Strengths, weaknesses, and parameters
In principle, there are two important parameters to the KNeighbors classifier: the
number of neighbors and how you measure distance between data points. In practice,
using a small number of neighbors like three or five often works well, but you should
certainly adjust this parameter. Choosing the right distance measure is somewhat
beyond the scope of this book. By default, Euclidean distance is used, which works
well in many settings.
One of the strengths of k-NN is that the model is very easy to understand, and often
gives reasonable performance without a lot of adjustments. Using this algorithm is a
good baseline method to try before considering more advanced techniques. Building
the nearest neighbors model is usually very fast, but when your training set is very
large (either in number of features or in number of samples) prediction can be slow.
When using the k-NN algorithm, it’s important to preprocess your data (see Chap‐
ter 3). This approach often does not perform well on datasets with many features
(hundreds or more), and it does particularly badly with datasets where most features
are 0 most of the time (so-called sparse datasets).
So, while the nearest k-neighbors algorithm is easy to understand, it is not often used
in practice, due to prediction being slow and its inability to handle many features.
The method we discuss next has neither of these drawbacks.
KNN:Strengths, weaknesses, and parameters
最新推荐文章于 2021-03-01 00:10:18 发布
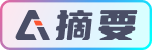