写在前面
空间数据的巨量化的背后,是对空间处理工具的处理速度的挑战。当你试图用半小时完成一个简单的空间连接的时候,就意味着你手里的这一套算法与工具,基本已经被判了死刑。而现实则是,市面上流行的大部分空间处理工具,如ArcGIS和Geopandas,在处理速度上都广为诟病。在动辄上亿个点面的空间处理中,十分之吃力,甚至无法运行。这个时候,如何找到更快的计算方法,就十分重要了。
本文介绍了一种通过空间索引来完成点面间快速空间连接的方法,在一定程度上参考了我之前做的点线间空间连接方法:https://zhuanlan.zhihu.com/p/53992405。方法的基本思路十分简单,就是把面转化为格点,然后借助空间索引快速定位出点集间的最小距离。本文尚未涉及分布式空间计算如GeoSpark,完全基于本地Python实现,并与Geopandas的空间连接结果进行了对比。
空间格网的构建与投影
习惯使用ArcGIS的同学在听到空间格网后的第一反应应该就是Fishnet和Tessellation了,没错,他们都是非常好用的空间格网产生工具。但既然本文讨论的提速,我们就没必要再借助ArcGIS了。我们来试图自己生成一个空间格网。
初学者容易弄错的一点就是:空间格网还不容易,给我一个多边形的最大顶点与最小顶点的经纬度,然后等距插值一下不就出来了。但这其中隐藏了一个bug,那就是经纬度的等距偏移,不等于距离的等距偏移。引用一下这段话[1]:
Each degree of latitude is approximately 69 miles (111 kilometers) apart. The range varies (due to the earth's slightly ellipsoid shape) from 68.703 miles (110.567 km) at the equator to 69.407 (111.699 km) at the poles.
A degree of longitude is widest at the equator at 69.172 miles (111.321) and gradually shrinks to zero at the poles.
简而言之,经纬度是球面坐标的表达,并不是我们传统意义的平面坐标,因此在空间处理时,任何涉及到距离计算的操作,都一定要预先定义好投影坐标。投影坐标就像把地球仪摊平了,球面变成了平面,这时候各种建立在平面坐标系上面的距离计算公式也就可以使用了。
那如何确定所在的地区的投影坐标呢?在图源没有指定具体坐标系统的情况下,绝大多数我们地理坐标系都会使用WGS84,而投影坐标系都会使用UTM分区系统。
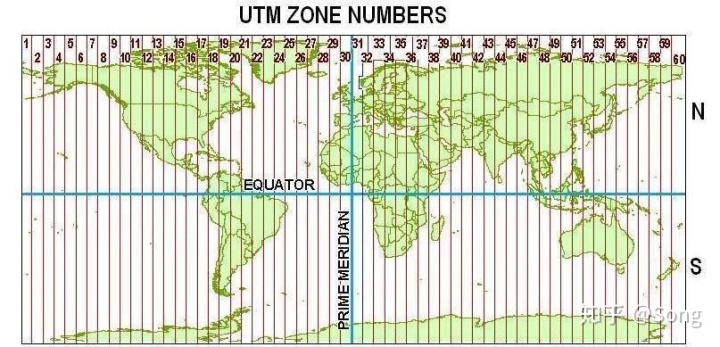
了解了投影之后,思路的确就很简单了,找到多边形的顶点经纬度后,先投影,再插值,即可得到真正意义的等距格点了,然后再基于得到的格点生成buffer即可。至于如何得到具体的UTM分区,我们也不需要去地图上对着自个比对,直接写代码即可输出对应的UTM分区了。在Geopandas中,WGS84的代号为EPSG:4326,而北半球UTM的代号为EPSG:326+分区号;南半球为EPSG:327+分区号。
import geopandas as gpd
import pandas as pd
import numpy as np
from geopandas import GeoDataFrame
from shapely.geometry import Point
import pyproj
import utm
import math
from geopandas.tools import sjoin
import datetime
import os
import matplotlib.pyplot as plt
import mapclassify
import scipy.spatial
# Get the UTM code
def convert_wgs_to_utm(lon, lat):
utm_band = str((math.floor((lon + 180) / 6) % 60) + 1)
if len(utm_band) == 1:
utm_band = '0' + utm_band
if lat >= 0:
epsg_code = '326' + utm_band # lat>0: N;
else:
epsg_code = '327' + utm_band
return epsg_code
# Read PGCOUNTY
# 'D:COVID19RNN_COVID_DataShapefileNational_County_4326.shp'
poly = gpd.GeoDataFrame.from_file(
r'D:COVID19RNN_COVID_DataShapefileNational_County_4326.shp', crs={'init': 'EPSG:4326'})
# Get the bounds
poly_bound = poly.geometry.bounds
