AMiner平台由清华大学计算机系研发,拥有我国完全自主知识产权。平台包含了超过2.3亿学术论文/专利和1.36亿学者的科技图谱,提供学者评价、专家发现、智能指派、学术地图等科技情报专业化服务。系统2006年上线,吸引了全球220个国家/地区1000多万独立IP访问,数据下载量230万次,年度访问量超过1100万,成为学术搜索和社会网络挖掘研究的重要数据和实验平台。
AMiner平台:https://www.aminer.cn
导语:EMNLP,自然语言处理经验方法会议(Conference on Empirical Methods in Natural Language Processing),是由国际语言学会(ACL)下属的SIGDAT小组主办的自然语言处理领域的顶级国际会议,也是自然语言算法的A类会议。EMNLP2020共审阅论文3359篇,接收754篇,接收率为22.4%。
Transformer由论文《Attention is All You Need》提出,现在是谷歌云TPU推荐的参考模型。论文相关的Tensorflow的代码可以从GitHub获取,其作为Tensor2Tensor包的一部分。哈佛的NLP团队也实现了一个基于PyTorch的版本,并注释该论文。
根据AMiner-EMNLP2020词云图和论文可以看出,Transformer在本次会议中也有许多不凡的工作,下面我们一起看看Transformer主题的相关论文。
论文链接:https://www.aminer.cn/pub/5e9d72b391e0117173ad2c33?conf=emnlp2020
作者:Liu Liyuan, Liu Xiaodong, Gao Jianfeng, Chen Weizhu, Han Jiawei
简介:
Transformers (Vaswani et al, 2017) have led to a series of breakthroughs in various deep learning tasks (Devlin et al, 2019; Velickovic et al, 2018).
They do not contain recurrent connections and can parallelize all computations in the same layer, improving effectiveness, efficiency, and scalability.
The authors conduct a comprehensive analysis in theoretical and empirical manners to answer the question: what complicates Transformer training
论文链接:https://www.aminer.cn/pub/5dca89783a55ac77dcb01f30?conf=emnlp2020
作者:Wieting John, Neubig Graham, Berg-Kirkpatrick Taylor
简介:
Learning useful representations of language has been a source of recent success in natural language processing (NLP).
The authors focus on learning semantic sentence embeddings in this paper, which play an important role in many downstream applications.
Since they do not require any labelled data for fine-tuning, sentence embeddings are useful for a variety of problems right out of the box.
Semantic similarity measures have downstream uses such as fine-tuning machine translation systems (Wieting et al, 2019a)
论文链接:https://www.aminer.cn/pub/5e7345fd91e011a051ebf819?conf=emnlp2020
作者:Desai Shrey, Durrett Greg
简介:
Neural networks have seen wide adoption but are frequently criticized for being black boxes, offering little insight as to why predictions are made (Benitez et al, 1997; Dayhoff and DeLeo, 2001; Castelvecchi, 2016) and making it difficult to diagnose errors at test-time.
The authors evaluate the calibration of two pre-trained models, BERT (Devlin et al, 2019) and RoBERTa (Liu et al, 2019), on three tasks: natural language inference (Bowman et al, 2015), paraphrase detection (Iyer et al, 2017), and commonsense reasoning (Zellers et al, 2018)
These tasks represent standard evaluation settings for pretrained models, and critically, challenging out-ofdomain test datasets are available for each.
Such test data allows them to measure calibration in more realistic settings where samples stem from a dissimilar input distribution, which is exactly the scenario where the authors hope a well-calibrated model would avoid making confident yet incorrect predictions
论文链接:https://www.aminer.cn/pub/5f7fe6d80205f07f68973153?conf=emnlp2020
作者:Goro Kobayashi, Tatsuki Kuribayashi, Sho Yokoi, Kentaro Inui
简介:
Transformers (Vaswani et al, 2017; Devlin et al, 2019; Yang et al, 2019; Liu et al, 2019; Lan et al, 2020) have improved the state-of-the-art in a wide range of natural language processing tasks.
The attention mechanism computes an output vector by accumulating relevant information from a sequence of input vectors.
It assigns attention weights to each input, and sums up input vectors based on their weights.
Attention computes each output vector yi ∈ Rd from the corresponding pre-update vector yi ∈ Rd and a sequence of input vectors X = {x1, .
论文链接:https://www.aminer.cn/pub/5f6c762f91e0119671e8597f?conf=emnlp2020
作者:Jaemin Cho, Jiasen Lu, Dustin Schwenk, Hannaneh Hajishirzi, Aniruddha Kembhavi
简介:
The past year has seen a spate of BERT-style (Devlin et al, 2019) transformer-based architectures (Lu et al, 2019; Chen et al, 2019; Li et al, 2019) proposed for vision-and-language tasks
These models are typically pre-trained on large image captioning corpora, extending ideas from masked language modeling to mask both the image and text modalities and produce state of the art results on a variety of vision and language tasks including visual question answering, visual grounding and image retrieval.
LXMERT consists of two types of encoders: single-modality encoders for each modality and a cross-modality encoder using bi-directional cross attention to exchange information and align entities across the modalities
论文链接:https://www.aminer.cn/pub/5f7fe6d80205f07f689733fe?conf=emnlp2020
作者:Jindřich Libovický, Alexander Fraser
简介:
State-of-the-art neural machine translation (NMT) models operate almost end-to-end except for input and output text segmentation.
Training character-level Transformer S2S models (Vaswani et al, 2017) is more complicated because the selfattention size is quadratic in the sequence length.
The authors observe that training a character-level model directly from random initialization suffers from instabilities, often preventing it from converging.
The authors' character-level models show slightly worse translation quality, but have better robustness towards input noise and better capture morphological phenomena.
The authors' approach is important because previous approaches have relied on very large transformers, which are out of reach for much of the research community
更详细了解EMNLP2020论文,可以关注公众号或者链接直达EMNLP2020专题,最前沿的研究方向和最全面的论文数据等你来~
扫码了解更多EMNLP2020会议信息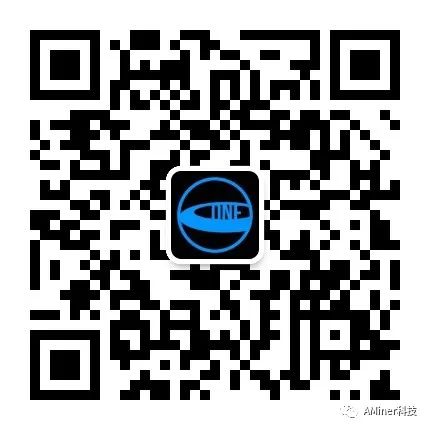
阅读原文,直达“EMNLP2020”会议专题,了解更多会议论文!