1、联通ColaB
目前方向:图像拼接融合、图像识别 联系方式:jsxyhelu@foxmail.com


2、运行最基础mnist例子,并且打印图表结果
# https://pypi.python.org/pypi/pydot
#!apt-get -qq install -y graphviz && pip install -q pydot
#import pydot
from __future__ import print_function
import keras
from keras.datasets import mnist
from keras.models import Sequential
from keras.layers import Dense, Dropout, Flatten
from keras.layers import Conv2D, MaxPooling2D
from keras import backend as K
from keras.utils import plot_model
import matplotlib.pyplot as plt
batch_size = 128
num_classes = 10
epochs = 12
#epochs = 2
# input image dimensions
img_rows, img_cols = 28, 28
# the data, shuffled and split between train and test sets
(x_train, y_train), (x_test, y_test) = mnist.load_data()
if K.image_data_format() == 'channels_first':
x_train = x_train.reshape(x_train.shape[0], 1, img_rows, img_cols)
x_test = x_test.reshape(x_test.shape[0], 1, img_rows, img_cols)
input_shape = (1, img_rows, img_cols)
else:
x_train = x_train.reshape(x_train.shape[0], img_rows, img_cols, 1)
x_test = x_test.reshape(x_test.shape[0], img_rows, img_cols, 1)
input_shape = (img_rows, img_cols, 1)
x_train = x_train.astype('float32')
x_test = x_test.astype('float32')
x_train /= 255
x_test /= 255
print('x_train shape:', x_train.shape)
print(x_train.shape[0], 'train samples')
print(x_test.shape[0], 'test samples')
# convert class vectors to binary class matrices
y_train = keras.utils.to_categorical(y_train, num_classes)
y_test = keras.utils.to_categorical(y_test, num_classes)
model = Sequential()
model.add(Conv2D(32, kernel_size=(3, 3),
activation='relu',
input_shape=input_shape))
model.add(Conv2D(64, (3, 3), activation='relu'))
model.add(MaxPooling2D(pool_size=(2, 2)))
model.add(Dropout(0.25))
model.add(Flatten())
model.add(Dense(128, activation='relu'))
model.add(Dropout(0.5))
model.add(Dense(num_classes, activation='softmax'))
model.compile(loss=keras.losses.categorical_crossentropy,
optimizer=keras.optimizers.Adadelta(),
metrics=['accuracy'])
#log = model.fit(X_train, Y_train,
# batch_size=batch_size, nb_epoch=num_epochs,
# verbose=1, validation_split=0.1)
log = model.fit(x_train, y_train,
batch_size=batch_size,
epochs=epochs,
verbose=1,
validation_data=(x_test, y_test))
score = model.evaluate(x_test, y_test, verbose=0)
print('Test loss:', score[0])
print('Test accuracy:', score[1])
plt.figure('acc')
plt.subplot(2, 1, 1)
plt.plot(log.history['acc'],'r--',label='Training Accuracy')
plt.plot(log.history['val_acc'],'r-',label='Validation Accuracy')
plt.legend(loc='best')
plt.xlabel('Epochs')
plt.axis([0, epochs, 0.9, 1])
plt.figure('loss')
plt.subplot(2, 1, 2)
plt.plot(log.history['loss'],'b--',label='Training Loss')
plt.plot(log.history['val_loss'],'b-',label='Validation Loss')
plt.legend(loc='best')
plt.xlabel('Epochs')
plt.axis([0, epochs, 0, 1])
plt.show()