# Get the utm code
utm_code = convert_wgs_to_utm(poly_bound['minx'][0], poly_bound['miny'][0])
# Project the lat/lon to meters
transformed_min = pyproj.transform(pyproj.Proj(init='EPSG:4326'), pyproj.Proj(init='EPSG:{0}'.format(utm_code)),
poly_bound['minx'][0], poly_bound['miny'][0]) # lon lat
transformed_max = pyproj.transform(pyproj.Proj(init='EPSG:4326'), pyproj.Proj(init='EPSG:{0}'.format(utm_code)),
poly_bound['maxx'][0], poly_bound['maxy'][0]) # lon lat
# Make a grid
Buffer_Value = 100
xmin, xmax, ymin, ymax = transformed_min[0], transformed_max[0], transformed_min[1], transformed_max[1]
xx, yy = np.meshgrid(np.arange(xmin, xmax, Buffer_Value), np.arange(ymin, ymax, Buffer_Value)) # meter
# Output
Grid_Point = GeoDataFrame({'geometry': [Point(x, y) for x, y in zip(xx.flatten(), yy.flatten())]})
Grid_Point.crs = {'init': 'EPSG:{0}'.format(utm_code)}
Grid_Point = Grid_Point.to_crs({'init': 'EPSG:4326'})
# Within
pointInPolys = sjoin(Grid_Point, poly, how='inner', op='within').reset_index(drop=True)
pointInPolys .to_file("result2.shp") # output shp to test
# Buffer
Buffer_S = pointInPolys.buffer(Buffer_Value / 2, cap_style=3).reset_index(drop=True) # cap_style=3
Buffer_S = Buffer_S.reset_index()
Buffer_S.columns = ['B_ID', 'geometry']
Buffer_S = gpd.GeoDataFrame(Buffer_S, geometry='geometry')
Buffer_S.crs = {'init': 'EPSG:{0}'.format(utm_code)}
Buffer_S = Buffer_S.to_crs({'init': 'EPSG:4326'})
在代码的最后,我们把得到的结果导出成shp文件在ARCGIS中打开,可见这正是我们需要的格点了。
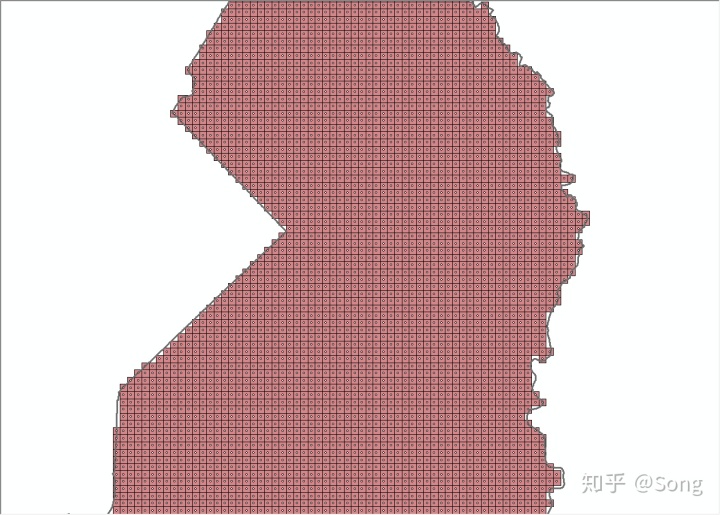
空间索引与空间连接
在得到空间格点之后,点面的空间连接实际就变成了点点的最短距离。在这方面的研究则十分成熟了[2],但依然要注意,点点的空间距离必须基于投影坐标计算。换而言之,我们的经纬度除了用来绘图,其余场景应当尽量避免使用才对。
在这里我们继续采用CKDtree。在计算之前,先确保把所有的经纬度全部投影到对应的坐标系中去了:
# The points of grids
pointInPolys = pointInPolys.to_crs({'init': 'EPSG:{0}'.format(utm_code)})
pointInPolys['lon'] = pointInPolys.geometry.apply(lambda p: p.x)
pointInPolys['lat'] = pointInPolys.geometry.apply(lambda p: p.y)
ref_points = pointInPolys[['lon', 'lat']].values
# The points we need to join with spatial grids
table = pd.read_pickle('MDPG_device_new_data_%04s' % date)
# table = table.sample(10000)
table['Time_index'] = round((table['DateTime'] - Today).dt.total_seconds() / (Time_Bandwith * 60))
