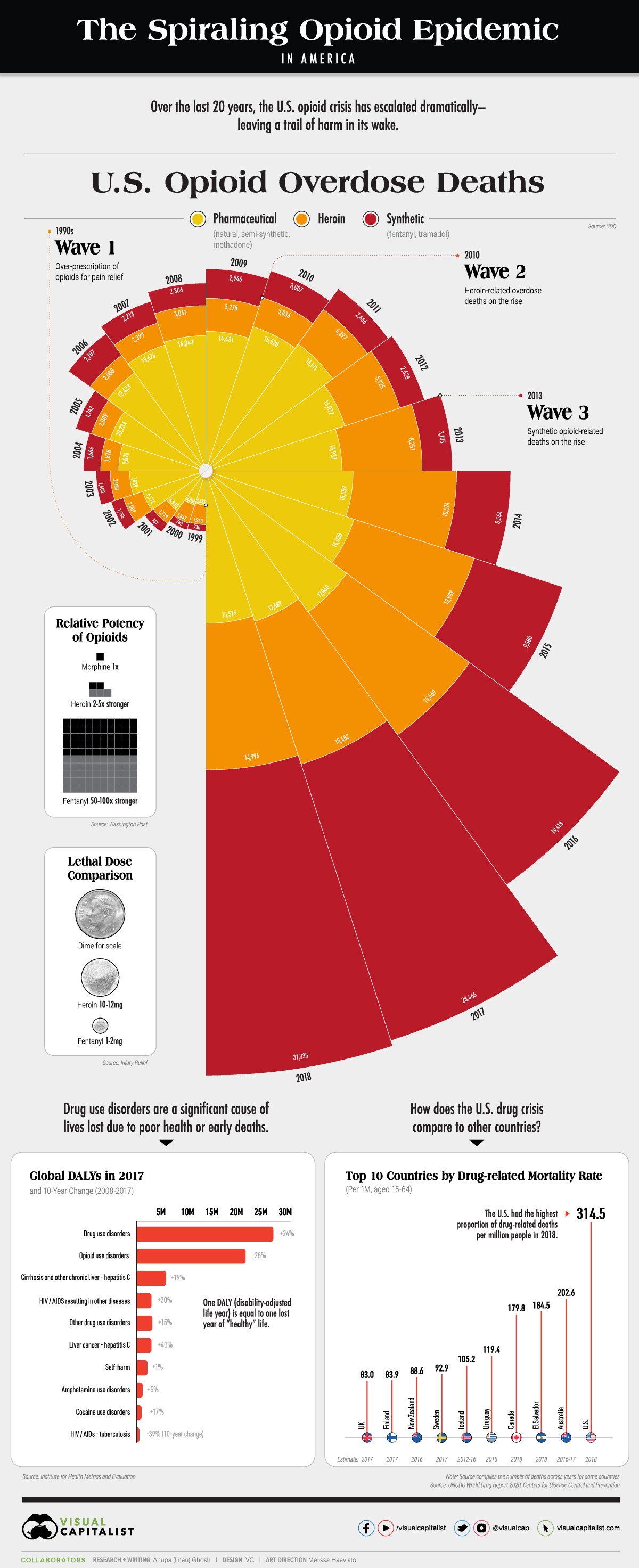
There are a couple of points where your dependence on opioids could have been avoided. One is before you were even prescribed the opioid. A person’s risk for OUD can actually be predicted by their provider: this is the job of opioid risk assessments. There are various factors that could indicate a likelihood of OUD; even just being a woman in your late 40s could mean that you are at a greater risk of developing OUD. Healthcare providers have access to a few opioid risk assessments, such as the Opioid Risk Tool (ORT), to take the known information about a patient to determine their level of risk. This is one of the places machine learning can be implemented to help patients and their providers.
在某些方面可以避免您对阿片类药物的依赖。 一种是在您未服用阿片类药物之前。 提供者实际上可以预测一个人的OUD风险:这是阿片类药物风险评估的工作。 有多种因素可以表明发生OUD的可能性。 即使只是在40多岁的时候成为女性,也可能意味着您患OUD的风险更大。 医疗保健提供者可以访问一些阿片类药物风险评估,例如阿片类药物风险工具( ORT ),以获取有关患者的已知信息来确定他们的风险水平。 这是可以实施机器学习来帮助患者及其提供者的地方之一。
Machine learning is a form of artificial intelligence that utilizes processes to allow a computer to learn from experience without much human intervention. It may seem odd to hear that a system can learn by itself, but that’s because it’s not exactly doing it all on its own: the system must be given large amounts of data to be able to “learn” effectively and, later, make “predictions” based on that data. This is similar to how a physician can use an opioid risk assessment to “predict” who may be at risk of OUD. Though machine learning and human learning are quite different, there are similarities to be found in each learning pattern. For example, toddlers see a kid riding something with wheels, and their parents tell them it is a bike. The toddler can now identify a bicycle with reasonable accuracy, and this accuracy can be improved as the child learns more about what makes a bike a bike — like handlebars and a seat. Similarly, a computer sees an amalgamation of pixels and is told they represent an image of a bike, and as it “learns” about more images of a bike, it is able to better “predict” what is a bike on its own.