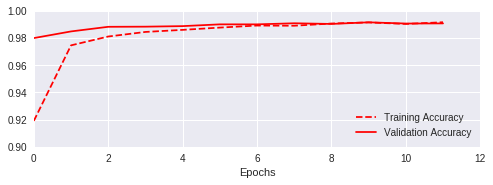
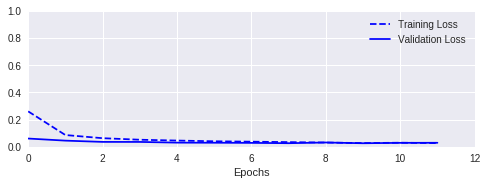
3、两句修改成fasion模式
# https://pypi.python.org/pypi/pydot
#!apt-get -qq install -y graphviz && pip install -q pydot
#import pydot
from __future__ import print_function
import keras
from keras.datasets import fashion_mnist
from keras.models import Sequential
from keras.layers import Dense, Dropout, Flatten
from keras.layers import Conv2D, MaxPooling2D
from keras import backend as K
from keras.utils import plot_model
import matplotlib.pyplot as plt
batch_size = 128
num_classes = 10
epochs = 12
#epochs = 2
# input image dimensions
img_rows, img_cols = 28, 28
# the data, shuffled and split between train and test sets
(x_train, y_train), (x_test, y_test) = fashion_mnist.load_data()
if K.image_data_format() == 'channels_first':
x_train = x_train.reshape(x_train.shape[0], 1, img_rows, img_cols)
x_test = x_test.reshape(x_test.shape[0], 1, img_rows, img_cols)
input_shape = (1, img_rows, img_cols)
else:
x_train = x_train.reshape(x_train.shape[0], img_rows, img_cols, 1)
x_test = x_test.reshape(x_test.shape[0], img_rows, img_cols, 1)
input_shape = (img_rows, img_cols, 1)
x_train = x_train.astype('float32')
x_test = x_test.astype('float32')
x_train /= 255
x_test /= 255
print('x_train shape:', x_train.shape)
print(x_train.shape[0], 'train samples')
print(x_test.shape[0], 'test samples')
# convert class vectors to binary class matrices
y_train = keras.utils.to_categorical(y_train, num_classes)
y_test = keras.utils.to_categorical(y_test, num_classes)
model = Sequential()
model.add(Conv2D(32, kernel_size=(3, 3),
activation='relu',
input_shape=input_shape))
model.add(Conv2D(64, (3, 3), activation='relu'))
model.add(MaxPooling2D(pool_size=(2, 2)))
model.add(Dropout(0.25))
model.add(Flatten())
model.add(Dense(128, activation='relu'))
model.add(Dropout(0.5))
model.add(Dense(num_classes, activation='softmax'))
model.compile(loss=keras.losses.categorical_crossentropy,
optimizer=keras.optimizers.Adadelta(),
metrics=['accuracy'])
#log = model.fit(X_train, Y_train,
# batch_size=batch_size, nb_epoch=num_epochs,
# verbose=1, validation_split=0.1)
log = model.fit(x_train, y_train,
batch_size=batch_size,
epochs=epochs,
verbose=1,
validation_data=(x_test, y_test))
score = model.evaluate(x_test, y_test, verbose=0)
print('Test loss:', score[0])
print('Test accuracy:', score[1])
plt.figure('acc')
plt.subplot(2, 1, 1)
plt.plot(log.history['acc'],'r--',label='Training Accuracy')
plt.plot(log.history['val_acc'],'r-',label='Validation Accuracy')
plt.legend(loc='best')
plt.xlabel('Epochs')
plt.axis([0, epochs, 0.9, 1])
plt.figure('loss')
plt.subplot(2, 1, 2)
plt.plot(log.history['loss'],'b--',label='Training Loss')
plt.plot(log.history['val_loss'],'b-',label='Validation Loss')
plt.legend(loc='best')
plt.xlabel('Epochs')
plt.axis([0, epochs, 0, 1])
plt.show()

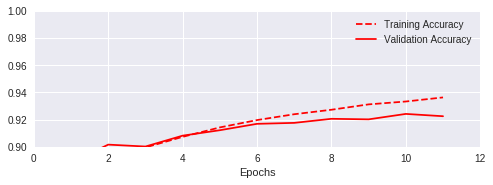
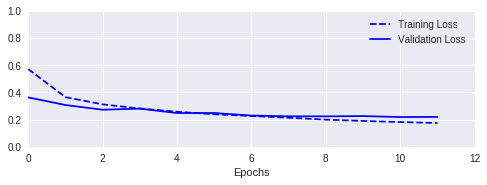
4、VGG16&Mnist
5、VGG16迁移学习
目前方向:图像拼接融合、图像识别 联系方式:jsxyhelu@foxmail.com