points = np.dstack(
pyproj.transform(pyproj.Proj(init='EPSG:4326'), pyproj.Proj(init='EPSG:{0}'.format(utm_code)),
table['Longitude'].values, table['Latitude'].values))[0] # lon lat
dist_ckd1, indexes_ckd1 = scipy.spatial.cKDTree(ref_points).query(points)
table['Min_Distance'] = dist_ckd1
table['B_ID'] = indexes_ckd1
# plt.plot(table['Min_Distance1'] - table['Min_Distance'], 'o')
table = table[table['Min_Distance'] <= Buffer_Value / 2]
我们把结果与Geopandas的sjoin对比一下,Geopandas虽然也引入了空间索引(rtree)[3],但速度依然是硬伤,这与其本身的空间数据结构有很大关系。
TjInPolys = gpd.GeoDataFrame(table, geometry=gpd.points_from_xy(x=table.Longitude, y=table.Latitude))
TjInPolys.crs = {'init': 'EPSG:4326'}
TjInPolys = sjoin(TjInPolys, Buffer_S, how='inner', op='within').reset_index(drop=True)
print(len(TjInPolys))
TjInPolys = TjInPolys.merge(table, on=['Cuebiq_ID', 'DateTime'], how='outer')
plt.plot(TjInPolys['B_ID'], TjInPolys['B_ID_right'], 'o', alpha=0.1,color='k')
先看空间索引的散点图,两者完全一致,说明通过空间格点+CKDTREE可以完美实现空间连接的功能。
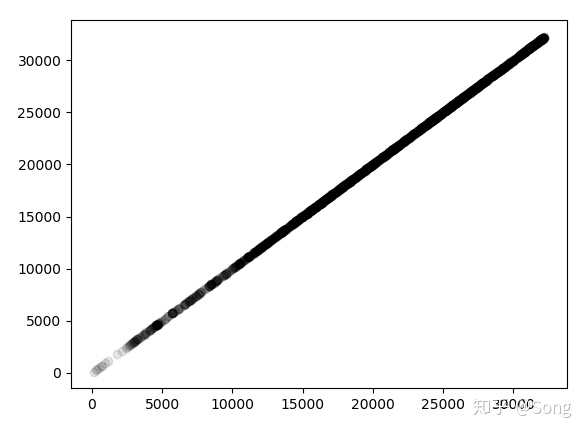
再对比一下运行速度,我测试了100000个sample,500000个sample,1000000个sample与32265个面的空间连接速度(后者为Geopandas的sjoin):
- 100000个:0.437787s;4.85921s
- 500000个:1.578362s;21.562538s
- 1000000个:3.45322s;42.703092s
可见速度提升了至少10倍以上,且会随着样本量的增大,提升速度更为明显。
最后,画个图展示一下对每个格网计数点位后的效果(其实就很类似热力图)。可见我们的格点基于点位个数上色后,几乎复盘了城市的道路系统,且存在道路等级越高颜色越深(观测到的点位信息越多)的趋势。
Encounter = table.groupby(['Time_index', 'B_ID'])['Cuebiq_ID'].nunique().reset_index()
# Count and output
Slice = Encounter.groupby(['index_right']).sum()['Cuebiq_ID'].reset_index()
Slice.columns = ['B_ID', 'Count']
Buffer_S_N = Buffer_S.merge(Slice, on='B_ID', how='left')
Buffer_S_N = Buffer_S_N.fillna(0)
fig, ax = plt.subplots(nrows=1, ncols=1, figsize=(12, 6))
# scheme = mapclassify.Quantiles(Buffer_S_N['Count'], k=6)
Buffer_S_N.plot(column='Count', ax=ax, legend=True, scheme='quantiles', k=5,
legend_kwds=dict(loc=4, frameon=False), linewidth=0.1, edgecolor='white',
cmap='OrRd')
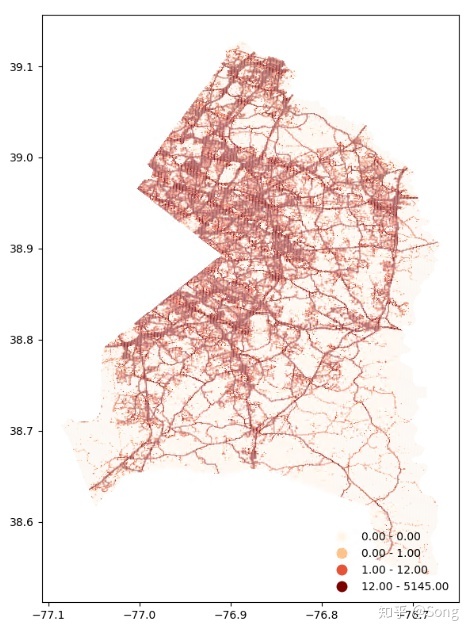
参考
- ^1 https://gis.stackexchange.com/questions/142326/calculating-longitude-length-in-miles
- ^2 https://zhuanlan.zhihu.com/p/53992405
- ^3 https://gis.stackexchange.com/questions/175228/geopandas-spatial-join-extremely-slow