机器学习是人工智能的一种形式,它利用过程使计算机可以从经验中学习,而无需过多的人工干预。 听到一个系统可以自我学习可能听起来很奇怪,但这是因为它不能完全自己完成所有工作:必须为系统提供大量数据,以便能够有效地“学习”,然后使“预测”。 这类似于医生如何使用阿片类药物风险评估来“预测”谁有OUD风险。 尽管机器学习和人类学习有很大的不同,但是每种学习模式都有相似之处。 例如,幼儿看到一个孩子骑着带轮的东西,父母告诉他们这是一辆自行车。 现在,幼儿可以识别出具有合理准确性的自行车,并且随着孩子对自行车的成因了解得更多,例如车把和座椅,可以提高这种准确性。 类似地,计算机看到像素的混合,并被告知代表自行车的图像,并且当它“了解”自行车的更多图像时,它能够更好地“预测”自己的自行车。
It’s cool that a computer can predict what is or isn’t a bike, but, to be honest, it is quite useless when it comes to the opioid crisis (there could be a connection between opioid misuse and bikes, but I’ll leave that to the scientists). A more pressing application is the implementation of machine learning algorithms to help combat the opioid crisis. A year ago, a team of researchers, headed by Dr. Lo-Ciganic and Dr. Huang and funded primarily by the National Institute of Drug Abuse, investigated the effectiveness of machine-learning algorithms at assessing opioid overdose risk in patients. The machine-learning models used were able to predict which individuals would be at a greater risk of overdose. This application of machine learning, however, fell a bit short, as it was unable to predict what patients would be at risk of OUD — which affects over 2 million people in just the U.S.
计算机可以预测出什么是自行车,这很酷,但是,老实说,当涉及阿片类药物危机时,它是毫无用处的(阿片类药物滥用与自行车之间可能存在联系,但我会离开对科学家而言)。 更加紧迫的应用是机器学习算法的实施,以帮助应对阿片类药物危机。 一年前,一个研究小组,由药物滥用研究所博士罗Ciganic和黄博士领导和资助为主, 调查在患者评估阿片类药物过量的风险的机器学习算法的有效性。 所使用的机器学习模型能够预测哪些人有更大的用药过量风险。 但是,由于无法预测哪些患者有OUD的风险,因此机器学习的这种应用略有不足-仅在美国就影响了超过200万人
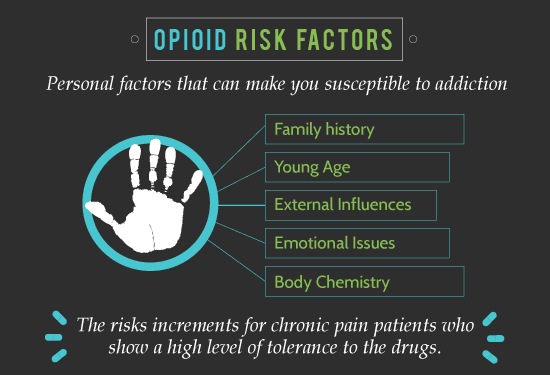
More recently, a study was conducted by the same team of researchers that focused on assessing the risk of OUD by utilizing machine learning. Both of these prediction models were significantly more accurate in correctly identifying all those who may be at greater risk than the traditional methods that are currently in place in numerous hospitals, such as the ORT. While both studies yield compelling results, the practice of using machine learning for pain management is not exactly widespread. However, what is widespread is the use of electronic health records: digital versions of a patient’s information and health history. These records hold the data that would be used by the predictive models, for a predictive model cannot make a reliable, accurate prediction without a sufficient amount of background information on a patient.
最近,同一组研究人员进行了一项研究 ,该研究的重点是通过利用机器学习来评估OUD的风险。 这两种预测模型在正确识别所有可能比那些目前在众多医院中采用的传统方法(例如ORT)中处于更大风险中的患者时,都更为准确。 尽管两项研究均取得了令人信服的结果,但使用机器学习进行疼痛控制的实践并未完全普及。 但是,普遍使用的是电子健康记录 :患者信息和健康史的数字版本。 这些记录保存了将由预测模型使用的数据,因为在没有足够的患者背景信息的情况下,预测模型无法进行可靠,准确的预测。
Let’s say that a traditional opioid risk tool, or even machine learning tool, wasn’t able to identify your risk of developing OUD. Continuing to take opioids could create an even greater dependence and tolerance to the medication, but stopping them completely could result in adverse withdrawal symptoms, such as muscle aches, anxiety, or vomiting. Luckily, your provider, or providers, have access to your electronic health record. At first, electronic health records were not meant to do much more than be records of healthcare and patient information, but the integration of various tools into these records has become a great help to providers. One of these tools is opioid tapering plans, which have been integrated into some electronic health records to allow for the automated creation and management of tapering plans. Daniel Seltzer, co-chair of the Electronic Health Record Association (EHRA)’s Opioid Crisis Task Force, opines that opioid tapering plans are “the next big frontier in this whole world of research for electronic health records.”
假设传统的阿片类药物风险工具,甚至机器学习工具,都无法识别您患上OUD的风险。 继续服用阿片类药物可能对药物产生更大的依赖性和耐受性,但是完全停止使用阿片类药物可能会导致不良的戒断症状,例如肌肉酸痛,焦虑或呕吐。 幸运的是,您的提供者可以访问您的电子健康记录。 最初,电子健康记录的作用不只是提供医疗保健和患者信息记录,还可以将各种工具集成到这些记录中,对提供者有很大帮助。 这些工具之一是阿片类药物逐渐减少计划,该计划已被集成到一些电子健康记录中,以允许自动创建和管理逐渐减少计划。 电子健康档案协会(EHRA)阿片类药物危机特别工作组的联合主席Daniel Seltzer认为,阿片类药物减量计划是“整个电子健康记录研究领域的下一个重要领域”。
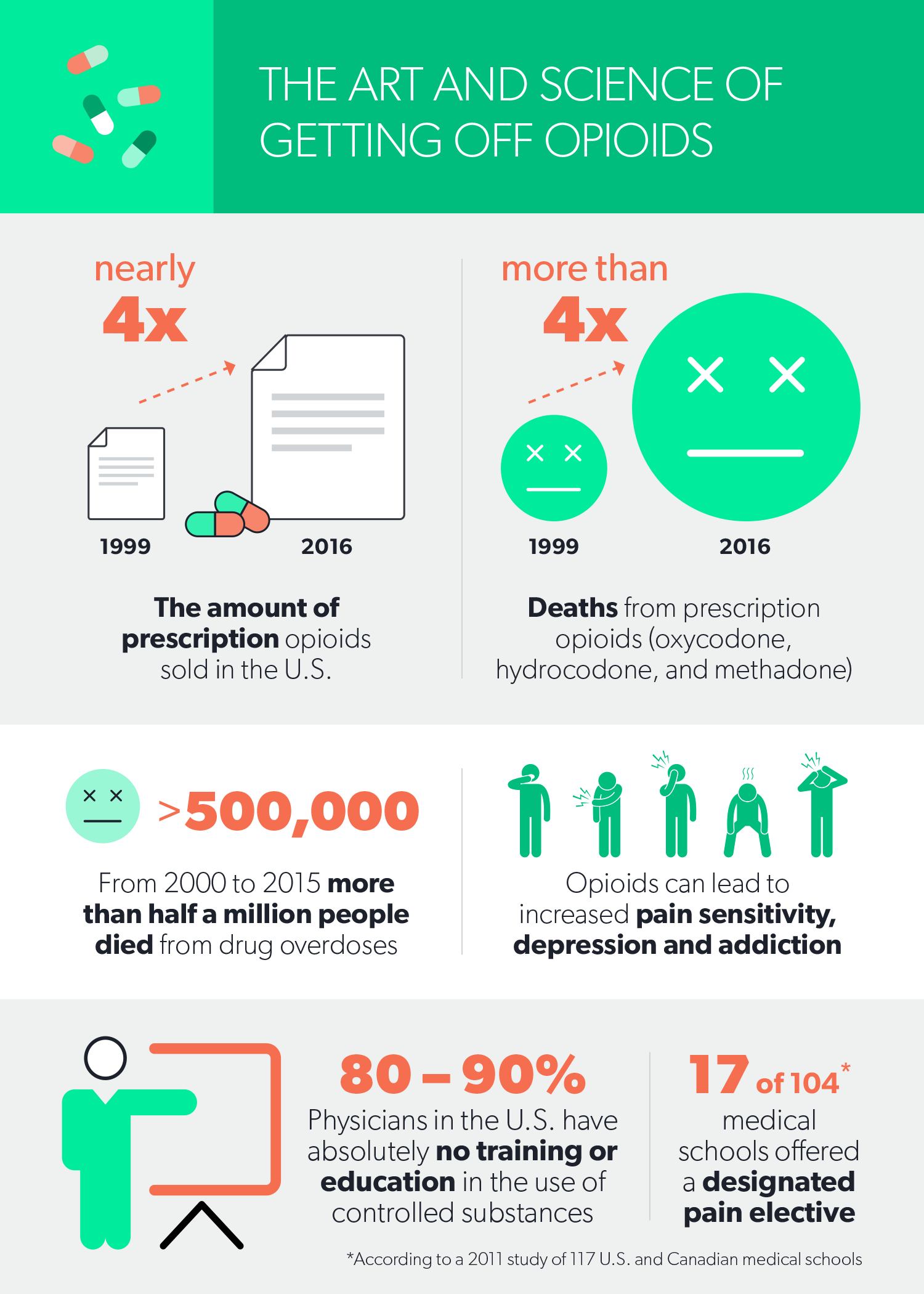
Opioid tapering plans have proven helpful for patients who are taking high doses of opioids or who misuse opioids. One way electronic health records help is by monitoring what are known as morphine milligram equivalents (MMEs); MMEs quantify the dose of an opioid by putting it in terms of morphine dosages. A common tapering plan would suggest a decrease in dosage by 10% each month. This seems simple, but creating and managing an actual schedule for a patient can be hard for a physician to do alone. For this reason, electronic health records can help with the “grunt work,” which leaves the physician more open to support the patient throughout the taper.
阿片类药物逐渐减少计划已被证明对服用大剂量阿片类药物或滥用阿片类药物的患者有帮助。 电子病历帮助的一种方法是监视所谓的吗啡毫克当量(MME)。 MME通过将吗啡剂量表示为阿片类药物来量化其剂量。 常见的渐缩计划将建议每月减少10%的剂量。 这似乎很简单,但是为医生创建和管理实际的时间表可能很难让医生独自完成。 因此,电子健康记录可以帮助完成“艰巨的工作”,从而使医生在整个锥度手术过程中更加开放地为患者提供支持。
Now you are on your way to overcoming your opioid use disorder. This is mainly attributable to your electronic health record. You may think that this is the work of your own hospital or healthcare provider, but, more likely, it is the work of electronic health record developers (most of whom are part of the EHRA). These are also the people who are leading the advancements toward predictive modeling and more tailored care for patients seeking pain management/opioid treatment. Dr. David Little, a physician advisor for the opioid management workgroup at Epic Systems, an electronic health record developer, explains how major developers have “developed the capability [. . .] to capture and process large volumes of data” in order to identify patients at risk of complications or future health conditions, and, in turn, address such possibilities before it is too late.
现在,您正在克服阿片类药物使用障碍。 这主要归因于您的电子健康记录。 您可能会认为这是您自己的医院或医疗服务提供者的工作,但更有可能是电子健康记录开发者的工作(其中大多数是EHRA的一部分)。 这些人也在引领着预测模型的发展,并为寻求疼痛处理/阿片类药物治疗的患者提供了更量身定制的护理。 电子病历开发人员Epic Systems的阿片类药物管理工作组的医师顾问David Little博士解释了主要开发人员如何“开发这种能力[。 。 “]捕获和处理大量数据”,以识别有并发症或未来健康状况风险的患者,并在为时已晚之前解决此类可能性。
To support the implementation of such predictive models and other electronic health record features related to opioid use, the EHRA created the Opioid Crisis Task Force in 2018. Despite the fact that these electronic health record developers are business competitors, members of different companies come together through the task force to accomplish their shared goal of reducing opioid abuse in America. The opioid crisis is ongoing, and overdose rates are seemingly on the rise again, as COVID-19 continues to limit patients’ access to opioid treatment. The automatization and digitalization of strategies, with the support of electronic health records, can help fight the ongoing opioid crisis, and, in time, could spell the decline of opioid overdose rates and the eventual end of the opioid crisis.
为了支持此类预测模型和其他与阿片类药物使用相关的电子健康记录功能的实施,EHRA于2018年成立了阿片类药物危机特别工作组。尽管这些电子健康记录开发人员是企业竞争对手,但不同公司的成员通过该工作队要实现他们在美国减少阿片类药物滥用的共同目标。 阿片类药物危机仍在持续,而且由于COVID-19继续限制患者使用阿片类药物的治疗, 用药过量率似乎再次上升。 在电子健康记录的支持下,策略的自动化和数字化可以帮助应对持续的阿片类药物危机,并及时解决阿片类药物过量率的下降和阿片类药物危机的最终终结。